- School of Foreign Languages, University of Economics Ho Chi Minh City (UEH), Ho Chi Minh City, Vietnam
The article presents a methodological update on the lexical profile of informal spoken English with the emphasis on movies, television programs, and soap operas. The study analyzed Mark Davies’s mega-corpora with data containing approximately 625 million words and employed Paul Nation’s comprehensive and up-to-date British National Corpus/Corpus of Contemporary American English (BNC/COCA) wordlists. Data from the analyses showed that viewers would need a vocabulary knowledge at 3,000 and 5,000 words frequency levels to understand 95 and 98% of the words in scripted dialogs, respectively. Soap operas were found to be less lexically demanding compared to TV programs and movies. Findings are expected to fill in the methodological gaps between vocabulary assessment and vocabulary profiling research.
Introduction
Vocabulary is the most fundamental aspect in language, and the importance of vocabulary has been constantly repeated (Nation, 2013; Webb, 2020). Together with the development of wordlists, such as the British National Corpus (BNC) lists (Nation, 2006) and the British National Corpus/Corpus of Contemporary American English (BNC/COCA) lists (Nation, 2017), researchers in the field of vocabulary studies have been continuously giving us interesting perspectives regarding our vocabulary knowledge as well as the lexical resource we would need to comprehend different text genres (Nurmukhamedov and Webb, 2019).
While vocabulary assessment and vocabulary profiling could be said to be the two fields of vocabulary studies that receive the most attention, it does seem to me that research in vocabulary testing are moving way so faster that findings in vocabulary profiling are almost left behind, causing certain gap in research methodology. For example, while vocabulary tests have long employed Nation’s (2017) up-to-date BNC/COCA wordlist as the source of test items (McLean and Kramer, 2015, 2016; McLean et al., 2015; Webb et al., 2017), many recent studies on lexical coverage of texts were still stick to the Nation’s (2006) BNC wordlist (Al-Surmi, 2014; Dang and Webb, 2014; Webb and Paribakht, 2015; Nurmukhamedov, 2017; Tegge, 2017). This led to the situation where researchers who utilized these modern vocabulary tests for their studies could not reliably relate their results to the existing findings in the field. Attempts have been made to fill in the methodological gaps (Hsu, 2018; Yang and Coxhead, 2020; Nurmukhamedov and Sharakhimov, 2021), however, they are few, and certain areas of the field, including scripted and unscripted spoken discourses, remained uncovered. As a result, research on the relationship between phonological vocabulary knowledge and listening comprehension (Cheng and Matthews, 2018; Lange and Matthews, 2020; Ha, 2021b) still had to rely on the findings of Webb and Rodgers (2009a,b), which are more than 10 years old and ripe for being updated. In response to the dire need for methodologically updated findings, the present study was conducted to revisit the vocabulary demands of informal spoken English.
Literature Review
Receptive Vocabulary Knowledge and Listening Comprehension
For decades, vocabulary linguists have documented a strong link between receptive vocabulary knowledge and listening comprehension (van Zeeland and Schmitt, 2013; Cheng and Matthews, 2018; Lange and Matthews, 2020; Ha, 2021b). One of the most interesting findings was the concept of lexical demand and lexical coverage. In short, lexical demand refers to the proportion of words in a text a learner need to know to adequately comprehend it. It has been generally agreed that the minimum threshold for acceptable comprehension is 95% coverage and the coverage for optimal text comprehension would be 98% (Schmitt et al., 2011; van Zeeland and Schmitt, 2013). As Hu and Nation (2000) explained, when learners knew 95% of the running word in a text, they would encounter an unfamiliar token in every 20 words, and that ratio would be reduced to 1/50 if they were to be familiar with 98% of the tokens, a huge gap for a 3% difference.
Lexical Profile of Spoken English
The best thing about lexical coverage studies is that they are often based on word frequency lists, which would show teachers and learners the fastest route to achieve their teaching or learning targets. These wordlists use “word family” as word counting unit. A word family generally refers to a headword and all of its inflectional and derivational forms through a level 6 affix criteria (also known as WF6; Bauer and Nation, 1993; Nation, 2020). For example, the WF6 for add in Nation’s (2017) BNC/COCA lists includes added, adding, addition, additional, additionality, additionally, additions, additive, additives, adds. The WF6 have been the foundation for several aspects of vocabulary studies (Nurmukhamedov and Webb, 2019).
Past studies based on Nation (2006) British National Corpus (BNC) have formed comprehensive guidelines for English teachers and learners on what and how much to learn. For example, for informal, spoken English, learners would need to know the around 2,000–3,000 word families to achieve 95% coverage and 5,000–7,000 word families for 98% coverage (Nation, 2006; Webb and Rodgers, 2009a,b; Al-Surmi, 2014; Tegge, 2017). Academic spoken English, the type of English we would encounter in TED talks, academic seminars, and university lectures, was a little bit more lexically demanding, generally requiring a knowledge of 4,000 and 8,000 most frequent word families in the BNC word list for 95 and 98% coverage, respectively, (Coxhead and Walls, 2012; Dang and Webb, 2014; Nurmukhamedov, 2017).
Research Gap and the Present Study
Improvement demands changes. As the English we use keeps developing every day, it is not surprising to say that the guidelines for vocabulary teaching and learning that has been built on Nation’s (2006) BNC lists would soon become obsolete, and therefore, require revisiting (Schmitt et al., 2017). In an influential paper, Schmitt et al. (2017) suggested two directions that future studies should take to replicate past lexical profile research. The first one is to increase the sample size. This statement was made based on the fact that these past studies employed relatively small corpora and their findings “now need to be checked with larger, more comprehensive corpora” (Schmitt et al., 2017, p. 217). The second suggestion Schmitt et al. (2017) put forward is the improvement in research methodology. Despite being extremely helpful and informative, Nation’s (2006) BNC word list is now 15 years old and contains primarily British English which should be “due for updating and revision” (Schmitt et al., 2017, p. 218). In an attempt to create a better version of the BNC, Paul Nation introduced the British National Corpus/Corpus of Contemporary American English (BNC/COCA) wordlist in 2012, which were later updated in 2017. The BNC/COCA is a highly regarded wordlist by researchers (Dang and Webb, 2016; Dang et al., 2020). As Schmitt et al. (2017) pressed, “Assuming the new combined BNC-COCA lists are a better indication of word frequency, then everything that has been done using the original BNC-based lists is ripe for replication using these new lists” (Schmitt et al., 2017, p. 218).
The present study was conducted in response to Schmitt et al. (2017) call and aimed at revisiting the vocabulary demands of informal spoken English. People often believe that the investigation of informal spoken English should involve real-life, conversations (Love et al., 2017). However, lexical research demonstrated that the examination on scripted English would yield similar results and be of as much help (Webb and Rodgers, 2009a,b; Al-Surmi, 2014; Davies, 2021). To date, four studies have been conducted to investigate the lexical profile of informal spoken English through soap operas (Al-Surmi, 2014), podcasts (Nurmukhamedov and Sharakhimov, 2021), TV programs (Webb and Rodgers, 2009a), and movies (Webb and Rodgers, 2009b). Tegge (2017) is not counted because song lyrics do not always reflect real-life conversations. Nurmukhamedov and Sharakhimov (2021) is very updated with their research methodology and a replication study would be unnecessary. As a result, only three of them need to be revisited in Schmitt et al. (2017) terms. Table 1 shows information regarding the sample size, the wordlist that was used as research methodology as well as the key findings of these past studies.
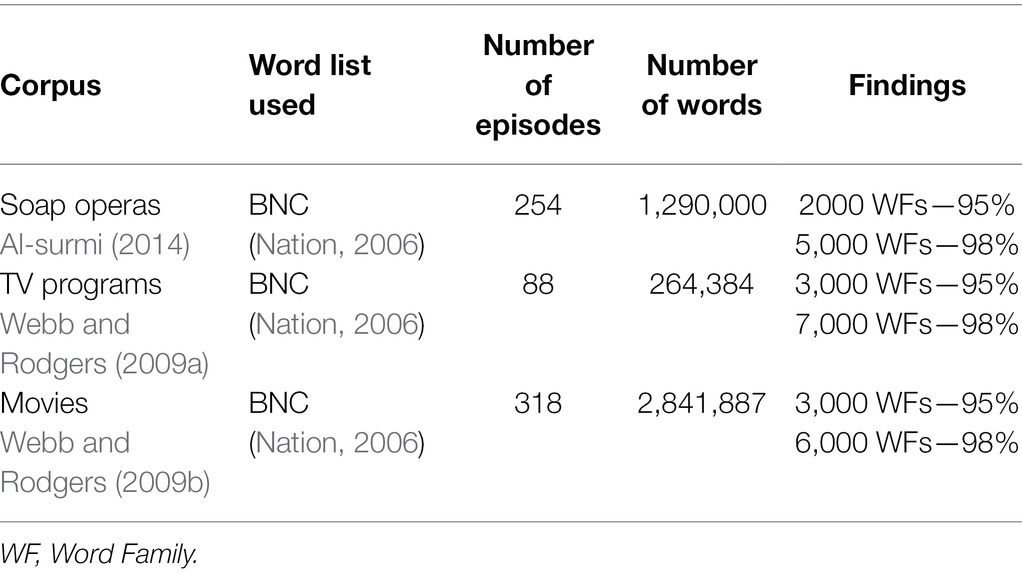
Table 1. A summary of past studies on the vocabulary demands of soap operas, TV programs, and movies.
By employing the most comprehensive and updated wordlist, the BNC/COCA, as well as the largest corpus of scripted English available (more details in the methodology section), the present study seeks to answer only one research question:
Would the previous findings concerning the lexical demands of TV, Movies, and Soap Opera change as larger corpora and the BNC/COCA wordlist were applied?
Methodology
Data Collection
The data analyzed in the present study were the TV (Davies, 2019a), Movies (Davies, 2019b), and SOAP (Davies, 2012) corpora, which were officially purchased and used under an academic license provided by Mark Davies.1 The TV, Movies, and SOAP corpora together could be said to be the largest available corpus of informal spoken English with data containing approximately 625 million tokens in total (Davies, 2021). Information regarding the three corpora is presented in Table 2.
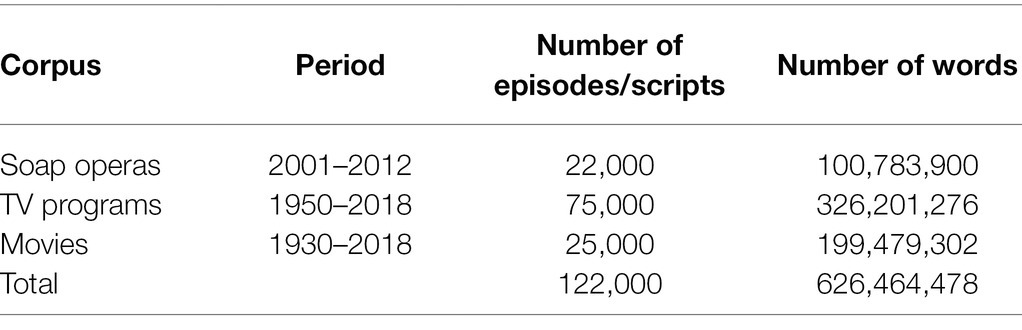
Table 2. General information about the corpora (Davies, 2012, 2019a,b).
Data Analysis
Preliminary analysis showed three major issues that had to be dealt with before the purchased corpora could be ready for any further data analysis. The first and most important thing was context-defining words. Davies’s spoken corpora are normally flooded with words that “represent the tone of style of speech” (Davies, 2021, p. 16) or gives additional information on the context, all of which are surrounded by parentheses, for example, (Enginechuggingnoisily), (doorknocking), and (treecracking), (gunfire). These words are “non-speech” words, (Davies, 2021, p. 16), and therefore, should not be included in the analysis. Another problem that needed attention was hyphenated words (second-hand, sky-high…) as lexical profiling software could not read them. The third issue involves words that accidentally stick together (whatcouldpossiblybegoing, whatchancehasawomangot…) and other typos errors, which were falsely classified by lexical profiling software as “Not in the lists.”
The parentheses that surrounded context-defining words were replaced with “<” and “>,” so that Range could identify and automatically exclude these words through the “ignore ‘< >’” function. These words accounted for 592,690, 2,577,943, and 1,689,465 tokens in the SOAP, TV, and MOVIES corpora, respectively. Hyphens in hyphenated words were then replaced by space so that the component words could be classified according to their frequency level. Finally, words that were classified as “Not in the lists” due to typos were then changed and returned to their frequency levels. These modifications were made using the mass search and replace function of Notepad++ (hotkeys: Ctrl + Shift + F).
The corpora’s lexical profile was then analyzed by Range (Heatley et al., 2002). Range is a computer program that could classify words to their frequency levels in accordance with the word lists we chose to use it with. Range were chosen for data analysis due to the researcher’s personal preference and familiarity. In fact, an analysis with AntWordProfiler 1.5.1 (Anthony, 2021) yielded near-identical results for the corpora. Therefore, such program choice should not be the cause for concern. Range can automatically identify and read contractions (cannot, do not…) and connected speech (wanna, gonna, kinda…). For instance, Range counts cannot as can and not and wanna as a family member of want.
The up-to-date, comprehensive, 25-level BNC/COCA wordlist (Nation, 2017) were used together with Range for the analysis. The BNC/COCA wordlist contains twenty-five 1,000-word levels which reflects current British and American English. The BNC/COCA lists are accompanied by four supplementary lists of proper nouns (Abraham, Portuguese, Waterloo…), marginal words (hm, yee, phew…), transparent compounds (racecar, railway, sailboat…), and acronyms (PHD, NATO, MPHI…; Nation, 2020).
Results
Table 3 presents the number of words and their proportion at each frequency level in the BNC/COCA wordlist for the SOAP, TV, and Movies corpora. Proper nouns, marginal words, transparent compounds, and acronyms were treated as separate word levels.
It is observable that around 85% of the three corpora were made up of the most frequent word families in the BNC/COCA and that nearly 90% of the corpora’s tokens were covered by the first two most frequent word families. This may be because the first two 1,000-word levels in the BNC/COCA lists primarily contain words taken from spoken corpora (Nation, 2020). The proportion of tokens showed a gradual decrease as the word frequency went down, and after the 5,000 level, the figures dropped below 1% for all the corpora, signaling the importance of high-frequency words.
Another detail that deserves attention is the proportion of proper nouns in the three corpora, which were relatively considerable, especially for the SOAP corpus. Proper nouns normally do not cause significant difficulties for reading comprehension since they can be easily recognized with the first-letter capitalization. However, concerns have been raised on the effect of proper nouns on listening comprehension (Kobeleva, 2012; Klassen, 2021). In general, auxiliary words including proper nouns (PN), marginal words (MW), transparent compounds (TC), and acronyms accounted for approximately 11% for the SOAP corpus and nearly 6% for the TV and Movies corpora.
The cumulative coverage at each word frequency level is illustrated in Table 4. At this stage, two assumptions were put forward, the first one supposes that learners did not know and could not recognize PNs, MWs, TCs, and acronyms, and the second one assumes that learners knew or could easily recognize these words.
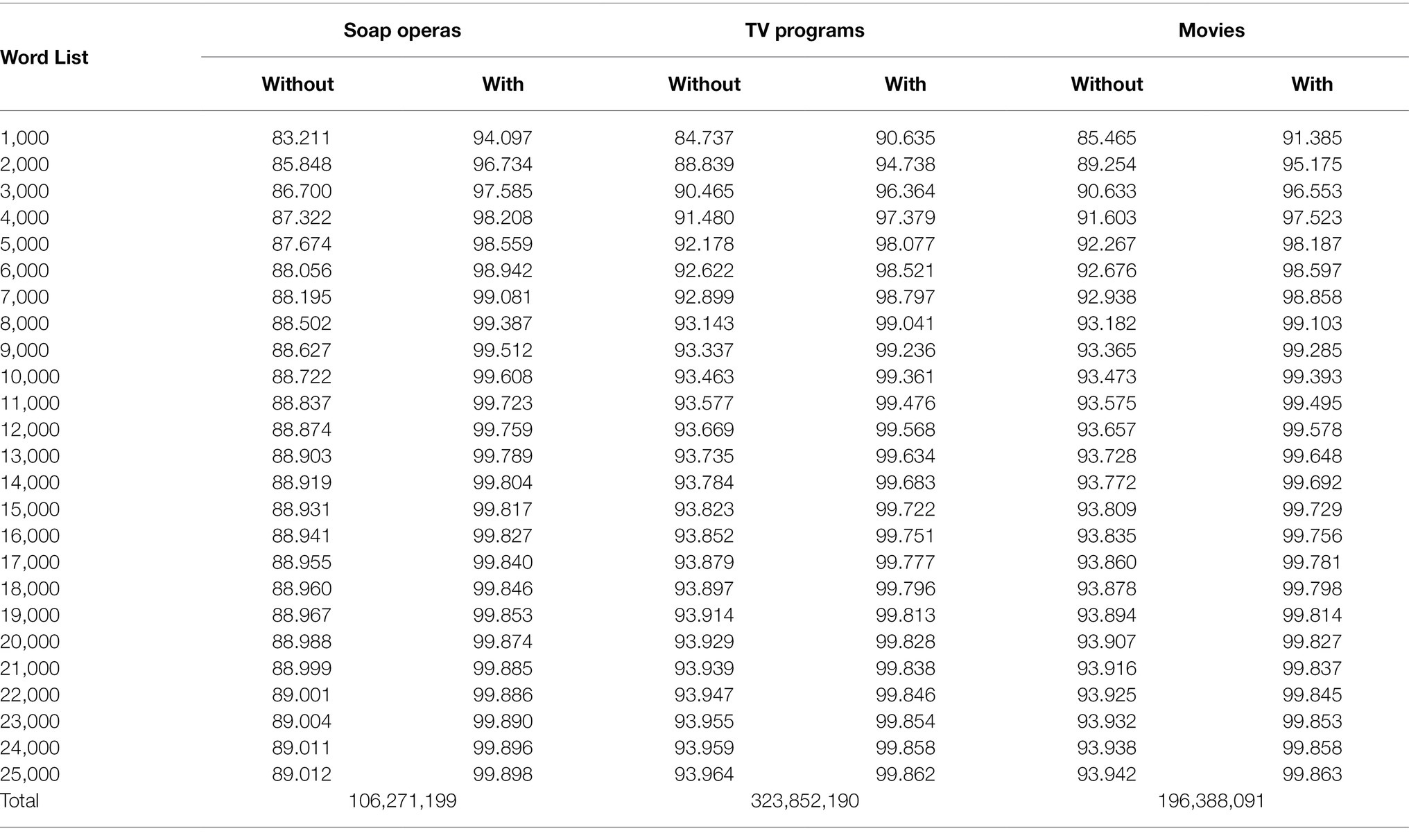
Table 4. Cumulative coverage with and without proper nouns, marginal words, transparent compounds, and acronyms.
It is obvious that without the knowledge of PN, MW, TC, and acronyms, it is impossible for viewers to achieve the minimum coverage threshold for comprehension, which is indeed worrying. However, when PN, MW, TC, and acronyms were assumed to be known, then we can once again see the optimistic scenario depicted in Webb and Rodgers (2009a,b), Tegge (2017), and Nurmukhamedov and Sharakhimov (2021). Generally, it only took 2,000–3,000 most frequent word families in the Nation’s (2017) BNC/COCA lists to cover 95% of the tokens in the corpora, and the vocabulary knowledge of the most frequent 4,000–5,000 word families was all required to reach the optimal threshold for comprehension.
Certain differences can be observed between the corpora. To be more specific, soap operas demanded the least lexical knowledge for 95% (2,000 WFs) and 98% (4,000 WFs) coverage compared to the other two. TV programs and movies share similar lexical demands when it comes to the 98% threshold. However, data from the analysis showed that the Movies corpus managed to reach the 95% coverage at the 2,000 level, while the TV programs would require a word knowledge at 3,000 level for 95% coverage. Still, it worth noting that the actual difference was really thin, approximately 0.2%, and had the tendency to become smaller as it moved down the word levels. Therefore, it is safe to state that movies and TV programs shared relatively similar vocabulary demands.
Discussion
The paper revisited research on the vocabulary profiles of soap operas (Al-Surmi, 2014), TV programs, and movies (Webb and Rodgers, 2009a,b) to see if changes in sample size and research methodology would result in changes in findings. Data from the analyses showed that if the BNC/COCA wordlist were to be used as an indicator of word frequency, then the lexical demands of the researched text genres would be generally reduced. To be more specific, if learners were to base their vocabulary learning on the BNC/COCA lists, they would only need to learn 4,000 (instead of 5,000) and 5,000 (instead of 6,000 and 7,000) word families to understand 98% of the words in soap operas and movies and TV programs, respectively. It should be noted that the 1,000–2,000 word families difference could be translated into 2–4 years of English learning or even more (Webb and Chang, 2012; Ozturk, 2016).
However, the so-called “reduced lexical demands” are, in actual practice, the additional effect of the four supportive lists (PN, MW, TC, and Acronym). If we were to compare the cumulative coverage at the 3,000 and 5,000 levels, we could easily find similar figures between BNC/COCA-based and BNC-based studies. And if we were to go even further and add the proportion of the four supportive lists (PN, MW, TC, and Acronym) of this study to the cumulative coverage figures at any word level in Webb and Rodgers (2009a,b) and Al-Surmi (2014), then the same reduced lexical coverage (or even better) could be recorded. Still, this does not mean that we could simply use the BNC lists together with the four additional lists in the BNC/COCA lists. Nation (2020) introduced the BNC/COCA wordlist with clear rationales which have been proven by other researchers (Dang and Webb, 2016; Dang et al., 2020), which means that these lists were designed to work together and scholars are not encouraged to apply questionable practices to avoid unnecessary problems.
The shift in lexical profiling studies from the BNC (Nation, 2006) to the BNC/COCA (Nation, 2017) could be said to be inevitable as it harmonizes different aspects of vocabulary research. Since most vocabulary tests now utilize the BNC/COCA as the source for test items (McLean and Kramer, 2015; McLean et al., 2015; Webb et al., 2017; Ha, 2021a), it would be somehow methodologically inconsistent to relate students’ results on vocabulary test that is based on the BNC/COCA wordlist to findings of lexical studies that employed the BNC lists. Together with the development of phonological vocabulary tests (McLean et al., 2015; Ha, 2021a), teachers and vocabulary linguists can now make a reliable connection between the aural vocabulary knowledge of their students and what they can possibly understand in the real world.
The study’s findings were also in line with Nurmukhamedov and Sharakhimov (2021) which employed Nation’s (2017) BNC/COCA lists to investigate the lexical profile of English podcasts. Nurmukhamedov and Sharakhimov (2021) found that a vocabulary knowledge at the 3,000 and 5,000 levels would cover 96.75 and 98.26% of the words in English podcasts, correspondingly. These results generally suggested that learning the 5,000 most frequent word families in the BNC/COCA wordlist, an attainable learning goal for English learners in most contexts, could help learners achieve unsupported listening comprehension for informal spoken English. This claim is supported by van Zeeland and Schmitt’s (2013) study which proved that knowing 98% of the running words in a listening text would result in very high degree of listening comprehension.
The study recorded considerable proportions of PN, MW, TC, and acronyms, which aligned well with Nurmukhamedov and Sharakhimov (2021). Vocabulary linguists tend to make the assumption that these words could be easily and recognized by learners (Nation, 2006; Webb and Rodgers, 2009a,b; Nurmukhamedov and Sharakhimov, 2021). Although these assumptions could be acceptable for reading, concerns have been raised about whether it is appropriate to assume the same thing to listening (Kobeleva, 2012; Klassen, 2021). It is true that without the support of orthographic form, proper nouns or even acronyms are difficult to distinguish, and for listening-only formats like podcasts, such concerns are in evidence. However, television programs, movies, and soap operas, which are strongly supported by visualization, are different from podcasts. These non-verbal clues, such as facial expression, body gestures, and lips movements, are considered be of significant support to the processing of aural input (Harris, 2003). Such visual clues may help viewers recognize and understand PN, MW, TC, and acronyms.
Conclusion
This research’s findings offer updates on the lexical profiles of informal spoken English by employing up-to-date research methodology and large sample size. In general, it is evident that the BNC/COCA wordlist (Nation, 2017) would give English learners and teachers a shorter route to their intended goals. This BNC/COCA-based update also connected research findings on vocabulary profiling and vocabulary assessment, which have been in conflict for several years due to incompatible methodologies.
Despite being informative, this brief research report bears certain limitations. First, the paper revisited the findings of several studies at once, which would give readers a broad overview of the new findings and how these findings related to each other. Therefore, it was not possible for the researcher to go deeper and explore the variation in lexical coverage among texts. Future research should take a deeper look into each corpus in isolation and examine the variation in lexical demands of each text genre. Secondly, although the study showed the number of word families learners would need to achieve 95 and 98% coverage in informal spoken English. It cannot guarantee that learners would successfully comprehend a text should these requirements be satisfied. Graham (2006) showed that people may understand every single word in a text and still fail to get the general meaning, which could be due to other factors in the learners’ language proficiency and metacognitive awareness. As a result, researchers are encouraged to investigate these issues, which could possibly be done by replicating van Zeeland and Schmitt’s (2013) research methodology.
Data Availability Statement
The data analyzed in this study is subject to the following licenses/restrictions: The corpora that support the findings of this study are available from Mark Davies. Restrictions apply to the availability of these corpora, which were used under academic license for this study. Data are available from https://www.english-corpora.org/ with the permission of Mark Davies. Requests to access these datasets should be directed to mark.davies@corpusdata.org.
Author Contributions
The author confirms sole responsibility for the following: study conception and design, data collection, analysis and interpretation of results, and manuscript preparation.
Conflict of Interest
The author declares that the research was conducted in the absence of any commercial or financial relationships that could be construed as a potential conflict of interest.
Publisher’s Note
All claims expressed in this article are solely those of the authors and do not necessarily represent those of their affiliated organizations, or those of the publisher, the editors and the reviewers. Any product that may be evaluated in this article, or claim that may be made by its manufacturer, is not guaranteed or endorsed by the publisher.
Abbreviations
BNC, British National Corpus; COCA, Corpus of Contemporary American English; ESL, English as a second language; MW, Marginal words; NOW, News on the web; PN, Proper nouns; TC, Transparent compounds.
Footnotes
References
Al-Surmi, M. (2014). “TV shows, word coverage, and incidental vocabulary learning,” in Teaching and Learning English in the Arabic-Speaking World. eds. K. Bailey and R. Damerow (London: Routledge), 132–147.
Bauer, L., and Nation, I. S. P. (1993). Word families. Int. J. Lexicogr. 6, 253–279. doi: 10.1093/ijl/6.4.253
Cheng, J., and Matthews, J. (2018). The relationship between three measures of L2 vocabulary knowledge and L2 listening and reading. Lang. Test. 35, 3–25. doi: 10.1177/0265532216676851
Coxhead, A., and Walls, R. (2012). TED talks, vocabulary, and listening for EAP. TESOL ANZ J. 20, 55–65.
Dang, T., and Webb, S. (2014). The lexical profile of academic spoken English. Engl. Specif. Purp. 33, 66–76. doi: 10.1016/j.esp.2013.08.001
Dang, T. N. Y., and Webb, S. (2016). Evaluating lists of high-frequency words. ITL Int. J. App. Ling. 167, 132–158. doi: 10.1075/itl.167.2.02dan
Dang, T. N. Y., Webb, S., and Coxhead, A. (2020). Evaluating lists of high-frequency words: teachers’ and learners’ perspectives. Lang. Teach. Res. 136216882091118. doi: 10.1177/1362168820911189
Davies, M. (2012). Corpus of American soap operas. Available at: https://www.english-corpora.org/soap/ (Accessed January 26, 2022).
Davies, M. (2019a). The movie corpus. Available at: https://www.english-corpora.org/movies/ (Accessed January 26, 2022).
Davies, M. (2019b). The TV corpus. Available at: https://www.english-corpora.org/tv/ (Accessed January 26, 2022).
Davies, M. (2021). The TV and movies corpora: design, construction, and use. Int. J. Corpus Ling. 26, 10–37. doi: 10.1075/ijcl.00035.dav
Graham, S. (2006). Listening comprehension: The learners’ perspective. System 34, 165–182. doi: 10.1016/j.system.2005.11.001
Ha, T. H. (2021a). A Rasch-based validation of the Vietnamese version of the listening vocabulary levels test. Language testing. Asia 11, 1–19. doi: 10.1186/s40468-021-00132-7
Ha, T. H. (2021b). Exploring the relationships between various dimensions of receptive vocabulary knowledge and L2 listening and reading comprehension. Lang. Test. 11, 1–20. doi: 10.1186/s40468-021-00131-8
Harris, T. (2003). Listening with your eyes: the importance of speech-related gestures in the language classroom. Foreign Lang. Ann. 36, 180–187. doi: 10.1111/j.1944-9720.2003.tb01468.x
Heatley, A., Nation, I. S. P., and Coxhead, A. (2002). Range: A program for the analysis of vocabulary in texts. Available at: http://www.victoria.ac.nz/lals/about/staff/paul-nation (Accessed January 26, 2022).
Hsu, W. (2018). The most frequent BNC/COCA mid- and low-frequency word families in English-medium traditional Chinese medicine (TCM) textbooks. Engl. Specif. Purp. 51, 98–110. doi: 10.1016/j.esp.2018.04.001
Hu, M., and Nation, I. S. P. (2000). Unknown vocabulary density and reading comprehension. Read. For. Lang. 13, 403–430.
Klassen, K. (2021). Proper name theory and implications for second language reading. Lang. Teach. 1–7. doi: 10.1017/S026144482100015X
Kobeleva, P. (2012). Second language listening and unfamiliar proper names: comprehension barrier? RELC J. 43, 83–98. doi: 10.1177/0033688212440637
Lange, K., and Matthews, J. (2020). Exploring the relationships between L2 vocabulary knowledge, lexical segmentation, and L2 listening comprehension. Stu. Sec. Lang. Learn. Teach. 10, 723–749. doi: 10.14746/ssllt.2020.10.4.4
Love, R., Dembry, C., Hardie, A., Brezina, V., and McEnery, T. (2017). The spoken BNC2014 designing and building a spoken corpus of everyday conversations. Int. J. Corpus Ling. 22, 319–344. doi: 10.1075/ijcl.22.3.02lov
McLean, S., and Kramer, B. (2016). The development of a Japanese bilingual version of the new vocabulary levels test. VERB 5, 2–5.
McLean, S., Kramer, B., and Beglar, D. (2015). The creation and validation of a listening vocabulary levels test. Lang. Teach. Res. 19, 741–760. doi: 10.1177/1362168814567889
Nation, I. S. P. (2006). How large a vocabulary is needed to reading and listening? Can. Mod. Lang. Rev. 63, 59–82. doi: 10.3138/cmlr.63.1.59
Nation, I. S. P. (2013). Learning Vocabulary in another Language. 2nd Edn. Cambridge: Cambridge University Press.
Nation, I. S. P. (2017). The BNC/COCA level 6 words family lists (version 1.0.0) [data file]. Available at: http://www.victoria.ac.nz/lals/staff/paul-nation.aspx (Accessed January 26, 2022).
Nation, I. S. P. (2020). About the BNC/COCA headword lists. Available at: https://www.wgtn.ac.nz/lals/resources/paul-nations-resources/vocabulary-lists (Accessed January 26, 2022).
Nurmukhamedov, U. (2017). Lexical coverage of TED talks: implications for vocabulary instruction. TESOL J. 8, 768–790. doi: 10.1002/tesj.323
Nurmukhamedov, U., and Sharakhimov, S. (2021). Corpus-based vocabulary analysis of English podcasts. RELC J. 1:0033688220979315. doi: 10.1177/0033688220979315
Nurmukhamedov, U., and Webb, S. (2019). Lexical coverage and profiling. Lang. Teach. 52, 188–200. doi: 10.1017/S0261444819000028
Ozturk, M. (2016). Second language vocabulary growth at advanced level. Lang. Learn. J. 44, 6–16. doi: 10.1080/09571736.2012.708054
Schmitt, N., Cobb, T., Horst, M., and Schmitt, D. (2017). How much vocabulary is needed to use English? Replication of van Zeeland and Schmitt (2012), Nation (2006) and Cobb (2007). Lang. Teach. 50, 212–226. doi: 10.1017/S0261444815000075
Schmitt, N., Jiang, X., and Grabe, W. (2011). The percentage of words known in a text and reading comprehension. Mod. Lang. J. 95, 26–43. doi: 10.1111/j.1540-4781.2011.01146.x
Tegge, F. (2017). The lexical coverage of popular songs in English language teaching. System 67, 87–98. doi: 10.1016/j.system.2017.04.016
van Zeeland, H., and Schmitt, N. (2013). Lexical coverage in L1 and L2 listening comprehension: The same or different from reading comprehension? Appl. Linguis. 34, 457–479. doi: 10.1093/applin/ams074
Webb, S. A., and Chang, A. C.-S. (2012). Second language vocabulary growth. RELC J. 43, 113–126. doi: 10.1177/0033688212439367
Webb, S., and Paribakht, T. S. (2015). What is the relationship between the lexical profile of test items and performance on a standardized English proficiency test? Engl. Specif. Purp. 38, 34–43. doi: 10.1016/j.esp.2014.11.001
Webb, S., and Rodgers, M. P. H. (2009a). Vocabulary demands of television programs. Lang. Learn. 59, 335–366. doi: 10.1111/j.1467-9922.2009.00509.x
Webb, S., and Rodgers, M. P. H. (2009b). The lexical coverage of movies. Appl. Linguis. 30, 407–427. doi: 10.1093/applin/amp010
Webb, S., Sasao, Y., and Balance, O. (2017). The updated vocabulary levels test. ITL Int. J. Appl. Linguist. 168, 33–69. doi: 10.1075/itl.168.1.02web
Keywords: lexical coverage, BNC, COCA, TV programs, movies, soap operas
Citation: Ha HT (2022) Vocabulary Demands of Informal Spoken English Revisited: What Does It Take to Understand Movies, TV Programs, and Soap Operas? Front. Psychol. 13:831684. doi: 10.3389/fpsyg.2022.831684
Edited by:
Mila Vulchanova, Norwegian University of Science and Technology, NorwayReviewed by:
Rining Wei, Xi'an Jiaotong-Liverpool University, ChinaJoanna Kolak, University of Warsaw, Poland
Copyright © 2022 Ha. This is an open-access article distributed under the terms of the Creative Commons Attribution License (CC BY). The use, distribution or reproduction in other forums is permitted, provided the original author(s) and the copyright owner(s) are credited and that the original publication in this journal is cited, in accordance with accepted academic practice. No use, distribution or reproduction is permitted which does not comply with these terms.
*Correspondence: Hung Tan Ha, hatanhung1991@gmail.com, orcid.org/0000-0002-5901-7718