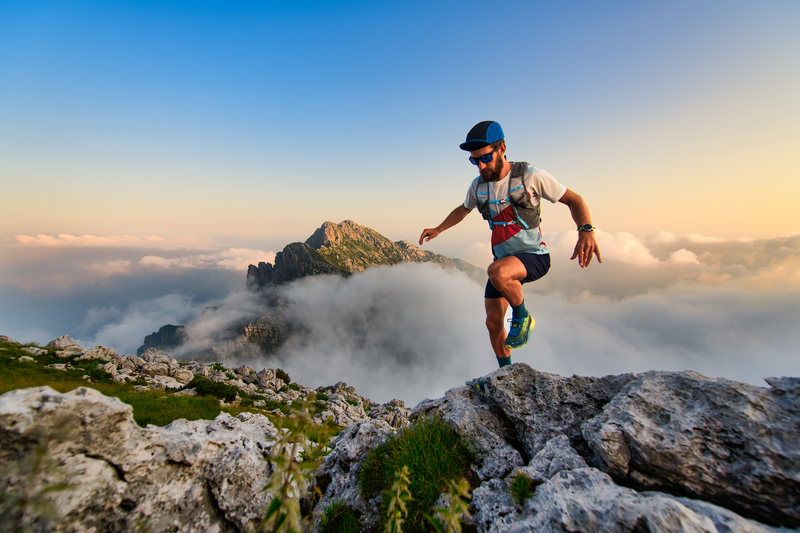
94% of researchers rate our articles as excellent or good
Learn more about the work of our research integrity team to safeguard the quality of each article we publish.
Find out more
ORIGINAL RESEARCH article
Front. Psychol. , 23 November 2022
Sec. Educational Psychology
Volume 13 - 2022 | https://doi.org/10.3389/fpsyg.2022.933974
This article is part of the Research Topic Towards Sustainable Online Learning Systems in Future Era View all 6 articles
Background: Learning digital technologies in higher education is a process of knowledge generation, and the rapid growth of technology in education has a significant impact on students’ learning behaviors, motivation, and knowledge development. Pakistan’s remarkable technological breakthrough has increased in the education field.
Study objectives: The study focuses on estimating students’ learning behaviors, identifying the positive influence of educational apps on digital learning platforms, and analyzing their impact on students’ motivation and knowledge development.
Materials and methods: According to the study’s objectives, a questionnaire survey was conducted to gather the primary data. The participants were students of universities in Lahore city of Pakistan. For this study, the sample size was N = 300, carefully chosen using the purposive sampling technique. Of the respondents, there were 146 male and 154 female students, and the sample consisted of individuals aged 25–35 years. Smart-PLS-Bootstrapping, T-Values (PLS) 3.2.9 and the structural equation model (SEM) were applied to get the appropriate outcomes from the proposed study framework.
Results: SEM analysis results shows that all proposed hypotheses [Animated Movies (AM) –> Student Motivation (SM), Educational Apps (EA) –> Knowledge Development (KD), Learning Behavior (LB) –> Animated Movies, Learning Behavior –> Educational Apps, Learning Behavior –> Knowledge Development, Learning Behavior –> Virtual Classrooms (VCr), Virtual Classrooms –> Knowledge Development, Virtual Classrooms –> Student Motivation] are confirmed while Learning Behavior –> Student Motivation is not confirmed.
Conclusion: This study found that digital learning platforms significantly impact students’ learning and what motivates them to learn. The study also found that using educational apps and virtual classrooms more often helps students learn more and be more motivated to learn.
This study examines the use of digital technology in higher education and the learning process of knowledge creation. Web technology is becoming more critical in many areas of Pakistan, including education. The rapid rise of Web technology in teaching and its effect on how people learn encourages students to use technology in the classroom. Interactive teaching tools may enhance the students’ active learning habits. The polytechnic university of the Philippines surveyed students’ attitudes about online and remote learning, motivation, and learning practices (Avila et al., 2021; Shieh and Hsieh, 2021). Recent studies compare direct lectures, interaction, and self-efficacy as determinants of online motivation and satisfaction in the COVID-19 pandemic scenario (Rahman et al., 2021). The research explores students’ learning behaviors and academic achievement in a comparative examination of certain faculty strata and gender and the impact of technological applications, objectives, and time flexibility on students’ digital learning behaviors (Farid et al., 2014; Zhang, 2021). The researchers looked at what factors influenced student learning online during the COVID-19 pandemic to conclude that all educational institutions, teachers, and students need to embrace technology and hone their digital literacy to keep up with the latest global trends and realities in education (Qiuhan et al., 2020; Younas et al., 2022a).
Web conferencing in education helps both educators and students achieve their critical goals of creating the ideal learning environment for kids and balancing life and teaching tasks for educators. Following the definition of online learning, an overview of recent pedagogical strategies has been used in online learning environments (Hartnett, 2016). It is important to note that students’ self-efficacy in computer and online learning, perceived utility, and simplicity of use are essential success criteria in online learning environments (Barclay et al., 2018). The critical challenges for current information technology integrated education are creating educational activities for digital learning and deploying technology tools flexibly. Higher education institutions may use web conferencing to improve instructors’ online access and create new teaching and learning opportunities (Lin and Chen, 2017; Adipat, 2021). The research evaluates the usefulness of e-learning technologies in teaching public institutions in the United Arab Emirates, focusing on course administration and interaction with the students’ information systems (Sandybayev, 2020). Passey and Higgins (2011), Sousa and Rocha (2018) Studies focus on digital learning and motivation among higher education students using an open education platform. These studies focus on current insights on learning outcomes resulting from the usage of learning platforms in schools by learners, students, and instructors. Younas et al. (2022b) indicate how homeschooled English language learners feel about different homeschooling platforms and apps, formulate learning principles, and analyze the broader implications of real-world learning. Vorwerk and Engenhart-Cabillic (2022), Long et al. (2021) discuss how students felt about a newly established digital instructional program, which included an interactive e-book and short learning videos on a YouTube channel. It looks at the connection between how people learn and how well they do in a course, and the flaws in the blended learning mode, which is a big help in improving the quality of education. Finally, the essential components of excellent education are digital platforms for learning and their influence on student motivation and knowledge growth. Digital platforms for learning have unique properties that assist the long-term development of education. The present study explores the learning behaviors and how digital learning impacts students’ motivation and study circles among confident objectives: (1) to estimate the learning behavior of students; (2) to identify the positive influence of educational apps on digital learning platforms; and (3) to analyze the impact of digital learning on students’ motivation and knowledge development.
This study aimed to determine the attractiveness of digital learning platforms and their impact on learning behaviors and knowledge development. The study illustrates that using interactive components of e-learning boosts the motivation of undergraduate students for the learning process (Abou El-Seoud et al., 2014). The research examines students’ self-efficacy, attitudes, confidence in utilizing technology, instructional tactics, the capacity to monitor and assess educational results, and student motivations (Hongsuchon et al., 2022). Game-based learning outcomes were examined, including academic success, problem-solving, critical thinking, and student attitudes and behaviors (Yu et al., 2021). The study looks at the self-regulated learning (SRL) techniques that students use in massive open online courses, emphasizing how learners’ reasons for participating impact their behavior and use of SRL tactics (Littlejohn et al., 2016). According to the research, the social regulation-based online learning strategy improves students’ learning outcomes and motivation (Hwang et al., 2021). As a result, the study indicated that widespread usage of educational apps and virtual classrooms provides better performance in motivating students and knowledge development. The proposed relationships of study variables have been shown in Figure 1, and the hypotheses are given below.
Lai et al. (2016) examined an online training platform that offered pedagogical rationales for self-directed learning and a strategic foundation for aligning technology choices with learning objectives and processes. According to Faridah et al. (2020), the objective of the research is to quantify students’ interest in and engagement with digital learning and investigate how students’ interest in and engagement with digital learning changed throughout the COVID-19 epidemic. Singh et al. (2021) attempt to explain the epochal correlations between the constructs and examine how information might be utilized to increase student acceptance of digital collaboration platforms. Aduba and Mayowa-Adebara’s (2022) study examines how internet platforms were used during the lockdown and (Luo et al., 2019) interpersonal connections have a good influence on the learners’ experience, strengthening their desire to utilize the e-learning platform. Cui et al. (2022) highlight the benefits of combining autonomy with structural assistance and the importance of instructors in a web-based inquiry learning environment. Kang and Zhang (2020) suggest that online education is becoming more popular globally because it engages and motivates students. Panigrahi et al. (2018) reported that technology is being used around the globe to improve education, minimizing the time and space issues associated with conventional learning. The survey results of Moroccan EFL university students are presented on the influence of digital technology on learning behavior and reading motivation. Most students use digital resources for learning and entertainment (Larhmaid et al., 2019).
Learning motivation is critical for success in online learning, particularly for K-12 students, and the impacts of K-12 students’ perceived presence and technical acceptability in online learning (Zuo et al., 2021). Abou El-Seoud et al. (2014) research shows that interactive e-learning features increase undergraduate students’ interest in learning. Sandybayev (2020) examined how digital technology may help culturally diverse students succeed in school by interviewing 46 students from different academic programs. Flipped teaching may encourage underachieving students to study acting and improve their learning efficacy and psychological needs through self-determination theory. (Chou et al., 2021; Chiu, 2022) studies examined student engagement in online learning and its effectson students learning outcomes. The study aims to determine if there is a strong correlation between e-learning and student motivation in higher education and the increasing use of e-learning (Harandi, 2015). It is more important than ever to figure out how to create electronic learning resources that cater to student motivation and make learning easier (Song and Bonk, 2016), and the study’s goal was to see how the design of a virtual learning environment affects adult learners’ motivation at work (Bashshar, 2017).
Recent studies show that the quick shift from traditional to digital learning has harmed students’ intrinsic and extrinsic motivation (Gustiani, 2020). Education-related departments strive to positively cultivate students’ professional information knowledge and skills (Sun and Pan, 2021). Rippa and Secundo’s (2019) work adds to the burgeoning notion of digital academic entrepreneurship, and Díaz-Noguera et al.’s (2022) study gives a development model of students’ adaptive capabilities to the digital revolution in university education. The study finds the essential abilities and information from studies that reinforce each other and help people learn more deeply (Makani et al., 2016). This research examined how tourism and hospitality students see how sharing economic platforms help them learn and develop their beliefs and attitudes (Horng et al., 2022). According to the findings, which looked at students’ motivation for online learning, most students said they were engaged in class and had strong time management skills while taking online courses (Cabansag et al., 2020).
Social cognitive theory (SCT) stresses learning from the social environment (Schunk and Usher, 2012). A key feature of SCT is its emphasis on external and internal social reinforcement. In the social climate, SCT stresses the unique way humans learn and maintain behavior. These earlier experiences build attitudes and expectancies, which decide whether or not a person engages in a particular activity. People engage in diverse vicarious, symbolic, and self-regulatory processes to build a feeling of agency. In addition to objectives and self-evaluations of achievement, key motivators include values, peer comparisons, and self-efficacy. People establish and track objectives. Progress perception boosts self-efficacy and motivation. People operate according to their principles and seek the desired results. Other people’s learning and goal achievement might be compared. Self-efficacy influences task choices, effort, perseverance, and accomplishment. Depending on their social surroundings, humans might be proactive, engaged, or estranged (Ryan and Deci, 2000). To this end, social–contextual elements that encourage or impede self-motivation and healthy psychological development have been studied. Specific variables, such as enhancing or reducing intrinsic motivation, self-regulation, and wellbeing were studied.
H1: Learning behavior (LB) is positively associated with watching animated movies (AMs).
H2: Student’s motivation (SM) is positively influenced by AM and LB.
H3: LB is positively impacted SM through virtual classroom (VCr).
H4: LB positively influences the use of educational apps (EAs).
H5: Knowledge development (KD) is positively influenced by VCr and LB.
H6: KD is positively influenced by the use of EAs and LB.
The present study has been conducted in Lahore, the capital of Punjab Province in Pakistan and is exploratory. The online survey was used to gather primary data according to the research goals. The research goal was explained to all survey participants and their agreement was obtained. The researchers did a quality check while the data were being gathered. All the people who participated in the study were entirely voluntary, and they were told their information would only be used for research purposes.
The sample size for this research was N = 300, which was chosen using purposive sampling. The criteria mentioned were students with four majors (such as social sciences, psychology, management studies, and education) who participated in this study and had different educational backgrounds. Finally, 75 students were selected from each primary subject, and participants of different genders and age groups participated in this study (Liébana-Presa et al., 2020). The information gathered during data collection was divided into categories depending on the frequency and percentages of each question in demographics, and Table 1 summarizes the findings. There were 146 male and 154 female students among the responders. Eighty-three respondents were under 25 years, 129 were between the ages of 25 and 30 years, and 88 were between the ages of 31 and 35 years. Similarly, for the question, 29 respondents belonged with a doctoral degree, 107 were from master’s degree, 95 were from bachelor’s degree, and 69 respondents had an associate degree. Similarly, students with four different significant studies (such as social sciences, psychology, management studies, and education) participated in this study, and 75 male and female students were chosen from each major equally to get unbiased data (please see Table 1).
The survey’s approach was employed to gather data, and a questionnaire on learning behavior and the influence of students’ motivation and knowledge growth was created. It was built on a five-point Likert scale, with one indicating strong agreement and five indicating extreme disagreement, and the scale was modified based on previous studies (DeVries et al., 2018). Dependent on the availability of participants, questionnaires were used to gather data, and the data for this research were gathered at Pakistani universities. Respondents filled out these surveys, and the demographic employed in this research included university students attending courses on learning practices, digital platforms, and their effects on knowledge acquisition. A survey was performed to gather primary data according to the research goals. The research goal was explained to all survey participants and their agreement was obtained. The researchers did a quality check while the data were being gathered. The survey participants’ anonymity and the confidentiality of the received information were guaranteed. While getting informed consent, it was clear that all data would only be used for research.
The questionnaires included six variables to gather data, and 23 items were included in the questionnaires. The study’s conceptual framework contained one independent variable (such as learning behavior), three mediators (namely animated movies, virtual classrooms, and educational Apps), and two dependent variables (such as student motivation and knowledge development). Some previous studies contain one independent variable, three or four moderators, and one or two dependent variables (Lin and Chen, 2017; DeVries et al., 2018).
Considering the previous research (Salkind, 2010), the study contains gender, age, qualifications, and study fields as demographic factors. All self-reported variables were categorized by gender (male or female), age (between 20 and 35 years), qualification (namely doctoral, master, associate, and bachelor), and study major (such as social sciences, psychology, management studies, and education).
Learner motivation and learning outcomes are better in digital education than in traditional education. Motivating oneself to learn has a significant favorable impact on one’s learning ability. To determine the elements impacting students’ happiness and performance in online courses and their interaction (Gopal et al., 2021). The digital learning impact on students was assessed by using the Likert-scale questions about AM, VCRs, and EAs.
Student motivation plays a pivotal role in knowledge development during learning with technological aspects. Developing student motivation is a challenging but vital part of teaching that instructors must address. This research aimed to see how technology affects students’ drive to learn and remember new material (Granito and Chernobilsky, 2012; Yarborough and Fedesco, 2020). The survey participants were assessed through motivation and knowledge development questions.
Smart PLS-Bootstrapping, T-Values (PLS) 3.0 and structural equation modeling (SEM) were used to examine the model, including internal consistency reliability, convergent validity, and discriminant validity as examples of indicator loadings (Hair et al., 2019). With the help of SEM, the Smart-PLS study strategy is a robust, scalable, and cutting-edge approach to creating a substantial statistical model (Abbas et al., 2019a). PLS-SEM looks at complicated models with both observable and latent parts. It may be able to give SEM results with different levels of structural complexity, such as higher-order structures that often solve problems with multicollinearity and look into the measurement and structural models (Ringle et al., 2015; Sharif et al., 2021).
The Internal consistency reliability (ICR) was implemented to assess the consistency of findings across indicators. The present technique reported Cronbach’s alpha and composite reliability (CR). ICR values should range from 0 to 1. Cronbach’s alpha and Cronbach’s coefficient of determination (CR) should be more than 0.700. Cronbach’s alpha and Cronbach’s CR reports are shown in Table 2. All constructs have a good Cronbach’s alpha, and their CR values meet or exceed what is needed.
Animated movies had a Cronbach’s alpha of 0.726 and a CR of 0.846, while educational apps had an alpha of 0.767 and a CR of 0.734. Knowledge development possessed an alpha of 0.774 and a CR of 0.869. Learning behavior had an Alpha of 0.714 and a CR of 0.755. Students’ motivation possessed an alpha of 0.869 and a CR of 0.906. Finally, virtual classrooms obtained an alpha of 0.791 and a CR of 0.864.
The prediction skills of the structural model were tested as part of the evaluation. However, the collinearity value should be indicated before providing the structural model by reporting the variance inflation factor (VIF) values. Notably, the predictors/mediators were assessed for the collinearity of animated movies, educational apps, and virtual classrooms as mediators of learning behavior, student motivation, and knowledge development, respectively (Hair et al., 2019). VIF levels should be less than three; values greater than three are generally associated with multicollinearity issues. According to the data analysis, all VIFs are less than three. As a result, collinearity is not a concern in this study’s model.
Convergent validity is a subtopic of construct validity in which tests with the same or comparable constructs should be substantially connected. The average variance derived from this research is used to calculate the convergent validity average variance extracted (AVE). The AVE was calculated using Smart PLS 3.0. According to the methodology, AVE values should be 0.500 or higher, explaining 50% or more of the variation (Table 2). All constructs had AVE values of more than 0.500, indicating that they presented more than half of the variation. Animated movies’ AVE value was 0.650, educational apps’ AVE value was 0.580, knowledge development’s learning behavior was 0.587, student motivation’s AVE value was 0.659, and virtual classrooms were 0.621.
Figure 2 shows the factor loadings acquired by PLS-SEM to confirm the validity. The loadings of reflective indicators attained in SEM should be more than 0.500, and all loadings should be greater than 0.500 based on the calculation. “Animated Movies (0.898, 0.855, 0.644), Educational Apps (0.723, 0.607, 0.742), Knowledge Development (0.799, 0.845, 0.846), Learning Behavior (0.533, 0.562, 0.536, 0.803, 0.635), Students Motivation (0.752, 0.872, 0.739, 0.806, 0.876), and Virtual Classrooms (0.861, 0.849, 0.854, 0.541).”
Discriminant validity (DV) showed how to quantify constructs that were conceptually unrelated to one another. Discriminant validation seeks to show any discriminating evidence based on all components’ dissimilarities (Campbell and Fiske, 1959). The overlap of measurements on each other is used to assess discriminant validity (please see Table 3). Comparing the square root of a factor’s AVE values with the correlation between constructs might indicate DV. AVE values should be greater than correlations (Campbell and Fiske, 1959). Table 2 shows that the AVE square root is more significant than correlation values, which indicates good assessment.
This work’s model fitness was assessed using Standardized-root-mean-square-residual (SRMR), normed fit index (NFI), and Chi-square (X2). It is a measure of model fitness that compares observed covariance to hypothesized matrices (Chen, 2007; Brown, 2015). The SRMR value must be less than or equal to 0.08 to be considered acceptable. Results show that the predicted SRMR value of 0.079 is a satisfactory model fit for the standardized root mean square residual. An NFI score of 0.475 and a X2 value of 2868.490 (please see Table 4) indicate that the two datasets are statistically insignificant.
The import of all straight effects was assessed for the structural model by examining the path coefficients, T-statistics, and p-value. We computed the data through a bootstrapping procedure. The bootstrapping computation results are presented in Table and Figure, with the Table informing the hypotheses, relationship, path, T-value, and p-value. Figure 3 illustrates the T-value and loading value of the path lines during the bootstrapping process.
The hypotheses’ statistical significance was assessed using a standard beta calculation. We can see how much the dependent component may vary from the independent factor using the beta number. Each association’s standardized beta (β) value was determined following the predicted study model (Table 5). High and significant beta (β) values indicate that endogenous latent variables have a strong influence. T-statistics were utilized to validate the significance of the beta value for each route in the experiment. The significance level of putative associations was assessed and evaluated using the beta (β) value acquired using the bootstrapping approach. The structural model’s hypothesized connections are shown in all beta (β) values in Figure 3, Table 5, respectively, to illustrate the link between T-values and observed variables (see Figure 3).
The maximum T-value was attained by the path between facilities and research activities (t = 15.771), while the last value was the association between practical activities and employability (t = 0.537). All hypotheses projected in this study were supported. In detail, H1 was reported to be significant in influencing students’ motivation (β = 0.716; t = 13.092; p < 0.001) and knowledge development. H2 reveals that educational apps have a significant impact (β = 0.292; t = 4.696; p < 0.001) on knowledge development. H3 was also supported where learning behavior is significantly predicted by animated movies (β = 0.630; t = 15.771; p < 0.001). Similarly, the significant role of learning behavior in educational apps (H4) was also reported (β = 0.463; t = 8.221; p < 0.001). Learning behavior is also a significant predictor for knowledge development, H5 (β = 0.280; t = 6.415; p < 0.001). The result of PLS-SEM supports H6 because there is a direct effect of learning behavior on student motivation (β = 0.017; t = 0.537; p < 0.592). H7 is also supported as learning behavior is significantly predicted by virtual classrooms (β = 0.543; t = 12.206; p < 0.001). Finally, the findings also support hypotheses 8 and 9. Positive relationships also emerged between virtual classrooms and knowledge development (β = 0.396; t = 5.751; p < 0.001). Virtual classrooms are also known to be a significant predictor of students’ motivation (β = 0.204; t = 3.551; p < 0.001).
It was determined that e-learning was successful and investigated major antecedents of e-learning success in the COVID-19 pandemic (Elshaer and Sobaih, 2022; Jaoua et al., 2022). The major motivators for the likely continuance of videoconferencing as a supplement to face-to-face tutorials at Spanish institutions (Infante-Moro et al., 2021). Sequential analysis is used to assess the impact of student motivation on online reading behavior and the perceived online social presence in an online course (Tao, 2009; Sun et al., 2018). According to the result of the SEM model, these hypotheses (H1: Animated Movies –> Student Motivation, β = 0.716; t = 13.092; p < 0.001; H2: Educational Apps –> Knowledge Development, β = 0.292; t = 4.696; p < 0.001; H3: Learning Behavior –> Animated Movies, β = 0.630; t = 15.771; p < 0.001; H4: Learning Behavior –> Educational Apps, β = 0.463; t = 8.221; p < 0.001; H5: Learning Behavior –> Knowledge Development, β = 0.280; t = 6.415; p < 0.001) are confirmed. The result of H6 indicates that (Learning Behavior –> Student Motivation, β = 0.017; t = 0.537; p < 0.592) there is no direct effect of learning behavior on student motivation. The approach suggests three characteristics to enhance students’ ability to adapt to digital change in university teaching: motives, digital pedagogy, and student autonomy (Hwang et al., 2015; Díaz-Noguera et al., 2022). Since information and computer technology (ICT) skills are becoming more important everywhere, especially in the workplace, one of the main goals of colleges has been to teach students how to deal with problems (Bond et al., 2018; Muhammad et al., 2020). Previous research examines the effect of enhancing overall wellness during the COVID-19 epidemic and university students’ dangerous online activity (Peng et al., 2022).
In education, digital transformation is a long-term process that has become a pressing issue. Studies indicate a positive association between openness to experience and creativity and a mediation function for intrinsic drive and creative process participation in this relationship (Tan et al., 2019; Bogdandy et al., 2020). The study analyzed teacher opinions on how technology affects student academic behavior and performance in a blended learning environment and also looked at students’ behavioral intentions (motivation) for adopting online learning technologies (Chen et al., 2002; McHone, 2020). The analysis results show that these hypotheses (H7: Learning Behavior –> Virtual Classrooms, β = 0.543; t = 12.206; p < 0.001: H8: Virtual Classrooms –> Knowledge Development, β = 0.396; t = 5.751; p < 0.001; H9: Virtual Classrooms –> Student Motivation, β = 0.204; t = 3.551; p < 0.001) are confirmed. To construct an efficient 21st century classroom that fits the requirements of students, a modern teacher must consider a student’s drive to study and the effect of technology has on inclusionary education (Granito and Chernobilsky, 2012; Kizilcec and Schneider, 2015). There has never been a study that looked specifically at how university students’ learning attitudes are affected by social media’s good and bad features (Abbas et al., 2019b).
Online education is rapidly developing due to a growing demand for higher and continuing education. Yet, many online students fail to fulfill their educational objectives due to the lack of face-to-face interaction (Zheng et al., 2015; Kizilcec et al., 2020). Learning behavior is also a significant predictor of knowledge development in H5 (β = 0.280; t = 6.415; p = 0.000). H6 results show that there is a direct effect of learning behavior on student motivation (β = 0.017; t = 0.537; p = 0.592). Students’ willingness to attend online courses was predicted using the Motivation Orientation Scale and the Unified Theory of User Acceptance of Technology (Cullum, 2016). The capacity of students to persevere and get high marks when taking online classes is compared with their ability to do so while taking face-to-face courses (Xu and Jaggars, 2013). Investigations were carried out to determine how strong the link between online learning and students’ motivation was among the participants and whether there was a direct correlation between student views of their motivation to read and their accomplishments in a blended learning environment (Minda, 2020; Miller, 2021). Researchers wanted to determine how e-learning activities interacted with such characteristics as gender, maturity level, field of study, geographic location, and grade level (Thomas, 2020). As the world becomes more digital, it is important to define information literacy and investigate studies on game-based learning (Press, 2017). This research found that digital platforms significantly influence learning behavior and affect students’ motivation and knowledge growth. Learner motivation and learning outcomes are greater in digital education than in traditional education.
With the growing usage of digital platforms for learning, the introduction of various technology applications, and their influence on learning behavior, student motivation, and knowledge acquisition, the whole domain of learning and education in Pakistan and throughout the globe has altered. This study also examines students’ studying habits in light of digital learning’s impact on education. Intentions for digital learning are more vivid in students who participate in digital learning. Motivating oneself to study has a substantial favorable effect on one’s learning ability. Furthermore, goal-setting behavior and social pressures have increased students’ digital learning practices. These results have been a significant step forward in creating knowledge and improving student learning. The findings of this study have a wide range of ramifications for future academics and organizations that want to replicate this research in various places with their resources. New research paths like these may benefit significantly from the use of these.
There are several limitations regarding the current study. The respondent must be 20 + years old university student. There are a lot of digital platforms for academic learning. Some of them are freely available and some of them are paid or subscription-based. Most universities have paid or subscription-based access to their students’ digital platforms of academic learning. Only those university students can participate in the study survey that uses their universities’ available digital platforms. Purposive sampling was used as part of the non-probability sampling (NPS) technique since it best suited the study’s goals and objectives. The conclusions of this research cannot be extended to the whole population since it is difficult to repeat the results of the purposeful sample.
The raw data supporting the conclusions of this article will be made available by the authors, without undue reservation.
The studies involving human participants were reviewed and approved by School of Education, Soochow University. The patients/participants provided their written informed consent to participate in this study.
XQ is the principal investigator. UN and MY collected/analyzed data and wrote the manuscript. RM designed the study model and hypothesis and contributed to the discussion section. HS contributed to drafting the literature review, discussion, and overall editing of the manuscript. All authors contributed to the article and approved the submitted version.
This project research was supported by study on the Construction Quality of Research Groups in Universities in China, the National Office for Education Sciences Planning China. Project No: BIA200166.
We thank all the researchers for data collection and processing and acknowledge our study’s survey participants.
The authors declare that the research was conducted in the absence of any commercial or financial relationships that could be construed as a potential conflict of interest.
All claims expressed in this article are solely those of the authors and do not necessarily represent those of their affiliated organizations, or those of the publisher, the editors and the reviewers. Any product that may be evaluated in this article, or claim that may be made by its manufacturer, is not guaranteed or endorsed by the publisher.
Abbas, J., Aman, J., Nurunnabi, M., and Bano, S. (2019a). The impact of social media on learning behavior for sustainable education: Evidence of students from selected universities in Pakistan. Sustainability 11:1683. doi: 10.3390/su11061683
Abbas, J., Mahmood, S., Ali, H., Ali, R. M., Ali, G., Aman, J., et al. (2019b). The effects of corporate social responsibility practices and environmental factors through a moderating role of social media marketing on sustainable performance of business firms. Sustainability. 11:3434. doi: 10.3390/su11123434
Abou El-Seoud, M. S., Taj-Eddin, I. A. T. F., Seddiek, N., El-Khouly, M. M., and Nosseir, A. (2014). E-learning and students’ motivation: A research study on the effect of e-learning on higher education. Int. J. Emerg. Technol. Learn. 9, 20–26. doi: 10.3991/ijet.v9i4.3465
Adipat, S. (2021). Why web-conferencing matters: Rescuing education in the time of COVID-19 pandemic crisis. Front. Psychol. 6:752522. doi: 10.3389/feduc.2021.752522
Aduba, D. E., and Mayowa-Adebara, O. (2022). Online platforms used for teaching and learning during the COVID-19 era: The case of lis students in delta state university. Abraka. Int. Inf. Libr. Rev. 54, 17–31. doi: 10.1080/10572317.2020.1869903
Avila, E. C., Abin, G. J., Bien, G. A., Acasamoso, D. M., and Arenque, D. D. (2021). Students’ perception on online and distance learning and their motivation and learning strategies in using educational technologies during COVID-19 pandemic. Paper Presented J. Physics 1933:012130. doi: 10.1088/1742-6596/1933/1/012130
Barclay, C., Donalds, C., and Osei-Bryson, K.-M. (2018). Investigating critical success factors in online learning environments in higher education systems in the Caribbean. Inf. Technol. Dev. 24, 582–611. doi: 10.1080/02681102.2018.1476831
Bashshar, C. E. (2017). Virtual Learning Environments’ Impact on Adult Learners’ Motivation in the Workplace, Ph.D thesis. Minneapolis, MN.
Bogdandy, B., Tamas, J., and Toth, Z. (2020). “Digital transformation in education during covid-19: A case study,” in Paper Presented at the 2020 11th IEEE International Conference on Cognitive Infocommunications (CogInfoCom). (Piscataway, NJ) doi: 10.1109/CogInfoCom50765.2020.9237840
Bond, M., Marín, V. I., Dolch, C., Bedenlier, S., and Zawacki-Richter, O. (2018). Digital transformation in German higher education: Student and teacher perceptions and usage of digital media. Int. J. Educ. Technol. High. Educ. 15:48. doi: 10.1186/s41239-018-0130-1
Brown, T. A. (2015). Confirmatory Factor Analysis for Applied Research. New York, NY: Guilford publications.
Cabansag, P., Cabansag, V., and Soriano, R. (2020). students’ motivation toward online learning: Basis for policy making. ASTR Res. J. 4:1.
Campbell, D. T., and Fiske, D. W. (1959). Convergent and discriminant validation by the multitrait-multimethod matrix. Psychol. Bull. 56:81. doi: 10.1037/h0046016
Chen, F. F. (2007). Sensitivity of goodness of fit indexes to lack of measurement invariance. Struct. Equ. Modeling 14, 464–504. doi: 10.1080/10705510701301834
Chen, Y., Lou, H., and Luo, W. (2002). Distance learning technology adoption: A motivation perspective. J. Comput. Inf. Syst. 42, 38–43.
Chiu, T. K. (2022). Applying the self-determination theory (SDT) to explain student engagement in online learning during the COVID-19 pandemic. J. Res. Technol. Educ. 54:S14–S30. doi: 10.1080/15391523.2021.1891998
Chou, C.-P., Chen, K.-W., and Hung, C.-J. (2021). A study on flipped learning concerning learning motivation and learning attitude in language learning. Front. Psychol. 12:753463. doi: 10.3389/fpsyg.2021.753463
Cui, Y., Zhao, G., and Zhang, D. (2022). Improving students’ inquiry learning in web-based environments by providing structure: Does the teacher matter or platform matter? Br. J. Educ. Technol. 53, 1049–1068. doi: 10.1111/bjet.13184
Cullum, A. W. (2016). Student Motivation and Intent to Take Online Courses. Ph.D thesis. Statesboro, GA: Georgia Southern University.
DeVries, J. M., Rathmann, K., and Gebhardt, M. (2018). How does social behavior relate to both grades and achievement scores? Front. Psychol. 9:857. doi: 10.3389/fpsyg.2018.00857
Díaz-Noguera, M. D., Hervás-Gómez, C., De la Calle-Cabrera, A. M., and López-Meneses, E. (2022). Autonomy, motivation, and digital pedagogy are key factors in the perceptions of spanish higher-education students toward online learning during the COVID-19 pandemic. Int. J. Environ. Res. Public Health 19:654. doi: 10.3390/ijerph19020654
Elshaer, I. A., and Sobaih, A. E. E. (2022). Flower: An approach for enhancing e-learning experience amid COVID-19. Int. J. Environ. Res. Public Health 19:3823. doi: 10.3390/ijerph19073823
Farid, S., Luqman, M., Tariq, S., and Ahmad Warraich, I. (2014). An analysis of learning behavior and academic achievement: A case study of bahauddin zakariya university multan. Pakistan J. Soc. Sci. 34, 193–204.
Faridah, I., Sari, F. R., Wahyuningsih, T., Oganda, F. P., and Rahardja, U. (2020). “Effect Digital Learning on Student Motivation during Covid-19,” in Paper Presented at the 2020 8th International Conference on Cyber and IT Service Management (CITSM), (Pangkal: IEEE). doi: 10.1109/CITSM50537.2020.9268843
Gopal, R., Singh, V., and Aggarwal, A. (2021). Impact of online classes on the satisfaction and performance of students during the pandemic period of COVID 19. Educ. Inf. Technol. 26, 6923–6947. doi: 10.1007/s10639-021-10523-1
Granito, M., and Chernobilsky, E. (2012). “The effect of technology on a student’s motivation and knowledge retention,” in NERA Conference Proceedings. (White Plains, NY).
Gustiani, S. (2020). Students’motivation in online learning during covid-19 pandemic era: A case study. Holistics 12, 23–40. doi: 10.1145/3442355.3433688
Hair, J. F., Risher, J. J., Sarstedt, M., and Ringle, C. M. (2019). When to use and how to report the results of PLS-SEM. Eur. Bus. Rev. 31, 2–24. doi: 10.1108/EBR-11-2018-0203
Harandi, S. R. (2015). Effects of e-learning on students’ motivation. Procedia Soc. Behav. Sci. 181, 423–430. doi: 10.1016/j.sbspro.2015.04.905
Hartnett, M. (2016). “The importance of motivation in online learning,” in Motivation in Online Education, (Singapore: Springer), 5–32. doi: 10.1007/978-981-10-0700-2_2
Hongsuchon, T., Emary, I. M. M. E., Hariguna, T., and Qhal, E. M. (2022). Assessing the impact of online-learning effectiveness and benefits in knowledge management, the antecedent of online-learning strategies and motivations: An empirical study. Sustainability 14:2570. doi: 10.3390/su14052570
Horng, J.-S., Liu, C.-H., Chou, S.-F., Yu, T.-Y., Fang, Y.-P., and Huang, Y.-C. (2022). Student’s perceptions of sharing platforms and digital learning for sustainable behaviour and value changes. J. Hosp. Leis. Sport Tour. Educ. 31:100380. doi: 10.1016/j.jhlste.2022.100380
Hwang, G.-J., Lai, C.-L., and Wang, S.-Y. (2015). Seamless flipped learning: A mobile technology-enhanced flipped classroom with effective learning strategies. J. Comput. Educ. 2, 449–473. doi: 10.1007/s40692-015-0043-0
Hwang, G.-J., Wang, S.-Y., and Lai, C.-L. (2021). Effects of a social regulation-based online learning framework on students’ learning achievements and behaviors in mathematics. Comput. Educ. 160:104031. doi: 10.1016/j.compedu.2020.104031
Infante-Moro, A., Infante-Moro, J. C., Gallardo-Pérez, J., and Luque-de la Rosa, A. (2021). Motivational factors in the use of videoconferences to carry out tutorials in spanish universities in the post-pandemic period. Int. J. Environ. Res. Public Health 18:10474. doi: 10.3390/ijerph181910474
Jaoua, F., Almurad, H. M., Elshaer, I. A., and Mohamed, E. S. (2022). E-learning success model in the context of COVID-19 pandemic in higher educational institutions. Int. J. Environ. Res. Public Health 19:2865. doi: 10.3390/ijerph19052865
Kang, X., and Zhang, W. (2020). An experimental case study on forum-based online teaching to improve student’s engagement and motivation in higher education. Int. Learn. Environ. 28, 1–12. doi: 10.1080/10494820.2020.1817758
Kizilcec, R. F., and Schneider, E. (2015). Motivation as a lens to understand online learners: Toward data-driven design with the OLEI scale. ACM Trans. Comput. Hum. Interact. 22, 1–24. doi: 10.1145/2699735
Kizilcec, R. F., Reich, J., Yeomans, M., Dann, C., Brunskill, E., Lopez, G., et al. (2020). Scaling up behavioral science interventions in online education. Psychol. Cogn. Sci. 117, 14900–14905. doi: 10.1073/pnas.1921417117
Lai, C., Shum, M., and Tian, Y. (2016). Enhancing learners’ self-directed use of technology for language learning: The effectiveness of an online training platform. Comput. Assist. Lang. Learn. 29, 40–60. doi: 10.1080/09588221.2014.889714
Larhmaid, M., Nour, T., and Afflerbach, P. (2019). Assessing the effects of digital technologies on learning behavior and reading motivation among moroccan efl university students. Int. J. Digit. Lit. Digit. Competence 10, 1–24. doi: 10.4018/IJDLDC.2019100101
Liébana-Presa, C., Martínez-Fernández, M. C., Benítez-Andrades, J. A., Fernández-Martínez, E., Marqués-Sánchez, P., and García-Rodríguez, I. (2020). Stress, emotional intelligence and the intention to use cannabis in spanish adolescents: Influence of COVID-19 confinement. Front. Psychol. 11:582578. doi: 10.3389/fpsyg.2020.582578
Lin, M.-H., and Chen, H.-G. (2017). A study of the effects of digital learning on learning motivation and learning outcome. Eurasia J. Math. Sci. Technol. Educ. 13, 3553–3564. doi: 10.12973/eurasia.2017.00744a
Littlejohn, A., Hood, N., Milligan, C., and Mustain, P. (2016). Learning in MOOCs: Motivations and self-regulated learning in MOOCs. Internet High. Educ. 29, 40–48. doi: 10.1016/j.iheduc.2015.12.003
Long, Z., Mu, X., Song, C., and Tian, D. (2021). “Research on the Learning Behavior of Students in Blended Learning Mode,” in Paper Presented at the 2021 IEEE 6th International Conference on Big Data Analytics (ICBDA), (Xiamen: IEEE). doi: 10.1109/ICBDA51983.2021.9403027
Luo, N., Zhang, Y., and Zhang, M. (2019). Retaining learners by establishing harmonious relationships in e-learning environment. Interact. Learn. Environ. 27, 118–131. doi: 10.1080/10494820.2018.1506811
Makani, J., Durier-Copp, M., Kiceniuk, D., and Blandford, A. (2016). Strengthening deeper learning through virtual teams in e-learning: A synthesis of determinants and best practices. Int. J. E-Learn. Distance Educ. 31, 1–16
McHone, C. (2020). Blended Learning Integration: Student Motivation and Autonomy in a Blended Learning Environment, Ph.D Thesis. Johnson City, TN: East Tennessee State University.
Miller, J. Y. (2021). Digital Literacy: The Impact of a Blended Learning Model on Student Motivation and Achievement. Boiling Springs, NC: Gardner-Webb University.
Minda, S. (2020). “Online-Learning and Students’ Motivation: A Research Study on the Effect of Online Learning on students’ motivation in IAIN Padangsidimpuan,” in Paper Presented at the International Online Conference on English and Education. (Padang Sidempuan).
Muhammad, Y., Noor, U., Khalid, S., and Imran, M. (2020). The effective role of call in teaching english at postgraduate level: A case study of university of the Punjab, Pakistan. Dilemas Contemporáneos Educación Política Valores 7, 1–17. doi: 10.46377/dilemas.v32i1.1974
Panigrahi, R., Srivastava, P. R., and Sharma, D. (2018). Online learning: Adoption, continuance, and learning outcome—A review of literature. Int. J. Inf. Manag. 43, 1–14. doi: 10.1016/j.ijinfomgt.2018.05.005
Passey, D., and Higgins, S. (2011). Learning platforms and learning outcomes–insights from research. Learn. Media Technol. 36, 329–333. doi: 10.1080/17439884.2011.626783
Peng, X., Menhas, R., Dai, J., and Younas, M. (2022). The COVID-19 pandemic and overall well-being: Mediating role of virtual reality fitness for physical-psychological health and physical activity. Psychol. Res. Behav. Manag. 15, 1741–1756. doi: 10.2147/PRBM.S369020
Press (2017). Digital and Information Literacy in Higher Education through Game-based learning. Cambridge, MA: Academic Press.
Qiuhan, L., Afzaal, M., Alaudan, R., and Younas, M. (2020). COVID 19 pandemic and online education in Hong Kong: An exploratory study. Int. J. Emerg. Technol. 11, 411–418.
Rahman, M. H. A., Uddin, M. S., and Dey, A. (2021). Investigating the mediating role of online learning motivation in the COVID-19 pandemic situation in Bangladesh. J. Comput. Assist. Learn. 37, 1513–1527. doi: 10.1111/jcal.12535
Ringle, C. M., Da Silva, D., and Bido, D. D. S. (2015). Structural equation modeling with the SmartPLS. Brazilian J. Mark. 13, 56–73. doi: 10.5585/remark.v13i2.2717
Rippa, P., and Secundo, G. (2019). Digital academic entrepreneurship: The potential of digital technologies on academic entrepreneurship. Technol. Forecast. Soc. Change 146, 900–911. doi: 10.1016/j.techfore.2018.07.013
Ryan, R. M., and Deci, E. L. (2000). Self-determination theory and the facilitation of intrinsic motivation, social development, and well-being. Am. Psychol. 55:68. doi: 10.1037/0003-066X.55.1.68
Salkind, N. J. (2010). Encyclopedia of Research Design. Thousand Oaks, CA: SAGE Publications, doi: 10.4135/9781412961288
Sandybayev, A. (2020). The impact of e-learning technologies on student’s motivation: Student centered interaction in business education. Int. J. Res. Tour. Hosp. 6, 16–24. doi: 10.20431/2455-0043.0601002
Schunk, D. H., and Usher, E. L. (2012). Social cognitive theory and motivation. Oxford Handbook Hum. Motivat. 2, 11–26. doi: 10.1093/oxfordhb/9780190666453.013.2
Sharif, S. P., Naghavi, N., Ong, F. S., Nia, H. S., and Waheed, H. (2021). Health insurance satisfaction, financial burden, locus of control and quality of life of cancer patients: A moderated mediation model. Int. J. Soc. Econ. 48, 513–530. doi: 10.1108/IJSE-10-2019-0629
Shieh, M.-D., and Hsieh, H.-Y. (2021). Study of influence of different models of e-learning content product design on students’ learning motivation and effectiveness. Front. Psychol. 12:753458. doi: 10.3389/fpsyg.2021.753458
Singh, A., Sharma, S., and Paliwal, M. (2021). Adoption intention and effectiveness of digital collaboration platforms for online learning: The Indian students’ perspective. Int. Technol. Smart Educ. 18, 493–514. doi: 10.1108/ITSE-05-2020-0070
Song, D., and Bonk, C. J. (2016). Motivational factors in self-directed informal learning from online learning resources. Cogent Educ. 3:1205838. doi: 10.1080/2331186X.2016.1205838
Sousa, M., and Rocha, A. (2018). “Digital learning in an open education platform for higher education students**,” in Proceedings of the EDULEARN18 Conference, Palma, Mallorca, Spain (Palma: IATED). doi: 10.21125/edulearn.2018.2770
Sun, J. C.-Y., Lin, C.-T., and Chou, C. (2018). Applying learning analytics to explore the effects of motivation on online students’ reading behavioral patterns. Int. Rev. Res. Open Distrib. Learn. 19, 209–227. doi: 10.19173/irrodl.v19i2.2853
Sun, L., and Pan, C. E. (2021). Effects of the application of information technology to e-book learning on learning motivation and effectiveness. Front. Psychol. 12:752303. doi: 10.3389/fpsyg.2021.752303
Tan, C.-S., Lau, X.-S., Kung, Y.-T., and Kailsan, R. A. L. (2019). Openness to experience enhances creativity: The mediating role of intrinsic motivation and the creative process engagement. J. Creat. Bahav. 53, 109–119. doi: 10.1002/jocb.170
Tao, Y. (2009). The Relationship Between Motivation and Online Social Presence in an Online Class. Orlando, FL: University of Central Florida.
Thomas, D. (2020). Thailand university students’ e-learning behavior during the global pandemic. human behavior. Dev. Soc. 21, 57–65.
Vorwerk, H., and Engenhart-Cabillic, R. (2022). Students’ learning behavior in digital education for radiation oncology. Strahlenther. Onkol. 198, 12–24. doi: 10.1007/s00066-021-01858-2
Xu, D., and Jaggars, S. (2013). Adaptability to online learning: Differences across types of students and academic subject areas. CCRC Working Paper No. 54. New York, NY: Community College Research Center
Yarborough, C. B., and Fedesco, H. (2020). Motivating Students. Nashville: Vanderbilt University Center for Teaching.
Younas, M., Khalid, S., and Noor, U. (2022a). Applied pedagogies for higher education: Real-world learning and innovation across the curriculum. Soc. Sci. J. 1, 1–3. doi: 10.1080/03623319.2022.2084688
Younas, M., Noor, U., Zhou, X., Menhas, R., and Qingyu, X. (2022b). COVID-19, students satisfaction about e-learning and academic achievement: Mediating analysis of online influencing factors. Front. Psychol. 13:948061. doi: 10.3389/fpsyg.2022.948061
Yu, Z., Gao, M., and Wang, L. (2021). The effect of educational games on learning outcomes, student motivation, engagement and satisfaction. J. Educ. Comput. Res. 59, 522–546. doi: 10.1177/0735633120969214
Zhang, P. (2021). Understanding digital learning behaviors: Moderating roles of goal setting behavior and social pressure in large-scale open online courses. Front. Psychol. 12:783610. doi: 10.3389/fpsyg.2021.783610
Zheng, S., Rosson, M. B., Shih, P. C., and Carroll, J. M. (2015). “Understanding Student Motivation, Behaviors and Perceptions in MOOCs,” in Paper Presented at the Proceedings of the 18th ACM Conference on Computer Supported Cooperative Work & Social Computing, (Vancouver, BC), doi: 10.1145/2675133.2675217
Keywords: learning behavior, digital platforms, technological applications, student motivation, knowledge development
Citation: Noor U, Younas M, Saleh Aldayel H, Menhas R and Qingyu X (2022) Learning behavior, digital platforms for learning and its impact on university student’s motivations and knowledge development. Front. Psychol. 13:933974. doi: 10.3389/fpsyg.2022.933974
Received: 24 May 2022; Accepted: 27 October 2022;
Published: 23 November 2022.
Edited by:
Manzoor Ahmed Hashmani, University of Technology Petronas, MalaysiaReviewed by:
Muhammad Afzaal, Shanghai International Studies University, ChinaCopyright © 2022 Noor, Younas, Saleh Aldayel, Menhas and Qingyu. This is an open-access article distributed under the terms of the Creative Commons Attribution License (CC BY). The use, distribution or reproduction in other forums is permitted, provided the original author(s) and the copyright owner(s) are credited and that the original publication in this journal is cited, in accordance with accepted academic practice. No use, distribution or reproduction is permitted which does not comply with these terms.
*Correspondence: Xu Qingyu, cXl4dUBzdWRhLmVkdS5jbg==
Disclaimer: All claims expressed in this article are solely those of the authors and do not necessarily represent those of their affiliated organizations, or those of the publisher, the editors and the reviewers. Any product that may be evaluated in this article or claim that may be made by its manufacturer is not guaranteed or endorsed by the publisher.
Research integrity at Frontiers
Learn more about the work of our research integrity team to safeguard the quality of each article we publish.