- 1Department of Communication and Media Studies, Universidad Carlos III de Madrid, Getafe, Madrid, Spain
- 2Institute on Gender Studies, Universidad Carlos III de Madrid, Getafe, Madrid, Spain
- 3Department of Electronic Technology, Universidad Carlos III de Madrid, Leganés, Spain
- 4Department of Audiovisual Communication and Advertising, Universidad Rey Juan Carlos, Fuenlabrada, Spain
Audiovisual communication is greatly contributing to the emerging research field of affective computing. The use of audiovisual stimuli within immersive virtual reality environments is providing very intense emotional reactions, which provoke spontaneous physical and physiological changes that can be assimilated into real responses. In order to ensure high-quality recognition, the artificial intelligence (AI) system must be trained with adequate data sets, including not only those gathered by smart sensors but also the tags related to the elicited emotion. Currently, there are very few techniques available for the labeling of emotions. Among them, the Self-Assessment Manikin (SAM) devised by Lang is one of the most popular. This study shows experimentally that the graphic proposal for the original SAM labelling system, as devised by Lang, is not neutral to gender and contains gender biases in its design and representation. Therefore, a new graphic design has been proposed and tested according to the guidelines of expert judges. The results of the experiment show an overall improvement in the labeling of emotions in the pleasure–arousal–dominance (PAD) affective space, particularly, for women. This research proves the relevance of applying the gender perspective in the validation of tools used throughout the years.
Introduction
The last decades have witnessed a growing interest in the multisensorial and multimodal aspects of science and technology, the integration of the measurement of emotion through the use of smart sensors being one of the emerging research lines in fields such as communication, engineering, and psychology among others. Affective computing is based on the study, analysis, and interpretation of human emotional reactions by means of artificial intelligence (AI; Picard, 1995; Picard et al., 2001), which requires the usage of complete databases where not only the measurements from different sensors are compiled rigorously but also the tags of the experimented emotions. These tags can be unconstrained or previously predefined. The predefined ones can be discrete—chosen from a finite, predefined set of emotions—or continuous, within a predefined affective space, such as the tridimensional pleasure–arousal–dominance (PAD) space (Fontaine et al., 2016), where the experimented emotion is represented via numerical values on a Likert scale in the dimensions of pleasure, arousal and dominance. In any case, the tags must always be gathered while the different emotions are being elicited in volunteers via various external stimuli.
The most used scientific databases for the study of emotions—MANHOB (Soleymani et al., 2012) and DEAP (Koelstra et al., 2012)—use the Self-Assessment Manikin (SAM) designed by Lang (1980) and Hodes et al. (1985) in the 1980s, first as a computerised, interactive graphical interface tool, although a manual version of it was later made. In fact, this non-verbal pictorial assessment technique has generally been adopted for mapping emotions in a three-dimensional space (PAD), according to the levels of pleasure (P), arousal (A), and dominance (D) every emotion draws out of the person.
The SAM technique has been consolidated throughout the years in the scientific community as a globally reliable system to classify emotions. It provides a well-defined measure with strong psychometric properties (Lang, 1980; Bradley and Lang, 1994; Leen-Feldner et al., 2008; Olatunji et al., 2009; Soares et al., 2013; Bilsky et al., 2018). For example, in their study, Zaki and Ochsner (2015) confirm that the manikins allow people to express their emotional reactions beyond linguistic barriers or discrete labels, leveraging their empathy with the figures’ expressions when observing and contemplating the image or drawing.
The SAM system provides three independent scales—PAD—associated with the emotional response to external stimuli. Each scale contains five similar figures with different expressions:
• The first scale (valence/pleasure) ranges from positive sensations to negative feelings. The farthest figure on the left shows a smile, while the one farthest to the right displays a worried/sad expression.
• The second scale (arousal/excitement) measures from the highest states of excitement to calmness. The farthest figure on the left seems ready to explode, while the one on the opposite side looks calm or asleep.
• The third scale, related to dominance, corresponds to the ability to control the intensity of the emotion experimented by the subject (Verma and Tiwary, 2015); it presents a small human figure in the square, growing from left to right, where it can be seen outside of the square because of its size.
Through these images, the person can mark any figure or space between two figures with an “x” to indicate the closest emotion to the elicited one.
For the most part, SAMs have suffered variations in the sequential order of the figures in the scales of valence and arousal, being displayed from negative to positive feelings in the case of valence and from calmness to excitement in arousal (Koelstra et al., 2012; Miranda-Correa et al., 2018). This variation in the figures’ sequential order must be considered for future comparisons with results from different research papers published.
The manikins have also suffered aesthetical modifications in the figures’ design (Koelstra et al., 2012; Miranda-Correa et al., 2018), up to the point of proposing the use of avatars instead of manikins (Sonderegger et al., 2016). Nonetheless, these modifications have not been validated through experimental research to the best of our knowledge, nor have they considered sociocultural or gender biases.
In this context, keeping in mind that one of the main objectives of this study is the validation of aesthetic modifications of the manikins, cultural and gender biases should be taken into consideration in the same way as the contents of video clips used to cause emotional reactions in order to generate audiovisual databases—the UC3M4Safety database for Spain (Blanco-Ruiz et al., 2021a,b) or Emotional Film for Asian culture (Deng et al., 2017). Gender and cultural differences have also been confirmed (Gantiva et al., 2011; Moltó et al., 2013) in the International Affective Picture System (IAPS; Lang et al., 2008), which includes over 1,000 pictures that represent a set of normative emotional stimuli for experimental research about attention and emotions.
The identification with human-like figures is a key concept in understanding and explaining the processes and effects that the stimuli provoke in the subjects while the experiments are being conducted. Through the figures, many emotions felt during direct encounters in personal experiences are recalled, activating what is known as autobiographical memory (Cohen, 2001; Sainz-de-Baranda et al., 2021b).
The different experiments in emotion recognition have detected that, in addition to individual differences in empathising with others (Lockwood et al., 2017; Israelashvili et al., 2019; Blanco-Ruiz et al., 2020; Sainz-de-Baranda et al., 2021a), there are also cultural, linguistic, sexual and age differences (Hagemann et al., 1999; Trommsdorff et al., 2016; Di Girolamo et al., 2019; Ge et al., 2019; Grégoire and Greening, 2020) that should be addressed and adapted so that every subject can reach a greater empathy with the audiovisual speeches being studied. In this sense, recent studies from feminist technoscience studies have highlighted that digital technologies and AI have biases in terms of gender, sex, job, class, ethnicity, and (dis)ability among others (Sumartojo et al., 2016; Hicks, 2017; Dunbar-Hester, 2019; Thaler, 2022).
Gender1 analysis of the world around us, and thus of technology, shows that from its design to its operation, it is not gender neutral (Haraway, 1988; Harding, 1991; Wajcman, 2006; Zafra, 2011). Examples, such as the design of autonomous cars with a gender perspective to correct inequalities in the design of the traditional belt (Saleh et al., 2022), differences in cardiovascular rehabilitation (Kentner and Grace, 2017) or the John–Jennifer effect (Moss-Racussin et al., 2012), are evidence of the need for this shift towards gender sensitivity. However, this perspective must be complemented by the intersectional perspective (Crenshaw, 1991). Recent studies on the effects of AI algorithms, such as the studies by Buolamwini and Gebru (2018), Cirillo et al. (2020), Noble (2018), and Nurock (2020) among others, point out that not only gender biases are reproduced but also those of race, class, or age.
In Europe, the European Commission (2020) has incorporated the gender perspective and the intersectional perspective into research and innovation content in the Horizon Europe framework programme, with AI being one of the key areas. Examples of this line of work include projects such as VITAPATCH in Austria, which are developing a multifunctional data patch for vital and movement monitoring in everyday environments, where its researchers are integrating knowledge on feminist technoscience into the technology design process. In the case of Spain, the EMPATÍA-CM project is working to generate automatic detection mechanisms to protect victims of gender-based violence in situations of danger, and from its beginnings, it has incorporated the gender and victim perspective into its development. As Tannenbaum et al. (2019) point out; taking a gender-sensitive view improves science and technology.
In this context, and considering that one of the main objectives of this work is the validation of aesthetic modifications of the manikins, cultural and gender biases should be taken into consideration.
Materials and methods
The initial hypothesis of this research was that the tools designed and traditionally used to measure emotions, and therefore train the intelligent systems used in affective computing, were not gender neutral. For this reason, they required a methodological revision from the gender studies perspective to produce a more equal, inclusive, and diverse science.
The aim of this study was to validate aesthetic modifications to the SAMs that serve in tagging emotions within the PAD space. This question arose when the multidisciplinary UC3M4Safety team raised the need to generate an audiovisual database—the UC3M4Safety database (Blanco-Ruiz et al., 2021a,b)—to elicit emotions through audiovisual stimuli and launch an intelligent system with the ability to determine the emotional state of a person (San-Segundo et al., 2021) known as Bindi (Miranda et al., 2021). In this sense, this work focused on analysing possible gender biases in the labelling system and thus avoiding their effects in emotion recognition. It is important to note that the labelling system conditioned the resulting intelligent system because the latter is based on supervised learning.
In this section, the different aspects of the methodology followed by this research are detailed (Ortega-Toro et al., 2008). First, the protocol, the participants, and the design of the different experiments conducted are explained and, finally, the instrument of reference is shown (Supplementary material).
Protocol
In the design of questionnaires for emotional self-labelling, we have used a stepping stone of those questionnaires that are currently used in scientific databases devoted to studying emotions and that use audiovisual stimuli of different natures to elicit emotions: FilmStim (Schaefer et al., 2010), MANHOB (Soleymani et al., 2012), DEAP (Koelstra et al., 2012), and the Emotional Film database for Asian culture (Deng et al., 2017). These are among the most used and referenced ones. All of them use the SAM tool as the emotion labelling procedure in the PAD space. It is worth noting that, despite its use in these and other publications within the field, more research on the PAD model is still needed to conceive it as a solid and proved emotional dimensional model (Bakker et al., 2014). Thus, this work claims to deepen this kind of research and deals specifically with the gender bias problem within this field. To this end, the protocol followed is based on the three following phases (Figure 1):
• The first phase was aimed at acquiring the validity of the content and the form of the survey (Table 1). To this end, the questionnaire that included the SAMs with the original aesthetic designed by Lang (1980) was sent to a group of expert judges (16 women and 14 men).
• The second phase consisted of the interpretation of each of the expert judges’ answers, after which the original aesthetic of the manikins was redesigned (Table 2).
• In the third phase, a two-step experiment was designed to confirm or discard the improvement in labelling between Lang’s SAMs and those designed by the UC3M4Safety team (UC3M4Safety’s SAMs), namely:
1. Asking the expert judges to label 12 basic emotions—described in the “Instrument” section, Table 3. This labeling has been used as the reference test (gold standard) in order to compare them with the labels provided by the sample.
2. Conducting an experiment where a sample of persons, divided into two groups, use both models of the SAMs under comparison to label a set of audiovisual stimuli (with emotional content); each group uses the two models of the SAMs in a different order to avoid biases.
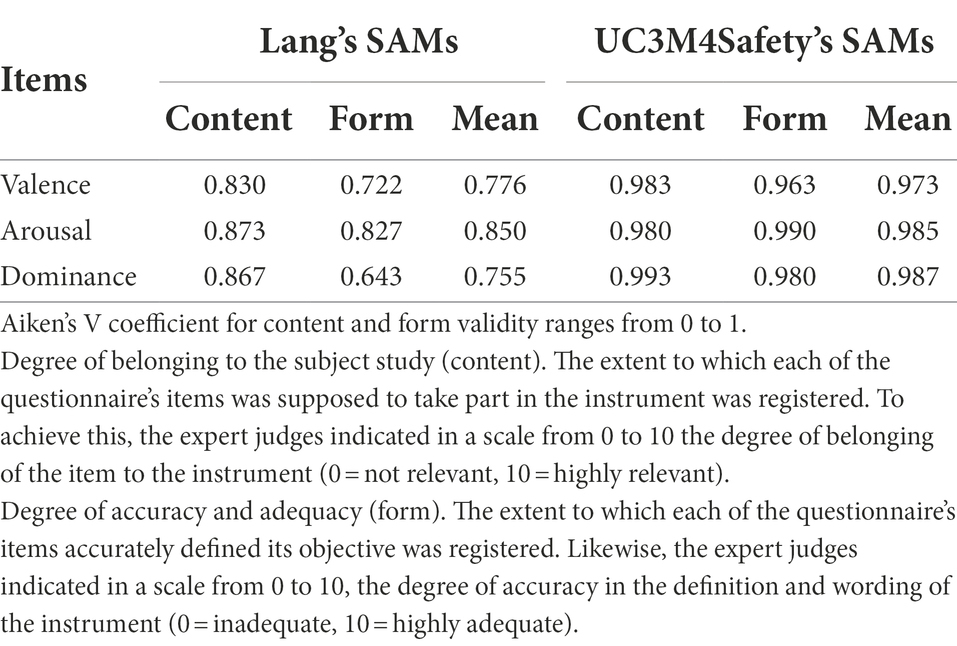
Table 1. Quantitative assessment issued by the expert judges about the Self-Assessment Manikins (Lang’s vs. UC3M4Safety’s).
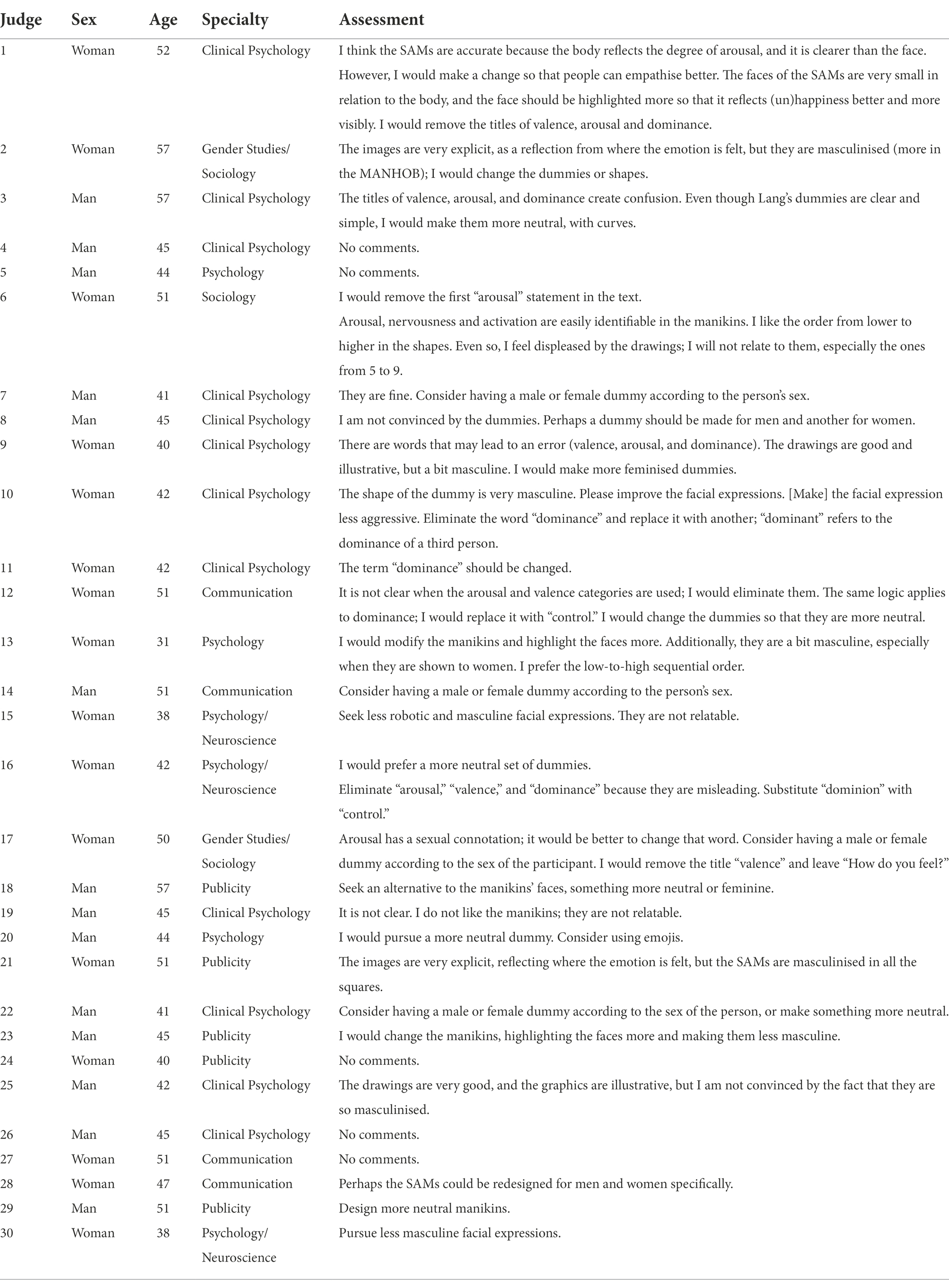
Table 2. Qualitative assessment issued by the expert judges about Self-Assessment Mankins of Lang (1985).
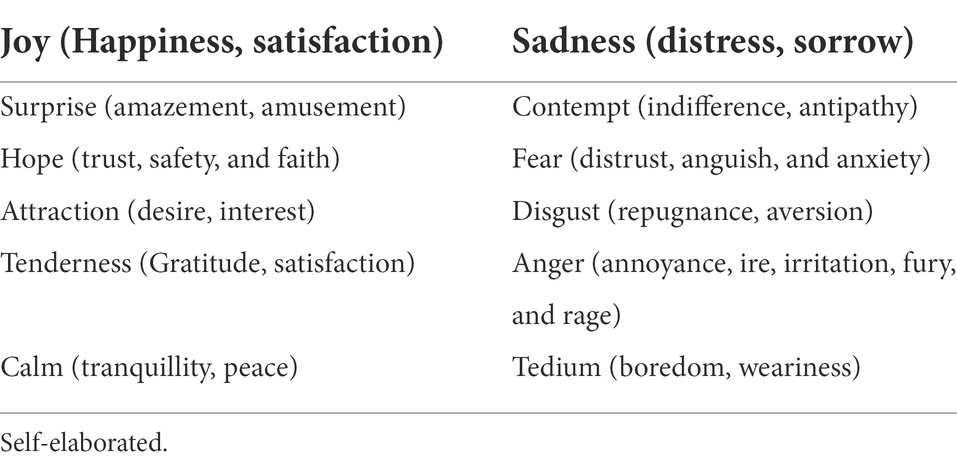
Table 3. Classification of discrete emotions in the UC3M4Safety database (Blanco-Ruiz et al., 2021a,b).
The results validate both test A (Lang) and test B (UC3M4Safety) with the gold standard.
Sample
In the three stages of the protocol, 30 expert judges—16 women and 14 men—took part in this experiment, out of which 16 were female researchers in the fields of communication, publicity, sociology, psychology, and gender studies, and the remaining 14 were male clinical psychologists and neuropsychologists. All of them had wide professional experience (over 6 years) and knowledge of gender perspective due to their profession or tuition. The age of the participants ranged between 38 and 57 years old. All participants were Spanish speakers from the Ibero-American countries. These expert judges were asked to assess the validity of the content and the form of both manikin models (SAM Lang/SAM UC3M4Safety, Figure 2), as well as to label 12 discrete emotions selected with the SAM UC3M4Safety model (as described in the “Instrument” section, Table 3). This labeling was used as a reference test in the last phase of the experiment. The sampling method was non-probabilistic, snowball sampling. The expert judges participated voluntarily. They were informed in advance of the aims of the study and the treatment of the data collected, and they had as much time as they considered necessary.
In the third phase (2), in order to obtain the information about the labelling comparison of both manikin models (Figure 2), a sample of 282 people (151 women and 131 men) was recruited via an intentional sampling among students and professors in advertising and marketing studies (bachelor’s and master’s degrees in 2020/21 and 2021/22 academic years) from universities in the region of Madrid. The sample was between 20 and 52 (32.14 ± 9.09) years old. Previously, all were informed of the study’s purpose and the treatment of the data collected. Only those who voluntarily agreed to participate in the experiment were recruited.
Before the online questionnaires were disseminated (through the Google Form platform), all participants received a lesson on measuring emotions through audiovisual stimuli and the different variables included in the SAM labelling procedure (valence, arousal, and dominance).
Afterward, those who agreed to participate voluntarily completed the questionnaire. All people were Spanish-speaking or fluent in Spanish (a prerequisite for evaluating the video clips that formed part of the sample).
The survey was conducted individually via each person’s personal electronic devices. It was distributed during the months of October 2021 to February 2022. The average response time was 30 min.
Design
As indicated in the procedure description, section “Protocol”, the study of the validity of the questionnaires that included the SAMs was conducted in the first phase, taking the “validity of the content” as the degree to which a test represented adequately its mission or objective (Wiersma, 2001; Thomas and Nelson, 2007; Ortega-Toro et al., 2008).
In order to reach optimal levels of content validity in the questionnaire designed for the collection of discrete tags (discrete emotions) and continuous tags (PAD space represented by SAM), the technique of the expert judges (Pedrosa et al., 2013) was used. To that end, these judges were asked to assess different aspects of the initial information, the measurement scale, and the questionnaire items and to perform a global assessment of each (Wiersma, 2001; Ortega-Toro et al., 2008). This process was carried out in two phases: first, Lang’s SAMs were assessed, and then UC3M4Safety’s SAMs, following the guidelines obtained in the first phase. Regarding each item of the instrument, the judges were asked to indicate the:
1. Degree of belonging to the subject study (content). The extent to which each item of the questionnaire was supposed to take part in the instrument was registered. To achieve this, the expert judges indicated in a scale from 0 to 10 the degree of belonging of the item to the instrument (0 = not relevant, 10 = highly relevant).
2. Degree of accuracy and adequacy (form). The extent to which each of the questionnaire’s items accurately defined its objective was registered. Likewise, the expert judges indicated in a scale from 0 to 10 the degree of accuracy in the definition and wording of the instrument (0 = inadequate, 10 = highly adequate).
3. Global assessment of each item.
In the third phase, as described in the “Protocol” section, the experiment was designed to measure the validity of the labelling of the new manikins (SAM UC3M4Safety) and compare them with Lang’s SAM. The experiment was proposed and designed to check if the new manikins (SAM UC3M4Safety) improved the labelling procedure, leveraging the results for both genders and bringing them closer to the “golden” labels. The spirit of the experiment stemmed from the proposal by Ortega-Toro et al. (2008). The phases of the experiment were:
1. First of all, the expert judges established the references for the 12 basic emotions in the PAD tridimensional space (valence, arousal, and dominance). These basic emotions were tedium, joy, disgust, attraction, contempt, hope, tenderness, anger, fear, surprise, calm, and sadness, as described in the “Instrument” section (Table 3). Emotions were balanced between positive and negative emotions.
2. Second of all, the experiment was designed so that every participant performed two tests using Lang’s SAM with a change in the sequential order as proposed by MANHOB (Soleymani et al., 2012) and DEAP (Koelstra et al., 2012) and recommended by the experts. Additionally, the UC3M4Safety SAMs were designed following the recommendations of the experts. The participants assessed each video in the three PAD dimensions, marking an “x” on each of the five figures or in any of the spaces between them, resulting in a score ranging from 1 (minimal pleasure, minimal activation, and minimal control) to 9 (maximum pleasure, maximum activation, and maximum control) per dimension.
Both questionnaires were completed by 282 participants (151 women and 131 men). The measurements were separated in time by 1 week, and they were performed in practically identical circumstances (Baumgartner, 2000).
Twelve video clips were assessed in each questionnaire, which had been previously tagged with the 12 selected basic emotions (Blanco-Ruiz et al., 2020). The videos used, one for each target emotion, were extracted from the UC3M4Safety database.2 Two groups were created to alternate the original manikins with the new designs in order to avoid labelling biases due to the sequential order in which they were presented.
3. Finally, the responses of the participants were analysed in three aspects:
a. Comparison of the discrete labeling of the participants with pre-tags associated with the video clips (Blanco-Ruiz et al., 2020) and between the participants for both questionnaires
b. Consistency analysis measured by the intraclass correlation coefficient (ICC) of the continuous PAD labelling of both models of manikins (Lang’s SAM and UC3M4Safety’s SAM) for the labelling of the 12 intraclass and interevaluator basic emotions, using as a reference test the one established by the expert judges
c. All of this included an analysis of the gender differences between men and women in the discrete and continuous labeling with both models, Lang’s SAM and UC3M4Safety’s SAM. To do so, reliability was defined (Thomas and Nelson, 2007; Ortega-Toro et al., 2008) as the repeatability of a measurement.
Instrument
The reference instrument—a questionnaire for the labeling of the elicited emotion after viewing an audiovisual stimulus (see Supplementary Material)—was elaborated by the UC3M4Safety research team for the creation of an audiovisual database (Blanco-Ruiz et al., 2021a,b) and its future use to build an emotional response database capable of measuring physical (voice audio) and physiological variables (heart rate, skin temperature and conductivity, electromyogram, and breathing). The labelling questionnaire of elicited emotions via audiovisual stimuli consisted of a brief introduction in which the usage, the way to answer the items, the definition on the scale, and the aim of the study among others were explained. Subsequently, various sets of questions were asked about emotional response and the 12 pre-tagged audiovisual stimuli with the 12 basic emotions (Supplementary Material) were displayed to participants.
The list of emotions for this study (Table 3) was obtained from the coincidences in the Ekman studies (Ekman, 1992, 1999; Ekman and Cordaro, 2011), Izard (2016), Mauss and Robinson (2009), and Plutchik (2001), taking into account the variables used in previous audiovisual databases, such as FilmStim (Schaefer et al., 2010), MANHOB (Soleymani et al., 2012), DEAP (Koelstra et al., 2012), and Emotional Film for Asian culture (Deng et al., 2017), while incorporating the contributions from Ekman (1999, 2016) and the work of Robinson (2008) among others, in which any emotion can be represented in a positive/constructive or negative/destructive way.
Statistical analysis
The statistical analysis of data was conducted using RStudio® (RStudio, Boston, MA, United States). First, within the scope of calculating the content validity made by expert judges, Aiken’s V test (Penfield and Giacobbi, 2004; Ortega-Toro et al., 2008) was used. Afterwards, in order to know the reliability of the categorical variables (discrete emotions), Kappa coefficient of Fleiss (1971) was calculated following the reference values from Altman (1991). It was an adaptation of Cohen’s Kappa for evaluating the level of agreement between two or more raters. It can be expressed as follows: kappa(κ) = (Po-Pe)/(1-Pe), where Po is the observed agreement and Pe is the expected agreement.
For the continuous variables (PAD indicators), the ICC (Conroy and Metzler, 2003; Correa-Rojas, 2021) was calculated. R functions kappam.fleiss and icc from irr package were used.
Results
Expert judges: Content validity of the SAMs and PAD reference values
The quantitative assessment performed by the expert judges provided data about the validity of the content and the shape of Lang’s SAM model, which signalled an Aiken’s V of 0.85 in the best case (Table 1). Aiken’s V values that were similar or greater than 0.8 were found both in the content of valence (0.830), arousal (0.873), and dominance (0.867). However, in terms of shape, only arousal (0.873) was higher than 0.8. Valence (0.722) and dominance (0.643) did not cross this threshold. These results showed a low assessment of the initial information.
The qualitative analysis (Table 2) provided by the expert judges contributed relevant information about the design of a new version of the SAMs: SAM UC3M4Safety.
After analysing the assessments, it was concluded that the gender biases were present in Lang’s SAMs, especially in the case of dominance (the degree of control over the emotional reaction to a stimulus), alluding to the fact that the representation was very masculine, and the lines and expressions were dominant, which can be detrimental when working in emotional identification with a gender perspective.
After this result, the design of the SAMs was reviewed following the experts’ guidelines, creating a seemingly more neutral model (Figure 2), and the terms used in the instructions given to the participants were also reviewed. Afterwards, the expert judges were asked once again to quantitatively assess the items that integrated the instrument, including their degree of relevance and that of precision and adequacy, as well as a global assessment of the instrument itself. The outcomes of the items related to UC3M4Safety’s SAMs demonstrated a high assessment of the final information (Table 1).
In order to establish the reference values (Table 4; Figure 3) that allow the comparisons with the outcomes of the participants, the expert judges were asked to deliver the reference values for the valence, arousal, and dominance variables for each of the 12 basic emotions (Table 3) that represented the 12 basic audiovisual stimuli chosen from the UC3M4Safety audiovisual database (Blanco-Ruiz et al., 2021a,b). In Figure 3, the gold standard representation of these 12 emotions is presented in three-dimensional PAD space, which places every emotion in a low-medium-high level of excitement, pleasure, and dominance.
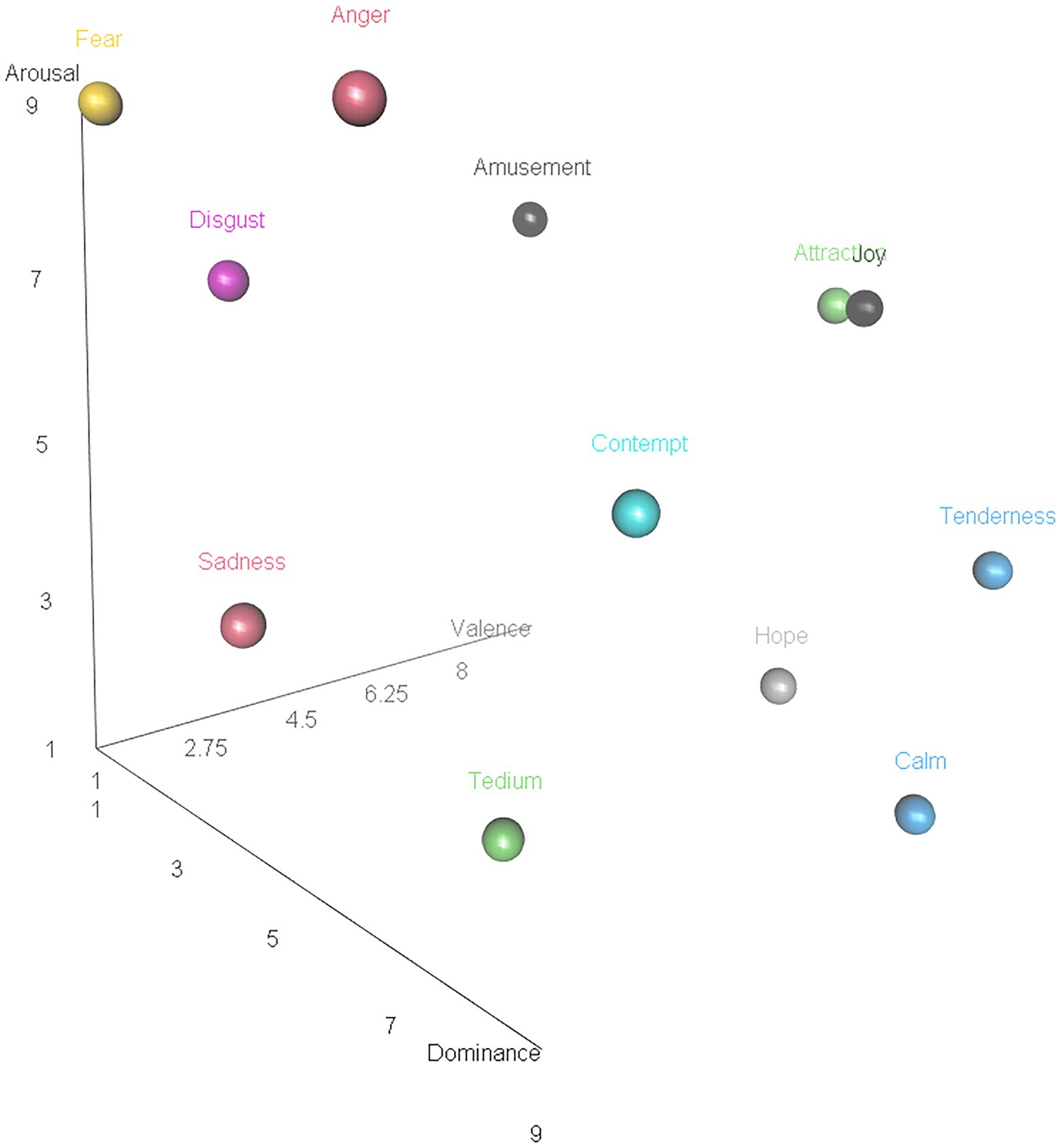
Figure 3. Representation in the pleasure–arousal–dominance space of the reference values established by the expert judges (gold standard). The colours are just to help to identify which point represents each emotion. This representation presents the gold standard in the three-dimensional pleasure–arousal–dominance (PAD) space and places each emotion in a low-medium-high level of excitement, pleasure, and dominance.
Experiment results
Validity and consistency of the discrete-labeling emotions
With the intent of confirming the agreement between the 12 emotions under study (Table 3) that represented the 12 previously tagged audiovisual stimuli (Blanco-Ruiz et al., 2021a,b) and those reported by the participants, a study was conducted using Kappa coefficient of Fleiss (1971). This coefficient measured the degree of correlation among raters of the nominal categories when the same samples were evaluated. The global results showed indices between 0.841 and 0.97 (Table 5) with practically no variation (delta). These results confirmed that the audiovisual stimuli, independent of the assessment system of manikins, generated an emotion in a unique fashion.
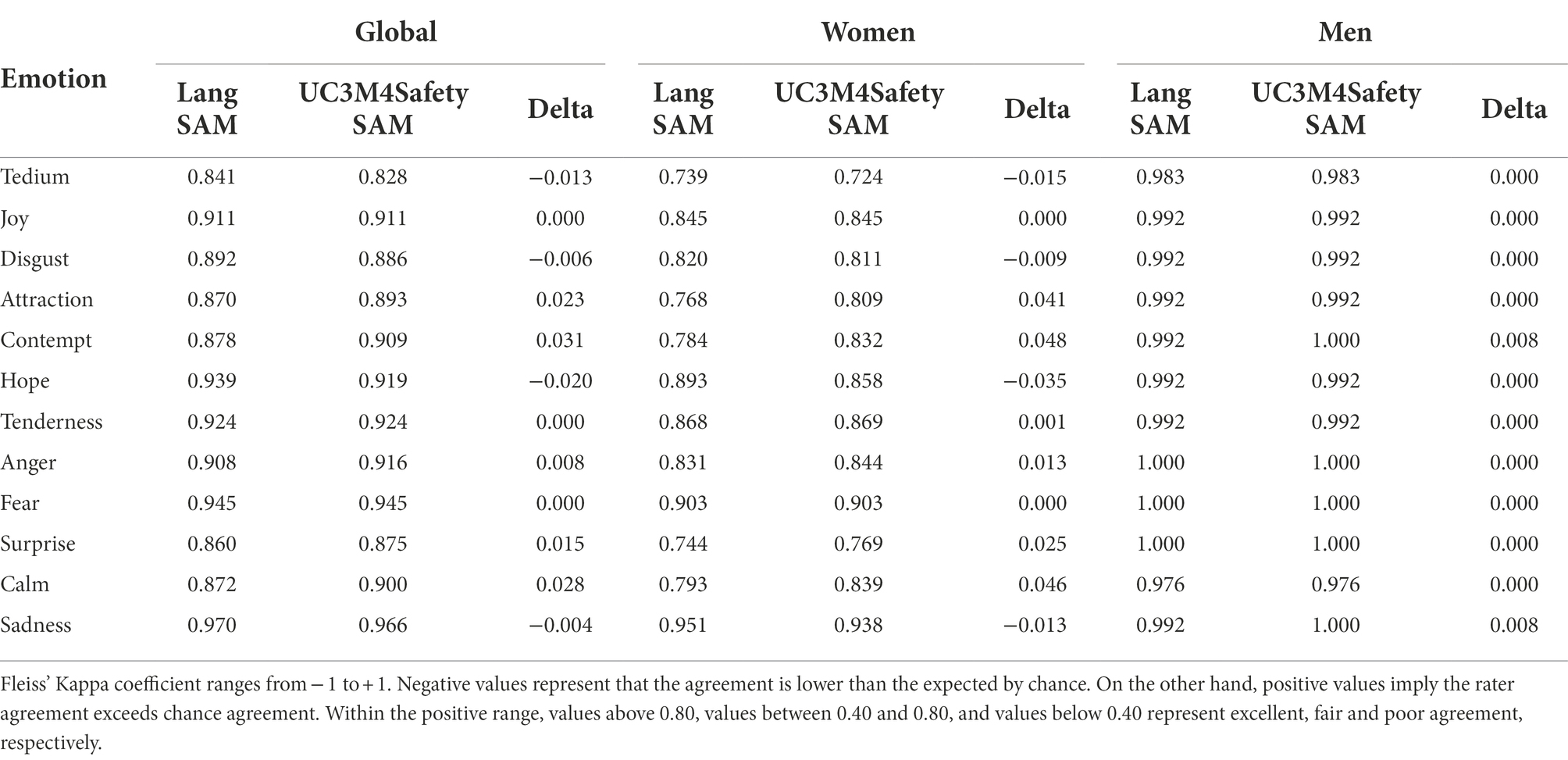
Table 5. Fleiss’ Kappa index for the measurement of consistency of experienced discrete emotions with both Self-Assessment Manikin models.
From a gender perspective, we observed that men obtained results with almost no variation (delta) and sustained Kappa index values between 0.97 and 1, that is, they showed practically perfect agreement. Women obtained a Kappa index higher than 0.7, which is a good level of agreement. However, this result confirmed that women have greater variability than men. An improvement was observed in the discrete labelling for women and, to a lesser extent, for men as well when the UC3M4Safety SAMs were used in the questionnaires to classify the experienced emotions.
Validity and consistency of emotions of the continuous labeling (pleasure–arousal–dominance)
Once the existence of a high level of agreement between the participants when labelling using discrete emotions was confirmed, the consistency of the continuous tags used for every emotion by the participants was analysed. This analysis considered intraclass and interassessor consistency, that is, if there was a variation in the measurements made by the instrument about the same topic in the same conditions. For this purpose, the ICC was used with the single-rating, absolute-agreement, Two-Way Mixed Effects Model (Table 6). The results corroborated the changes that were taking place in the continuous labelling (PAD) from Lang’s model to UC3M4Safety’s model.
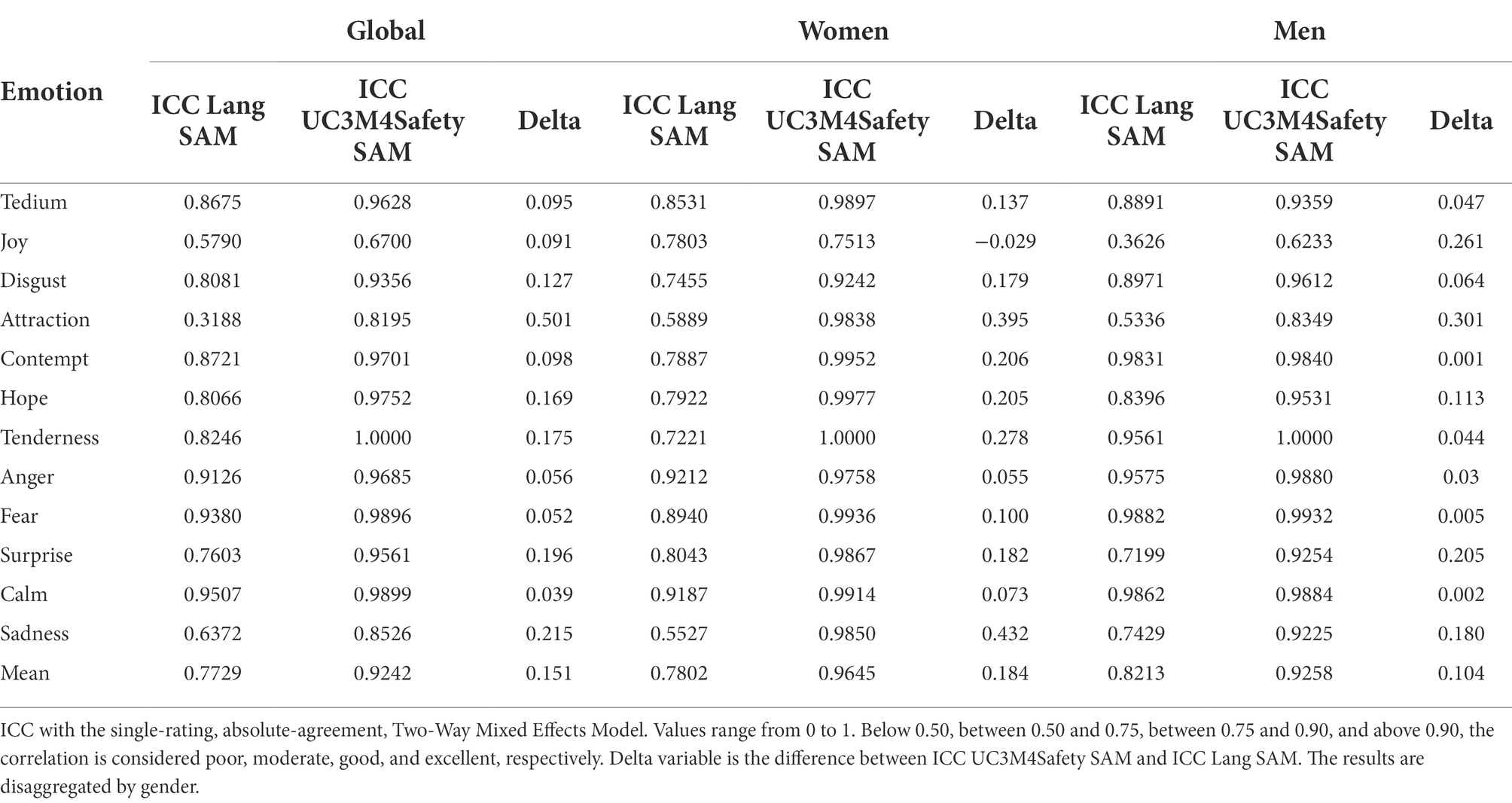
Table 6. Assessment of the intraclass pleasure–arousal–dominance for each emotion with both Self-Assessment-Manikin models.
Afterwards, for every emotion provided by the expert judges, agreement with the reference test (golden test) was evaluated (Table 7) in an independent manner for every participant (Figure 7), utilising the ICC index with the single-rating, absolute-agreement, Two-Way Random-Effects Model for each of the labelling methods. The results showed an increase in consistency and agreement between the data corresponding to UC3M4Safety’s SAMs, increasing the ICC to 0.21, 0.22, or 0.23 in the emotions of joy, attraction and surprise, respectively. Additionally, due to that greater agreement, it could be observed that the position of the emotions in the PAD space was more closely adjusted to the one reported by the expert judges, and had a lower standard deviation.
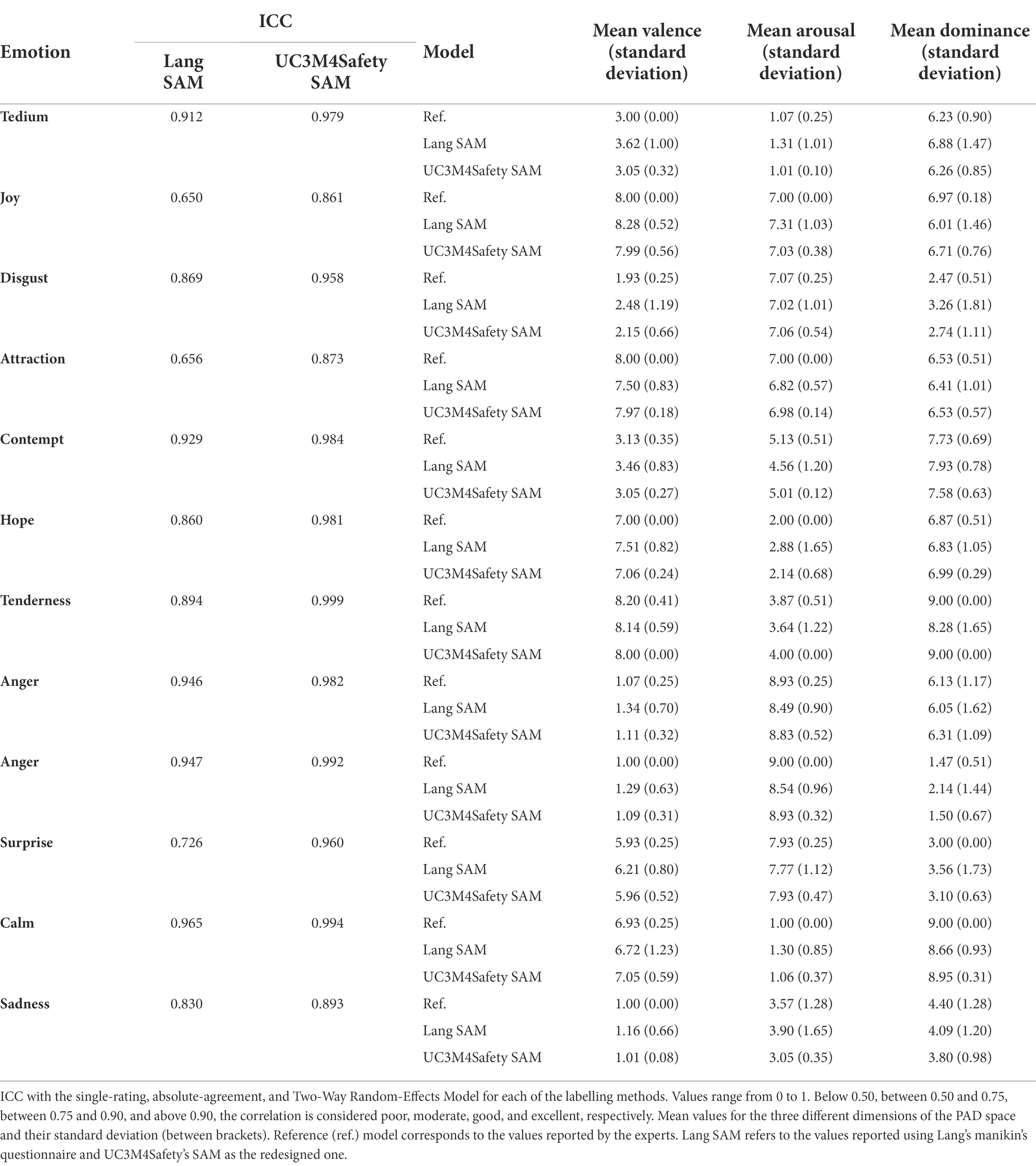
Table 7. Degree of agreement between the continuous labelling comparison of the participants with the gold standard for each of the emotions.
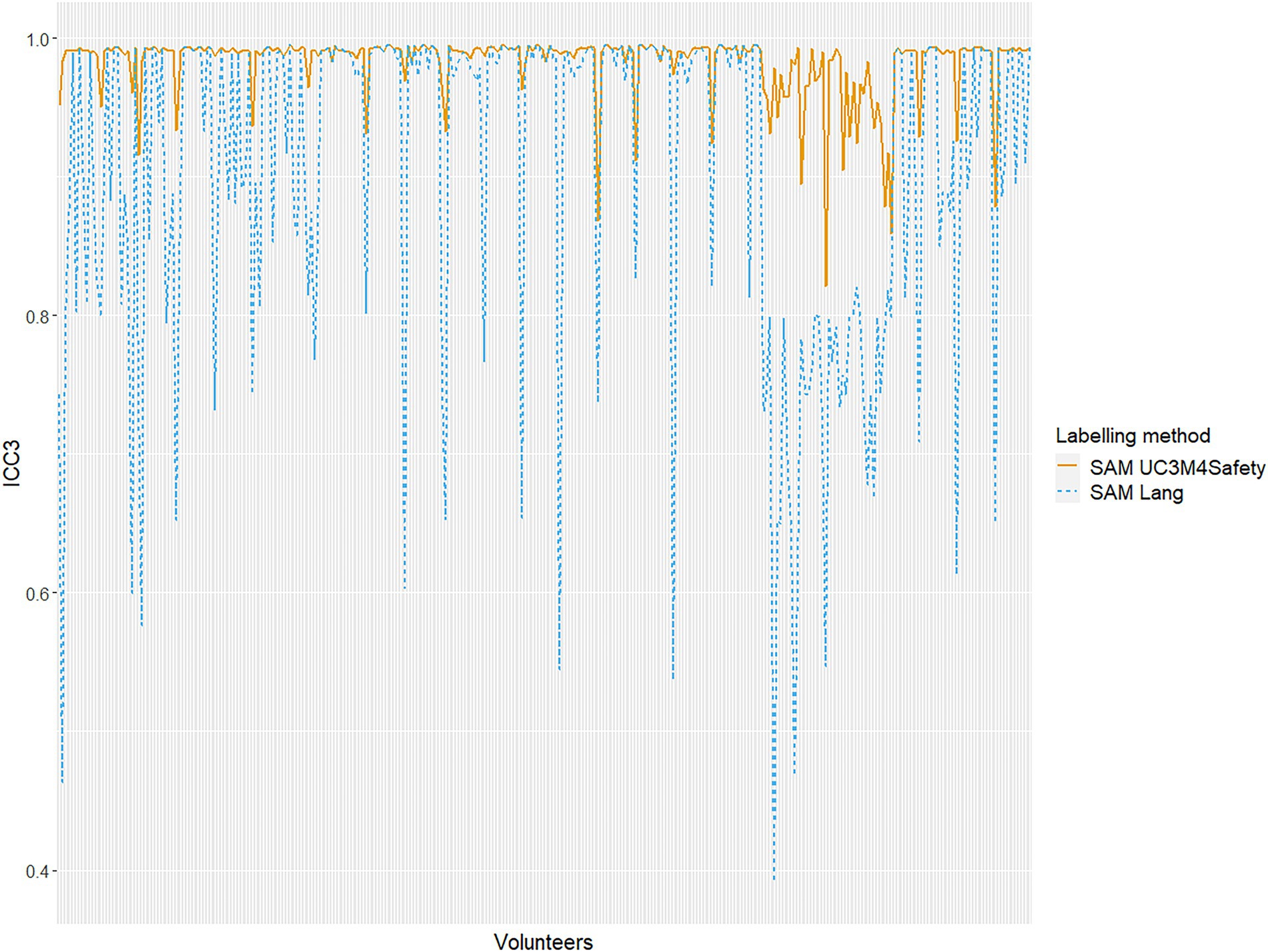
Figure 4. Mean intraclass correlation index of the 12 emotions for each of the participants in relation to the reference test for both models. The y-axis represents the mean intraclass correlation coefficient (ICC) value for the 12 emotions with respect to the gold standard. The x-axis represents each of the volunteers by identifier. The yellow line shows the results corresponding to answers collected using the UC3M4Safety SAM labelling questionnaire. On the other hand, the blue dotted line presents the values obtained by means of the Lang SAM questionnaire.
Finally, the greater agreement found for UC3M4Safety’s SAMs was studied. In order to do this, the data reported with UC3M4Safety’s SAMs and Lang’s SAMs were analysed, comparing them to the golden labels provided by the expert judges in an individual way for every participant.
Women started off with worse data with Lang’s SAMs to obtaining better results than men according to UC3M4Safety’s SAMs. In Figure 4, the mean correlation index of the 12 emotions for each of the participants in relation to the reference test for both models, as can be observed in almost all cases as a dotted yellow line, is above the blue one, meaning the agreement between the gold standard set by the experts and the participants is higher using the new methodology. Moreover, these results show that there was a greater consistency in the data in relation to the reference (golden) test when the UC3M4Safety SAMs were used, especially in the case of women. Out of 57 participants that obtained the same ICC results with both manikins, only six were women.
Discussion
This research started from the hypothesis that the tools traditionally used to measure emotions, and therefore train intelligent systems used in affective computing, were not gender neutral. In particular, whether the SAM instrument as a methodology could be considered a neutral tool was evaluated.
The results have shown that the manikins (SAMs), despite being designed with the objective of being neutral, are not perceived as such by the participants. In particular, the case of the graphic representation of dominance is paradigmatic since what is understood as neutral is perceived as a masculine trait. This particular result is not isolated but is part of a mainstream in scientific knowledge and technology that takes the androcentric point of view as neutral (Leavy, 2018). As Haslanger (2000) points out, in science and innovation, men are the norm and women are deviations from it.
The United Nations Organisations (ONU Mujeres, 2021, para. 3) define gender perspective as ‘the assessment process of the consequences for women and men of any planned activity, including laws, policies or programs, in all sectors and at all levels’. The European Commission—the Directorate-General for Research and Innovation—and currently the State Research Agency (Agencia Estatal de Investigación) in Spain argue that engaging the gender research dimension ‘implies that gender is considered a key analytical and explanatory variable in research’ (Dirección General de Investigación e Innovación, 2011, p. 10). This study corroborates the importance of applying the gender perspective so that results are not partial and constitute quality, egalitarian research.
Technology development is increasingly influencing the behaviour of people in everyday life. However, according to Leavy (2018) and Wajcman (2006), the over-representation of men in the design of these technologies could perpetuate gender inequality. Different researchers have demonstrated that AI algorithms are not neutral and contribute to reproducing existing biases in today’s society, the most evident being those of gender and race (O’Neil, 2016; Buolamwini and Gebru, 2018; Noble, 2018; Cirillo et al., 2020). The main types of biases in AI include gender, ethnicity, and age, and these can increase social inequalities or discrimination. Furthermore, these biases affect all sectors in which AI intervenes—from resource allocation in healthcare, justice, education, or employment—and concern both sectors that may look anecdotal—and are not in any way—and relational machines (especially with personal assistants) or vehicles with integrated voice recognition systems (Nurock, 2020).
A clear example is the controversial area of the application of AI in facial recognition software used by law enforcement agencies (Domingo, 2021). Buolamwini and Gebru (2018) proved that the software utilised by the police in the United States had an error rate regarding gender, ethnicity, and age. This error rate clearly favoured young, white men, while negatively affecting black, elderly women.
The newest line in the measurement of emotions for the prediction of scenarios and human behaviour allows interdisciplinary work between disciplines, such as social sciences and engineering, with the aim of making new technologies increasingly “more human.” The applicability of this interdisciplinary synergy that is being applied intends to improve scientific knowledge by introducing the gender perspective into the design of technologies and into the selection of data to train algorithms (Sainz-de-Baranda et al., 2021a, 2022).
The incorporation of areas such as communication with gender perspective in the processes of research of technology and AI allows the advancement of technological development towards solutions that really improve people’s lives (Rituerto-González et al., 2019, 2020; Sainz-de-Baranda et al., 2021a, 2022; Miranda et al., 2022).
Audiovisual communication is greatly contributing to the emerging research field of affective computing. Within immersive virtual reality environments, the elicitation of emotions via audiovisual stimuli is showing very intense emotional reactions that can be assimilated into real ones in terms of physical and physiological bio-signals (Blanco-Ruiz et al., 2020; Miranda et al., 2021). However, in order to guarantee a high-quality emotional recognition, the AI system must be trained with adequate data sets, including not only those collected by smart sensors but also the tags related to the elicited emotion. Currently, there are very few techniques available to label emotions. Among them, the SAM, which was created by Lang (1980) and Hodes et al. (1985), is one of the most popular.
The results of this study show that the fact that gender socialisation grants differentiating roles to men and women is not considered. These roles start in childhood, from their initiation in social and cultural life, and are reinforced by the influence of socialising agents. Certain cognitive, attitudinal, and behavioural styles are adopted as well as axiological codes and stereotypical morals and rules that follow the social conduct assigned to each gender (Bosch and Ferrer-Pérez, 2002). The trend of identifying people with their peers—or those just like them—(Igartua and Muñiz, 2008; Soto-Sanfiel et al., 2010) has added to the learning of emotions according to individual experiences, which can serve as an explanation for the existing discrepancy in the discrete labelling between men and women. Men have obtained more favourable results, with a high level of agreement, while women have greater variability. Even though discrete tags are not variable and generally have a high level of agreement with previously reported ones, a raise in the level of agreement when questionnaires containing UC3M4Safety’s SAMs are used has been observed, thus clarifying the new design of manikins when participants experience an emotion during the watching/visualisation of a video after assessing the rest of the PAD characteristics of emotion – especially for women.
In the case of the analysis of emotions reported in a numerical way by the participants and which were represented in a tridimensional fashion in the PAD affective space (valence, activation, and dominance), the differences between the tagged emotion a priori and those reported by gender were bigger if both SAM models were applied.
The labelling process of each emotion in the PAD space using the UC3M4Safety SAMs had a higher degree of coincidence with the reference test (gold standard) than that of Lang’s SAMs, both in men and women. These results prove the UC3M4Safety SAM as a reliable and useful tool for the assessment of emotions.
An intersectional feminist approach to new technologies exposes the discriminatory biases of gender, race, and class in the generation and usage of data through information communication technologies (D’Ignazio and Klein, 2020; Blanco-Ruiz, 2022). These results make the inclusion of the gender perspective an imperative in the design of technology and in the generation of databases that are used to train AI systems that coincide with the proposal made by Revi Sterling (2013), who criticises the fact that women, as potential beneficiaries of those technologies, continue to be excluded in design processes.
As pointed out by Schiebinger (2021), identifying gender bias and understanding how it operates is crucially important, “but analysis cannot stop there” (p.3). Future technological developments should be influenced by an intersectional feminist approach (Crenshaw, 1991) in order to avoid reproducing discriminatory gender, race, and class biases, not only in design but also in use (D’Ignazio and Klein, 2020; Blanco-Ruiz, 2022). Incorporating sex, gender, and intersectionality analysis in research is a crucial component that contributes to science and technology (Tannenbaum et al., 2019). Companies such as Google, Amazon, and Facebook are beginning to be aware of the benefits of these inclusive policies. Still, the change must go further; it must permeate the three domains of scientific infrastructure: funding agencies, peer-reviewed journals, and universities (Schiebinger, 2021).
This study is also limited by its own cultural context; it should be tested in other countries to see if the gendered re-reading of the SAM that has been carried out in this study also works in other cultural contexts.
Conclusion
The new version of UC3M4Safety’s SAMs considers gender perspective in its design and its contribution to the communication field, which allows for the generation of databases that enable better creation of AI systems (affective computing) in order to improve quality of life and avoiding gender biases for both women and men.
The need to revise the procedures used for decades in science—and more concretely, in AI—in order to avoid biases of any kind due to age, ethnicity, gender, or others is left on record.
It has been confirmed that Lang’s SAMs contain gender biases and, consequently, the data resulting from the labelling of emotional reactions that former studies used based on audiovisual databases may be biased, and the generated AI systems could be identifying emotions incorrectly from the analysis of these bio-signals.
This type of research could serve as an inspiration to increase the interest of young people, especially women, in Science, Technology, Engineering, and Mathematics (STEM) fields, as it shows how a small change in the representation of a measuring instrument, such as the SAM, could mean that the perception of half of the population is not considered. Audiovisual and emotions are very attractive areas for young people and can serve as magnets to attract their attention to other possibilities of transferring knowledge to society through the STEM disciplines and their cooperation with other areas of knowledge. The national and international equality policies that foster inclusion of the gender dimension in research and that propel interdisciplinary work—which in our case is that of communication, gender studies, and engineering—produce breakthroughs to develop a more egalitarian scientific knowledge.
Data availability statement
The datasets presented in this study can be found in online repositories. The names of the repository/repositories and accession number(s) can be found at: https://edatos.consorciomadrono.es/dataverse/empatia.
Ethics statement
The studies involving human participants were reviewed and approved by Universidad Carlos III de Madrid. The patients/participants provided their written informed consent to participate in this study.
Author contributions
CS contributed to the study conception and design, did the experiment, performed the material preparation and data analysis, wrote the first draft of the manuscript and commented on previous versions of the manuscript, and read and approved the final manuscript. LG-M performed the material preparation and data analysis, wrote the first draft of the manuscript and commented on previous versions of the manuscript, and read and approved the final manuscript. JM-C and MB-R contributed to the study design, did the experiment, and commented on previous versions of the manuscript. CL-O contributed to the study conception and design, wrote the first draft of the manuscript and commented on previous versions of the manuscript, and read and approved the final manuscript. All authors contributed to the article and approved the submitted version.
Funding
This work was supported by the Department of Research and Innovation of Madrid Regional Authority under Grant EMPATÍA-CM:Y2018/TCS-5046; and State Research Agency (Spain) under grant PID2019-106695RB-I00/AI-GENBIAS/10.13039/501100011033.
Conflict of interest
The authors declare that the research was conducted in the absence of any commercial or financial relationships that could be construed as a potential conflict of interest.
Publisher’s Note
All claims expressed in this article are solely those of the authors and do not necessarily represent those of their affiliated organizations, or those of the publisher, the editors and the reviewers. Any product that may be evaluated in this article, or claim that may be made by its manufacturer, is not guaranteed or endorsed by the publisher.
Supplementary material
The Supplementary material for this article can be found online at: https://www.frontiersin.org/articles/10.3389/fpsyg.2022.955530/full#supplementary-material
Footnotes
1. ^Gender refers to the socially defined roles, characteristics and opportunities that are considered appropriate for men, women, boys, girls and people with non-binary identities. Gender is also a product of the relationships between people and can reflect the distribution of power between them (ONU Mujeres, 2021). According to Díaz Martínez, 2016, gender perspective implies that sex and gender are reflected in research content. Gender as a perspective can manifest itself in research questions, theories, approaches, methods and dissemination. This means that sex, gender and their interaction must be adequately represented and addressed in the groups under study, and should be kept in mind if the impact of the research and the results are different. In relation to this, it is interesting to note the work developed by Anne Fausto-Sterling and Londa Schiebinger.
2. ^You can access the video clips here: https://edatos.consorciomadrono.es/dataset.xhtml?persistentId=doi:10.21950/LUO1IZ
References
Bakker, I., van der Voordt, T., Vink, P., and de Boon, J. (2014). Pleasure, arousal, dominance: Mehrabian and Russell revisited. Curr. Psychol. 33, 405–421. doi: 10.1007/s12144-014-9219-4
Baumgartner, T. A. (2000). Estimating the stability reliability of a store. Meas. Phys. Educ. Exerc. Sci. 4, 175–178. doi: 10.1207/S15327841Mpee0403_3
Bilsky, S. A., Feldner, M. T., Bynion, T., Rojas, S. M., and Leen-Feldner, E. W. (2018). Child anxiety and parental anxiety sensitivity are related to parent sick role reinforcement. Parenting 18, 110–125. doi: 10.1080/15295192.2018.1444132
Blanco-Ruiz, M. (2022). “Perspectiva de género en el entorno digital [Gender perspective in the digital environment],” in Curación Digital and Género en la Ciencia de la Información: Acceso and Preservación. eds. M. J. Vicentini, R. San-Segundo, J. A. F. Montoya, D. Martínez-Ávila, and L. A. Landim (Salamanca (Spain): Ediciones Universidad de Salamanca), 45–59.
Blanco-Ruiz, M., Gutiérrez, L., Miranda, J. A., Canabal, M. F., Romero, E., Sainz-de-Baranda, C., et al. (2021a). UC3M4Safety database—list of audiovisual stimuli.
Blanco-Ruiz, M., Gutiérrez, L., Miranda, J. A., Canabal, M. F., Romero, E., Sainz-de-Baranda, A.C., et al. (2021b). UC3M4Safety database—list of audiovisual stimuli [video]
Blanco-Ruiz, M., Sainz-de-Baranda, A. C., Gutiérrez-Martín, L., Romero-Perales, E., and López-Ongil, C. (2020). Emotion elicitation under audiovisual stimuli reception: should artificial intelligence consider the gender perspective? Int. J. Environ. Res. Public Health 17:8534. doi: 10.3390/ijerph17228534
Bosch, F. E., and Ferrer-Pérez, V. A. (2002). La voz de las Invisibles: Las Víctimas de un mal Amor Que Mata [The Voice of the Invisible: Victims of a Bad Love That Kills]. Valencia (Spain): Cátedra.
Bradley, M. M., and Lang, P. J. (1994). Measuring emotion: the self-assessment manikin and the semantic differential. J. Behav. Ther. Exp. Psychiatry 25, 49–59. doi: 10.1016/0005-7916(94)90063-9
Buolamwini, J., and Gebru, T. (2018). Gender shades: intersectional accuracy disparities in commercial gender classification. Proc. Machine Learn. Res. 81, 1–15.
Cirillo, D., Catuara-Solarz, S., Morey, C., Guney, E., Subirats, L., Mellino, S., et al. (2020). Sex and gender differences and biases in artificial intelligence for biomedicine and healthcare. NPJ Digit. Med. 3:81. doi: 10.1038/s41746-020-0288-5
Cohen, J. C. (2001). Defining identification: A theoretical look at the identification of audiences with media characters. Mass Commun. Soc. 4, 245–264. doi: 10.1207/S15327825MCS0403_01
Conroy, D. E., and Metzler, J. (2003). Temporal stability of performance failure appraisal inventory items. Meas. Phys. Educ. Exerc. Sci. 7, 243–261. doi: 10.1207/S15327841MPEE0704_3
Correa-Rojas, J. (2021). Coeficiente de correlación intraclase: Aplicaciones Para estimar la estabilidad temporal de un instrumento de medida [Intraclass correlation coefficient: applications for estimating the temporal stability of a measuring instrument]. Ciencias Psicol. 15, 1–16. doi: 10.22235/cp.v15i2.2318
Crenshaw, K. (1991). Mapping the margins: Intersectionality, identity politics, and violence against women of color. Stanford Law Rev. 43, 1241–1299. doi: 10.2307/1229039
Deng, Y., Yang, M., and Zhou, R. (2017). A new standardized emotional film database for Asian culture. Front. Psychol. 8, 56–66. doi: 10.3389/fpsyg.2017.01941
Di Girolamo, M., Giromini, L., Winters, C. L., Serie, C. M. B., and de Ruiter, C. (2019). The questionnaire of cognitive and affective empathy: a comparison between paper-and-pencil versus online formats in Italian samples. J. Pers. Assess. 101, 159–170. doi: 10.1080/00223891.2017.1389745
Díaz Martínez, C. (2016). “La perspectiva de género en investigación social [Gender perspective in social research],” in El análisis de la realidad social: métodos y técnicas de investigación. ed. Alianza (Madrid (Spain)), 176–201.
Dirección General de Investigación e Innovación (2011). Toolkit Gender in EU-Funded Research. Brussels (Belgium): Publications Office of the European Union.
Domingo, J. C. (2021). Utilización del sistema de reconocimiento facial Para preservar la seguridad ciudadana [Use of the facial recognition system to preserve public safety]. El Criminal. Digit. 9, 20–37.
Dunbar-Hester, C. (2019). Hacking Diversity: The Politics of Inclusion in Open Technology Cultures. Princeton, New Jersey (United States): Princeton University Press.
Ekman, P. (1992). Are there basic emotions? Psychol. Rev. 99, 550–553. doi: 10.1037/0033-295X.99.3.550
Ekman, P. (1999). “Basic emotions,” in Handbook of Cognition and Emotion. eds. T. Dalgleish and M. J. Power (Hoboken, New Jersey (United States): John Wiley and Sons Ltd.), 45–60.
Ekman, P. (2016). What scientists who study emotion agree about. Perspect. Psychol. Sci. 11, 31–34. doi: 10.1177/1745691615596992
Ekman, P., and Cordaro, D. (2011). What is meant by calling emotions basic? Emot. Rev. 3, 364–370. doi: 10.1177/1754073911410740
European Commission (2020). Gendered innovations 2: how inclusive analysis contributes to research and innovation: policy review. Publications Office of the European Union. Available at: https://data.europa.eu/doi/10.2777/316197
Fleiss, J. L. (1971). Measuring nominal scale agreement among many raters. Psychol. Bull. 76, 378–382. doi: 10.1037/h0031619
Fontaine, J. R., Klaus, R. S., Etienne, B. R., and Phoebe, C. E. (2016). The world of emotions is not two-dimensional. Psychol. Sci. 18, 1050–1057. doi: 10.1111/j.1467-9280.2007.02024.x
Gantiva, C., Guerra, P., and Vila, J. (2011). Validación colombiana del sistema internacional de imágenes afectivas: Evidencias del origen transcultural de la emoción [Colombian validation of the international affective imagery system: evidence of the cross-cultural origin of emotion]. Acta Colombiana de Psicología 14, 103–111.
Ge, Y., Zhao, G., Zhang, Y., Houston, R. J., and Song, J. (2019). A standardised database of Chinese emotional film clips. Cognit. Emot. 33, 976–990. doi: 10.1080/02699931.2018.1530197
Grégoire, L., and Greening, S. G. (2020). Fear of the known: semantic generalisation of fear conditioning across languages in bilinguals. Cognit. Emot. 34, 352–358. doi: 10.1080/02699931.2019.1604319
Hagemann, D., Naumann, E., Maier, S., Becker, G., Lürken, A., and Bartussek, D. (1999). The assessment of affective reactivity using films: validity, reliability and sex differences. Personal. Individ. Differ. 26, 627–639. doi: 10.1016/S0191-8869(98)00159-7
Haraway, D. (1988). Situated knowledges: the science question in feminism and the privilege of partial perspectives. Fem. Stud. 14, 575–599. doi: 10.2307/3178066
Harding, S. (1991). Whose Science? Whose Knowledge? Thinking From Women’s Lives. Ithaca, New York (United States): Cornell University Press.
Haslanger, S. (2000). “Feminism in metaphysics: managing the natural” in The Cambridge Companion to Feminism in Philosophy. eds. M. Fricker and J. Hornsby (Cambridge (UK): Cambridge University Press), 107–126.
Hicks, M. (2017). Programmed Inequality: How Britain Discarded Women Technologists and Lost Its Edge in Computing. Cambridge, MA (USA): MIT Press.
Hodes, R. L., Cook, E. W., and Lang, P. J. (1985). Individual differences in autonomic response: conditioned association or conditioned fear? Psychophysiology 22, 545–560. doi: 10.1111/j.1469-8986.1985.tb01649.x
Igartua, J. J., and Muñiz, C. (2008). Identificación con los personajes and disfrute ante largometrajes de ficción. Una investigación empírica [Character identification and enjoyment of fiction feature films. An empirical investigation]. Comunicacion Soc. 21, 25–51.
Israelashvili, J., Oosterwijk, S., Sauter, D., and Fischer, A. (2019). Knowing me, knowing you: emotion differentiation in oneself is associated with recognition of others’ emotions. Cognit. Emot. 33, 1461–1471. doi: 10.1080/02699931.2019.1577221
Izard, C. E. (2016). Basic emotions, natural kinds, emotion schemas, and a new paradigm. Perspect. Psychol. Sci. 2, 260–280. doi: 10.1111/j.1745-6916.2007.00044.x
Kentner, A. C., and Grace, S. L. (2017). Between mind and heart: sex-based cognitive bias in cardiovascular disease treatment. Front. Neuroendocrinol. 45, 18–24. doi: 10.1016/j.yfrne.2017.02.002
Koelstra, S., Mühl, C., Soleymani, M., Jong-Seok, L., Yazdani, A., Ebrahimi, T., et al. (2012). DEAP: a database for emotion analysis: using physiological signals. IEEE Trans. Affect. Comput. 3, 18–31. doi: 10.1109/T-AFFC.2011.15
Lang, P. J. (1980). “Behavioral treatment and bio-behavioral assessment: computer applications” in Technology in Mental Health Care Delivery Systems. eds. J. B. Sidowski, J. H. Johnson, and T. A. Williams (New York City, New York (USA): Ablex Publishing Corporation), 119–137.
Lang, P. J. (1985). “The cognitive psychophysiology of emotion: Fear and anxiety,” in Anxiety and the Anxiety Disorders. eds. A. H. Tuma and J. D. Maser (Lawrence Erlbaum Associates, Inc.), 131–170.
Lang, P. J., Bradley, M., and Cuthbert, B. N. (2008). International affective picture system (IAPS): instruction manual and affective ratings. (Technical Report A-8). The Center for Research in Psychophysiology, University of Florida. Available at: https://csea.phhp.ufl.edu/Media.html
Leavy, S. (2018). Gender bias in artificial intelligence: the need for diversity and gender theory in machine learning in “2018 IEEE/ACM 1st International Workshop on Gender Equality in Software Engineering (GE), 2018,” 14–16
Leen-Feldner, E. W., Blumenthal, H., Babson, K., Bunaciu, L., and Feldner, M. T. (2008). Parenting-related childhood learning history and panic vulnerability: a test using a laboratory-based biological challenge procedure. Behav. Res. Ther. 46, 1009–1016. doi: 10.1016/j.brat.2008.06.002
Lockwood, P. L., Ang, Y.-S., Husain, M., and Crockett, M. J. (2017). Individual differences in empathy are associated with apathy-motivation. Sci. Rep. 7, 17293–17210. doi: 10.1038/s41598-017-17415-w
Mauss, I. B., and Robinson, M. D. (2009). Measures of emotion: a review. Cognit. Emot. 23, 209–237. doi: 10.1080/02699930802204677
Miranda, J. A., Canabal, M. F., Gutiérrez-Martín, L., Lanza-Gutiérrez, J. M., Portela-García, M., and López-Ongil, C. (2021). Fear recognition for women using a reduced set of physiological signals. Sensors 21, 1587. doi: 10.3390/s21051587
Miranda, J. A., Rituerto-González, E., Luis-MinguezaCanabal, C., Canabal, M. F., Ramírez Bárcenas, A., Lanza-Gutiérrez, J. M., et al. (2022). Bindi: affective internet of things to combat gender-based violence. IEEE Internet Things J. doi: 10.1109/JIOT.2022.3177256
Miranda-Correa, J. A., Abadi, M. K., Sebe, N., and Patras, I. (2018). AMIGOS: A dataset for affect, personality and mood research on individuals and groups. IEEE Trans. Affect. Comput. 12, 479–493. doi: 10.1109/TAFFC.2018.2884461
Moltó, J., Segarra, P., López, R., Esteller, À., Fonfría, A., Pastor, M., et al. (2013). Adaptación española del “international affective picture system” (IAPS): Tercera parte [Spanish adaptation of the “international affective picture system” (IAPS): part 3]. Anal. Psicol. 29, 965–984. doi: 10.6018/analesps.29.3.153591
Moss-Racussin, C. A., Dovidio, J. F., Brescoll, V. L., Graham, M. J., and Handelsman, J. (2012). Science faculty’s subtle gender biases favor male students. Proc. Nat. Acad. Sci. U.S.A. 109, 16474–16479. doi: 10.1073/pnas.1211286109
Noble, S. (2018). Algorithms of Oppression: How Search Engines. New York City, New York (USA): NYU Press.
Nurock, V. (2020). ¿Puede prestar cuidados la Inteligencia Artificial? [Can artificial intelligence provide care?]. Cuadernos Relac. Lab. 38, 217–229. doi: 10.5209/crla.70880
O’Neil, C. (2016). Weapons of Math Destruction: How big data Increases Inequality and Threatens Democracy. New York City, New York (USA): Crown Random House.
Olatunji, B. O., Wolitzky-Taylor, K. B., Babson, K. A., and Feldner, M. T. (2009). Anxiety sensitivity and CO₂ challenge anxiety during recovery: differential correspondence of arousal and perceived control. J. Anxiety Disord. 23, 420–428. doi: 10.1016/j.janxdis.2008.08.006
ONU Mujeres (2021). Incorporación de la perspectiva de género. Available at: https://www.unwomen.org/es/how-we-work/un-system-coordination/gender-mainstreaming#:~:text=La%20Cuarta%20Conferencia%20Mundial%20sobre,compromisos%20en%20igualdad%20de%20g%C3%A9nero
Ortega-Toro, E., Jiménez-Egido, J. M., Palao-Andrés, J. M., and Sainz-de-Baranda, A. P. (2008). Diseño and validación de un cuestionario para valorar las preferencias and satisfacciones en jóvenes jugadores de baloncesto [Design and validation of a questionnaire to assess preferences and satisfaction in young basketball players]. Cuadern. Psicol. Deporte 8, 39–58.
Pedrosa, I., Suárez-Álvarez, J., and García-Cueto, E. (2013). Evidencias sobre la validez de contenido: avances teóricos and métodos para su estimación [Evidence on content validity: theoretical advances and methods for its estimation]. Acción Psicol. 10, 3–18. doi: 10.5944/ap.10.2.11820
Penfield, R. D., and Giacobbi, P. R. (2004). Applying a score confidence interval to Aiken’s item content-relevance index. Meas. Phys. Educ. Exerc. Sci. 8, 213–225. doi: 10.1207/s15327841mpee0804_3
Picard, R. W. (1995). Affective computing. M.I.T media laboratory perceptual computing section. Tech. Rep. 321, 1–26.
Picard, R. W., Vyzas, E., and Healey, J. (2001). Toward machine emotional intelligence: analysis of affective physiological state, IEEE T. Pattern Analy. Machine Intellig. 23, 1175–1191. doi: 10.1109/34.954607
Plutchik, R. (2001). The nature of emotions: human emotions have deep evolutionary roots, a fact that may explain their complexity and provide tools for clinical practice. Am. Sci. 89, 344–350. doi: 10.1511/2001.4.344
Revi Sterling, S. (2013). “Designing for trauma: the roles of ICTD in combating violence against women (VAW).” in ICTD ‘13: Proceedings of the Sixth International Conference on Information and Communications Technologies and Development. eds. G. Marsden, J. May, M. Chetty, and U. Rivett; 2, 159–162, Association for Computing Machinery.
Rituerto-González, E., Mínguez-Sánchez, A., Gallardo-Antolín, A., and Peláez-Moreno, C. (2019). Data augmentation for speaker identification under stress conditions to combat gender-based violence. Appl. Sci. 9, 2298. doi: 10.3390/app9112298
Rituerto-González, E., Miranda, J. A., Canabal, M. F., Lanza-Gutiérrez, J. M., Peláez-Moreno, C., and López-Ongil, C. (2020). “A hybrid data fusion architecture for BINDI: A wearable solution to combat gender-based violence” in Multimedia Communications, Services and Security. eds. A. Dziech, W. Mees, and A. Czyżewski (Cham: Springer), 223–237.
Robinson, D. L. (2008). Brain function, emotional experience and personality. Neth. J. Psychol. 64, 152–168. doi: 10.1007/BF03076418
Sainz-de-Baranda, A. C., Blanco-Ruiz, M., Miranda, J. A., Gutiérrez-Martín, L., Canabal, M. F., San-Segundo, R., et al. (2021a). Perspectiva de género and social en las STEM: La construcción de sistemas inteligentes para detección de emociones [Gender and social perspective in STEM: Building intelligent systems for emotion detection]. Soc. Technosci. 11, 83–115. doi: 10.24197/st.Extra_1.2021.83-115
Sainz-de-Baranda, A. C., Blanco-Ruiz, M., and San-Segundo, R. (2021b). El rol del audiovisual en la activación de la memoria autobiográfica en víctimas de violencia de género [The role of audiovisuals in the activation of autobiographical memory in victims of gender violence]. Cuestion. Género 16, 810–835. doi: 10.18002/cg.v0i16.6918
Sainz-de-Baranda, A. C., De-Lamo Velado, I., and Nieto Rojas, P. (2022). Use of technological devices and (re)victimization in gender-based crimes in Spain: a qualitative study on professionals’ perceptions. Soc. Technosci. 12, 56–72. doi: 10.24197/st.1.2022.56-72
Saleh, W., Leva, M. C., Ababio-Donkor, A., and Thimnu, A. (2022). “Gender and equality in transport.” in Proceedings of the 2021 Travel Demand Management Symposium.
San-Segundo, M. R., Sainz-de-Baranda, A. C., Blanco-Ruiz, M., Larrabeiti-López, D., Urueña, P. M., Robledo, G. J. C., et al. (2021). Modelo de utilidad N° de solicitud. U202130953. Sistema y método para determinar un estado emocional de un usuario [System and method for determining an emotional state of a user]. Available at: https://bopiweb.com/2021-05-17.779#bopi_3753503
Schaefer, A., Nils, F., Sanchez, X., and Philippot, P. (2010). Assessing the effectiveness of a large database of emotion-eliciting films: a new tool for emotion researchers. Cognit. Emot. 24, 1153–1172. doi: 10.1080/02699930903274322
Schiebinger, L. (2021). Gendered innovations: integrating sex, gender, and intersectional analysis into science, health and medicine, engineering, and environment. Tapuya Latin Am. Sci. Technol. Soc. 4, 1867420. doi: 10.1080/25729861.2020.1867420
Soares, A. P., Pinheiro, A. P., Costa, A., Frade, S., Comesaña, M., and Pureza, R. (2013). Affective auditory stimuli: adaptation of the international affective digitized sounds (IADS-2) for European Portuguese. Behav. Res. Methods 45, 1168–1181. doi: 10.3758/s13428-012-0310-1
Soleymani, M., Pantic, M., and Pun, T. (2012). Multimodal emotion recognition in response to videos. IEEE Trans. Affect. Comput. 3, 211–223. doi: 10.1109/T-AFFC.2011.37
Sonderegger, A., Heyden, K., Chavaillaz, A., and Sauer, J. (2016). “AniSAM and AniAvatar: animated visualizations of affective states.” in The 2016 CHI Conference. eds. J. Kaye, and A. Druin, 4828–4837.
Soto-Sanfiel, M. T., Aymerich-Franch, L., and Ribes-Guàrdia, X. (2010). Impacto de la interactividad en la identificación con los personajes de ficciones. Psicothema 22, 822–827.
Sumartojo, S., Pink, S., Lupton, D., and LaBond, C. H. (2016). The affective intensities of datafied space. Emot. Space Soc. 21, 33–40. doi: 10.1016/j.emospa.2016.10.004
Tannenbaum, C., Ellis, R. P., Eyssel, F., Zou, J., and Schiebinger, L. (2019). Sex and gender analysis improves science and engineering. Nature 575, 137–146. doi: 10.1038/s41586-019-1657-6
Thaler, A. (2022). “Saving lives with gender studies? Putting technofeminism into practice” in Proceedings of the 4th International Conference on Gender Research. (eds.) E. T. Pereira, C. Costa, and Z. Breda.
Thomas, J. R., and Nelson, J. K. (2007). Métodos de investigación en actividad física [Research methods in physical activity]. Barcelona: Paidotribo
Trommsdorff, G., Friedlmeier, W., and Mayer, B. (2016). Sympathy, distress, and prosocial behavior of preschool children in four cultures. Int. J. Behav. Dev. 31, 284–293. doi: 10.1177/0165025407076441
Verma, G. K., and Tiwary, U. S. (2015). Affect representation and recognition in 3D continuous valence–arousal–dominance space. Multimed. Tools Appl. 76, 2159–2183. doi: 10.1007/s11042-015-3119-y
Wiersma, L. D. (2001). Conceptualization and development of the sources of enjoyment in youth sport questionnaire. Meas. Phys. Educ. Exerc. Sci. 5, 153–177. doi: 10.1207/S15327841MPEE0503_3
Zafra, R. (2011). Un cuarto propio conectado. Feminismo and creación desde la esfera público-privada online [A connected room of one’s own. Feminism and creation from the online public-private sphere]. Asparkía Investig. Femin. 22, 115–129.
Keywords: Self-Assessment Manikin, gender, emotion, affective space, pleasure-arousal-dominance, affective computing, artificial intelligence
Citation: Sainz-de-Baranda Andujar C, Gutiérrez-Martín L, Miranda-Calero J&, Blanco-Ruiz M and López-Ongil C (2022) Gender biases in the training methods of affective computing: Redesign and validation of the Self-Assessment Manikin in measuring emotions via audiovisual clips. Front. Psychol. 13:955530. doi: 10.3389/fpsyg.2022.955530
Edited by:
Milagros Sainz, Open University of Catalonia, SpainReviewed by:
Alicia García-Holgado, University of Salamanca, SpainAndrea Vera-Gajardo, Universidad de Valparaíso, Chile
Copyright © 2022 Sainz-de-Baranda Andujar, Gutiérrez-Martín, Miranda-Calero, Blanco-Ruiz and López-Ongil. This is an open-access article distributed under the terms of the Creative Commons Attribution License (CC BY). The use, distribution or reproduction in other forums is permitted, provided the original author(s) and the copyright owner(s) are credited and that the original publication in this journal is cited, in accordance with accepted academic practice. No use, distribution or reproduction is permitted which does not comply with these terms.
*Correspondence: Clara Sainz-de-Baranda Andujar, cbaranda@hum.uc3m.es; Laura Gutiérrez-Martín, lagutier@ing.uc3m.es