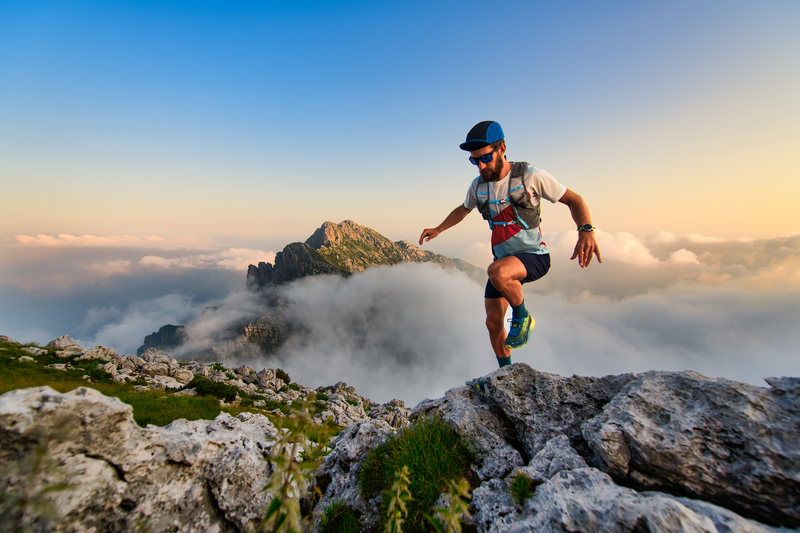
94% of researchers rate our articles as excellent or good
Learn more about the work of our research integrity team to safeguard the quality of each article we publish.
Find out more
ORIGINAL RESEARCH article
Front. Psychol. , 25 April 2022
Sec. Organizational Psychology
Volume 13 - 2022 | https://doi.org/10.3389/fpsyg.2022.874851
This article is part of the Research Topic The Psychological Facets of Consumer-Brand Relationship in The Digital World View all 16 articles
Along with the increasing trend of transactions occurring on social media, the consumption of Chinese cultural and creative products has increased even against the background of the COVID-19 pandemic. In this context, this article aims to analyze the relationships between virtual community-based social identity and cultural and creative product customer engagement (CE) behaviors. To this end, social identity theory and CE behavior theory were applied to previous research model. Structural equation modeling (SEM) was conducted using data from 520 self-administered questionnaires from online virtual community members. The results show that social identity has a significant effect on customer knowledge behavior, participation behavior, and influencer behavior. Moreover, influencer behavior mediates the effect of social identity on purchase intention. The study also identified gender differences in the mediation for influencer behaviors. Our results suggest that women are more sensitive to influencer behaviors than men, and thus generate more purchase behaviors.
According to the Chinese National Bureau of Statistics (2021), the business revenue of enterprises in China’s cultural and related industries increased by 2.2% in 2020 compared to the previous year. The museum industry grew rapidly in China between 2011 and 2021 (Chinese National Bureau of Statistics, 2021). The last 2 years have presented many obstacles to museum visits due to the COVID-19 pandemic. However, the statistics mentioned above show that the museum industry still maintained a relatively high growth rate during the pandemic. A typical example of these successes is one of the cultural products developed by Henan Museum in 2020. This was in the form of a blind box with characteristics resembling those of museum artifacts, and by digging themselves, customers could find and open the blind box to reveal scaled-down versions of the artifacts, allowing them to experience the fun of digging for treasure. However, in 2020, people’s enthusiasm for visiting the exhibition remained relatively low due to the social isolation measures brought about by the pandemic. So how did this museum creation generate such a buzz under such difficult circumstances? Through our investigation, we found that the popularity of the Henan Museum’s product was due to enthusiasts sharing posts about it on social media, which triggered even more attention. The search term #henanmuseumarchaeologyblindbox was viewed more than 28 million times on Weibo—the largest social media platform in China—and discussed more than 12,000 times in less than a month.
Inspired by this phenomenon, we assumed that interest-based online communities must also contribute to brand growth in a subtle way; we wanted to find out how the sharing behavior of these enthusiasts on social media, which caused other enthusiasts to purchase the product, actually occurred. How can brands leverage interest-based communities to help their brands grow?
Interest-based communities are communication platforms created spontaneously and autonomously by certain users, while other users search and join these interest-based platforms on their own initiative. There are many communities of fans of cultural and creative products on Chinese Internet platforms, such as the “We All Love to Buy Cultural and Creative Products” group on Douban where a group of people who are interested in museum cultural products gather. These users actively exchange and share their purchase experiences and their knowledge about certain products, and also disseminate knowledge and perceptions of new products rapidly in these communities. In the community, the topics discussed inevitably include the attributes of certain products, which means that every discussion about cultural and creative products is, for a brand, an act of consumer participation in the brand. At this level, community members are co-creators of brand value. Through online interaction, people can get the information they need and buy the products they are interested in without having to leave home. As for minor culture, virtual communities help promote brand familiarity. Interest-based members are able to form social relationship nets more easily (Zhao et al., 2009), which also provides potential opportunities for increasing customer engagement (CE) behaviors. CE through social media generates positive impressions of the brand (Osei-Frimpong and McLean, 2018).
Previous studies have focused on the impact on consumer behavior of brand communities created by firms for the purpose of binary interactions with consumers (Zaglia, 2013; Elbedweihy et al., 2016). He et al. (2012) argued that identification with the company on the part of consumers causes engagement behaviors. Researchers have also explored various theories to explain community member behaviors and to examine the relationship between community identity and community participation behavior in online Internet communities. The engagement behaviors in these interest-based virtual communities imply differences in the social psychologies of their users. Social identity is considered to be a motivation to participate in online communities. Bagozzi and Dholakia (2002) study adopted a marketing viewpoint to identify how social influence and variable social identity impact virtual community participation. The focus of the present study is on how social identity influences engagement behaviors with brands among members of user-created interest-based communities. This is valuable for the study of brand communities, because the spontaneity of user-created community members differs from the passivity of members of brand communities: in user-created interest-based communities members’ behavior is autonomous rather than passive. Another difference between user-created communities and brand communities is that the content exchanged by members of user-created communities may include experiences of a large number of different brands, rather than a single brand. It is also valuable for brands to gain insight into the psychology of consumers and trends in content production within the community from the perspective of consumer self-motivation, as a complement to the brand-consumer relationship, and this can provide a consumer perspective on the direction of product marketing.
This study will examine the relationship between community identity and the within-community behavior of members of a Chinese Internet community which is based on interest in cultural and creative products. To determine how social influences impact users’ engagement, our model uses social identity theory framing. Once the role of social identity in user-created communities is established, subsequent studies can continue to further explore the motivation of social identity.
Social identity has been defined by Tajfel (1978) as “that part of an individual’s self-concept which derives from his knowledge of his membership of a group (or groups) together with the value and emotional significance attached to the membership.” Tajfel (1978) research on categorization and the development of accentuation theory highlighted the cognitive consequences of (social) categorization processes, an important component of social identity theory and later of self-categorization theory. He proposed that classifying physical stimuli could lead people to perceive between-category differences as larger (Tajfel and Wilkes, 1963). Tajfel (1978) defined social identity (or group identity), in both cognitive and evaluative terms, as that part of the self-concept corresponding to the knowledge of group membership, together with the value and emotional significance of that membership. This has proven to further influence different fields such as organizational behavior (Ashforth and Mael, 1989) and fandom behavior study (He, 2005). This paper argues that social identity theory can be used to study the influence of individual psychological characteristics on the behavior of community members who share similar passions for cultural and creative products.
Tajfel’s definition of social identity involved cognitive, affective, and evaluative components (Bergami and Bagozzi, 2000). In a cognitive sense, social identity is a self-categorization process whereby the individual forms a self-awareness of virtual community membership, which includes awareness of both similarities with other members and dissimilarities with non-members (Ashforth and Mael, 1989). In this case, the members of the community share a similar passion for cultural and creative products. Tajfel (1978) definition also implies that a virtual community has an emotional and evaluative impact on its members. In the emotional dimension, social identity represents a sense of emotional investment in the group, which researchers have defined as attachment or affective commitment (Bagozzi and Dholakia, 2002). Affective social identity strengthens members’ bonds with each other and fosters their engagement behaviors in the group (Ellemers et al., 1999; Bergami and Bagozzi, 2000). Aside from affective social identity, evaluative components also play a crucial role in explaining users’ coherence in a group (Bergami and Bagozzi, 2000). The evaluative component represents group self-esteem and is defined as the evaluation of self-worth based on community “belongingness” (Ellemers et al., 1999). Members of the cultural and creative community, the subject of this paper, develop their contributions to the community based on their perceptions of cultural and creative preferences. The emotion of investment also leads them to identify with, and emotionally link themselves to, the community.
Despite the discrepancies among these three dimensions, they have not been fully studied in the current literature; instead, social identity has generally been measured and treated as a unidimensional construct (Ellemers et al., 1999). In this study, we propose that each dimension exerts a positive effect on CE behaviors, and that the cognitive, affective, and evaluative dimensions are first-order construct components of a second-order social identity construct.
The concept of CE emerged in 2006 as a sub-set of the term “engagement” related to the study of customers’ behaviors and emotions in terms of their interaction/participation with brands (Vivek et al., 2014). According to the literature, most CE-related studies have viewed CE from a behavioral perspective, considering that engagement behaviors—especially non-transactional behaviors—can affect a company’s development (Verhoef et al., 2010). Non-transactional behaviors indirectly produce profits for a firm (Kahn, 1990). Furthermore, CE behavior has been examined beyond purchase behavior; this research was driven by the understanding that a consumer’s motivation comes from the emotional bond between them and a company (Van Doorn et al., 2010). Prior literature has considered non-transactional behaviors to be comprised of various related behaviors such as word-of-mouth (WOM) information transmission, referrals, co-creations, and ratings (Van Doorn et al., 2010; Wei et al., 2013). In the online community, users’ spontaneous exchanges of opinions and sharing of knowledge about a product represent the emotional ties between users and the product, which are related to CE behaviors. At the same time, the extensive discussions about products and brands generated by customers’ engagement in the community stimulate the interest of other members within the community in these brands. As a result, when consumers instinctively identify with a brand, positive feedback is generated accordingly.
The creation of value by customers for firms occurs through a more elaborate mechanism than purchase alone (Kumar et al., 2010). In their study of customer engagement value (CEV), Kumar et al. (2010) proposed four components of CEV: customer life value, customer referral value, customer influencer value, and customer knowledge value. These four dimensions of CEV can be derived from four dimensions of customer behavior: (1) customer purchase behavior, (2) customer referral behavior, (3) customer influencer behavior, and (4) customer knowledge behavior. The four dimensions of customer behavior proposed by Kumar et al. (2010) are based on the combination of the concept of purchase behavior with the three other defined behaviors. Customer referral behavior is extrinsically motivated by, and related to, the acquisition of new customers. Almost all behavior that would be classified as contributing to consumer influencer behavior is based on the intrinsic motivation of the customer. Customer influencer behavior is mostly represented by WOM activity, such that a customer voluntarily generating WOM about the firm and its products is engaging in consumer influencer behavior. Customer knowledge behavior includes feedback behaviors, such as providing feedback to a community or firm. In the process of developing knowledge, customers assist a company by adding value (Joshi and Sharma, 2004).
Based on this CEV theory, we split CE behavior into four similar dimensions—in this case, four outcomes of social identity. Customer influencer behavior is the perceived behavior of community members as influenced by others. Customer participation behavior is an indicator of how active the community is for individual users. Knowledge behavior is the interaction and information sharing that occurs between customers about a product.
Zhou and Lu (2009) have proposed a model to explain the correlation between social identity, community members’ sharing intentions, and their actual behavior. This model specifically considers members’ knowledge-sharing behavior. We extracted part of this model and added other customer behaviors as outcomes to adapt the model to our research, using the social identity theory (Tajfel, 1978) and consumer engagement behavior theory as underlying frameworks (Figure 1).
The correlation between social identity and CE has been widely studied. Social factors have been commonly used as mediators or antecedents in various CE behavior studies (Shen, 2012; Sharma and Crossler, 2014; De Oliveira et al., 2016). A previous study has also verified the importance of social presence to the extent that it has been found to impact customers’ sociable interactions in the social commerce (s-commerce) environment (Xiang et al., 2014). Other studies have examined the mediating effect of social factors on the behavior of online customers participating in s-commerce activities (Hajli et al., 2015; Kucharska, 2019). Prior research on the overall effect of these three dimensions on social identity has generally supported positive associations with CE behaviors, such as participation behaviors. As a minor cultural group, cultural and creative product consumers, and the degree to which their behavior is affected by their group identity, represent the starting point for this study. Prior literature about social media’s role for firms has been more focused on researching brands’ social media pages. Past studies have revealed how social interaction motives influence CE (de Silva, 2019). In particular, in an online community context, the ways in which customers’ identification with their community might increase CE behavior have also been studied (Prentice et al., 2019). This study explores how interest-based virtual communities are also able to generate a positive impact on customers’ engagement behaviors. Moreover, with the knowledge that existing consumer behavior studies have focused more on specific behaviors, such as participation behavior (Dholakia et al., 2004) or knowledge behavior (Shi, 2010), this study examines four components of CE behavior to more generally identify the influence of social identity.
Past studies have supported the position that searching for information is the main form of gratification that consumers seek in online brand communities (Zaglia, 2013). When customers identify with companies, they are more likely to develop a psychological attachment to them and to voluntarily contribute to the company’s goals (Bhattacharya and Sen, 2003). Posting and sharing information is one of the ways in which community members can actively develop a mental attachment to a company. Based on the above discussion, we have developed the following hypotheses:
Hypothesis 1: Social identity positively influences knowledge behavior in cultural and creative product virtual communities.
Most studies use social motivations to explain active participation in virtual communities (Tsai and Bagozzi, 2014; Chih et al., 2017; Hsu et al., 2018). Social identity is one of the social motivations for virtual community participation (Bagozzi and Dholakia, 2002; Hsu et al., 2018), which develops through in-group ties prompting people to interact with other members (Wolter and Cronin, <suffix>Jr.</suffix>, 2017). Researchers believe that a user’s social identification with social media means that the user has a sense of belonging to that social media community and will willingly continue to engage with the social media platform (Yang et al., 2021). Members who are connected to the community are more likely to dedicate more time and energy to engaging in community activities (Choi, 2013). We assumed that social identity played a similar role in members’ participation behavior in the user-created interest community. On the basis of the this discussion, we developed Hypothesis 2:
Hypothesis 2: Social identity positively influences participation behavior in cultural and creative product virtual communities.
Prior research on social identity has revealed that identification with a group produces positive outcomes, in such a way that people who strongly identify themselves with organizations are more likely to support these organizations in various ways and to evaluate these organizations positively (Ahearne et al., 2005). Consumers who identify strongly with a company are more likely to actively engage in additional behaviors, such as passing on positive WOM information about the company and influencing other customers (Castro et al., 2022). On the basis of this discussion, we developed Hypothesis 3:
Hypothesis 3: Social identity positively influences influencer behavior in cultural and creative product virtual communities.
Formerly, individuals tended to rely on social relationships and interactions with peers when exhibiting purchasing behaviors (Vivek et al., 2014). In modern market development conditions, many products are purchased by customers not because of their physical characteristics, but because the customers belong to a particular social group (Ivanova et al., 2022). In the context of online communities, social relationships are generated through the interaction of members in the community. On the basis of this discussion, we developed Hypothesis 4:
Hypothesis 4: Social identity positively influences purchase behavior in cultural and creative product virtual communities.
Customer influencer behavior can influence customers’ perceptions. This study views WOM in communities as the perceived behavior of community members having an influence on others, which represents influencer behavior in this paper. In many product categories, WOM, interaction, and assistance from other customers post-acquisition can significantly affect people’s behavior through increased persuasion, the conversion of people into customers and the recipient customer’s continued usage of a product (Katz and Shapiro, 1985). Several researchers have studied the role of individuals’ influence on others in the diffusion and adoption of products (Van den Bulte and Wuyts, 2007). It is widely acknowledged that WOM can significantly influence a firm’s sales and consumer behavioral intentions (Tseng and Hsu, 2010). The worldwide spread of the Internet has engendered a less personal but more ubiquitous form of WOM communication: online WOM (e-WOM) communication (Jalilvand and Samiei, 2012). The influencer behavior represented by WOM that is explored in this paper is e-WOM behavior in the community. This new type of WOM communication has become an important venue for consumer opinions (Chih et al., 2013) and is assumed to be even more effective than in-person WOM communication due to its greater accessibility and reach (Chatterjee, 2001). Reza Jalilvand et al. (2012) argued that e-WOM is one of the most effective factors influencing brand purchase intention in consumer markets. Investigations, have found that e-WOM information impacts purchase intention. Thus, we have defined connections between the constructs of influencer behavior and purchase intention, and have generated Hypothesis 5:
Hypothesis 5: Influencer behavior mediates the influence of social identity on purchase intention in cultural and creative product virtual communities.
Data were collected through an online self-reported survey supported by Sojump, a questionnaire website that is widely used in mainland China. The study used convenience sampling, which is a type of non-probability sampling. Because the research scope was limited to online communities, we choose to distribute our questionnaire in online virtual communities. In addition, because this study examines cultural and creative product users, the virtual communities in which we chose to distribute the questionnaire were related to cultural and creative products, where the users’ interests were consistent with the nature of the communities. The questionnaires were distributed in virtual communities based in the Chinese mainland (e.g., Douban groups, Weibo communities, and WeChat groups). This questionnaire was developed on the basis of evidence from prior literature on social identity theory and CE. Of the questionnaires distributed, 661 were returned. After removing invalid questionnaires, we obtained 520 samples, yielding a 78.7% response rate from the participants.
According to Chinese investigative reports on Chinese cultural and creative products in 2019 (PR World, 2019), more than 1 billion online consumers of cultural and creative products were born during the period 1990–1999, which is consistent with our data. The generation born during this decade is known as Generation Z. Unlike previous generations, Generation Z are Internet natives, who are more reliant on digital media and more active within online communities. They are also savvy consumers who do not trust brands and prefer personalized services (Gutfreund, 2016). They trust information shared with their peers within spontaneously engaged online communities more than information that they receive through direct advertising. This is in line with the demographic profile of respondents as belonging to Generation Z. Furthermore, our gender data is also in line with the aforementioned investigative reports. The demographic information of the participants is presented in Table 1.
The scale of measurement for the constructs included in the research model was defined on the basis of the measures used in the sources, as shown in Table 2.
The research model employed in this study examines the effects of cognitive, affective, and evaluative identification on CE behaviors. The questionnaire was composed of two sections. In the first section, participants indicated their agreement or disagreement with 24 items using a 7-point Likert scale that ranged from “strongly disagree” (1) to “strongly agree” (7). The advantage of using an interval scale is that it permits the researchers to use a variety of statistical techniques that can be applied to nominal and ordinal scale data, in addition to the arithmetic mean, standard deviation, product-moment correlations, and other statistics commonly used in marketing research (Malhotra and Galletta, 1999).
The 24 items were grouped into the seven constructs considered in the model. According to Ellemers et al. (1999), social identity has been commonly measured and treated as a unidimensional construct. Therefore, social identity was set as a second-order construct, as mentioned above. Cognitive, affective, and evaluative social identities were used as first-order constructs and were measured by three items each, a format adapted from Bagozzi and Dholakia (2002). The cognitive dimension of social identity refers to a person’s self-categorization as a group member (Dholakia et al., 2004). Affective identification refers to the emotional attachment of a person to the group (Ellemers et al., 1999). The evaluative aspect of social identity refers to the assessment of self-worth that results from group membership (Dholakia et al., 2004).
Three aspects of social identity were both measured with items adapted from Bagozzi and Dholakia (2002) and Dholakia et al. (2004). We refer to Wang’s (2017) adaptation of the measurement items of cognitive, affective, and evaluative identification. Engagement behavior outcomes, including knowledge behavior, influencer behavior, participation behavior, and purchase intention, were operationalized by four items, six items, two items, and four items, respectively. Knowledge behavior measurement items are referenced in the Shi’s study on ability of knowledge contribution (Shi, 2010). We chose e-WOM behavior to represent influencer behavior, following the definition of Kumar et al. (2010), and measured by the items adapted from Jalilvand and Samiei’s (2012) research. We replaced referral behavior with participation behavior, which measured with items in Wang’s (2017) research on usage of social networking site (Wang, 2017). Purchase intention measurement items are adapted from Jalilvand and Samiei’s (2012) research. All measurement items and their sources are listed in Table 2.
The quantitative data analysis included two processes: (1) an analysis of the descriptive statistics and the reliability of the measurement using SPSS 26.0 and Amos 24.0 software, and (2) structural equation modeling (SEM) testing. Amos 24.0 was used to evaluate the correlation coefficient between variables in the factor analysis and path analysis of the equation model. A detailed explanation of the application of both analytical procedures and their results is given below.
To test the validity and reliability of the measurement, confirmatory factor analysis (CFA) was conducted using AMOS 24.0. The results indicate an acceptable fit with the data (χ2 = 557.836, df = 163, normed χ2 = 3.422). The goodness of fit index (GFI = 0.899) and comparative fit index (CFI = 0.950) were also acceptable, and the standardized root mean square residual (SRMR = 0.0480) and root mean square error of approximation (RMSEA = 0.068) were less than 0.8 (HooperD and Mullen, 2008). All factor loadings were statistically significant at p < 0.001. The average variance extracted (AVE) for the seven constructs ranged from 0.662 to 0.826, exceeding 0.5 (Fornell and Larcker, 1981), and the composite reliability (CR) for the constructs was higher than 0.8 (Hair et al., 2009), indicating satisfactory convergent validity.
Confirmatory factor analysis revealed a standard factor loading of pab2, one of the measurements of participation behavior construct, below 0.6 (Hair et al., 2009). Thus, this measurement item was removed. To analyze one item, according to Schumacker and Lomax (2004), the CR of pab3 was set as 0.8 to modify the model, which required the use of SPSS 26.0 to calculate the covariance of item pab3 (2.734) and include it in our model. The other construct, Cronbach’s alpha, exceeded the marginal value of 0.7 (Hair et al., 2009), indicating satisfactory internal consistency for all constructs, as presented in Table 3.
To test discriminant validity, the values of AVE and the squared correlation of constructs were compared for each pair of constructs. All squared correlations were acceptable; thus, the discriminant validity of the constructs was satisfactory (Table 4).
According to Marsh and Hocevar (1985), by calculating the target coefficient, this study compared CFA of the first order and second order to decide the fitness with the data. At-value closer to 1 implies that the second-order CFA can replace the first-order CFA, making the model more precise. In this case, the target coefficient was used to test for the existence of a higher-order social identity construct. A target coefficient of 1 provided satisfactory evidence of a second-order social identity construct. The fitness index of the second-order CFA of social identity implies that the fitness was good.
Structural equation modeling was conducted using AMOS 24.0 to test the hypotheses. To test the model, social identity as a second-order construct was used to test the model. As shown in Figure 2, the SEM analysis results showed that social identity had a significant influence on consumer knowledge behavior (β = 0.91, p < 0.001), consumer participation behavior (β = 0.64, p < 0.001), consumer influencer behavior (β = 0.63, p < 0.001), and consumer purchase intention (β = 0.40, p < 0.001). This result confirms that Hypotheses 1–4 were supported (Table 5).
Figure 2. Results of the structural equation model (N = 520). cogid, cognitive social identity; affid, affective social identity; evaid, evaluative social identity; socialid, social identity; kbh, knowledge behavior; ibh, influencer behavior; pabh, participation behavior; pbh, purchase intention.
To test Hypothesis 5, which explored whether influencer behavior has a mediating effect on the relationship between social identity and purchase behavior, we conducted bias-corrected percentile bootstrapping and percentile bootstrapping at a 95% confidence interval with 1,000 bootstrap samples (Taylor et al., 2008). We also calculated the lower and upper bounds of the confidence interval to test for indirect effects. As presented in Table 6, the results for the test of indirect effects from social identity to purchase intentions, where it can be seen that effects exist (indirect effect Z = 7.72 > 1.96) (Sobel, 1982). The results confirm that Hypothesis 5 was supported.
According to the Chinese investigation reports of Chinese cultural and creative products in 2019 (PR World, 2019), women had stronger intentions to purchase the products. Based on this report, we aimed to examine whether differences existed between our model’s samples of men and women. Thus, we conducted a multi-sample analysis. Multi-sample analysis with unconstrained model showed the model fit the data well [χ2 (326) = 819.22, p < 0.05, NFI = 0.90, NNFI = 0.93, CFI = 0.94, RMSEA = 0.05]. Then, to test the invariance of the factor loadings across gender, we constrained factor loadings to be equal across two groups. Data showed constrained model fit well [χ2 (339) = 838.82, p < 0.05, NFI = 0.90, NNFI = 0.93, CFI = 0.94, RMSEA = 0.05]. Also, the χ2 test between unconstrained and constrained model was not significant [χ2 (13) = 19.607, p = 0.11 > 0.05], showing that factor loadings of both gender groups were invariant (Cheung and Rensvold, 1999). Due to participation behavior is measured by on item, according to Schumacker and Lomax (2004), the CR of pab3 was set as 0.8 to modify the model. Therefore, we measured the gender factor separately after. The results of the SEM analysis of the different genders are displayed in Figures 3, 4.
Figure 3. Results of the structural equation model with the female sample. χ2 = 487.649, df = 163, normed χ2 = 2.992, goodness of fit index (GFI) = 0.860, AGFI = 0.819, comparative fit index (CFI) = 0.936, standardized root mean square residual (SRMR) = 0.058, root mean square error of approximation (RMSEA) = 0.08.
Figure 4. Results of the structural equation model with the male sample. χ2 = 331.564, df = 163, normed χ2 = 2.034, goodness of fit index (GFI) = 0.864, AGFI = 0.825, comparative fit index (CFI) = 0.941, standardized root mean square residual (SRMR) = 0.0476, root mean square error of approximation (RMSEA) = 0.07.
According to Figures 3, 4, Hypotheses 1–4 are still supported. However, in the model with the male sample, influencer behavior doesn’t mediate the effect of social identity on purchase intention (indirect effect Z = 1.122 < 1.96, p = 0.266) (Sobel, 1982), as shown in Table 7.
In this study, we explore the direct impact of social identity generated from user-created interested community on CE to parse out the differences in effects between communities with different attributes, providing a novel perspective on the relationship of community scope to CE for future researchers to build upon and insights on the self-driven proactive psychology of consumers that managers can use to improve brand value. In addition, we explore the different effects of gender, offering a novel influencing factor between the relation of social identity and CE behaviors for the research model. The discrepancy in the results implies that gender as a variable requires further study.
We aimed to apply social identity theory to the formation of an explanation of CE behaviors in virtual communities. The nature of cultural and creative products is to some degree uneconomic, according to Chinese common sense. Thus, it seems paradoxical that community members are willing to engage in contributing their knowledge and suggestions to such a community voluntarily. Social identity theory, from the perspective of individual-based psychology, offers insights into this practice. By going beyond Zhou and Lu (2009) model, we developed a further step for cultural and creative consumer behaviors which includes both transactional and non-transactional aspects and the relationship between them. Unlike previous cultural and creative product studies which have focused on product features, this research explored the significance of personal traits. Knowledge behavior consists of various knowledge interactions, including sharing feedback and suggestions in virtual communities. These spontaneous actions tend to be unprofitable for consumers and require self-motivation, which results from social identity in the community. In this process, cognitive, affective, and evaluative identities have almost equal impacts on social identity, as this research verifies. Interaction frequency in the cultural and creative online community, which is a measurement of participation behavior, represents the degree to which someone wants to invest time in that community even though they have multiple choices in the virtual world. The study broadens perspectives on cultural and creative products by incorporating social identity theory and CE behavior, not only considering personal traits as antecedents but also examining the interconnections between non-transactional behavior and purchasing behavior. Previous literature in the social network context has been more centered on brand fan pages (Kucharska, 2017; Chen and Tsai, 2020) rather than virtual communities. The target groups of this study were selected from several social network communities in the Chinese mainland. The concept of social identity was developed based on these virtual communities, further expanding the context of social networks.
The participants in our research came from both interest-based virtual communities (e.g., Douban and Weibo) and small-group-based virtual communities (WeChat). The interest-based community was comprised of people who shared the same interests in cultural and creative products and who shared content about their purchasing experiences. Long-term investment in the operation of brand pages while ignoring the value of virtual communities may no longer be a good strategy. The role of social identity in virtual communities must be taken into account by firms, and it is possible to do this by making only slight strategic changes. Firms can actively foster customer relationships in communities by posting content and interacting with consumers, allowing the firms to indirectly increase transactions and reduce transactional costs. The more that firms connect with influencers, the more WOM information transmission they can achieve. A previous study has proven that influencer behaviors play crucial roles in purchase behavior as well as in community activity (Wielki, 2020). Therefore, firms benefit from maintaining healthy relationships with influential supporters. A favorable community atmosphere is the result of the maintenance of a group, which provides a spirit of connection between people and enhances members’ affective, cognitive, and evaluative experiences. Some studies have examined whether companies should strategically cultivate environments that encourage customers to assist other customers (Verhoef et al., 2010). In this case, a firm may need to balance its responsibilities as both organizer and participant. In addition, people like to contribute content and information in a positive community atmosphere, and therefore positive feedback is repeatedly activated. It is easy to understand that a positive atmosphere leads to customer recognition. Taking cultural and creative firms as an example, the more that attractive content is shared with in-group members, the more the members are likely to reply and share similar items in the community. At the same time, companies can use enthusiastic users as a tool to gain new customers and increase sales. Community value is reflected in the recognition of members on both cognitive and evaluative levels, which is based on rational judgment. On an affective level, people’s need to belong can be met in a virtual community by engaging with others who have similar values. Firms and communities are not mutually exclusive, as the people that firms serve have double identities as consumers and community members. To achieve the common goal of creating people’s social identity to stimulate CE behavior, it is crucial to consider how consumers think.
The effect of social identity on customer behavior can be explained by the standardized regression coefficient. After noticing that female customers are more prone to influencing purchasing behaviors, we split our sample into two groups to examine whether gender affects the relationships between model constructs and to explore how social identity affects female and male customers’ behaviors separately. This analysis provided us with some interesting insights. Social identity is positively and significantly related to knowledge behavior for both male and female consumers. We found a positive impact of social identity on influencer behavior that was significantly stronger for men (β = 0.84, p < 0.001) than for women (β = 0.57, p < 0.001). Conversely, the effect of social identity on participation behavior was slightly greater for women (β = 0.67, p < 0.001) than for men (β = 0.51, p < 0.001). In addition, influencer behavior had a significant mediating effect on social identity and purchase behavior only in the female model. Not surprisingly, these results support prior studies. However, our study went a step further, to find that gender-based discrepancies could explain to some degree why female customers have higher purchasing intentions for cultural and creative products. The results further imply that the more that influencer behavior exists in virtual communities, the more women will participate in transaction behaviors. Previous studies have characterized men as agentic (assertive, self-centered) and women as communal (friendly, other-oriented) (Putrevu, 2001). Women can easily sympathize with others’ opinions and interests when they connect with others (Putrevu, 2001), which may partially explain why influencer behavior mediates the effect of social identity on purchase behavior in the female model. In the process of online shopping, the identification of groups is somewhat significant for women and can prompt their interaction with products.
In their study of virtual community, Dholakia et al. (2004) categorized virtual community types as either network-based or small-group-based. In our research, we focused on the effects of social identity on customer behaviors. Future studies may be conducted on the features of different types of virtual communities. There are several types of Chinese mainland virtual communities, including network-based communities where public discussions are open to anyone, and small-group-based communities where discussions are private and for members only. Furthermore, the comment areas below online videos have gradually become a type of knowledge and information exchange community where followers gather. Moreover, the relationship between how firms get involved in virtual communities and customers’ engagement behaviors has not yet been explored, and should be discussed in depth in the future.
The datasets presented in this study can be found in online repositories. The names of the repository/repositories and accession number(s) can be found in the article/Supplementary Material.
The studies involving human participants were reviewed and approved by the University of Shanghai for Science and Technology Ethics Committee. The patients/participants provided their written informed consent to participate in this study.
ZZ and WL: drafting of the manuscript, acquisition of data, and statistical analysis. Both authors contributed to the article and approved the submitted version.
This research was funded by the Humanities and Social Science Fund of the Ministry of Education of China, grant number 19YJC760046.
The authors declare that the research was conducted in the absence of any commercial or financial relationships that could be construed as a potential conflict of interest.
All claims expressed in this article are solely those of the authors and do not necessarily represent those of their affiliated organizations, or those of the publisher, the editors and the reviewers. Any product that may be evaluated in this article, or claim that may be made by its manufacturer, is not guaranteed or endorsed by the publisher.
The Supplementary Material for this article can be found online at: https://www.frontiersin.org/articles/10.3389/fpsyg.2022.874851/full#supplementary-material
Ahearne, M., Bhattacharya, C. B., and Gruen, T. (2005). Antecedents and consequences of customer-company identification: expanding the role of relationship marketing. J. Appl. Psychol. 90, 574–585. doi: 10.1037/0021-9010.90.3.574
Ashforth, B. E., and Mael, F. (1989). Social Identity Theory and the Organization. Acad. Manag. Rev. 14, 20–39. doi: 10.5465/amr.1989.4278999
Bagozzi, R. P., and Dholakia, U. M. (2002). Intentional social action in virtual communities. J. Interact. Mark. 16, 2–21. doi: 10.1002/dir.10006
Bergami, M., and Bagozzi, R. P. (2000). Self-categorization, affective commitment and group self-esteem as distinct aspects of social identity in the organization. Br. J. Soc. Psychol. 39, 555–577. doi: 10.1348/014466600164633
Bhattacharya, C. B., and Sen, S. (2003). Consumer–company identification: A framework for understanding consumers’ relationships with companies. J. Mark. 67, 76–88. doi: 10.1509/jmkg.67.2.76.18609
Castro, S., Lacap, J. P., Garbosa, T. M., Jimenez, E., and Mallari, S. F. (2022). Philippine television network companies’ corporate social responsibility engagements and electronic word-of-mouth: the intervening role of consumer-company identification. J. Asia Pac. Bus. 1–22. doi: 10.1080/10599231.2022.2025643
Chatterjee, P. (2001). “Online reviews: do consumers use them?,” in Proceedings of the ACR, eds M. C. Gilly and J. Myers-Levy (Provo, UT: Association for Consumer Research).
Chen, M. H., and Tsai, K. M. (2020). An empirical study of brand fan page engagement behaviors. Sustain 12:434. doi: 10.3390/SU12010434
Cheung, G. W., and Rensvold, R. B. (1999). Testing factorial invariance across groups: A reconceptualization and proposed new method. J. Manage. 25, 1–27. doi: 10.1177/014920639902500101
Chih, W.-H., Hsu, L.-C., and Liou, D.-K. (2017). Understanding virtual community members’ relationships from individual, group, and social influence perspectives. Ind. Manag. Data Syst. 117, 990–1010. doi: 10.1108/IMDS-03-2016-0119
Chih, W. H., Wang, K. Y., Hsu, L. C., and Huang, S. C. (2013). Investigating electronic word-of-mouth effects on online discussion forums: The role of perceived positive electronic word-of-mouth review credibility. Cyberpsychology, Behav. Soc. Netw. 16, 658–668. doi: 10.1089/cyber.2012.0364
Chinese National Bureau of Statistics. (2021). National-Scale Enterprises in Culture and Related Industries to Grow their Business Revenue by 2.2% in 2020. Available online at: http://www.stats.gov.cn/tjsj/zxfb/202101/t20210129_1812934.html (accessed on Jan 31, 2021).
Choi, N. (2013). Information systems attachment: An empirical exploration of its antecedents and its impact on community participation intention. J. Am. Soc. Inf. Sci. Technol. 64, 2354–2365. doi: 10.1002/asi.22939
De Oliveira, M. J., Huertas, M. K. Z., and Lin, Z. (2016). Factors driving young users’ engagement with Facebook: Evidence from Brazil. Comput. Human Behav. 54, 54–61. doi: 10.1016/j.chb.2015.07.038
de Silva, T. M. (2019). Building relationships through customer engagement in Facebook brand pages. Mark. Intell. Plan. 38, 713–729. doi: 10.1108/MIP-02-2019-0085
Dholakia, U. M., Bagozzi, R. P., and Pearo, L. K. (2004). A social influence model of consumer participation in network- and small-group-based virtual communities. Int. J. Res. Mark. 21, 241–263. doi: 10.1016/j.ijresmar.2003.12.004
Elbedweihy, A. M., Jayawardhena, C., Elsharnouby, M. H., and Elsharnouby, T. H. (2016). Customer relationship building: The role of brand attractiveness and consumer–brand identification. J. Bus. Res. 69, 2901–2910. doi: 10.1016/j.jbusres.2015.12.059
Ellemers, N., Kortekaas, P., and Ouwerkerk, J. W. (1999). Self-categorisation, commitment to the group and group self-esteem as related but distinct aspects of social identity. Eur. J. Soc. Psychol 29, 371–389. doi: 10.1002/(sici)1099-0992(199903/05)29:2/3<371::aid-ejsp932<3.0.co;2-u
Fornell, C., and Larcker, D. F. (1981). Evaluating structural equation models with unobservable variables and measurement error. J. Mark. Res. 18, 39–50. doi: 10.1177/002224378101800104
Gutfreund, J. (2016). Move over. Millennials: Generation Z is changing the consumer landscape. J. Brand Strateg. 5, 245–249.
Hair, J. F. Jr., Black, W. C., and Babin, B. J. (2009). Multivariate Data Analysis. 7th Edn. London: Pearson, 677–678.
Hajli, N., Shanmugam, M., Powell, P., and Love, P. E. D. (2015). A study on the continuance participation in on-line communities with social commerce perspective. Technol. Forecast. Soc. Change 96, 232–241. doi: 10.1016/j.techfore.2015.03.014
He, H., Li, Y., and Harris, L. (2012). Social identity perspective on brand loyalty. J. Bus. Res. 65, 648–657. doi: 10.1016/j.jbusres.2011.03.007
He, X. (2005). On idolatry and education among youth and adolescence. International Journal of Adolescence and Youth 11, 1–26.
HooperD, C. J., and Mullen, M. (2008). Structural equation modelling: guidelines for determining model fit. Electron J Bus Res Methods 6, 53–60.
Hsu, L.-C., Wang, K.-Y., and Chih, W.-H. (2018). Investigating virtual community participation and promotion from a social influence perspective. Ind. Manag. Data Syst. 118, 1229–1250. doi: 10.1108/IMDS-10-2017-0477
Ivanova, N., Volkova, A., and Patosha, O. (2022). The Relationship Between Social Identity and Consumer Behavior. Dubrovnik International Economic Meeting 7, 72–79. doi: 10.17818/DIEM/2022/1.8
Jalilvand, M. R., and Samiei, N. (2012). The effect of electronic word of mouth on brand image and purchase intention: An empirical study in the automobile industry in Iran. Mark. Intell. Plan. 30, 460–476. doi: 10.1108/02634501211231946
Joshi, A. W., and Sharma, S. (2004). Customer knowledge development: Antecedents and impact on new product performance. J. Mark. 68, 47–59. doi: 10.1509/jmkg.68.4.47.42722
Kahn, W. A. (1990). Psychological conditions of personal engagement and disengagement at work. Acad. Manag. J. 33, 692–724. doi: 10.5465/256287
Katz, M. L., and Shapiro, C. (1985). Network externalities, competition, and compatibility. Am. Econ. Rev. 75, 424–440.
Kucharska, W. (2017). Consumer social network brand identification and personal branding. How do social network users choose among brand sites? Cogent Bus. Manag 4:1315879. doi: 10.1080/23311975.2017.1315879
Kucharska, W. (2019). Online brand communities’ contribution to digital business models: Social drivers and mediators. J. Res. Interact. Mark. 13, 437–463. doi: 10.1108/JRIM-05-2018-0063
Kumar, V., Aksoy, L., Donkers, B., Venkatesan, R., Wiesel, T., and Tillmanns, S. (2010). Undervalued or overvalued customers: Capturing total customer engagement value. J. Serv. Res. 13, 297–310. doi: 10.1177/1094670510375602
Malhotra, Y., and Galletta, D. F. (1999). Extending the Technology Acceptance Model to account for social influence: Theoretical bases and empirical validation. Proc. Hawaii Int. Conf. Syst. Sci* 5, doi: 10.1109/hicss.1999.772658
Marsh, H. W., and Hocevar, D. (1985). Application of Confirmatory Factor Analysis to the Study of Self-Concept. First- and Higher Order Factor Models and Their Invariance Across Groups. Psychol. Bull. 97, 562–582. doi: 10.1037/0033-2909.97.3.562
Osei-Frimpong, K., and McLean, G. (2018). Examining online social brand engagement: A social presence theory perspective. Technol. Forecast. Soc. Change 128, 10–21. doi: 10.1016/j.techfore.2017.10.010
Prentice, C., Han, X. Y., Hua, L.-L., and Hu, L. (2019). The influence of identity-driven customer engagement on purchase intention. J. Retail. Consum. Serv. 47, 339–347. doi: 10.1016/j.jretconser.2018.12.014
PR World. (2019). 2019 Museum Cultural and Creative Products Market Statistical Analysis Report. PR World. Germany: Federal Ministry for Economic Affairs and Energy.
Putrevu, S. (2001). Exploring the origins and information processing differences between men and women: Implications for advertisers. Acad. Mark. Sci. Rev. 10, 1–14.
Reza Jalilvand, M., Samiei, N., Dini, B., and Yaghoubi Manzari, P. (2012). Examining the structural relationships of electronic word of mouth, destination image, tourist attitude toward destination and travel intention: An integrated approach. J. Destin. Mark. Manag. 1, 134–143. doi: 10.1016/j.jdmm.2012.10.001
Schumacker, R. E., and Lomax, R. G. (2004). A Beginner’s Guide to Structural Equation Modeling. East Sussex: psychology press.
Sharma, S., and Crossler, R. E. (2014). Disclosing too much? Situational factors affecting information disclosure in social commerce environment. Electron. Commer. Res. Appl. 13, 305–319. doi: 10.1016/j.elerap.2014.06.007
Shen, J. (2012). Social comparison, social presence, and enjoyment in the acceptance of social shopping websites. J. Electron. Commer. Res. 13:198.
Shi, Y. (2010). Research on Knowledge Sharing and It’s Influencing Factors in SNS Virtual Community. Doctoral dissertation. Shanxi: Shanxi University.
Sobel, M. E. (1982). Asymptotic intervals for indirect effects in structural equations models. S. Leinhart. Sociol. Methodol 13, 290–312. doi: 10.2307/270723
Tajfel, H., and Wilkes, A. L. (1963). Classification and quantitative judgement. Br. J. Psychol. 54, 101–114. doi: 10.1111/j.2044-8295.1963.tb00865.x
Tajfel, H. E. (1978). Differentiation between social groups: Studies in the social psychology of intergroup relations. Cambridge: Academic Press.
Taylor, A. B., MacKinnon, D. P., and Tein, J. Y. (2008). Tests of the three-path mediated effect. Organ. Res. Methods 11, 241–269. doi: 10.1177/1094428107300344
Tsai, H.-T., and Bagozzi, R. P. (2014). Contribution behavior in virtual communities: Cognitive, emotional, and social influences. Mis Q. 38, 143–164. doi: 10.25300/misq/2014/38.1.07
Tseng, F.-M., and Hsu, F.-Y. (2010). “The influence of eWOM within the online community on consumers’ purchasing intentions-the case of the Eee PC,” in Proceedings of the The 2010 International Conference on Innovation and Management, (IAM 2010), Penang. doi: 10.1108/yc-03-2019-0982
Van den Bulte, C., and Wuyts, S. H. K. (2007). Social networks in marketing. Massachusetts: Marketing Science Institute.
Van Doorn, J., Lemon, K. N., Mittal, V., Nass, S., Pick, D., Pirner, P., et al. (2010). Customer engagement behavior: Theoretical foundations and research directions. J. Serv. Res. 13, 253–266. doi: 10.5465/256287
Verhoef, P. C., Reinartz, W. J., and Krafft, M. (2010). Customer engagement as a new perspective in customer management. J. Serv. Res. 13, 247–252. doi: 10.1177/1094670510375461
Vivek, S. D., Beatty, S. E., Dalela, V., and Morgan, R. M. (2014). A generalized multidimensional scale for measuring customer engagement. J. Mark. Theory Pract. 22, 401–420. doi: 10.2753/MTP1069-6679220404
Wang, T. (2017). Social identity dimensions and consumer behavior in social media. Asia Pacific Manag. Rev. 22, 45–51. doi: 10.1016/j.apmrv.2016.10.003
Wei, W., Miao, L., and Huang, Z. (2013). Customer engagement behaviors and hotel responses. Int. J. Hosp. Manag. 33, 316–330. doi: 10.1016/j.ijhm.2012.10.002
Wielki, J. (2020). Analysis of the role of digital influencers and their impact on the functioning of the contemporary on-line promotional system and its sustainable development. Sustain 12, 7138. doi: 10.3390/su12177138
Wolter, J. S., and Cronin Jr. (2017). Unique influences of cognitive and affective customer-company identification. J. Bus. Res. 78, 172–179. doi: 10.1016/j.jbusres.2017.05.010
Xiang, L., Zheng, X., and Hu, X. (2014). “What drives social commerce: the role of parasocial interaction,” in Proceedings of the PACIS 2014 (AIS eLibrary), Chengdu, 86.
Yang, M., Hu, S., Kpandika, B. E., and Liu, L. (2021). Effects of social attachment on social media continuous usage intention: the mediating role of affective commitment. Hum. Syst. Manag. 40, 619–631. doi: 10.3233/HSM-201057
Zaglia, M. E. (2013). Brand communities embedded in social networks. J. Bus. Res. 66, 216–223. doi: 10.1016/j.jbusres.2012.07.015
Zhao, L., Lu, Y., and Deng, Z. (2009). A Study on Trust and Members’ Purchase Behavior in Virtual Communities. Ind. Eng. Manag 14, 105–111. doi: 10.19495/j.cnki.1007-5429.2009.03.021
Keywords: social identity, cultural and creative products, customer engagement behaviors, social media, purchase intention
Citation: Zhang Z and Li W (2022) Customer Engagement Around Cultural and Creative Products: The Role of Social Identity. Front. Psychol. 13:874851. doi: 10.3389/fpsyg.2022.874851
Received: 13 February 2022; Accepted: 23 March 2022;
Published: 25 April 2022.
Edited by:
Huayu Shi, Shanghai Institute of Technology, ChinaReviewed by:
Manish Gupta, Mahindra University, IndiaCopyright © 2022 Zhang and Li. This is an open-access article distributed under the terms of the Creative Commons Attribution License (CC BY). The use, distribution or reproduction in other forums is permitted, provided the original author(s) and the copyright owner(s) are credited and that the original publication in this journal is cited, in accordance with accepted academic practice. No use, distribution or reproduction is permitted which does not comply with these terms.
*Correspondence: Wenjia Li, bGl3ZW5qaWFAdXNzdC5lZHUuY24=
Disclaimer: All claims expressed in this article are solely those of the authors and do not necessarily represent those of their affiliated organizations, or those of the publisher, the editors and the reviewers. Any product that may be evaluated in this article or claim that may be made by its manufacturer is not guaranteed or endorsed by the publisher.
Research integrity at Frontiers
Learn more about the work of our research integrity team to safeguard the quality of each article we publish.