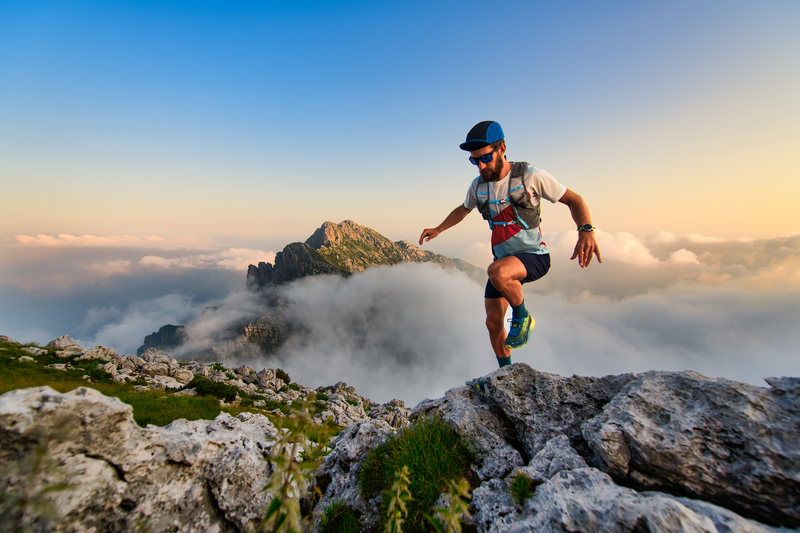
94% of researchers rate our articles as excellent or good
Learn more about the work of our research integrity team to safeguard the quality of each article we publish.
Find out more
ORIGINAL RESEARCH article
Front. Psychol. , 11 August 2022
Sec. Quantitative Psychology and Measurement
Volume 13 - 2022 | https://doi.org/10.3389/fpsyg.2022.944976
Mobile health (mHealth) services have been widely used in medical services and health management through mobile devices and multiple channels, such as smartphones, wearable equipment, healthcare applications (Apps), and medical platforms. However, the number of the users who are currently receiving the mHealth services is small. In China, more than 70% of internet users have never used mHealth services. Such imbalanced situation could be attributed to users’ traditional concept of medical treatment, psychological factors (such as low self-efficacy) and privacy concerns. The purpose of this study is to explore the direct and indirect effects of mHealth users’ self-efficacy and privacy concerns on their intention to adopt mHealth services, providing guidelines for mHealth service providers to enhance users’ intention of adoption. A questionnaire was designed by the research team and 386 valid responses were collected from domestic participants in China. Based on the unified theory of acceptance and use of technology (UTAUT) model, a research model integrated self-efficacy and privacy concerns was constructed to investigate their effects on users’ intention to adopt mobile mHealth services. The results show that self-efficacy could facilitate users’ intention to adopt mHealth services, and had a significantly positive effect on perceived ubiquity, effort expectancy, performance expectancy and subjective norm. This study verifies the direct and indirect effects of self-efficacy and privacy concerns on users’ intention to adopt mHealth services, providing a different perspective for studying mHealth adoption behavior. The findings could provide guidelines for mHealth service providers to improve their service quality and enhance users’ intention of adoption.
Mobile health (mHealth) services refer to the provision of medical services to users through mobile devices, such as smartphones, tablet computers, and satellite communications (Jovanov and Zhang, 2004; Bai et al., 2020; Bally and Cesuroglu, 2020; Lee, 2021; Sujarwoto et al., 2022). mHealth services have changed the traditional healthcare and played an increasing important role in the medical service delivery through their unique features, such as ease of use, usefulness and convenience (Liu et al., 2019; Crowell et al., 2022; Zhao et al., 2022). More and more patients are obtaining medical information and services through mobile devices, including making appointment, seeking treatment, viewing electronic test reports and consulting the doctors (Lee and Han, 2015; Wang and Qi, 2021; Balica, 2022; Jenkins, 2022), which effectively alleviates the problem of the “queuing for 3 h but seeing the doctor for 3 m” in China. mHealth services have become a new approach for people’s health management (Park, 2016).
With the integration and development of “Internet + medical healthcare,” mHealth service users refer to people who obtain mHealth services through channels such as hospital’s official website, Weibo (a platform for information sharing, dissemination, commenting and acquisition based on user relationship; Zhang and Pentina, 2012), WeChat public account (a platform for interaction and communication between the host and subscribers; Geng, 2016), WeChat mini program (a fast App with click-to-use function and free download and installation; Cheng et al., 2019), mHealth service App, and provincial and municipal medical platforms. By December 2020, there were 989 million netizens in China, but only 215 million mHealth service users. That means more than 70% of netizens have never used mHealth services. In addition, the users’ intention of continuous use of mHealth services is not high (Serlachius et al., 2019) due to the influence of traditional concept of medical treatment, users’ psychological factors and privacy concerns considering that mHealth is an innovative App of information technology in the medical field (Hsiao and Tang, 2015; Zhou et al., 2019). Therefore, how to motivate and guide users to use mHealth services and meet their medical needs more effectively has become an urgent issue for mHealth providers.
Existing studies have explored the influential factors and mechanisms of users’ adoption behavior of mHealth services based on different theoretical models. Many scholars have mainly focused on technical features of mHealth services (AlBar and Hoque, 2019; Nadal et al., 2020; Nezamdoust et al., 2022), App platform design (Miao et al., 2018; Wu et al., 2020) or factors related to the external environment which influence users’ adoption and continuous use of mHealth services (Li et al., 2016; Chen et al., 2018). Less attention has been paid to the individual cognitive factors that affect users’ adoption of mHealth services.
According to previous studies, in the context of mobile healthcare, the individual cognitive factors that affect users’ adoption behavior mainly include attitude, trust, intrinsic motivation, self-efficacy, privacy concerns, IT personal innovativeness, mobile technology identity, technology anxiety, and electronic health knowledge (Andronie et al., 2021b, pp. 863–888). These individual cognitive factors have been used in numerous studies to explain the decision process of mHealth adoption behavior (Andronie et al., 2021a, pp. 2497; Lãzãroiu et al., 2022). For example, Deng et al. (2018) identified trust and privacy risk as the most dominant individual cognitive factors and critical factors explaining Chinese patients’ behavioral intention on mHealth. Rajak and Shaw (2021) showed that technology anxiety negatively affected perceived usefulness and perceived ease of use, while data privacy might be a cause of technology anxiety. In addition, self-efficacy was acknowledged to play a crucial role in influencing users’ adoption behavior towards mHealth (Zhang et al., 2017). Alam et al. (2020) found that self-efficacy was positively associated with mHealth adoption intentions.
In users’ acceptance and use of mHealth services, self-efficacy and privacy concerns are critical to adoption behaviors because users may refuse to accept or use mHealth services when users believe that they are incapable to access medical services through mobile devices, or fear that personal privacy information will be leaked without authorization. In this study, self-efficacy refers to the user’s self-perception about whether they will be capable of using mHealth services, while privacy concerns refer to the level of the users’ anxiety about how the mHealth App collect, utilize and protect their personal information. Balapour et al. (2019) conducted an innovative study on patients’ self-monitoring health using mHealth Apps, confirming that self-efficacy promoted patients’ healthy behaviors and the use of mHealth Apps. Li (2020) studied the adoption and use of mHealth services by Chinese users, pointing out that privacy concerns were an important factor hindering users from adopting mHealth services. However, the limitations of these studies are that they did not consider the influence of self-efficacy and privacy concerns, the two typical individual cognitive factors, on users’ adoption behavior of mHealth services at the same time.
mHealth is an emerging form of healthcare with mobile technology in the medical field. Users are the main body of the adoption of mHealth services. However, existing studies did not pay enough attention to the psychological factors of individuals, ignoring the influence of users’ self-efficacy and privacy concerns on their intentions to adopt mHealth services. To better understand and predict the adoption behavior of mHealth users, based on the unified theory of acceptance and use of technology (UTAUT) model, this study introduces two individual cognition factors, self-efficacy and privacy concerns into the model and investigates their effects on users’ intention to adopt mHealth services. Therefore, this paper aims to answer the following research questions:
(1) How do mHealth users’ intention of adoption arise? What are the individual cognitive factors that influence users’ intention to adopt?
(2) What is the role of users’ self-efficacy, privacy concerns, and UTAUT-related variables in the decision process of mHealth users’ intention to adopt?
Compared with existing studies, this study focuses on user’s individual cognition and uses UTAUT theory to analyze the influence of self-efficacy and privacy concerns on the intention to adopt mHealth. By adding two representative individual cognitive factors (self-efficacy and privacy concerns) into the UTAUT model, we can predict and explain users’ mHealth adoption behavior more comprehensively and provide insights for mHealth adoption behavior research. Meanwhile, exploring the four perceptual factors of UTAUT as mediating variables helps better the interpret UTAUT in mHealth and also provides new ideas to extend the App of the UTAUT model in mHealth services. Finally, the findings of this paper help mHealth service providers understand the effect of individual cognitive factors on users’ intention to adopt, and provide theoretical basis and pragmatic suggestions for improving their healthcare services and enhancing users’ intention to adopt.
The remaining chapters of this study are organized as follows: section “Literature review and hypotheses development” provides the theoretical background. Section “Research methodology” presents the hypotheses. Section “Results” describes the research design, and section “Discussion” discusses the results. Section “Conclusion” is the conclusion of the study.
Existing literature related to mobile health mainly focuses on the definition of mobile healthcare, service types, stakeholders, and user adoption behaviors. Istepanian et al. (2007) first proposed the concept of “mobile healthcare,” and defined it as a medical system that used mobile communication and network equipment to provide health services. Motamarri et al. (2014) classified the mHealth services into medical information service, diagnosis service, disease monitoring service, health data monitoring service and telemedical service. Meanwhile, Dehzad et al. (2014) categorized mHealth stakeholders as policy makers, users, service providers and other stakeholders. In addition, regarding the adoption behavior of mHelath users, existing studies have explored the influential factors of users’ adoption behavior based on different theoretical models, as shown in Table 1. Garavand et al. (2019) studied the influencing factors of medical students’ adoption of mHealth services based on the UTAUT model, and pointed out that performance expectancy and social influence were not significantly related to adoption behavior. Deng et al. (2018) considered the influence of trust and perceived risk on users’ intention to adopt based on the technology acceptance model (TAM), and the results showed that trust, perceived usefulness and perceived ease of use had a significant positive impact on intention of adoption.
In addition, many studies have confirmed that some main individual cognition factors, such as self-efficacy, privacy concerns, perceived risk, and technology anxiety, have a direct or indirect impact on the adoption behavior of mHealth users. Pan et al. (2019) conducted a study on the adoption intentions of clinicians and non-clinicians from the perspective of technology transfer, and confirmed that subjective norm had a positive effect on clinicians’ behavioral intention, while perceived risk had a negative effect only on non-clinicians’ attitude. Based on protection motivation theory (PMT), Guo et al. (2015) explored the influence of threat appraisal and coping appraisal on the adoption intention of mHealth users. The results showed that users’ threat appraisal and coping appraisal negatively influenced the intention of adoption through attitude. At the same time, privacy concerns are regarded as one of the risks for users to adopt mHealth services. Guo et al. (2016), based on the privacy personalization paradox, pointed out that privacy concerns had a significant negative impact on users’ intention to adopt mHealth services. It is worth noting that some studies segment users according to different service types, which often leads to inconsistent conclusions. For example, Meng et al. (2020), explored the negative impact of elderly users’ characteristics (health anxiety and technology anxiety) on intention of continuance of use based on trust theory. Lim et al. (2011) studied Singaporean women’s acceptance of using mobile phones to seek health information and found that technology anxiety had no significant effect on female users’ intention to use. In addition, Deng (2013) pointed out that the perceived ease of use had no significant effect on the behavioral attitude of elderly users, while the empirical results of Hsiao and Chen (2015) showed that the perceived ease of use had significant effects.
Mobile health is an innovative App of mobile technology in healthcare, and the study of mobile health users’ intention to adopt is a part of the study of information technology adoption behavior. The TAM is used as a common model of user adoption behavior for new technologies, explaining how perceived usefulness and ease of use affect the user adoption decision process. On the basis of TAM, Venkatesh et al. (2003) proposed the UTAUT, in which users’ behavioral intentions are influenced by effort expectancy, performance expectancy, social influence and facilitating conditions. Alaiad et al. (2019) argued that the UTAUT model could reflect about 70 percent of the variables in users’ intention of adoption. To enhance the understanding of the extent of technology adoption in the UTAUT model, Venkatesh et al. (2012) proposed UTAUT2 with the addition of variables of hedonic motivation, price value, and habit. With the emerging technologies, the UTAUT model has been frequently applied to users’ adoption behaviors in various health-related studies, including information systems (Hadji and Degouelt, 2016), medical institutions (Sun and Rau, 2015), and mobile healthcare (Nisha et al., 2019) and telehealth care services (Park, 2009).
In the context of mHealth services, Shiferaw and Mehari (2019) introduced self-efficacy into the research on the acceptance behavior of electronic medical record systems by doctors and nurses based on the UTAUT model, and found that self-efficacy, effort expectancy, performance expectancy, social influence and facilitating conditions significantly and positively affected the actual usage behavior of users. Similarly, Nisha et al. (2019) chose the UTAUT model as their theoretical framework to explore the factors that affected the users’ intention of adoption of mHealth in Bangladesh. The results showed that new users were particularly sensitive to effort expectancy, performance expectancy, social influence, facilitating conditions and trust, which could significantly promote the usage intention of mHealth. In addition, based on PMT and UTAUT model, Hsieh et al. (2017) showed that perceived ease of use, self-efficacy and perceived usefulness were the important factors that affected behavioral intention of personal health record.
Although TAM, UTAUT and their extended models are useful, many efforts have been made to improve their explanatory power. The Theory of Reasoned Action (TRA) has proven to be one of the effective models to explain users’ behavioral intentions (Fishbein and Ajzen, 1975). Individuals are always rational in the behavioral decision making, and the actual behavior made by users is mainly influenced by behavior intention, while attitude toward behavior and subjective norm are two important factors that influence users’ intention to adopt. Subjective norm refers to the pressure exerted by people or organizations that have a significant influence on the user when making behavioral decisions and is equivalent to the variable of social influence in UTAUT (Taylor and Todd, 1995a). When the user’s attitude toward a behavior is positive and the person with significant influence also suggests the user to adopt the behavior, it will reinforce the user to produce the actual usage behavior. In this study, subjective norm from the TRA was selected as an individual cognitive factor to explore the decision process of users’ intention to adopt.
The Health Belief Model (HBM) has been widely used in the study of user information technology adoption behavior and proven as an effective model to explain behavioral intention. According to the HBM, human behavior is determined by health beliefs, behavioral cues or intentions, and constraints on behavior. Kim and Park (2012) constructed mHealth users’ adoption model from three dimensions: health, information and technology, based on TAM and Health belief model. Comparing TAM and HBM predictions of user behavioral intention, Mathieson (1991) concluded that both theories were appropriate. The former model is easy to be applied, while the latter can capture most aspects of an individual’s beliefs through a large number of variables (Chuttur, 2009).
With the development of mHealth services, privacy concerns are gaining attraction from researchers. In mHealth services contexts, users have relatively limited control over health data collection and usage, and are therefore more likely to suffer losses from privacy breaches. The privacy-personalization paradox has been developed to explain users’ behavioral intentions. Users’ desire for a wide range of personalized services requires service providers to collect more personal information, yet users are reluctant to disclose, leading to an apparent technological paradox (Awad and Krishnan, 2006). Milholland (1994) argued that the electronic storage of personal information and medical records posed a threat to user privacy. This threat is exacerbated by mHealth Apps. Privacy concern is defined as the extent to which individuals are concerned about the security of their privacy information (Cocosila and Archer, 2010). Based on the privacy-personalization paradox, Guo et al. (2016) constructed an adoption intention model including privacy concern, perceived personalization and trust, and the findings showed that privacy concern had a significant negative impact on the intention of adoption.
Previous studies on mHealth user adoption behavior based on TAM, UTAUT, TRA related or independent conceptual models are summarized in Figure 1. In this study, based on the UTAUT model, we replace social influence in UTAUT by subjective norm. Ajzen (1991) argued that subjective norm was an important factor to explain and predict the user’s usage behavior of information system. Meanwhile, perceived ubiquity is proposed in the field of information technology and is similar to perceived mobility of services, i.e., the extent to which users can access information or services anytime and anywhere (Amberg et al., 2004). Compared to traditional access channels, the most important feature of mHealth is the ubiquity of services that enables users to access mHealth services anytime, anywhere in any situation. We replace facilitating conditions in UTAUT by perceived ubiquity, which is more in line with the significant features that influence potential users’ adoption behavior in the mobile technology context. Extending the UTAUT model (i.e., effort expectancy, performance expectancy, subjective norm, and perceived ubiquity) by introducing two prediction variables of self-efficacy and privacy concerns in, we comprehensively examine the effect of self-efficacy and privacy concerns on the adoption of mHealth services in China.
Effort expectancy refers to the level of effort that users believe is required to use the new technology. Studies have confirmed that effort expectancy is equivalent to perceived ease of use in TAM (Wu and Wang, 2005; Schaper and Pervan, 2007), and the effort expectancy of different user groups varies. Effort expectancy has a significant positive effect on users’ behavioral intention (Kim et al., 2015; Duarte and Pinho, 2019). Regarding mHealth services, if users perceive the simpler use or operation of mHealth services is and the less effort (including time, energy, etc.) they need to make, the stronger their intention to adopt mHealth services will be. In summary, this study proposes the following hypothesis:
Hypothesis 1: Effort expectancy has a positive effect on users’ intention to adopt mHealth services.
Performance expectancy refers to the user’s judgment that the mHealth services are beneficial to him. Many studies have shown that performance expectancy can directly and significantly affect users’ intention to adopt (Wu et al., 2011; Ifinedo, 2012). In the UTAUT model, Venkatesh et al. (2003) proposed and confirmed that performance expectancy had a significant positive effect on individuals’ behavioral intentions. Semiz and Semiz (2021) also reported that performance expectancy had an important impact on users’ intention to use mHealth Apps in their survey. Mobile healthcare can provide users with timely and valuable information resources, which can significantly reduce the time for users to queue for registration, saving much time, energy and physical costs. Therefore, we speculate that the higher the users perceive the usefulness of mHealth, the stronger their intention to adopt mHealth will be. In summary, this study proposes the following hypothesis:
Hypothesis 2: Performance expectancy has a positive effect on users’ intention to adopt mHealth services.
In this study, subjective norm refers to the degree to which users perceive those important persons who want or do not want them to use mHealth, similar to the social influence in UTAUT (Taylor and Todd, 1995b). Ajzen (1991) argued that subjective norm was an important factor to explain and predict the user’s usage behavior of information system. mHealth is an innovative App of “mobile technology + medical service.” Therefore, when users decide whether to adopt mHealth services, they often make decisions following others’ advices. In fact, the particularity of mHealth services makes subjective norm play a very important role in users’ intention of adoption. When important persons (relatives, friends, experts) recommend them to use mHealth services, their trust in products or services will be significantly improved, and the intention to use mHealth will be stronger (Semiz and Semiz, 2021). In summary, this study proposes the following hypothesis:
Hypothesis 3: Subjective norm has a positive effect on users’ intention to adopt mHealth services.
Service ubiquity is a distinguishing feature of mobile technology, similar to the facilitating conditions in UTAUT (Okazaki and Mendez, 2013; Hsiao and Chen, 2015). Qian and Niu (2019) showed that the perceived ubiquity of mobile payment business had a positive influence on Alipay user adoption. The ubiquity of mHealth services is reflected in the possibilities for users to making appointment, seeking treatment, and inquiring about medical information or services anytime, anywhere, which brings users more freedom and convenience and effectively improve the efficiency of medical services. The higher the user’s perception of the ubiquity of mHealth services is, the higher satisfaction the users gain from their continuous use of mHealth services (Sneha and Varshney, 2009). At the same time, based on a meta-analysis, Zhu et al. (2020) also confirmed that perceived ubiquity had a significant positive effect on users’ intention to adopt mHealth. Their results showed that the feature that mobile healthcare was not limited by time, space and situation greatly improved the healthcare efficiency and users’ intention to adopt. Consequently, this study proposes the following hypothesis:
Hypothesis 4: Perceived ubiquity has a positive effect on users’ intention to adopt mHealth services.
Self-efficacy refers to a user’s self-perception of his/her own abilities, essentially an individual’s subjective judgment, which can significantly affect the user’s intention to adopt (Balapour et al., 2019; Shiferaw and Mehari, 2019). Gagnon et al. (2014) found that when users were confident in their ability to use new technologies, they felt that the operation was easier. In the Chinese domestic healthcare industry, self-efficacy has a significant positive influence on users’ effort expectancy and performance expectancy (Zhu and Liu, 2016). Users with low self-efficacy will think that the operation of mHealth is more complicated, so they are less likely to use the services. When users believe that they have sufficient ability to use mobile technology, they will have a positive opinion of mobile healthcare, and believe that the use of mHealth services has brought convenience for them. Therefore, this study proposes the following hypotheses:
Hypothesis 5: User’s self-efficacy has a positive effect on effort expectancy.
Hypothesis 6: User’s self-efficacy has a positive effect on performance expectancy.
In the context of mHealth services, self-efficacy is an important factor affecting users’ intention to adopt (Wu et al., 2007; Weimer et al., 2017; Chao, 2019). Specifically, self-efficacy has a positive effect on subjective norm (Cho et al., 2015). If users have high self-efficacy and believe that they can learn or have the skills to use mHealth proficiently, this confidence may strengthen the willingness of people around them to use mHealth services. On the contrary, when the users’ self-efficacy is low, they may reduce the use of mHealth services to a certain extent, and even negatively affect the adoption behavior of the surrounding people. The stronger the sense of self-efficacy, the stronger the user’s confidence in using mHealth services, which leads to stronger subjective norm. Thus, this study proposes the following hypothesis:
Hypothesis 7: User’s self-efficacy has a positive effect on subjective norm.
Meanwhile, self-efficacy also influences perceived ubiquity to a certain degree. Studies have shown that the higher the user’s self-efficacy in the medical field, the stronger their perception of the service’s ubiquity, and the more positive their attitude toward mHealth services (Jian et al., 2012; Vanneste et al., 2013). In other words, when users have a high level of self-efficacy and are confident that they can obtain mHealth services by completing the operation process, they are more likely to accept or adopt mHealth services, and then believe that mHealth services are ubiquitous. Therefore, this study believes that when users have high perception of their ability or confidence in using mHealth service, the more ubiquitous they perceive mHealth services in their lives. Based on this claim, this study proposes the following hypothesis:
Hypothesis 8: User’s self-efficacy has a positive effect on perceived ubiquity.
In the study of information technology adoption, self-efficacy is often reported to have an effect on the intention of adoption as a user’s individual cognitive factor. Users with low self-efficacy are less likely to adopt a certain behavior (Bandura, 1986). Hsu and Chiu (2004) illustrated that self-efficacy was an important factor to explain consumers’ usage decisions in e-commerce, and confirmed that users’ self-efficacy had a positive influence on usage intention. Zhang et al. (2017) explored the influential factors of adoption behavior of mHealth service users. The results showed that self-efficacy played an important role in users’ intention of adoption. Fox and Connolly (2018) also found that the self-efficacy had a significant positive effect on intention to adopt mHealth services. Consequently, this study proposes the following hypothesis:
Hypothesis 9: User’s self-efficacy has a positive effect on intention to adopt mHealth services.
Privacy concerns are defined as the degree to which users are concerned about the disclosure of personal information (Cocosila and Archer, 2010). In the context of mHealth services, when users do not know about or are not familiar with the services, they are afraid of leakage or abuse of their own privacy, especially if their information is used for other purposes without authorization, resulting in privacy leakage, property loss and other adverse consequences (Zhu et al., 2020). Many mHealth services involve personal health data and are carried out in a virtual network environment. Users’ privacy concerns will weaken their perception of the ease of use of the services, that is, users’ concerns about privacy will significantly reduce their effort expectancy. Therefore, this study proposes the following hypothesis:
Hypothesis 10: Users’ privacy concerns negatively influence effort expectancy.
Furthermore, health data are regarded as absolutely private information (Matteo et al., 2018), and users’ concerns about health data security and privacy are one of the reasons why they do not adopt or continue to use mHealth services (Wang and Qi, 2021). As mobile healthcare is in a rapid development period, users are uncertain about the security of mHealth services and may worry that their private information and health data might be leaked. It is not difficult to find that when users believe that the adoption of mHealth services may bring them risk of privacy, it will directly reduce their perception of the usefulness of mHealth services, that is, users’ privacy concerns negatively affect performance expectancy. Based on this claim, this study proposes the following hypothesis:
Hypothesis 11: Users’ privacy concerns negatively influence performance expectancy.
Privacy concerns are a factor that cannot be ignored in the adoption of mHealth services. The negative effect of privacy concerns on users’ intention to adopt mHealth services has been confirmed by many studies (Wiljer et al., 2008; Najaftorkaman et al., 2015). For example, the collection, processing, analysis and storage of personal health date in the use of mHealth services make users worry that third parties may leak their private information, which triggers users’ privacy concerns (Kaushik et al., 2018). Concerns about the leakage of medical information can reduce users’ intention of adoption (Anna and Jouni, 2018). Gagnon et al. (2016a) found that privacy security was one of the main factors hindering the widespread adoption and use of personal e-health systems. At the same time, Guo et al. (2016) also claimed that privacy concerns had a negative effect on users’ intention to adopt mHealth services in their study of the privacy paradox phenomenon in mHealth services. Therefore, this study proposes the following hypothesis:
Hypothesis 12: Users’ privacy concerns have a negative effect on intention to adopt mHealth services.
The use of mHealth services involves a large amount of personal health data including basic personal data, which raises concerns about privacy. Because mobile healthcare is an emerging technology service, users are more sensitive to privacy protection. If users believe that there are privacy and security issues in using mHealth services, they may reject suggestions from others and react negatively to adopt mHealth services (Najaftorkaman et al., 2015; Gagnon et al., 2016b). This is even more obvious in the healthcare context in China. When one thinks that there may be privacy and security issues in the use of mobile healthcare, one may decline or reject the advice from important people around, even doctors, nurses and other professionals, which will significantly weaken the intention to adopt mHealth services (Hsiao and Chen, 2015). Thus, this study proposes the following hypothesis:
Hypothesis 13: Users’ privacy concerns negatively influence subjective norm.
Regarding mHealth services, when users’ privacy concerns are high, their perceived ubiquity of mHealth services will become weaker. Worrying about the leakage of individual private information, they are resistant to mHealth services, which may automatically block the convenience and mobility of mHealth services. Users’ perception of ubiquity in mHealth services is reduced, leading to lower intention of adoption (Kim and Park, 2012; Guo et al., 2016). This study believes that mHealth services are different from other technical services. Because mHealth services are closely related to personal health and personal data, users are more concerned about the privacy issues, which will cause users to automatically block the ubiquity of mHealth service. Consequently, this study proposes the following hypothesis:
Hypothesis 14: Users’ privacy concerns have a negative effect on perceived ubiquity.
By reviewing related theoretical models and literature on users’ intention to adopt mHealth services, this study constructs a model of users’ intention to adopt mHealth services based on the UTAUT model and takes the individual cognitive factors - self-efficacy and privacy concerns – into consideration. The specific conceptual model is shown in Figure 2.
In order to make the participants fully understand mHealth, the questionnaire started with a detailed introduction to the mHealth services and channels. The first part of the questionnaire set the screening question “Have you ever searched for disease or medical information on the mobile devices?” If the participant answered “No,” the questionnaire was regarded as invalid. The second part consisted of latent variable items. In order to ensure the validity of the measurement, all the items were adapted from mature scales from home and abroad and modified to make them relevant to the context of mHealth in China.
Specifically, effort expectancy and performance expectancy mainly referred to the scale of Venkatesh et al. (2003). Subjective norm, perceived ubiquity, self-efficacy, and privacy concerns were derived from the scales of Lee (2005), Cocosila and Archer (2010), Holden and Karsh (2010), and Guo et al. (2012), and intention to adopt came from the scale of Bhattacherjee (2001a) (see Table 2 for details). All scales were measured using a 7-point Likert-type scale (e.g., 1 = Strongly disagree, 7 = Strongly agree). The third part of the questionnaire included demographic questions and questions about the use of mobile healthcare. Before the final questionnaire was issued, it was first sent to experts in medical-related fields for review and then modified based on their feedback. A pilot testing of questionnaire by 30 respondents was conducted through the platform of Questionnaire Star to ensure the reliability and validity of the questionnaire items, the appropriate difficulty level and rational layout. Participants answered the questions and submitted the questionnaires through the link of Questionnaire Star, a professional online questionnaire survey, evaluation and voting platform. The creator of the questionnaire can download or analyze the data on the Questionnaire Star server (Duan et al., 2020).
This study used a combination of online and on paper survey to collect empirical data. In the first round, the electronic questionnaire was designed on the questionnaire star website. The questionnaire links were released through instant messaging tools, such as WeChat and QQ, and the secondary release was carried out in a snowball manner. A total of 447 questionnaires were collected. In the second round, 100 paper questionnaires were randomly distributed around a large hospital in China, and 100 questionnaires were recovered. A total of 547 questionnaires were collected in the two rounds, of which 95 questionnaires were excluded due to “no intention to use mHealth,” and 48 samples with the same choices for the items in one construct, 2 samples with the same answers completely, and 16 samples with the mean value greater than or less than 2 SD were deleted (Hair et al., 2014). A total of 386 valid questionnaires were retained, with a valid rate of 70.6%. Among the participants in this survey, there were 204 females, accounting for 52.8%, and 182 males, accounting for 47.2%, with a reasonable gender ratio. In terms of education background, the majority of participants were undergraduates, accounting for about 65%, and nearly 20% of participants had a master’s degree or above. It can be seen that more than 80% of participants have higher education background, indicating that the participants have the ability to effectively fill in the questionnaire, which ensures the effectiveness of data collection to a certain extent. The specific descriptive statistics are listed in Table 3.
From the questions about the usage of mHealth services (Table 4), 87.3% of participants have used mHealth services, and nearly 80% of participants have used mHealth services more than twice, indicating that most of the participants have the experience of using mHealth services. They can better fill out the questionnaire based on their personal experience, thus ensuring the accuracy and reliability of the data. The channels through which the participants mainly use mHealth services include the hospital’s official website, WeChat public account and mini programs, accounting for 89.6%. The mHealth services that the participants mainly engage in are making appointment, viewing department and doctor information, and checking about queuing and calling information. In addition, 10.4% of participants used mHealth services before but no longer use it now. The important reasons for participants to stop using mHealth are “No habit of using mHealth services” and “Unguaranteed professionalism and reliability of information,” as shown in Table 5.
To test the reliability and validity of the data, SPSS 25.0 and AMOS 24.0 were used in this study. At the same time, the least squares PLS structural equation model was used to test the posited hypotheses. The proposed model was revised according to the path analysis results to construct the final revised model of intention to adopt mHealth services.
In addition, the Harman single factor test was used to estimate the Common Source Bias (Harris and Mossholder, 1996). This systematic error will make the measurement results deviated from the facts due to the characteristics of subjects, context of items and the single or similar data sources. Podsakoff et al. (2003) suggested that unrotated principal component analysis could be performed on all items at the same time. If no unique factor is formed, the influence of homology bias is insignificant. The test results show that seven factors with eigenvalues greater than 1 were formed without rotation, and the first principal component obtained was 39.74% < 50% (Hair et al., 2007), indicating that there is no serious problem of homologous bias in this study.
Table 6 presents the standardized factor loadings, AVE values, CR values and Cronbach’ a coefficient for each latent construct. Confirmatory factor analysis (CFA) was performed on 33 items of latent constructs. The results show that the CFA model fitting index is χ2 = 1274.863, d.f. = 474, χ2/d.f. = 2.690, GFI = 0.825, CFI = 0.919, NFI = 0.878, IFI = 0.920, RMSEA = 0.066, indicating the model has a satisfactory fit. The standard loading coefficients of all factors were between 0.538 and 0.922, which met the validity requirements. The average extraction variance (AVE) value was greater than 0.5, and the CR value and Cronbach’ a value were greater than 0.8. From the above indicators, it can be concluded that the questionnaire meets the requirements of internal consistency reliability and has good convergent validity.
The correlations between constructs are presented in Table 7. Except that the correlation coefficient between performance expectancy and subjective norm was slightly higher than the square root of AVE of subjective norm, the square root of AVE of other constructs was significantly larger than the correlation coefficient between this construct and other constructs, indicating that the measurement model had satisfactory discriminant validity.
AMOS24.0 was used for the path analysis of the structural equation model. The path coefficients and significance levels are shown in Figure 3, and the fitting degree of the model before revision is presented in Table 8. According to the data before revision in Table 8, the overall fitting index of the structural model was poor, so the original hypothetical model was revised accordingly (Whittaker, 2011). In order to improve the fitting degree of the structural model, the model was revised according to Modification Indices in AMOS24.0. The model was revised with the principle of “modification with the highest parameters at a time” (Whittaker, 2011, pp. 694–701). The revised model is shown in Figure 3, and the overall fitting index after the model revision is shown in Table 8. The fitting indices after the model revision were all within the reference value range, so the revised model was acceptable.
Figure 3. The model of mHealth users’ intention to adopt before correction (standardized path coefficient). *p < 0.05, **p < 0.01, ***p < 0.001. The dotted line indicates that the path relationship is insignificant.
Based on the revised model, we examined the effects of self-efficacy, privacy concerns, effort expectancy, performance expectancy, subjective norm, and perceived service ubiquity on intention to adopt mHealth services. The standardized path coefficients were obtained with a maximum likelihood estimation (Figure 4). It can be seen from the significance of the standardized path coefficient that the four related constructs of the UTAUT model positively and significantly influenced the participants’ intention to adopt mHealth services. Self-efficacy had a significantly positive effect on users’ intention to adopt through effort expectancy, performance expectancy, subjective norm and perceived ubiquity. Privacy concerns only negatively influenced perceived ubiquity, but had no significant effect on effort expectancy, performance expectancy and subjective norm. In addition, the SMCs of effort expectancy, performance expectancy, subjective norm, perceived ubiquity, and intention to adopt were 0.483, 0.352, 0.325, 0.532, and 0.527, respectively, indicating that the model had high explanatory power.
Figure 4. The model of mHealth users’ intention to adopt after correction (standardized path coefficient). p < 0.05, p < 0.01, p < 0.001.
This study aims to investigate users’ intention to adopt mHealth services based on the UTAUT theoretical model. Results from the data analysis provide support for our proposed theoretical model.
First, by analyzing the standardized path coefficients of the original hypothetical model, it can be seen that the main factors affecting users’ intention to adopt mHealth are subjective norm, self-efficacy and performance expectancy. Min et al. (2008); Zhang et al. (2017), Nunes et al. (2019), and Qian and Niu (2019) have all confirmed that these variables have a significant influence on users’ intention to adopt in the fields of mHealth App, mobile payment, mobile shopping and other information systems. In the context of mHealth services, the conclusions of previous studies still hold.
Second, the original hypotheses H2, H3, H5, H6, H7, H8, and H14 are strongly supported both before and after the revision of the model. Both performance expectancy and subjective norm significantly and positively affect users’ intention to adopt mHealth services, among which subjective norm has a greater impact on intention to adopt (β = 0.406, P < 0.001), followed by performance expectancy (β = 0.176, P < 0.05), indicating that mHealth services indeed increase the convenience of life and increase the willingness of users to use them. In addition, self-efficacy has a significant positive effect on perceived ubiquitous (β = 0.731, P < 0.001), effort expectancy (β = 0.704, P < 0.001), performance expectancy (β = 0.685, P < 0.001) and subjective norm (β = 0.685, P < 0.001), indicating that self-efficacy can comprehensively improve users’ perception of mHealth services, and thus improve their willingness to use mHealth services. Privacy concerns have a significantly negative impact on perceived ubiquity (β = –0.086, P < 0.05). The higher the user’s concerns about personal privacy, the more likely they are to seek medical treatment through traditional medical service channels, that is, the perceived ubiquity of mHealth services is weakened.
Third, the hypotheses H1, H4, H10, H11, H12, and H13 are not supported in the model before revision, that is, the user’s effort expectancy, privacy concerns and perceived service ubiquity have insignificant effect on intention to adopt mHealth services, while privacy concerns have insignificant impact on effort expectancy, performance expectancy and subjective norm. Of mHealth service users, the youth accounts for more than 70%. They often come into contact with various mobile Apps and have good information literacy. When using mHealth services, they can deal with common operational problems independently. Therefore, effort expectancy and perceived ubiquity do not have significant effect on intention of adoption. In addition, the reason for the insignificant effect of privacy concerns is probably that the young and middle-aged respondents in the sample, who constituted more than half of the mHealth users, were more ready to accept new things and preferred to adopt more convenient mHealth service. Because the youth are less sensitive to personal privacy, and most of the mHealth service channels used by them are highly authoritative platforms such as hospital official websites, WeChat public accounts, and provincial and municipal medical platforms, which shows that information and services are more trustworthy, privacy concerns do not have a significant effect on users’ intention to adopt mHealth services.
Fourth, in the revised users’ intention to adopt mHealth service model, self-efficacy significantly and positively affects users’ effort expectancy (β = 0.695, P < 0.001), performance expectancy (β = 0.594, P < 0.001), subjective norm (β = 0.568, P < 0.001) and perceived ubiquity (β = 0.727, P < 0.001). The stronger the user’s self-efficacy, the stronger the intention to adopt mHealth services. For the four variables in the UTAUT model, effort expectancy (β = 0.128, P < 0.05), performance expectancy (β = 0.244, P < 0.001), subjective norm (β = 0.390, P < 0.001) and perceived ubiquity (β = 0.198, P < 0.001) can significantly and positively influence users’ intention to adopt mHealth services. Studies by Venkatesh et al. (2003) have verified that effort expectancy and performance expectancy significantly affect users’ intention to adopt. Zhu et al. (2020) also confirmed that subjective norm and perceived ubiquity had a positive effect on users’ intention of adoption. It is easy to find that the conclusions of previous studies still hold in the context of mHealth services.
Compared with the existing literature, this paper focuses on the user’s individual cognitive factors and uses the UTAUT theory to analyze the influence of the user’s self-efficacy and privacy concerns on the intention to adopt mHealth services, providing several implications for theory. On the one hand, creatively building an adoption model, this study provides more comprehensive prediction and explanation of users’ adoption behavior of mHealth services by introducing self-efficacy and privacy concerns into the UTAUT model. On the other hand, most of the existing studies focus on the external characteristics, system design, technical environment and usage context of mHealth and many other factors (Chen et al., 2018; Nadal et al., 2020; Wu et al., 2020), but few studies have analyzed the influence of user’s subjective perception on adoption behavior. This paper expands the research perspective of users’ adoption behavior of mHealth service by examining individual cognitive factors and comprehensively considering the direct or indirect effects of users’ self-efficacy and privacy concerns on the intention of adoption.
In China’s COVID-19 environment, mHealth services help solve many problems of users’ access to medical care in an epidemic control state. Through mobile terminal devices, users can easily and quickly access various medical services, which reduces the mobility of people to a certain extent and meets the urgent need for epidemic prevention and control. This study conducts an in-depth investigation of users’ intention to adopt mHealth services in China, which helps domestic and foreign mHealth service providers and developers to better optimize the design of mHealth services while enhancing users’ intention to adopt them.
First, the finding in this study suggests that users’ self-efficacy be enhanced by optimizing product design. The stronger the users’ self-efficacy is, the more confident they are in using mHealth services, and the stronger intention they will have to adopt mHealth services. Service providers should optimize product design and user experience, such as providing one-click functional services, simplifying the operation of different sections and improving the ease of use of the interface, so as to enhance users’ confidence in using mHealth services. At the same time, appropriate education on how to use mHealth services should be carried out to improve users’ ability to retrieve, obtain and use medical information or services.
Second, it is desirable that subjective norm should be enhanced by strengthening word-of-mouth communication and recommendation from opinion leaders. The platform should increase the publicity and promotion of typical service cases, paying attention to word-of-mouth communication. The key opinion leaders and experts in the medical field should appropriately recommend mHealth services to users, further expanding the effect of the subjective norm. In addition, service providers should make full use of the trust that users have for the hospitals and doctors to facilitate the adoption of mHealth services of potential users.
Third, it is suggested that the level of privacy security management should be improved in technology, legislation and supervision. It is very important to promote people’s awareness of privacy protection. The service providers should pay close attention to the technical development of privacy protection and make up for the privacy loopholes in the information system, preventing privacy leakage caused by improper technical operation or lack of morality. In addition, the supervision department should strengthen the supervision of mHealth services, formulate relevant laws and regulations, and strengthen the risk assessment and supervision of the operation of mHealth service providers, effectively ensuring the security of users’ private information.
Fourth, the study also implies that the quality of mHealth services should be improved by enhancing the ease of use of the system. The service providers should make effort to simplify the operation interface and operation process, effectively reducing the cost of use. The platform services should be enriched, and the information resources should be updated in time. The users’ inquiries, registration and queuing information should be responded to in a timely manner. In addition, the intelligent recommendations should be added on the medical platform. Personalized recommendations based on full user history, recent activity, or consultation record should be enhanced so that the user can enjoy a better experience and have a sense of acquisition.
Fifth, it is reasonable for service provider to enhance users’ perceived ubiquity by increasing the publicity of the service ubiquity, such as being free from time and space constraints, real-time interaction, convenience and flexibility. The more people know about the convenience that mHealth services bring, the stronger intention of adoption people will have for such services. The platform can also connect users’ hospital treatment data and mHealth service data with smart wearable devices, allowing users to enjoy personalized medical services anytime, anywhere.
Currently, countries around the world are facing healthcare issues, such as imbalance of healthcare resources and healthcare reform, to varying degrees. UNSDGs are 17 global development goals set by the United Nations to guide global social, economic and environmental development from 2015 to 2030. No. 3 of UNSDGs is ensuring healthy lifestyles and promoting well-being for all at all ages. mHealth services can effectively address the problem of unequal distribution of healthcare resources, improve healthcare coverage, access to medical information, services and skills, as well as promote positive changes in health behaviors, such as in the prevention of emergency and chronic diseases. This study investigates the factors influencing mHealth users’ intention to adopt mHealth by combining the characteristics of mHealth services and users’ individual cognitive factors. The findings can help mHealth service providers provide accurate and effective mHealth services to users, continuously improving users’ access experience and usage stickiness. More importantly, the results of the study will facilitate mHealth services to be widely recognized and used, promoting the sustainable development of mHealth services.
This study created an integrated model to explain the determinants of users’ intention to adopt toward using mHealth service at the individual cognitive level, extending the UTAUT model by introducing two prediction variables of self-efficacy and privacy concerns. Data were collected from 386 domestic users in China with experiences of using mHealth services. The results reveal that self-efficacy was a key factor that significantly influenced users’ intention to adopt mHealth services. In addition, this study also exhibited that effort expectancy, performance expectancy, subjective norm, and perceived ubiquity all positively influenced users’ intention to adopt. Finally, privacy concerns only had a significantly negative effect on perceived ubiquity, while their effects on effort expectancy, performance expectancy, subjective norm, and intention to adopt were not significant. This study has demonstrated its values to the mHealth service providers by explaining the role of individual perceptions in the decision process of the adoption.
This study also has certain limitations. Firstly, it only studies the user’s intention of adoption, but the intention to adopt is not equal to the behavior of adoption (King and He, 2006). In the future, new variables will be further introduced to explore the user’s intention of adoption and intention of continuous use. Secondly, the questionnaire survey is mainly conducted among 18–40 years old young and middle-aged groups, which cannot fully reflect the adoption behaviors of different age groups. Finally, the scope of mHealth services in the survey is somewhat broad, and users mainly use mHealth services for making appointment, which to a certain extent dilutes or even weakens the effect of privacy concerns on intention of adoption. Therefore, in future research, the samples of the questionnaire survey should be expanded, such as users over the age of 40 such as patients, women and the elderly, and a stratified sampling survey should be conducted in different age groups. The method of “scenario experiment + questionnaire survey” can be used to more comprehensively and empirically investigate the use of mHealth services among different user groups.
The raw data supporting the conclusions of this article will be made available by the authors, without undue reservation.
Ethical review and approval was not required for the study on human participants in accordance with the local legislation and institutional requirements. The patients/participants provided their written informed consent to participate in this study.
YL contributed to the study design and wrote the manuscript drafts. CL supervised the study and provided suggestions for the revision of the manuscript drafts. XL contributed to the analysis of the data. GZ and JS provided some reviews on the manuscript. All authors contributed to the article and approved the submitted version.
This research was supported by the Research on Risk Cognition and Management Strategy of the Acquirement of Online Healthcare Information under Grant Number: 71673245.
The authors wish to appreciate Qian Chen for her guidance during the translation of the manuscript.
The authors declare that the research was conducted in the absence of any commercial or financial relationships that could be construed as a potential conflict of interest.
All claims expressed in this article are solely those of the authors and do not necessarily represent those of their affiliated organizations, or those of the publisher, the editors and the reviewers. Any product that may be evaluated in this article, or claim that may be made by its manufacturer, is not guaranteed or endorsed by the publisher.
The Supplementary Material for this article can be found online at: https://www.frontiersin.org/articles/10.3389/fpsyg.2022.944976/full#supplementary-material
Ajzen, I. (1991). The theory of planned behavior. Organ. Behav. Hum. Dec. 50, 179–211. doi: 10.1016/0749-5978(91)90020-T
Alaiad, A., Alsharo, M., and Alnsour, Y. (2019). The determinants of M-Health adoption in developing countries: An empirical investigation. Appl. Clin. Inform. 10, 820–840. doi: 10.1055/s-0039-1697906
Alam, M. Z., Hu, W., Kaium, M. A., Hoque, M. R., and Alam, M. M. D. (2020). Understanding the determinants of mHealth applications adoption in Bangladesh: A SEM-Neural network approach. Technol. Soc. 61:101255. doi: 10.1016/j.techsoc.2020.101255
AlBar, A. M., and Hoque, M. R. (2019). Patient acceptance of e-health services in Saudi Arabia: An integrative perspective. Telemed. J. E-Health 25, 847–852. doi: 10.1089/tmj.2018.0107
Amberg, M., Hirschmeier, M., and Wehrmann, J. (2004). The compass acceptance model for the analysis and evaluation of mobile services. Int. J. Mobile Commun. 2, 248–259. doi: 10.1504/ijmc.2004.005163
Andronie, M., Lãzãroiu, G., Ştefãnescu, R., Ionescu, L., and Cocoşatu, M. (2021b). Neuromanagement Decision-Making and Cognitive Algorithmic Processes in the Technological Adoption of Mobile Commerce applications. Oecon. Copernica. 12, 863–888. doi: 10.24136/oc.2021.028
Andronie, M., Lãzãroiu, G., Iatagan, M., U tã, C., Ştefãnescu, R., and Cocoşatu, M. (2021a). Artificial Intelligence-Based Decision-Making Algorithms, Internet of Things Sensing Networks, and Deep Learning-Assisted Smart Process Management in Cyber-Physical Production Systems. Electronics 10:2497. doi: 10.3390/electronics10202497
Anna, R., and Jouni, M. (2018). Development of personal information privacy concerns evaluation. Encyclopedia. Inform. Sci. Technol. 117–126. doi: 10.4018/978-1-5225-2255-3.ch421
Awad, N. F., and Krishnan, M. S. (2006). The personalization privacy paradox: An empirical evaluation of information transparency and the willingness to be profiled online for personalization. MIS Q. 30, 13–12. doi: 10.2307/25148715
Bai, M. Y., Mobbs, R. J., Walsh, W. R., and Betteridge, C. (2020). mHealth applications for enhanced management of spinal surgery patients: A review. Front. Surg. 7:573398. doi: 10.3389/fsurg.2020.573398
Balapour, A., Reychav, I., Sabherwal, R., and Azuri, J. (2019). Mobile technology identity and self-efficacy: Implications for the adoption of clinically supported mobile health applications. Int. J. Inform. Manage. 49, 58–68. doi: 10.1016/j.ijinfomgt.2019.03.005
Balica, R. -Ş (2022). Networked Wearable Devices, Machine Learning-based Real-Time Data Sensing and Processing, and Internet of Medical Things in COVID-19 Diagnosis, Prognosis, and Treatment. Am. J. Med. Res. 9, 33–48. doi: 10.22381/ajmr9120223
Bally, E. L., and Cesuroglu, T. (2020). Toward integration of mHealth in primary care in the Netherlands: A qualitative analysis of stakeholder perspectives. Front. Public Health 7:407. doi: 10.3389/fpubh.2019.00407
Bandura, A. (1986). Social foundations of thought and action: A social cognitive theory. Englewood Cliffs, NJ: Prentice-hall, 1–60.
Bhattacherjee, A. (2001a). An empirical analysis of the antecedents of electronic commerce service continuance. Decis. Support Syst. 32, 201–214. doi: 10.1016/s0167-9236(01)00111-7
Bhattacherjee, A. (2001b). Understanding Information Systems Continuance: An Expectation-Confirmation Model. Mis. Quart. 25, 351–370. doi: 10.2307/3250921
Chao, C.-M. (2019). Factors Determining the Behavioral Intention to Use Mobile Learning: An APP and Extension of the UTAUT Model. Front. Psychol. 10:1652. doi: 10.3389/fpsyg.2019.01652
Chen, Y., Yang, L., Zhang, M., and Yang, J. (2018). Central or peripheral? Cognition elaboration cues’ effect on users’ continuance intention of mobile health applications in the developing markets. Int. J. Med. Inform. 116, 33–45. doi: 10.1016/j.ijmedinf.2018.04.008
Cheng, A., Ren, G., Hong, T., Nam, K., and Koo, C. (2019). “An exploratory analysis of travel-related WeChat mini program usage: Affordance theory perspective,” in Information and Communication Technologies in Tourism 2019, eds J. Pesonen and J. Neidhardt (Cham: Springer), 333–343. doi: 10.1007/978-3-030-05940-8_26
Cho, H., Chung, S., and Filippova, A. (2015). Perceptions of social norms surrounding digital piracy: The effect of social projection and communication exposure on injunctive and descriptive social norms. Comput. Hum. Behav. 48, 506–515. doi: 10.1016/j.chb.2015.02.018
Chuttur, M. (2009). Overview of the technology acceptance model: Origins, developments and future directions, Indiana University, USA: Working papers on information system, Vol. 9. Raleigh, CA: Sprouts.
Cocosila, M., and Archer, N. (2010). Adoption of Mobile ICT for Health Promotion: An Empirical Investigation. Electron. Mark. 20, 241–250. doi: 10.1007/s12525-010-0042-y
Crowell, B., Cug, J., and Katarina Michalikova, K. (2022). Smart Wearable Internet of Medical Things Technologies, Artificial Intelligence-based Diagnostic Algorithms, and Real-Time Healthcare Monitoring Systems in COVID-19 Detection and Treatment. Am. J. Med. Res. 9, 17–32. doi: 10.22381/ajmr9120222
Davis, F. D. (1989). Perceived usefulness, perceived ease of use, and user acceptance of information technology. MIS Q. 13, 319–340. doi: 10.2307/249008
Dehzad, F., Hilhorst, C., de Bie, C., and Claassen, E. (2014). Adopting health applications, what’s hindering doctors and patients? Health-london 6, 2204–2217. doi: 10.4236/health.2014.616256
Deng, W. J., Yeh, M. L., and Sung, M. L. (2013). A customer satisfaction index model for international tourist hotels: Integrating consumption emotions into the American Customer Satisfaction Index. Int. J. Hosp. Manage. 35, 133–140. doi: 10.1016/j.ijhm.2013.05.010
Deng, Z. (2013). Understanding public users’ adoption of mobile health service. Int. J. Mob. Commun. 11, 351–373. doi: 10.1504/IJMC.2013.055748
Deng, Z., Hong, Z., Ren, C., Zhang, W., and Xiang, F. (2018). What predicts patients’ adoption intention toward mHealth services in China: Empirical study. JMIR. Mhealth. Uhealth. 6:e172. doi: 10.2196/mhealth.9316
Duan, L., Shao, X., Wang, Y., Huang, Y., Miao, J., Yang, X., et al. (2020). An investigation of mental health status of children and adolescents in china during the outbreak of COVID-19. J. Affect. Disorders. 275, 112–118. doi: 10.1016/j.jad.2020.06.029
Duarte, P., and Pinho, J. C. (2019). A mixed methods UTAUT2-based approach to assess mobile health adoption. J. Bus. Res. 102, 140–150. doi: 10.1016/j.jbusres.2019.05.022
Fishbein, M., and Ajzen, I. (1975). Belief, attitude, intention, and behavior: An introduction to theory and research. Reading, MA: Addison-Wesley.
Fox, G., and Connolly, R. (2018). Mobile health technology adoption across generations: Narrowing the digital divide. Inform. Syst. J. 28, 995–1019. doi: 10.1111/isj.12179
Gagnon, M. P., Ngangue, P., Payne-Gagnon, J., and Desmartis, M. (2016a). m-Health adoption by healthcare professionals: A systematic review. J. Am. Med. Inform. Assoc. 23, 212–220. doi: 10.1093/jamia/ocv052
Gagnon, M. P., Payne-Gagnon, J., Breton, E., Fortin, J. P., Khoury, L., Dolovich, L., et al. (2016b). Adoption of electronic personal health records in Canada: Perceptions of stakeholders. Int. J. Health Policy 5, 425–433. doi: 10.15171/ijhpm.2016.36
Gagnon, M. P., Talla, P. K., Simonyan, D., Godin, G., Labrecque, M., Ouimet, M., et al. (2014). Electronic health record acceptance by physicians: Testing an integrated theoretical model. J. Biomed. Inform. 48, 17–27. doi: 10.1016/j.jbi.2013.10.010
Garavand, A., Samadbeik, M., Nadri, H., Rahimi, B., and Asadi, H. (2019). Effective factors in adoption of mobile health applications between medical sciences students using the UTAUT model. Methods Inf. Med. 58, 131–139. doi: 10.1055/s-0040-1701607
Geng, Z. (2016). An analysis of the US new media public diplomacy toward China on WeChat public account. Sociol. Study 6, 18–27. doi: 10.17265/2159-5526/2016.01.002
Guo, X., Han, X., Zhang, X., Dang, Y., and Chen, C. (2015). Investigating m-health acceptance from a protection motivation theory perspective: Gender and age differences. Telemed. J. E-health 21, 661–669. doi: 10.1089/tmj.2014.0166
Guo, X., Zhang, X., and Sun, Y. (2016). The privacy–personalization paradox in mHealth services acceptance of different age groups. Electron. Commer. R. A. 16, 55–65. doi: 10.1016/j.elerap.2015.11.001
Guo, X. T., Yuan, J. Q., Cao, X. F., and Chen, X. D. (2012). “Understanding the acceptance of health services: A service participants analysis,” in 2012 International Conference on Management Science & Engineering 19th Annual Conference Proceedings, (Dallas, TX: IEEE), 1868–1873. doi: 10.1109/icmse.2012.6414426
Hadji, B., and Degouelt, P. (2016). Information system end-user satisfaction and continuance intention: A unified modeling approach. J. Biomed. Inform. 61, 185–193. doi: 10.1016/j.jbi.2016.03.021
Hair, J. F., Babin, B. J., Anderson, R. E., and Black, W. C. (2014). Multivariate data analysis, 7th Pearson new international ed. Harlow: Pearson.
Hair, J. F., Black, B., Babin, B., Anderson, R. E., and Tatham, R. L. (2007). Multivariate data analysis:international edition. J. Rheumatol. 35, 357–358. doi: 10.1080/09553006814550601
Harris, S. G., and Mossholder, K. W. (1996). The affective implications of perceived congruence with culture dimensions during organizational transformation. J. Manage. 22, 527–547. doi: 10.1016/S0149-2063(96)90023-9
Holden, R. J., and Karsh, B. T. (2010). The technology acceptance model: Its past and its future in health care. J. Biomed. Inform. 43, 159–172. doi: 10.1016/j.jbi.2009.07.002
Hsiao, C. H., and Tang, K. Y. (2015). Examining a model of mobile healthcare technology acceptance by the elderly in Taiwan. J. Glob. Inf. Tech. Man. 18, 292–311. doi: 10.1080/1097198x.2015.1108099
Hsiao, M. H., and Chen, L. C. (2015). Smart phone demand: An empirical study on the relationships between phone handset, internet access and mobile services. Telemat. Inform. 32, 158–168. doi: 10.1016/j.tele.2014.06.001
Hsieh, H. L., Kuo, Y. M., Wang, S. R., Chuang, B. K., and Tsai, C. H. (2017). A study of personal health record user’s behavioral model based on the PMT and UTAUT integrative perspective. Int. J. Env. Res. Pub. He. 14:8. doi: 10.3390/ijerph14010008
Hsu, M., and Chiu, C. (2004). Internet self-efficacy and electronic service acceptance. Decis. Support. Syst. 38, 369–381. doi: 10.1016/j.dss.2003.08.001
Ifinedo, P. (2012). “Technology Acceptance by Health Professionals in Canada: An Analysis with a Modified UTAUT Model,” in Proceedings of the Annual Hawaii International Conference on System Sciences, (Maui, HI: IEEE), 2937–2946. doi: 10.1109/HICSS.2012.556
Istepanian, R., Laxminarayn, S., and Pattichis, C. S. (2007). M-health:emerging mobile health systems. Berlin: Springer.
Jenkins, T. (2022). Wearable Medical Sensor Devices, Machine and Deep Learning Algorithms, and Internet of Things-based Healthcare Systems in COVID-19 Patient Screening, Diagnosis, Monitoring, and Treatment. Am. J. Med. Res. 9, 49–64. doi: 10.22381/ajmr9120224
Jian, W. S., Syed-Abdul, S., Sood, S. P., Lee, P., Hsu, M. H., Ho, C. H., et al. (2012). Factors influencing consumer adoption of USB-based Personal Health Records in Taiwan. BMC Health Serv. Res. 12:277. doi: 10.1186/1472-6963-12-277
Jovanov, E., and Zhang, Y. T. (2004). Introduction to the special section on M-Health: Beyond seamless mobility and global wireless health-care connectivity. IEEE. T. Inf. Technol. 8, 405–414. doi: 10.1109/TITB.2004.840019
Kaushik, K., Kumar, J., and Kumar, S. A. (2018). Antecedents and outcomes of information privacy concerns: Role of subjective norm and social presence. Electron. Commer. R. A. 32, 57–68. doi: 10.1016/j.elerap.2018.11.003
Kim, J., and Park, H. A. (2012). Development of a health information technology acceptance model using consumers’ health behavior intention. J. Med. Internet. Res. 14:e133. doi: 10.2196/jmir.2143
Kim, S., Lee, K. H., Hwang, H., and Yoo, S. (2015). Analysis of the factors influencing healthcare professionals’ adoption of mobile electronic medical record (EMR) using the unified theory of acceptance and use of technology (UTAUT) in a tertiary hospital. BMC Med. Inform. Decis. 16:12. doi: 10.1186/s12911-016-0249-8
King, W. R., and He, J. (2006). A meta-analysis of the technology acceptance model. Inform. Managem-amster. 43, 740–755. doi: 10.1016/j.im.2006.05.003
Lãzãroiu, G., Andronie, M., Iatagan, M., Geamãnu, M., Ştefãnescu, R., and Dijmãrescu, I. (2022). Deep Learning-Assisted Smart Process Planning, Robotic Wireless Sensor Networks, and Geospatial Big Data Management Algorithms in the Internet of Manufacturing Things. ISPRS Int. J. Geo-Inform. 11:277. doi: 10.3390/ijgi11050277
Lee, D. (2021). Knowledge Gaps in Mobile Health Research for Promoting Physical Activity in Adults With Autism Spectrum Disorder. Front. Psychol. 12:635105. doi: 10.3389/fpsyg.2021.635105
Lee, E., and Han, S. (2015). Determinants of adoption of mobile health services. Online Inf. Rev. 39, 556–573. doi: 10.1108/oir-01-2015-0007
Lee, T. M. (2005). The Impact of Perceptions of Interactivity on Customer Trust and Transaction Intentions in Mobile Commerce. J. Electron. Commer. Re. 6, 165–180.
Li, H., Wu, J., Gao, Y., and Shi, Y. (2016). Examining individuals’ adoption of healthcare wearable devices: An empirical study from privacy calculus perspective. Int. J. Med. Inform. 88, 8–17. doi: 10.1016/j.ijmedinf.2015.12.010
Li, Q. (2020). Healthcare at Your Fingertips: The Acceptance and Adoption of Mobile Medical Treatment Services among Chinese Users. Int. J. Env. Res. Pub. He. 17:6895. doi: 10.3390/ijerph17186895
Lim, S., Xue, L., Yen, C. C., Chang, L., Chan, H. C., Tai, B. C., et al. (2011). A study on Singaporean women’s acceptance of using mobile phones to seek health information. Int. J. Med. Inform. 80, e189–e202. doi: 10.1016/j.ijmedinf.2011.08.007
Liu, F., Ngai, E., and Ju, X. (2019). Understanding mobile health service use: An investigation of routine and emergency use intentions. Int. J. Inform. Manage. 45, 107–117. doi: 10.1016/j.ijinfomgt.2018.09.004
Mathieson, K. (1991). Predicting user intentions: Comparing the technology acceptance model with the theory of planned behavior. Inf. Syst. Res. 2, 173–191. doi: 10.1287/isre.2.3.173
Matteo, D. D., Fine, A., Fotinos, K., Rose, J., and Katzman, M. (2018). Patient Willingness to Consent to Mobile Phone Data Collection for Mental Health applications: Structured Questionnaire. JMIR. Ment. Health 5:e56. doi: 10.2196/mental.9539
Meng, F., Guo, X., Zhang, X., Peng, Z., and Lai, K. H. (2020). “Examining the role of technology anxiety and health anxiety on elderly users’ continuance intention for mobile health services use,” in Proceedings of the 53rd hawaii international conference on system sciences, (Honolulu, HI: University of Hawai’i at Mânoa), doi: 10.24251/HICSS.2020.403
Miao, Y., Cui, T., and Jiang, B. (2018). “Research on Service Process Design of Mobile Medical Platform Based on Patient’s Emotional Demand,” in International Conference of Design, User Experience, and Usability, (Cham: Springer), 41–51. doi: 10.1007/978-3-319-91806-8_4
Milholland, D. K. (1994). Privacy and confidentiality of patient information. Challenges for nursing. J. Nurs. Adm. 24, 19–24. doi: 10.1097/00005110-199402000-00008
Min, Q., Ji, S., and Qu, G. (2008). Mobile commerce user acceptance study in China: A revised UTAUT model. Tsinghua. Sci. Technol. 13, 257–264. doi: 10.1016/S1007-0214(08)70042-7
Motamarri, S., Akter, S., Ray, P., and Tseng, C. L. (2014). Distinguishing “mHealth” from other healthcare services in a developing country: A study from the service quality perspective. Commun. Assoc. Inf. Sys. 34, 669–692. doi: 10.17705/1cais.03434
Nadal, C., Sas, C., and Doherty, G. (2020). Technology acceptance in mobile health: Scoping review of definitions, models, and measurement. J. Med. Internet. Res. 22:e17256. doi: 10.2196/17256
Najaftorkaman, M., Ghapanchi, A. H., Talaei-Khoei, A., and Ray, P. (2015). A taxonomy of antecedents to user adoption of health information systems: A synthesis of thirty years of research. J. Assoc. Inf. Sci. Tech. 66, 576–598. doi: 10.1002/asi.23181
Nezamdoust, S., Abdekhoda, M., and Rahmani, A. (2022). Determinant factors in adopting mobile health App in healthcare by nurses. BMC Med. Inform. Decis. 22:47. doi: 10.1186/s12911-022-01784-y
Nisha, N., Iqbal, M., and Rifat, A. (2019). The changing paradigm of health and mobile phones: An innovation in the health care system. J. Glob. Inf. Manag. 27, 19–46. doi: 10.4018/JGIM.2019010102
Nunes, A., Limpo, T., and Castro, S. L. (2019). Acceptance of mobile health applications: Examining key determinants and moderators. Front. Psychol. 10:2791. doi: 10.3389/fpsyg.2019.02791
China Internet Network Information Center [CNNIC] (2021). The 47th china statistical report on internet development, office of the central cyberspace affairs commission. Available online: http://www.cac.gov.cn/2021-02/03/c_1613923423079314.htm (accessed on 31 July 2022).
Okazaki, S., and Mendez, F. (2013). Perceived ubiquity in mobile services. J. Interacy. Mark. 27, 98–111. doi: 10.1016/j.intmar.2012.10.001
Pan, J., Ding, S., Wu, D., Yang, S., and Yang, J. (2019). Exploring behavioural intentions toward smart healthcare services among medical practitioners: A technology transfer perspective. Int. J. Prod. Res. 57, 5801–5820. doi: 10.1080/00207543.2018.1550272
Park, S. Y. (2009). An analysis of the technology acceptance model in understanding university students’ behavioral intention to use E-learning. J. Educ. Technol. Soc. 12, 150–162.
Park, Y. T. (2016). I Emerging new era of mobile health technologies. Healthc. Inform. Res. 22, 253–254. doi: 10.4258/hir.2016.22.4.253
Podsakoff, P. M., MacKenzie, S. B., Lee, J. Y., and Podsakoff, N. P. (2003). Common method biases in behavioral research: A critical review of the literature and recommended remedies. J. Appl. Psychol. 88:879. doi: 10.1037/0021-9010.88.5.879
Qian, C., and Niu, X. (2019). Integrating context-awareness and UTAUT to explain Alipay user adoption. Int. J. Ind. Ergonom. 69, 9–13. doi: 10.1016/j.ergon.2018.09.004
Rajak, M., and Shaw, K. (2021). An extension of technology acceptance model for mHealth user adoption. Technol. Soc. 67:101800. doi: 10.1016/j.techsoc.2021.101800
Schaper, L. K., and Pervan, G. P. (2007). ICT and OTs: A model of information and communication technology acceptance and utilisation by occupational therapists. Int. J. Med. Inform. 76, S212–S221. doi: 10.1016/j.ijmedinf.2006.05.028
Semiz, B. B., and Semiz, T. (2021). Examining consumer use of mobile health applications by the extended UTAUT model. Bus. Manag. Stud. 9, 267–281. doi: 10.15295/bmij.v9i1.1773
Serlachius, A., Schache, K., Kieser, A., Arroll, B., Petrie, K., and Dalbeth, N. (2019). Association Between User Engagement of a Mobile Health App for Gout and Improvements in Self-Care Behaviors: Randomized Controlled Trial. JMIR. Mhealth. Uhealth. 7:e15021. doi: 10.2196/15021
Shiferaw, K. B., and Mehari, E. A. (2019). Modeling predictors of acceptance and use of electronic medical record system in a resource limited setting: Using modified UTAUT model. Inform. Med. Unlocked 17:100182. doi: 10.1016/j.imu.2019.100182
Sneha, S., and Varshney, U. (2009). Enabling ubiquitous patient monitoring: Model, decision protocols, opportunities and challenges. Decis. Support. Syst. 46, 606–619. doi: 10.1016/j.dss.2008.11.014
Sujarwoto, S., Augia, T., Dahlan, H., Sahputri, R. A. M., Holipah, H., and Maharani, A. (2022). COVID-19 Mobile Health applications: An Overview of Mobile applications in Indonesia. Front. Public Health 10:879695. doi: 10.3389/fpubh.2022.879695
Sun, N., and Rau, P. L. P. (2015). The acceptance of personal health devices among patients with chronic conditions. Int. J.Med. Inform. 84, 288–297. doi: 10.1016/j.ijmedinf.2015.01.002
Taylor, S. N., and Todd, P. A. (1995b). Decomposition and cross effects in the theory of planned behavior: a study of consumer adoption intentions. Int. J. Res. Mark. 18, 137–155. doi: 10.1016/0167-8116(94)00019-K
Taylor, S., and Todd, P. (1995a). Assessing IT usage: The role of prior experience. MIS Q. 19, 561–570. doi: 10.2307/249633
Vanneste, D., Vermeulen, B., and Declercq, A. (2013). Healthcare professionals’ acceptance of BelRAI, a web-based system enabling person-centred recording and data sharing across care settings with interRAI instruments: A UTAUT analysis. BMC Med. Inform. Decis. 13:129. doi: 10.1186/1472-6947-13-129
Venkatesh, V., Morris, M. G., Davis, G. B., and Davis, F. D. (2003). User acceptance of information technology: Toward a unified view. MIS Quart. 27, 425–478. doi: 10.2307/30036540
Venkatesh, V., Thong, J. Y., and Xu, X. (2012). Consumer acceptance and use of information technology: Extending the unified theory of acceptance and use of technology. MIS Q. 36, 157–178. doi: 10.2307/41410412
Wang, C., and Qi, H. (2021). Influencing factors of acceptance and use behavior of mobile health APP users: Systematic review. Healthcare 9:357. doi: 10.3390/healthcare9030357
Weimer, A. A., Dowds, S. J. P., Fabricius, W. V., Schwanenflugel, P. J., and Suh, G. W. (2017). Development of constructivist theory of mind from middle childhood to early adulthood and its relation to social cognition and behavior. J. Exp. Child. PsycholL. 154, 28–45. doi: 10.1016/j.jecp.2016.10.002
Whittaker, T. A. (2011). A Beginner’s Guide to Structural Equation Modeling (3rd ed.). Struct. Equ. Modeling. 18, 694–701. doi: 10.1080/10705511.2011.607726
Wiljer, D., Urowitz, S., Apatu, E., DeLenardo, C., Eysenbach, G., Harth, T., et al. (2008). Patient accessible electronic health records: Exploring recommendations for successful implementation strategies. J. Med. Internet. Res. 10:e34. doi: 10.2196/jmir.1061
Wu, I. L., Li, J. Y., and Fu, C. Y. (2011). The adoption of mobile healthcare by hospital’s professionals: An integrative perspective. Decis. Support. Syst. 51, 587–596. doi: 10.1016/j.dss.2011.03.003
Wu, J. H., and Wang, S. C. (2005). What drives mobile commerce?: An empirical evaluation of the revised technology acceptance model. Inf. Manage. 42, 719–729. doi: 10.1287/mnsc.42.1.85
Wu, J. H., Wang, S. C., and Lin, L. M. (2007). Mobile computing acceptance factors in the healthcare industry: A structural equation model. Int. J. Med. Inform. 76, 66–77. doi: 10.1016/j.ijmedinf.2006.06.006
Wu, Q., Tang, P., and Yang, M. (2020). Data processing platform design and algorithm research of wearable sports physiological parameters detection based on medical internet of things. Measurement 165:108172. doi: 10.1016/j.measurement.2020.108172
Zhang, L., and Pentina, I. (2012). Motivations and usage patterns of Weibo. Cyberpsych. Beh. Soc. N. 15, 312–317. doi: 10.1089/cyber.2011.0615
Zhang, X., Han, X., Dang, Y., Meng, F., Guo, X., and Lin, J. (2017). User acceptance of mobile health services from users’ perspectives: The role of self-efficacy and response-efficacy in technology acceptance. Inform. Health. Soc. Ca. 42, 194–206. doi: 10.1080/17538157.2016.1200053
Zhao, S., Cao, Y., Cao, H., Liu, K., Lv, X., Zhang, J., et al. (2022). Chinese Version of the mHealth App Usability Questionnaire: Cross-Cultural Adaptation and Validation. Front. Psychol. 13:813309. doi: 10.3389/fpsyg.2022.813309
Zhou, L., Bao, J., Watzlaf, V., and Parmanto, B. (2019). Barriers to and facilitators of the use of mobile health applications from a security perspective: Mixed-methods study. JMIR. Mhealth. Uhealth. 7:e11223. doi: 10.2196/11223
Zhu, Z. X., and Liu, Y. M. (2016). A Study of Youth Groups’ Behavioral Intention to Transfer Usage from Traditional Medical Channel to Mobile Health. Chin. J. Manage. 13, 1728–1736. doi: 10.3969/j.issn.1672-884x.2016.11.018
Keywords: self-efficacy, privacy concerns, UTAUT model, mHealth services, intention to adopt
Citation: Liu Y, Lu X, Zhao G, Li C and Shi J (2022) Adoption of mobile health services using the unified theory of acceptance and use of technology model: Self-efficacy and privacy concerns. Front. Psychol. 13:944976. doi: 10.3389/fpsyg.2022.944976
Received: 16 May 2022; Accepted: 15 July 2022;
Published: 11 August 2022.
Edited by:
Gheorghe Popescu, Dimitrie Cantemir Christian University, RomaniaCopyright © 2022 Liu, Lu, Zhao, Li and Shi. This is an open-access article distributed under the terms of the Creative Commons Attribution License (CC BY). The use, distribution or reproduction in other forums is permitted, provided the original author(s) and the copyright owner(s) are credited and that the original publication in this journal is cited, in accordance with accepted academic practice. No use, distribution or reproduction is permitted which does not comply with these terms.
*Correspondence: Chengjiang Li, Y2hlbmdqaWFuZy5saUB1dGFzLmVkdS5hdQ==
Disclaimer: All claims expressed in this article are solely those of the authors and do not necessarily represent those of their affiliated organizations, or those of the publisher, the editors and the reviewers. Any product that may be evaluated in this article or claim that may be made by its manufacturer is not guaranteed or endorsed by the publisher.
Research integrity at Frontiers
Learn more about the work of our research integrity team to safeguard the quality of each article we publish.