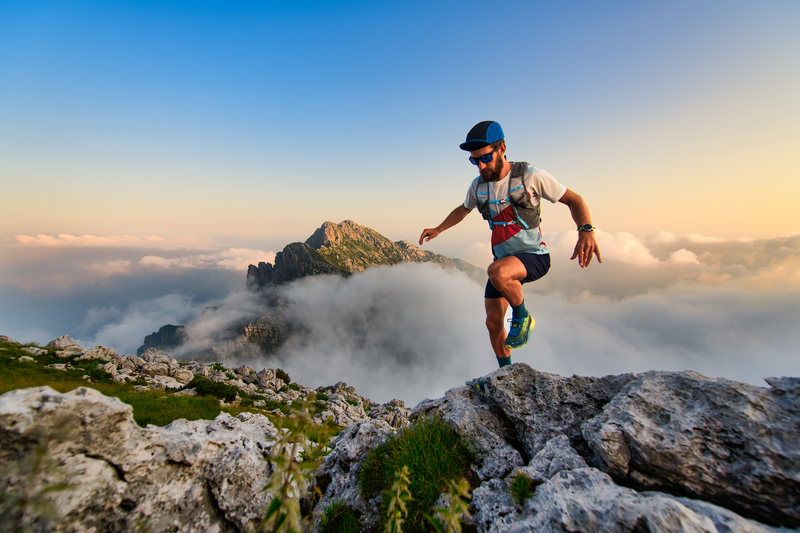
94% of researchers rate our articles as excellent or good
Learn more about the work of our research integrity team to safeguard the quality of each article we publish.
Find out more
ORIGINAL RESEARCH article
Front. Psychol. , 05 January 2023
Sec. Organizational Psychology
Volume 13 - 2022 | https://doi.org/10.3389/fpsyg.2022.1077614
Introduction: Industry–university–research cooperation innovation, which is often characterized by resource complementarity and the sharing technology, has become one of the most preferred innovation cooperation methods for enterprises. However, various problems still occur in the process of industry–university–research cooperations, such as poor innovation performance and difficulty in sustaining cooperation. Existing studies mostly focus on the macroscopic perspectives of geographic location, cooperation scale, concentration, and diversification of industry–university–research cooperation subjects, and fail to explore the microscopic behavioral mechanisms.
Methods: Therefore, this paper establishes the interactive behavior of industry–university–research subjects and defines its concepts and dimensions in an attempt to provide a mechanism for improving the cooperative innovation performance of industry–university–research from the micro-behavioral perspective. On the basis of theoretical analysis, this paper develops a model of the relationship between cooperative trust, cooperative communication, and cooperative innovation performance for interactive behavior, while exploring the mediating role of knowledge absorptive capacity. The model was validated by stepwise regression using data from 325 questionnaires.
Results: The paper found that cooperative trust and cooperative communication in the cooperative interactive behavior of industry–university–research positively contribute to the improvement of cooperative innovation performance. Knowledge absorptive capacity plays a partially mediating role between the interactive behaviors and cooperative innovation performance. More specifically, knowledge absorptive capacity partially mediates cooperative communication in cooperative innovation performance and completely mediates cooperative trust in cooperative innovation performance. The results are largely consistent with the results of the heterogeneity analysis of the sample.
Discussion: This paper not only explains why the cooperative innovation performance of industry–university–research is poor from the perspective of interactive behavior, but also enriches the research perspective of industry–university–research and provides theoretical support for enterprises to optimize the relationship between industry, university, and research institutes.
Strengthening industry–university–research cooperation, making good use of the innovative resources of universities, and promoting knowledge transfer and the transformation of achievements play an important role in accelerating the innovation–driven and high–quality development of China’s economy (Wang et al., 2018). With the introduction of China’s innovation–driven strategy, industry–university–research cooperation has made rapid progress. The enthusiasm of cooperation subjects has increased significantly, cooperative innovation has become increasingly active and the transformation of scientific and technological achievements has been deepened. Industry–university–research cooperation plays an important role in accelerating the innovation–driven and high–quality development of the economy. In recent years, an increasing number of enterprises have chosen to cooperate with universities and research institutes in an attempt to break the innovation chain more rapidly (Wang and Hu, 2022). It has been proven that industry–university–research cooperation is an effective means for enterprises to obtain complementary resources, create new knowledge and develop new technologies, and improve their technological innovation capabilities in the era of open innovation (Ma et al., 2018). According to the 2020 China Enterprise Innovation Capability Statistical Monitoring Report, the proportion of enterprises cooperating with industry, universities, and research institutes was 34.7%, i.e., enterprises conducting innovation cooperation. However, in the process of promoting industry–university–research cooperation, as a result of the information asymmetry of technology supply and demand, low trust in cooperative communication, and the concept of emphasizing results over process deviation, the practical problems of insufficient breadth, insufficient depth, and insufficient performance of industry–university–research cooperation exist. For many years, industry–university–research cooperation has been an important issue in the management of innovation academia (Qing and Qi, 2020; Li et al., 2022), and how to effectively improve the cooperative innovation performance of industry–university–research is an urgent problem to be solved (Cao et al., 2013; Yan et al., 2017; Liu et al., 2020).
The current research results can be summarized as follows. Firstly, focusing on the network structure of industry–university–research cooperation, it is demonstrated that network centrality, structural holes, network location, network density, and other network factors affect the cooperative innovation performance of industry–university–research (Li et al., 2020; Guo et al., 2022; Li and Jian, 2022). Secondly, focusing on the geographical location of industry–university–research subjects, it is shown that the objective geographical environment, including factors such as geographical proximity, geographical distance, and geographical concentration of industry–university–research subjects, affects the innovation performance of industry–university–research (Wang and Luan, 2019; Zhang and Shen, 2019; Zhang and Chen, 2022). Thirdly, focusing on the characteristics of the industry–university–research cooperative subjects themselves, it is indicated that the resource environment, including diversity, heterogeneity, matching, and complementarity of industry–university–research subjects, affects the innovation performance of industry–university–research (Li and Zhu, 2019; Zhang et al., 2021; Yang et al., 2022). Fourth, focusing on the external environment of industry–university–research, it is shown how factors such as the business environment, institutional development stage, market competition, financing environment, and government subsidies affect the innovation performance of industry–university–research (Zhang et al., 2019, 2021, 2022; Song et al., 2022). Fifth, focusing on enterprises’ own capabilities, it is pointed out that the soft environment of enterprises, which includes factors such as knowledge absorptive capacity, knowledge base, and learning capacity, affects the innovation performance of industry–university–research (Lu and Ye, 2017; Li et al., 2020; Zhang et al., 2022). The above research results are relatively rich, and provide explanations for the influence of the macromarket network environment, geographical environment, and resource environment on the cooperative innovation performance of industry–university–research. This also led the authors to ask whether, in addition to the impact of the macroenvironment on the performance of industry–university–research cooperation innovation, whether the microbehavior of the cooperation subject also has an impact on cooperative innovation performance?
On the basis of the shortcomings of existing research, this paper begins to explore the micro-impact mechanisms of the cooperative innovation performance of industry–university–research cooperation. Various scholars have proposed that the role of industry–university–research in encouraging innovation performance in China is not obvious, mainly because the internal subjects of industry–university–research lack long-term and deep interactions (Chen et al., 2009). Through interaction, universities, research institutes, and enterprises form a relationship of interdependence, interaction, mutual promotion, mutual penetration, and mutual restriction, which is the basis of effective cooperation between industry, university, and research (Li and Ye, 2011; Li and Liu, 2013). However, this kind of cooperation is only a static relationship, and its cooperative role and effectiveness must be realized through the interaction between the subjects (Li and Liu, 2013). Li Chenglong first proposed the concept of interactive behavior in industry–university–research cooperation, but unfortunately did not provide a detailed introduction to or explore the essence of interactive behavior. In 2016, Hu Junyan and others divided industry–university–research interaction into low interaction and high interaction, and proposed that interaction intensity has a positive impact on performance (Hu et al., 2016). In addition, the essence of the goal of industry–university–research cooperation is to realize the transfer of knowledge. Industry–university–research cooperation has built channels for knowledge transfer (Jin et al., 2015). However, in actual industry–university–research cooperation, the mismatch between the capabilities of enterprises and universities leads to a structural dilemma, which makes it impossible to effectively internalize external resources and knowledge (Wang et al., 2022), resulting in poor knowledge transfer. The efficiency of knowledge transfer largely depends on the knowledge absorptive capacity of enterprises (Ye and Chen, 2022). Knowledge absorptive capacity not only determines the breadth and depth of knowledge flow, but also promotes the process of knowledge flow (Rupietta and Backes-Gellner, 2017).
At present, the theoretical explanation of the impact of micro behavior on cooperative innovation performance is seriously insufficient. The logical relationship between micro behavior and enterprise knowledge absorptive capacity is relatively unexplored. Wu et al. (2022) pointed out that the trust relationship of industry–university–research cooperation can accelerate the flow of knowledge (Wu et al., 2022). When cooperative organizations have a degree of trust, knowledge is more likely to be transferred. However, knowledge transfer does not mean the improvement of cooperative innovation performance, but increases the possibility of this. Therefore, on the basis of transaction cost theory and a resource-based view, this paper attempts to explore two points. Firstly, we assess interactive behavior of industry–university–research cooperation subjects, and how to affect cooperative innovation performance. Secondly, we explore whether the knowledge absorption capacity of enterprises can improve the cooperative innovation performance of industry–university–research, and what the logical relationship between interactive behavior is composed of. In this paper, our research combined with existing achievements provides a breakthrough from the microperspective in terms of exploring the impact of the behavior characteristics of the cooperation subject on the cooperative performance of industry–university–research, which belongs to the category of organizational behavior.
The academic community has not yet reached a consensus on the conceptual definition and dimensional division of the interaction behavior of industry–university–research subjects. More research is conducted based on the interaction process, and it is believed that the interaction behavior is mainly expressed through the interaction process, such as through trust, communication, conflict, cohesion, coordination, and learning in the interaction process (Tison and Poirier, 2021; Yang et al., 2022). One of the more representative definitions is Marks’ definition. He thinks that interactions between members and between members and outside subjects constitute interaction processes. Communication and conflict resolution, and communication and trust in interpersonal relationships are frequent interaction activities (Hoegl and Gemuenden, 2001). Li and Liu (2013) defined industry–university–research interaction behavior as the manifestation of the interaction between partners. It is the cognitive, verbal, and behavioral activities that maintain contact and connectivity between partners. It is more reflected in the interaction of various partners to complete the task objectives, emphasizing behavior and process (Li and Liu, 2013). Hu et al. (2016) believe that industry–university–research cooperation is actually a kind of cooperation between heterogeneous organizations, and the process of interaction between cooperative subjects includes knowledge spillover, information exchange, trust, and cohesion.
In 1987, Hackman classified interaction behaviors into two categories: affective interaction behaviors and task-based interaction behaviors. Affective interaction behaviors are interpersonal processes that occur during collaboration, including trust, cohesion, and conflict reduction. Task interaction behaviors are those directly related to the R&D innovation task and include knowledge sharing, communication and balanced member contributions (Hackman, 1987). Judging from the essence of industry–university–research cooperation, industry–university–research comprises realizing the knowledge transfer (Jin et al., 2015). However, as universities and enterprises are in different knowledge positions, there is a conflict in the consistency of cooperative innovation goals, a situation of nonfinite rationality, a high degree of uncertainty, and information asymmetry, which leads an increase in the risk of industry–university–research (Li et al., 2015). The conflict can be effectively managed if the parties can have complete trust in one another throughout the interaction process, which can enhance transaction efficiency and innovation performance and lower transaction costs (Veugelers and Cassiman, 2005). Domestic scholars have also paid attention to the communication behavior in the interaction process. When the cooperative organization has a certain degree of trust, it can strengthen the willingness of the subject to share knowledge. At this time, knowledge is more easily transferred and it is easier to create a good atmosphere for innovation (Wang et al., 2018) In addition, the communication behavior in the process of interaction has also attracted the attention of domestic scholars. Communication is the basis of interaction between universities, research institutes, and enterprises, facilitating the effective transfer of innovative ideas in the collaborative process and making these ideas subject to evaluation by other partners, which, in turn, has a positive impact on innovation performance (Li and Liu, 2013). Therefore, better cooperative communication and integration arrangements are key to enhancing collaborative performance (Bai and Chen, 2022). Judging from the interaction process of industry–university–research cooperation, it also is a game. The cooperative game is similar to the prisoner’s dilemma. To increase the probability of the emergence of the Pareto-optimal Nash equilibrium, the same coordination game can be played repeatedly (Duan et al., 2022). In contrast, the emergence of a Pareto-optimal Nash equilibrium can only be achieved by information communication in the game.
On the basis of the above analysis, it can be judged that trust and communication in the process of industry–university–research cooperation are two very important factors affecting the cooperative innovation performance. Industry–university–research interaction behavior refers to the process of cognitive, linguistic, and behavioral activities that are carried out in order to accomplish the goal of cooperation and to maintain mutual contact, and mutual assistance between universities, research institutes, and enterprises. It is also a manifestation of interaction, emphasizing the behavior and process between subjects. The realization of this process relies on trust and communication between subjects. Therefore, industry–university–research trust and communication are the main microfactors that were revealed in this study. In order to fit the context of industry–university–research cooperation and to distinguish it from trust and communication in human behavior, this study denotes trust and communication in the interaction behavior of industry–university–research cooperation as cooperative trust and cooperative communication, respectively.
Cooperative trust means that, in order to achieve the expected cooperative goals, enterprises believe universities and research institutes are capable and willing to fulfill their commitments as expected, and are willing to take the risks associated with opportunistic behaviors and others. That is, it is believed that universities and research institutes will provide true and useful explicit and implicit knowledge as required, and will not take advantage of a enterprises’ weaknesses for profits (Li et al., 2015). According to transaction cost theory, although industry–university–research cooperation has the advantages of reducing R&D uncertainty, shortening R&D time costs, and optimizing resource allocation to reduce transaction costs (Kafouros et al., 2015; Wang et al., 2021; Cui and Li, 2022), there are also certain problems of rising transaction costs in industry–university–research cooperation, such as the cost of finding partners, the cost of cooperative communication, the cost of digesting the asymmetry between theoretical research and actual needs, and the potential risk cost of knowledge leakage (Wang and Hu, 2022). The increase in the above costs will reduce some enterprises’ willingness to cooperate, or reduce the input of cooperation. The reason for this is that enterprises do not have enough trust in universities’ technical ability and emotion. This virtually increases the cost of evaluation. Li pointed out that the trust mechanism is a prerequisite and foundation for innovation in industry–university–research cooperation, and it is a soft governance mechanism that can make up for the shortcomings of the formal management mechanisms (Li et al., 2015). Industry–university–research cooperative trust not only affects the psychological process of cooperative innovation subjects, reduces the expectation of the risks faced by cooperative innovation, and improves the willingness of participants to cooperate in innovation and the level of participation in cooperative innovation, but also directly and indirectly affects people’s attitudes, expectations, behaviors, and performance (Dirks, 1999; Dirks and Ferrin, 2001; Xu and Gao, 2006). Secondly, the mutuality between the subjects of industry–university–research cooperation will increase information sharing, teamwork, and other behaviors, improve information exchange, and thus improve performance (Zhang et al., 2020). This influence can be explained from two perspectives. From the perspective of knowledge base, the cooperative trust between subjects can effectively promote knowledge sharing, knowledge transfer, and learning innovation among members (Li and Liu, 2014; De Zubielqui et al., 2019; Wang et al., 2021). From the perspective of knowledge transfer, industry–university–research cooperative trust can reduce the uncertainty of knowledge exchange, inactivity, increase the frequency and success rate of knowledge transfer, improve the outcome of knowledge exchange, and thus improve cooperative performance (Hardwick et al., 2013). Takagi, D had verified that the spatial Durbin model showed that spatially weighted neighborhood trust was positively associated with behaviors (Takagi and Shimada, 2019). On the basis of the above analysis, the following hypothesis is proposed:
Hypothesis 1 (H1). Cooperative trust in industry–university–research cooperation is positively correlated with cooperative innovation performance.
Industry–university–research cooperative communication refers to the communication process of obtaining, transferring, and understanding information between the members of the cooperation subjects in order to accomplish the goal of cooperation, which can be divided into formal communication, such as seminar meetings, on-site guidance, and appropriate training and informal language communication according to the organizational form of cooperation communication. The essential goal of industry–university–research cooperation is to realize the knowledge transfer. Knowledge transfer is actually a communication process between the knowledge transmitter and the knowledge receiver (Li et al., 2022). The effect of cooperative communication on cooperative innovation performance mainly depends on three mechanisms: Firstly, cooperative communication reduces information asymmetry and cooperation risks; secondly, cooperative communication promotes the flow and transfer of knowledge; thirdly, cooperative communication optimizes resource the allocation of resource. In industry–university–research cooperation, there are asymmetries between technology supply and technology demand, and between theoretical research and practical needs. In addition, there are information asymmetries such as market changes and untimely changes in the technical parameters in the actual cooperation process, which increase the cooperation costs and cooperation risks of enterprises. Practice has proved that intentional communication can maintain the consistency of psychological state between subjects and form cooperative communication (Vasil et al., 2020), and strengthening cooperative communication can greatly reduce information asymmetry and cooperative cultural conflict (Kopelman et al., 2016). In addition, the essence of the goal of industry–university–research cooperation is to realize knowledge transfer (Li et al., 2022). Knowledge transfer is defined as a communication process between the knowledge transmitter and the knowledge receiver (Li et al., 2022). Cooperative communication between industry, university, and research institutes can broaden the breadth and depth of knowledge transfer channels, reduce the barriers to knowledge transfer and realize knowledge transfer more efficiently (Dong et al., 2005). However, universities and research institutes are in the “knowledge highland,” while enterprises are in the “knowledge lowland,” and there is a certain “gap” in knowledge. Through the cooperation of industry, university, and research, the knowledge “gap” can be filled and resources can be realized. However, industry–university–research cooperation is formed via a static relationship (Li and Liu, 2013). Resource complementation must be realized through communication; otherwise, cooperation is only a formality. Finally, as verified using game theory, industry–university–research cooperation can achieve better resource allocation (Huyck et al., 1990; Yang et al., 2008). Huyck et al. (1990) suggested that the game between industry–university–research cooperation is consistent with the prisoner’s dilemma, which can be achieved by repeatedly playing the same coordination game in order to increase the probability of Pareto-optimal Nash equilibrium. Suppose that, in the process of industry–university–research cooperation, the university dominant strategy benefits EU1, and the enterprise dominant strategy benefits EC1. Thus in the absence of cooperative communication and incomplete information, the total benefit is E1 = EU1 + EC1; however judging from cooperative economics, both parties cooperate and the benefit after information sharing is E, as the benefit of optimal strategy is E > E1, so the efficiency of cooperative communication is higher. But, the quality of communication will also affect performance (Gonzalez-Roma and Hernandez, 2014; Valls et al., 2016).On the basis of the above analysis, the following hypothesis is proposed:
Hypothesis 2 (H2). Cooperative communication in industry–university–research cooperation is positively correlated with cooperative innovation performance.
Cohen and other scholars first proposed the concept of knowledge absorptive capacity. They believed that knowledge absorptive capacity includes the ability of enterprises to identify and acquire external knowledge, digest it and apply it within the enterprise (Cohen and Levinthal, 1990). On the basis of Cohen’s definition, many other scholars divide knowledge absorptive capacity into four segments: knowledge acquisition, knowledge assimilation, knowledge transformation, and knowledge exploitation (Schweisfurth and Raasch, 2018; Meng et al., 2021). Among them, knowledge acquisition and knowledge assimilation are defined as potential absorptive capacity, and knowledge transformation and knowledge exploitation are defined as real absorptive capacity. In the industry–university–research cooperation relationship, parties having a high degree of trust in each other promote enterprises to establish positive behavioral expectations, making them more willing to take risks and actively share information (Norman, 2004). This helps to improve their own knowledge absorptive capacity. First of all, cooperative trust in industry–university–research cooperation means both parties do not need to spend more time and effort in establishing monitoring mechanisms and bargaining, saving time costs that can be spent on knowledge acquisition. Motivated by cooperative trust, universities and research institutes are more willing to share knowledge and ideas to promote knowledge acquisition, and enterprises are more willing to share valuable and trustworthy information, simplifying the process of knowledge acquisition, which, in turn, helps to improve the enterprise’ knowledge acquisition capacity (Li et al., 2015). Secondly, cooperative trust can increase the frequency of communication and interaction, increasing communication flow. It enables both sides to have more opportunities to discuss and communicate in depth about the difficulties existing in the knowledge interaction, and transfer and express the knowledge in an easily understandable form. This is conducive cooperative enterprises improving their knowledge assimilation capacity and knowledge absorption capacity the cooperative enterprises (He et al., 2021). However, Davenport and Prusak argue that the idea of “not invented here” hinders the application of externally acquired knowledge. Therefore, organizational trust is needed when using external knowledge. They also suggested that organizational trust would be more conducive to knowledge absorption and exploitation (Davenport and Prusak, 2000). Therefore, cooperative trust in the industry–university–research partnership is a non-institutional arrangement through which enterprises encourage the use of external knowledge, which helps to improve the enterprises’ knowledge absorptive capacity. On the basis of the above analysis, the following hypothesis is proposed:
Hypothesis 3 (H3). Cooperation trust in industry–university–research cooperation is positively correlated with knowledge absorptive capacity.
Cooperative communication in university–industry–research is the basis for knowledge acquisition, knowledge assimilation, knowledge transformation, and knowledge exploitation in university–industry–research cooperation (Xie et al., 2018). Industry–university–research cooperation is also a platform for building knowledge exchange and learning. Universities and research institutes can be seen as knowledge exporters and enterprises as knowledge receivers. Thus, in the process of exporting and receiving, continuous explanation and communication can enable enterprises to understand and master knowledge better and faster. In turn, it will promote their knowledge absorptive capacity. From the perspective of knowledge learning theory, cooperative communication promotes the realization of knowledge absorptive capacity mainly through the following means. Firstly, cooperative communication simplifies the process and practice of knowledge screening, which can save knowledge acquisition costs; secondly, cooperative communication can explain and illustrate explicit knowledge, helping enterprises to understand, digest, and absorb knowledge; thirdly, cooperative communication can also produce knowledge spillover effects, helping enterprise employees to establish the correct learning concept, learning methods, and approaches, and improve their knowledge utilization capacity. The above views have been supported by a number of scholars. Xie et al. (2018) and others use exploratory case analyses to demonstrate that the establishment of communication and exchange mechanism is inseparable from any stage of industry–university–research cooperation, and through communication and exchange, the ability of enterprises to acquire, digest, integrate and, utilize knowledge is improved. However, in addition to sharing explicit knowledge between the subjects of industry–university–research cooperation, there is also a larger sharing of tacit knowledge, which is not easily encoded, and the knowledge sender and receiver need to share tacit knowledge through close interactive communication (Qiu and Haugland, 2019), which improves the knowledge reception ability of members. Finally, industry–university–research cooperation communication reduces information asymmetry and cooperation risks, making cooperation members more willing to cooperate and take the initiative to improve their knowledge absorptive capacity. On the basis of the above analysis, the following hypothesis is proposed:
Hypothesis 4 (H4). Cooperative communication in industry–university–research cooperation is positively correlated with knowledge absorptive capacity.
The relationship between knowledge absorptive capacity and innovation performance is very well established and it is believed that knowledge absorptive capacity has an important positive contribution to innovation performance (Casanueva et al., 2013; Li and Zhang, 2020; Li and Zhu, 2021). Knowledge absorptive capacity can promote knowledge transfer, which, in turn, has an important positive effect on innovation performance (Casanueva et al., 2013; Li and Zhang, 2020; Li and Zhu, 2021), That is, as the knowledge absorptive capacity of enterprises increases, the innovation performance also increases (Zhou, 2022). The effect is manifested in several ways. On the one hand, the stronger the potential knowledge absorptive capacity, the more effective a enterprise is at identifying the new knowledge needed and at absorbing and utilizing it (Braojos et al., 2020). In contrast, actual absorptive capacity as an exploitative learning processes enables the translation and exploitation of absorbed knowledge (Braojos et al., 2020; Miroshnychenko et al., 2021). On the other hand, enterprises with a strong knowledge absorptive capacity have good internal knowledge digestion and reorganization mechanisms, which can increase the speed and frequency of innovation (Meng et al., 2021). Moreover, enterprises with strong knowledge absorptive capacity are better able to benefit from the corresponding knowledge transfer process (Zhao et al., 2019). In studying the relationship between knowledge absorptive capacity and enterprise innovation, Gao also found that knowledge absorptive capacity has a significant positive impact on enterprise innovation performance and that enterprises exhibit innovation inertia due to knowledge absorption (Gao and Zhang, 2019).
The above analysis focuses on the process through which knowledge absorptive capacity can promote knowledge acquisition, knowledge assimilation, knowledge transformation, and knowledge exploitation. The interactive behavior of the cooperative subjects of industry–university–research cooperation increases the sharing and exchange of knowledge and provides channels and possibilities for knowledge flow. However, the knowledge acquired by enterprises from the outside cannot be directly transformed into the enterprise’s innovation performance. External knowledge must be absorbed and digested before it can be transformed into actual output (Cohen and Levinthal, 1990). Knowledge absorptive capability, as a dynamic capability, not only helps enterprises to identify knowledge, but also promotes the knowledge absorption, knowledge assimilation, and knowledge transformation. It plays the role of a “connector” (Zhang et al., 2022). It has been shown that knowledge absorptive capacity plays an important mediating role in using external knowledge to improve the enterprise’s innovation performance (Ye and Chen, 2022; Zhang et al., 2022). The above analysis table shows that the interactive behavior of industry–university–research cooperation subjects helps to improve enterprise’s knowledge absorptive capacity, and knowledge absorptive capacity promotes enterprise’s innovation performance. Therefore, the interactive behavior of industry–university–research cooperation can promote cooperative innovation performance by influencing knowledge absorptive capacity. On the basis of the above analysis, the following hypothesis is proposed:
Hypothesis 5 (H5). Knowledge absorptive capacity mediates the relationship between interactive behavior and cooperative innovation performance.
The above analysis led to the theoretical research model, as shown in Figure 1.
Figure 1. Theoretical model. [+] plus sign indicates positive relationships, and [→] solid line indicates direct relationships.
All the variables covered in this study were obtained by means of a questionnaire. The items for the constructs were assessed using a five-point Likert scale, ranging from 1 = “strongly disagree” to 5 = “strongly agree.”
Li and Liu (2013) divided the trust in industry–university–research cooperation into three dimensions: computational trust, cognitive trust, and identity trust, and developed 12 measurement indicators based on previous research results. Unlike the study by Li, this study mainly focuses on technical trust and emotional trust. Technical trust refers to the belief that universities and research institutes are capable of overcoming technical problems. Emotional trust refers to the belief that universities and research institutes are willing to help solve technical problems and are sincere in their cooperation. On the basis of the above concepts, combined with the need for cooperative trust, and taking into account the studies of Nguyen, Luu and Trong, Mc Allister, and others, cooperative trust was measured using two constructs and six items (e.g., “enterprises believe in the ability of cooperative universities and their preparation for work” and “professional knowledge of cooperative universities can help enterprises solve practical problems”) (Trong Hai et al., 2014).
The Cooperative Communication Scale was referenced from Fan’s study (Fan et al., 2010). Combined with the research content of this paper, four professional doctors were invited to discuss and initially determine the items. After a small-scale preinvestigation, five items were identified (e.g., “the partners are able to have timely discussions on issues in the study”).
Knowledge Absorptive Capacity (KAC). This paper continues to classify knowledge absorptive capacity into two constructs according to four aspects of knowledge acquisition, assimilation, transformation, and exploitation. They are denoted potential knowledge absorptive capacity and real knowledge absorptive capacity. On the basis of the studies of various scholars (Li and Zhang, 2020; Linh Nguyen et al., 2022), the research team discussed the interviews and summaries from the research leaders of four enterprises in Zhejiang. After the prestudy, two constructs and 11 items were finally identified (e.g., “The enterprise is able to quickly determine the value and usefulness of new external knowledge to existing knowledge” and “The enterprise has the ability to organize and use new knowledge that has been acquired”).
Following the work of foreign scholars (Wu et al., 2017; Sun et al., 2019), combined with the study results of Fan et al. (2010), and considering the connotation of industry–university cooperative innovation performance in this paper, we finally decided to measure cooperative innovation performance using two constructs and six items. The two constructs are learning growth performance and innovation task performance (e.g., “enterprises can master certain knowledge about R & D innovation through industry–university–research cooperation” and “The project achieved the expected technological innovation requirements”).
Referring to the studies of Chen et al. (2020), Li et al. (2020), Zhang et al. (2022) and other scholars, enterprise age (Age), enterprise size (Size), and industry–university–research experience (Exp) were selected as control variables (Chen et al., 2020; Li and Zhang, 2020; Zhang and Chen, 2022). This paper argues that enterprises that have been in business for a long time are more sensitive to market information, which facilitates the accumulation of innovation resources and a priori knowledge, and therefore enhances their innovation capacity. Larger enterprises have the strength and capital to carry out corporate management and engage in product innovation activities, but there is also a certain degree of rigidity in enterprises of this size, which is not conducive to innovation. Enterprises’ willingness to innovate and innovative behavior are also closely related to their past experience of cooperation. The value of 1 was assigned to denote experience of industry–university–research, while 0 was assigned to denote the absence of such experience.
For the survey, we chose Zhejiang Province of China for the following reasons: (1) China attaches great importance to industry–university–research cooperation, and has issued a number reports to this end; (2) In 2020, the number of Chinese R&D institutions was 6,682; (3) the industrial R&D expenditure of above-scale industries in Zhejiang Province reached CNY13,988,988 million, ranking third in China; (4) new product development expenditure for industrial enterprises above the scale reached CNY1,762,995,000, ranking third in China; (5) Zhejiang Province has 109 higher education institutions, and 105,887 scientific and technological personnel, and cooperative supply resources are abundant; (6) in 2021, there were 49,876 research and development projects in the field of natural science in higher education institutions in Zhejiang Province, which is a solid foundation for cooperation; and (7) The authors all work in Zhejiang universities and are in contact with the local Science Technology Department, which was highly convenient.
In order to gain a deeper understanding of the relationship between the interactive behavior of industry–university–research cooperation and the cooperative innovation performance, this study selected three enterprises that we were in contact with for interviews and the case rooting analysis before the formal questionnaire was administered. Then, the questionnaire was designed and iterated after considering the relevant literature, the opinions of experts, and the opinions of the responsible individuals in the enterprises concerned. On the basis of a small-scale preinvestigation of 50 participants from enterprises in the innovation and entrepreneurship training course hosted by the Zhejiang Education Development Centre in 2021, the scale was revised and refined to produce the final questionnaire.
The formal survey was mainly conducted with the help of the platform of the Zhejiang-China Development Research Institute. Through various local cooperation projects, especially with the local Science Technology Department, we requested cooperation of relevant individuals from this department, to conduct the research. The collection procedures were as follows: Firstly, we requested the support of the leaders of the Science Technology Department, and explained the purpose of the survey, our methodology, and things of note to these individuals. Secondly, we sent the electronic questionnaire created by the team to the leaders. Thirdly, the leaders gave us the contact details of the leaders. We contacted the individuals and explained the purpose of the survey and the requirements for filling it out. Fourthly, the questionnaire was sent to the leaders, who contacted the research leaders regarding filling it out. This survey took nearly 2 months in total. A total of 400 questionnaires were distributed and 325 valid questionnaires were returned, with an effective rate of 81.25%. The sample was distributed in various regions of Zhejiang Province. We consider the sample valid. The statistics of the surveyed sample enterprises are shown in Table 1.
As for the common method deviation, on the one hand, an anonymous questionnaire and other methods were used to control in advance. On the other hand, the Harman one-way test was used to test. The results showed that the cumulative variance contribution rate of the first factor was 32.801 < 40%, indicating that there was no homologous deviation in the study.
In this paper, the orthogonal maximum variance method of the exploratory factor analysis was used to conduct the factor composition analysis and then to explore the structural validity of the questionnaire. The internal consistency coefficient Cronbach’s Alpha, Kaiser–Meyer–Olkin (KMO) sample measure, and Bartlett’s sphere test were used to test the reliability of the questionnaire. The internal consistency coefficient Cronbach’s Alpha analysis was conducted using the SPSS 23.0 software. As shown in Table 2, all variables were tested.
In this study, we conducted the confirmatory factor analysis using Amos 23.0 to test the discriminant validity of cooperative trust, cooperative communication, knowledge absorptive capacity, and cooperative innovation performance. As shown in Table 3, each fitting index of the four-factor model met the critical standard, and the fitting effect of the model was significantly better than that of other models. The analysis results show that the discrimination validity among the four variables was high, and the fitting effect of the four-factor model was good.
The results of the correlation analysis showed the correlation coefficient, mean, and variance of each variable. As shown in Table 4, cooperative trust had a significant positive correlation with knowledge absorptive capacity (r = 0.465, p < 0.01), and cooperative innovation performance (r = 0.369, p < 0.01). Cooperative communication was positively related to knowledge absorptive capacity (r = 0.394, p < 0.050), and cooperative innovation performance (r = 0.433, p < 0.01). Finally, there was a significant positive correlation between knowledge absorptive capacity and cooperative innovation performance (r = 0.545, p < 0.01).
Table 5 shows the results of the hypothesis test. Firstly, according to the results of model 1 and model 2, after controlling for age, size, and experience, the regression coefficient of cooperative trust was 0.379 (β = 0.379, p < 0.050) and the regression coefficient of cooperative communication was 0.120 (β = 0.120, p < 0.050). That is, if cooperative trust and cooperative communication are high, the knowledge absorptive capacity is better. Thus, the hypotheses H3 and H4 are verified. Secondly, according to model 3 and model 4, cooperative trust and cooperative communication (β = 0.200, p < 0.050; β = 0.370, p < 0.001) have a significant positive effect on cooperative innovation performance. Thus, the hypotheses H1 and H2 are verified. Using model 4 and model 6, cooperative trust, cooperative communication, and knowledge absorptive capacity were simultaneously entered into the regression. The results show that cooperative communication and knowledge absorptive capacity (β = 0.301, p < 0.001; β = 0.578, p < 0.001) have a significant positive effect on cooperative innovation performance. Whereas, cooperative trust (β = −0.019, p > 0.05) has no significant positive effect on cooperative innovation performance. Therefore, it can be concluded that knowledge absorptive capacity plays a partial intermediary role between interactive behavior and cooperative innovation performance. Thus, hypothesis H5 is verified.
In this study, the bootstrap method was used to verify the mediation. The data show that the indirect effect of cooperative trust on cooperative innovation performance through knowledge absorptive capacity was 0.2902, with a BootLLCI of 0.2098 and a BootULCI of 0.3812, and the indirect effect of cooperative communication on cooperative innovation performance through knowledge absorptive capacity was 0. 1933, with a BootLLCI of 0.1293 and a BootULCI of 0.2666. The range does not include 0. Therefore, knowledge absorptive capacity plays a significant mediating role in the relationship between interactive behavior and cooperative innovation performance.
Firstly, in order to investigate the impact of the interactive behavior of industry–university–research cooperation on the cooperative innovation performance of enterprises of different scales, we took the number of cooperative enterprises as a proxy variable for the size of the enterprises, and divided the enterprises with 200 persons or less in the whole sample into “enterprise size_low” and those with more than 200 persons are divided into “enterprise size_high” for regression. The results are shown in Table 6.
By comparing the regression coefficients in the table, we found that knowledge absorptive capacity moderated the effect of cooperative innovation performance in both groups. In the “enterprise size_high” group, knowledge absorptive capacity played a complete intermediary role between cooperative trust and cooperative innovation performance, and a partial intermediary role between cooperative and cooperative innovation performance. In the “enterprise size_low” group, knowledge absorptive capacity played a partial intermediary role between cooperative communication and cooperative innovation performance. In this group, the relationship between trust and innovation performance was not tested (Table 6).
Secondly, according to the age index from small to large, this study divides the enterprises into two groups. Enterprises aged under 10 years (and including 10 years) are named “low-group” and enterprises aged over 10 years are named “high-group.” By comparing the regression coefficients of the two groups, we found that knowledge absorptive capacity played a complete intermediary role between cooperative trust and cooperative innovation performance in the two groups, and a partial intermediary role between cooperative communication and cooperative innovation performance in the two groups. However, the relationship between cooperative trust and cooperative innovation performance was not verified in the “high-group” (Table 7).
Thirdly, this study investigated whether the previous experience of industry–university–research cooperation affects the cooperative innovation performance. In this study, the enterprises were divided into two types of samples, the “inexperienced group” and the “experienced group.” As shown in Table 8, enterprises with previous experience in cooperation considered cooperative trust to be important for knowledge absorption capacity and cooperative innovation performance. The lack of cooperative trust made them more concerned about “hitchhiking” on research and the risk of intellectual property leakage. For enterprises with no prior experience, cooperative trust had a relatively small impact on cooperative innovation performance, while cooperative communication had a relatively large impact on cooperative innovation performance.
According to the above analysis, the hypothesis is basically supported, as shown in Figure 2.
The study examines the relationship between interactive behavior (cooperative trust and cooperative communication), knowledge absorptive capacity, and cooperative innovation performance based on the perspective of knowledge learning theory. Using enterprises from Zhejiang Province as research subjects, the study reveals that interactive behaviors promote cooperative innovation performance, and that knowledge absorptive capacity plays a mediating role.
Firstly, the interactive behavior of industry–university–research cooperation has a positive impact on cooperative innovation performance. The results of this study are consistent with those of existing studies (Li and Liu, 2013). On basis of transaction cost theory, a higher the degree of cooperative trust in the cooperation of industry–university–research, reduces the explicit and implicit cost assessment from a psychological perspective. In turn, it can increase their willingness to cooperate and the initiative of knowledge sharing (Dirks and Ferrin (2001). On the other hand, in industry–university–research cooperation, cooperative trust not only strengthens mutual willingness to share knowledge and accelerate knowledge flow, long-term cooperative trust can even form a culture of cooperation, reducing knowledge protection and opportunistic behavior and promoting effective knowledge flow (Wu et al., 2022). It has been demonstrated that effective knowledge transfer must be achieved through communication. Effective cooperative communication helps to increase the breadth and depth of knowledge transfer channels and ensures smooth knowledge transfer (Dong et al., 2005). The results of this study also support this point of view.
Secondly, interactive behavior promotes the improvement of knowledge absorptive capacity. The trust mechanism is an important part of cooperation. Trust can increase the initiative of knowledge sharing and improve exploratory and exploitative learning capabilities (Wang and Hu, 2022). Cooperative communication can lead to more knowledge spillover effects. This all offers the potential to increase the knowledge absorptive capacity of enterprises (Pinjani and Palvia, 2013; Zhao et al., 2019; Braojos et al., 2020). The findings of the study also further validate the theoretical hypothesis.
Thirdly, knowledge absorptive capacity plays a partially mediating role between the interactive behavior and cooperative innovation performance. It was shown that knowledge absorptive capacity partially mediated the relationship between cooperative communication and cooperative innovation performance and completely mediated the relationship between cooperative trust on cooperative innovation performance. Ye and Chen (2022) also verified the existence of a partially mediating role of knowledge absorptive capacity and innovation performance (Ye and Chen, 2022). This study demonstrates that knowledge absorptive capacity plays a completely and partially mediating role between interactive behavior and cooperative innovation performance. A possible explanation for this is that if the industry–university–research cooperation remains on a trust basis and there is no activity, it is unlikely that performance is generated, i.e., there is no action, although there is the possibility of knowledge sharing. Therefore, knowledge absorptive capacity is needed to externalize the flow of knowledge, as cooperative communication itself is a dynamic communication. As long as it is not a “closed” state, employees will always consider transforming knowledge and improving performance. Therefore, it partially mediates cooperative communication and cooperative innovation performance (Valentim et al., 2016).
Fourthly, the heterogeneity analysis also produced interesting conclusions. The mediating role of knowledge absorptive capacity is significant in larger enterprises, but in the smaller enterprises, the mediating role is only significant in the relationship between cooperative communication and cooperative innovation performance. It can be inferred that the larger enterprises pay more attention to the growth of their own R&D capacity, and pay more attention to the improvement of their knowledge absorptive capacity, especially their potential knowledge absorption capacity. Their sense of “self-improvement” is more prominent. They pay particular attention to exchange, learning, and growth. This finding further supports the research of many scholars who postulate that enterprise size is positively related to learning capacity (Cao et al., 2013; Lu and Ye, 2017). For relatively young enterprises, cooperative communication and cooperative trust promote knowledge absorptive capacity, whereas cooperative trust in older enterprises does not promote knowledge absorptive capacity. This may be due to the fact that older enterprises develop a sense of territoriality, cognitive entrenchment, or even a “covering effect,” resulting in distrust or even rejection of foreign technology (Zhao and Ma, 2021). Young enterprises are not “leaders,” so they must strengthen their own viability. They must strive to improve their knowledge absorption capacity. In addition, enterprises with previous experience in industry–university–research cooperation are more likely to recognize cooperative trust. A possible explanation for this is that university–industry–research cooperation increases transaction costs, which is of concern to enterprises with previous experience, but enterprises without previous cooperation experience have a low perception of the risk of a lack of cooperative trust. This also suggests that any entities engaged in industry–university–research cooperation must weigh the risks and benefits, and must avoid conflicts of interest; otherwise, it will affect the subsequent industry–university–research cooperative behavior (Norman, 2004; Xu and Gao, 2006).
Firstly, this study breaks the macroperspective of existing research regarding the effects of cooperative innovation performance, and extends to the exploration of the microperspective. The study proposes the interactive behavior of industry–university–research from a micro perspective, and defines and analyzes the dimensions of the interactive behavior. It echoes the research of certain other scholars, such as Li and Ye (2011), Li and Liu (2013), and Liu (2013), and also broadens our perspectives regarding how to improve the cooperative innovation performance. Secondly, this study formally delineates interactive behavior dimensions. It classifies them into cooperative trust and cooperative communication, and verifies their effects on cooperative innovation performance, providing a theoretical analysis tool for subsequent research on the interactive behavior of industry–university–research. The division of the dimensions and the findings of this study also further various related academic views (Li and Liu, 2013; Xie et al., 2018; Qiu and Haugland, 2019). Thirdly, the mechanism of the impact of the interactive behavior on cooperative innovation performance is demonstrated, which provides micro evidence for better achieving the goals of industry–university–research cooperation.
Various conclusions were drawn in this study from the perspective of knowledge management. The central conclusion of this study shows that the interactive behavior should be strengthened in the process of industry–university–research cooperation. Firstly, enterprises should increase contact with relevant universities and research institutes and establish long-term and stable exchange and cooperation relationships. Enterprises should also make use of the innovation resources of universities and research institutes to improve their knowledge absorptive capacity and independent innovation power. Secondly, universities and research institutes should adhere to open scientific research and strengthen their ties with enterprises to actively engage in industry–university–research cooperation. They should also strengthen the construction of the platforms for the integration of industry–university–research, and make full use of enterprise resources to promote teaching and scientific research. Most importantly, it is necessary to improve and perfect the incentive system for industry–university–research cooperation, and deepen the relationship of cooperative trust. Thirdly, the government should establish a combined online and offline information platform to promote the exchange of information on industry–university–research cooperation. It should improve and perfect the policy system of industry–university–research cooperation, and create a fair, honest, and inclusive market environment. The above recommendations are valuable guidance for enterprises, universities, and research institutes, and for the government.
On the basis of the interactive behavior from a microperspective, this study explores the influence of interactive behavior on cooperative innovation performance. Firstly, the concept and dimensions of the interaction behavior of industry–university–research cooperation are defined. The existing theories and study results were also used to construct a theoretical model. The questionnaires were conducted with the help of the local Science Technology Department. A total of 325 valid questionnaires were collected. The study found that the interactive behavior of industry–university–research cooperation promotes the improvement of cooperative innovation performance. Specifically, cooperative communication and cooperative trust all play a role in promoting cooperative innovation performance. Knowledge absorptive capacity plays a partial role in mediating the relationship between interactive behavior and cooperative innovation performance. In the heterogeneity analysis, the effect of cooperative trust on cooperative innovation performance was not significant among low-size enterprises and was significant among large-size enterprises. Secondly, the effect of cooperative trust on cooperative innovation performance was significant among the “Low-Group” and not significant among the “High-Group.” Thirdly, the promoting effect of cooperation trust on cooperative innovation performance in the “Experienced group” was far greater than that in the “inexperienced group.” Future research can further explore the heterogeneity analysis of cooperative innovation performance.
On the basis of the process of industry–university–research cooperation, it would be a valuable research topic to examine the influence of the interactive behavior on the cooperative innovation performance of industry–university–research cooperation, and to propose improvement countermeasures from the microbehavioral level of the subjects of industry–university–research cooperation. Despite its strengths, the study has certain deficiencies. Firstly, we only selected data from Zhejiang Province for the study, which is geographically limited. Subsequent studies can explore national data and analyze the heterogeneity of geographical areas. Secondly, the factors that influence the interactive behavior of industry–university–research are complex. Future research should further explore the antecedents and post-variables of interactive behavior, and more micro-influencing factors of cooperative innovation performance.
The original contributions presented in the study are included in the article/supplementary material, further inquiries can be directed to the corresponding author.
XM and KD: conceptualization. XM: methodology, formal analysis, and writing—original draft preparation. HS: software. XJ and XH: validation. CW and LF: investigation and data curation. WL: resources. XM: writing—review and editing. All authors contributed to the article and approved the submitted version.
This research was funded by Zhejiang Provincial Philosophy and Social Science Planning Project "Research on Improving the Competitiveness of Zhejiang Service Outsourcing Park under the Perspective of Sharing Economy, grant number 18NDJC311YBM.
The authors gratefully acknowledge the immense support provided by Zhejiang Provincial Philosophy and Social Science Planning Project of China, a key research-based organization for science and technology.
The authors declare that the research was conducted in the absence of any commercial or financial relationships that could be construed as a potential conflict of interest.
All claims expressed in this article are solely those of the authors and do not necessarily represent those of their affiliated organizations, or those of the publisher, the editors and the reviewers. Any product that may be evaluated in this article, or claim that may be made by its manufacturer, is not guaranteed or endorsed by the publisher.
Bai, Y., and Chen, S. (2022). Study on the green innovation industry-university-research cooperation under the international technology spillover. Ind. Eng. Innov. Manage. 5, 44–49. doi: 10.23977/IEIM.2022.050306
Braojos, J., Benitez, J., Llorens, J., and Ruiz, L. (2020). Impact of IT integration on the firm's knowledge absorption and desorption. Inf. Manag. 57:103290. doi: 10.1016/j.im.2020.103290
Cao, D. H., Zhu, G. L., and Deng, Y. X. (2013). The impact of absorptive capacity on university and industry collaboration performance. Sci. Technol. Prog. Policy 30, 5–9. doi: 10.6049/kjjbydc.2011100166
Casanueva, C., Castro, I., and Galan, J. L. (2013). Informational networks and innovation in mature industrial clusters. J. Bus. Res. 66, 603–613. doi: 10.1016/j.jbusres.2012.02.043
Chen, J., Yang, X. H., Zheng, X. R., and Lv, P. (2009). Knowledge agglomeration:production-education-research cooperation model in the service industry of science and technology. Res. Educ. Eng. 4, 31–36.
Chen, H. C., Zhang, J., and Fei, Y. T. (2020). Has institutional support promoted the collaborative innovation of industry-university-research?—the moderation effect of absorptive capacity of enterprise and the mediation effect of cooperative tightness industry-university-research. Sci. Res. Manag. 41, 1–11. doi: 10.19571/j.cnki.1000-2995.2020.03.001
Cohen, W. M., and Levinthal, D. A. (1990). Absorptive capacity: a new perspective on learning and innovation. Adm. Sci. Q. 35:128. doi: 10.2307/2393553
Cui, Z., and Li, E. (2022). Does industry-university-research cooperation matter? An analysis of its coupling effect on regional innovation and economic development. Chin. Geogr. Sci. 32, 915–930. doi: 10.1007/s11769-022-1308-y
Davenport, T. H., and Prusak, L. (2000). Working knowledge: how organizations manage what they know. Ubiquity 2000:2. doi: 10.1145/347634.348775
De Zubielqui, G. C., Lindsay, N., Lindsay, W., and Jones, J. (2019). Knowledge quality, innovation and firm performance: a study of knowledge transfer in SMEs. Small Bus. Econ. 53, 145–164. doi: 10.1007/s11187-018-0046-0
Dirks, K. T. (1999). The effects of interpersonal trust on work group performance. J. Appl. Psychol. 84, 445–455. doi: 10.1037/0021-9010.84.3.445
Dirks, K. T., and Ferrin, D. L. (2001). The role of trust in organizational settings. Organ. Sci. 12, 450–467. doi: 10.1287/orsc.12.4.450.10640
Dong, G. K., Kirsch, L. J., and King, W. R. (2005). Antecedents of knowledge transfer from consultants to clients in Enterprise system implementations. MIS Q. 29:59. doi: 10.2307/25148668
Duan, X., Sun, P., Wang, X., and Zhan, B. (2022). Evolutionary game analysis of industry-university-research cooperative innovation in digital media Enterprise cluster based on GS algorithm. Wirel. Commun. Mob. Comput. 2022, 1–10. doi: 10.1155/2022/5701917
Fan, C., Hu, S. R., and Wei, J. (2010). Empirical study on influencement factors of interaction innovation performance between KIBS and manufacturing. J. Technol. Econ. 29, 12–18. doi: 10.3969/j.issn.1002-980X.2010.10.003
Gao, Z. J., and Zhang, H. R. (2019). A study of how institutional legitimacy and absorptive capacity influence technology standard competence. Manag. Rev. 31, 73–84. doi: 10.14120/j.cnki.cn11-5057/f.2019.12.007
Gonzalez-Roma, V., and Hernandez, A. (2014). Climate uniformity: its influence on team communication quality, task conflict, and team performance. J. Appl. Psychol. 99, 1042–1058. doi: 10.1037/a0037868
Guo, Y., Duan, W. Y., Meng, J., and Wang, M. X. (2022). Influence of the Chinese Academy of Sciences industry-university-research cooperation network characteristics on the transformation of scientific and technological achievements. Forum Sci. Technol. Chin. 5, 81–89. doi: 10.13580/j.cnki.fstc.2022.05.019
Hackman, J. R. (1987). “The design of work teams” in Handbook of Organizational Behavior. ed. J. Lorsch (Englewood Cliffs, NJ: Prentice-Hall), 299–301.
Hardwick, J., Anderson, A. R., and Cruickshank, D. (2013). Trust formation processes in innovative collaborations networking as knowledge building practices. Eur. J. Innov. Manag. 16, 4–21. doi: 10.1108/14601061311292832
He, Z. M., Chen, H. C., and Hou, J. M. (2021). Research on influence of relationship strength on knowledge governance of Alliance Enterprise: mediation effect of Alliance ability and knowledge absorption ability. Sci. Technol. Manage. Res. 41, 148–155. doi: 10.3969/j.issn.1000-7695.2021.11.018
Hoegl, M., and Gemuenden, H. G. (2001). Teamwork quality and the success of innovative projects: a theoretical concept and empirical evidence. Organ. Sci. 12, 435–449. doi: 10.1287/orsc.12.4.435.10635
Hu, J. Y., Chen, Z. H., Zhou, M. Z., and Jin, Y. (2016). The influence of government funding and industry-university-research cooperation on innovation performance of enterprises: based on the empirical study on Guangdong Province’Questionnaire data. Modern economic. Science 38, 99–105+127-128. doi: 10.3969/j.issn.1002-2848.2016.05.011
Huyck, J. B. V., Battalio, R. C., and Beil, R. O. (1990). Tacit coordination games, strategic uncertainty, and coordination failure. Am. Econ. Rev. 80, 234–248.
Jin, H. H., Xue, X. P., and Miu, Y. D. (2015). Industry-university cooperation and innovation from the perspective of knowledge transfer research on performance relationship. Sci. Technol. Prog. Policy 32, 88–95. doi: 10.6049/kjjbydc.2015040460
Kafouros, M., Wang, C., Piperopoulos, P., and Zhang, M. (2015). Academic collaborations and firm innovation performance in China: the role of region-specific institutions. Res. Policy 44, 803–817. doi: 10.1016/j.respol.2014.11.002
Kopelman, S., Hardin, A. E., Myers, C. G., and Tost, L. P. (2016). Cooperation in multicultural negotiations: how the cultures of people with low and high power interact. J. Appl.Psychol. 101, 721–730. doi: 10.1037/apl0000065
Li, P. Z., and Jian, L. R. (2022). Network structure, knowledgebase, and enterprise innovation performance. Complex Syst. Complexity Sci. 19, 31–38. doi: 10.13306/j.1672-3813.2022.02.004
Li, C. L., and Liu, Z. Y. (2013). The impact of coupling interaction of industry-university-reindustry search institute on innovation performance. Sci. Res. Manage. 34, 23–30. doi: 10.19571/j.cnki.1000-2995.2013.03.004
Li, W. D., and Liu, H. (2014). An empirical study on relationship of mutual trust and R&D professionals’ knowledge sharing intentions: the mediating effects of knowledge power loss and reciprocity. Manag. Rev. 26, 128–138. doi: 10.14120/j.cnki.cn11-5057/f.2014.03.017
Li, M. X., Su, J. L., and Hu, C. (2020). Research on the influence of enterprise network position and relationship intensity on technological innovation performance in IUR cooperation. Sci. Technol. Prog. Policy 37, 118–124. doi: 10.6049/kjjbydc.Q201908747
Li, Z., Wan, T., and Lan, J. (2022). Substitution or complementarity: influence of industry-university-research-institute cooperation governance mechanism on knowledge transfer-an empirical analysis from China. Sustain. For. 14, 1–24. doi: 10.3390/su14137606
Li, X. G., Xia, W. L., and Liu, D. J. (2015). Research on knowledge transfer dynamic mechanism ofSchool-enterprise cooperation innovation network based on the evolution of trust. Inf. Sci. 33, 42–47. doi: 10.13833/j.cnki.is.2015.05.008
Li, C. L., and Ye, L. (2011). Research on the collaborative innovation of industry university collaboration institutions from the perspective of interaction Sci. Technol. Prog. Policy. 28, 30–33. doi: 10.3969/j.issn.1001-7348.2011.24.008
Li, Q., and Zhang, Z. H. (2020). Influence of structure hole, relationship quality and knowledge absorption capacity on Enterprise innovation performance. J. Shandong Univ. of Fin. Econ. 32, 79–88. doi: 10.3969/j.issn.1008-2670.2020.04.008
Li, Z. X. H., and Zhu, G. L. (2019). The impact of partners difference on knowledge transfer in university-institute-industry collaboration. Stud. Sci. Sci. 37, 320–328. doi: 10.16192/j.cnki.1003-2053.2019.02.018
Li, Z. X. H., and Zhu, G. L. (2021). Knowledge transfer performance of industry-university-research institute collaboration in China: the moderating effect of partner difference. Sustain. For. 13:13202. doi: 10.3390/su132313202
Linh Nguyen, P., Khanh Cao, T., Nhuan Nguyen, D., and Uyen Nguyen, T. (2022). The impact of absorption capability, innovation capability, and branding capability on firm performance-an empirical study on Vietnamese retail firms. Sustain. For. 14:6422. doi: 10.3390/su14116422
Liu, Y., Liu, Z. Y., and Li, J. (2020). Research on efficiency and differences of regional industry-university-research synergetic innovation in China. Technol. Soc. 63, 1–12. doi: 10.1016/j.techsoc.2020.101369
Liu, S. S. (2013). Impact of industry-university cooperative organzizations’ interactive behavior on firms’ innovative performance: knowledge absorptive capacity as mediator. Southwestern Univ. Finance Econ.
Lu, Y. Q., and Ye, Y. P. (2017). Impact of network routines on the innovation performance in industry-university-institute innovation. Sci. Res. Manage. 38, 11–17. doi: 10.19571/j.cnki.1000-2995.2017.03.002
Ma, W. C., Ye, Y. P., Xu, M. D., and Zhu, G. (2018). To love each other or to be equal status: the university-industry collaboration partner fit and its impact on knowledge sharing and cooperation performance. Nankai Bus. Rev. 21, 95–106. doi: 10.3969/j.issn.1008-3448.2018.06.010
Meng, Q. L., Hang, Y., and Chen, X. J. (2021). Network position and crowdsourcing innovation contribution behavior: the moderating role of knowledge absorption capacity. Complexity 2021, 1–14. doi: 10.1155/2021/9969509
Miroshnychenko, I., Strobl, A., Matzler, K., and De Massis, A. (2021). Absorptive capacity, strategic flexibility, and business model innovation: empirical evidence from Italian SMEs. J. Bus. Res. 130, 670–682. doi: 10.1016/j.jbusres.2020.02.015
Norman, P. M. (2004). Knowledge acquisition, knowledge loss, and satisfaction in high technology alliances. J. Bus. Res. 57, 610–619. doi: 10.1016/S0148-2963(02)00395-8
Pinjani, P., and Palvia, P. (2013). Trust and knowledge sharing in diverse global virtual teams. Inf. Manag. 50, 144–153. doi: 10.1016/j.im.2012.10.002
Qing, Y., and Qi, W. (2020). Innovation ability of industry-university-research cooperation and innovation considering split-combined quadratic big data dynamic model construction. Int. J. Electr. Eng. Educ. 1–18. doi: 10.1177/0020720920929659
Qiu, X. L., and Haugland, S. A. (2019). The role of regulatory focus and trustworthiness in knowledge transfer and leakage in alliances. Ind. Mark. Manag. 83, 162–173. doi: 10.1016/j.indmarman.2019.03.014
Rupietta, C., and Backes-Gellner, U. (2017). Combining knowledge stock and knowledge flow to generate superior incremental innovation performance — Evidence from Swiss manufacturing. J. Bus. Res. 94, 209–222. doi: 10.1016/j.jbusres.2017.04.003
Schweisfurth, T. G., and Raasch, C. (2018). Absorptive capacity for need knowledge: antecedents and effects for employee innovativeness. Res. Policy 47, 687–699. doi: 10.1016/j.respol.2018.01.017
Song, Y., Sahut, J.-M., Zhang, Z., Tian, Y., and Hikkerova, L. (2022). The effects of government subsidies on the sustainable innovation of university-industry collaboration. Technol. Forecast. Soc. Chang. 174:121233. doi: 10.1016/j.techfore.2021.121233
Sun, Y. Y., Wang, T., and Gu, X. (2019). A sustainable development perspective on cooperative culture, knowledge flow, and innovation network governance performance. Sustain. For. 11:6126. doi: 10.3390/su11216126
Takagi, D., and Shimada, T. (2019). A spatial regression analysis on the effect of neighborhood-level trust on cooperative behaviors: Comparison with a multilevel regression analysis. Front. Psychol. 10:02799. doi: 10.3389/fpsyg.2019.02799
Tison, R., and Poirier, P. (2021). Active inference and cooperative communication: an ecological alternative to the alignment view. Front. Psychol. 12:708780. doi: 10.3389/fpsyg.2021.708780
Trong Hai, D., Ngoc Thanh, N., Duc Cuong, N., Thi Phuong, T. N., and Selamat, A. (2014). Trust-based consensus for collaborative ontology building. Cybern. Syst. 45, 146–164. doi: 10.1080/01969722.2014.874815
Valentim, L., Lisboa, J. V., and Franco, M. (2016). Knowledge management practices and absorptive capacity in small and medium-sized enterprises: is there really a linkage? R & D Management 46, 711–725. doi: 10.1111/radm.12108
Valls, V., Gonzalez-Roma, V., and Tomas, I. (2016). Linking educational diversity and team performance: team communication quality and innovation team climate matter. J. Occup. Organ. Psychol. 89, 751–771. doi: 10.1111/joop.12152
Vasil, J., Badcock, P. B., Constant, A., Friston, K., and Ramstead, M. J. D. (2020). A world unto itself: human communication as active inference. Front. Psychol. 11:417. doi: 10.3389/fpsyg.2020.00417
Veugelers, R., and Cassiman, B. (2005). R&D cooperation between firms and universities. Some empirical evidence from Belgian manufacturing. Int. J. Ind. Organ. 23, 355–379. doi: 10.1016/j.ijindorg.2005.01.008
Wang, X., Fang, H., Zhang, F., and Fang, S. R. (2018). The spatial analysis of regional innovation performance and industry-university-research institution collaborative innovation-an empirical study of Chinese provincial data. Sustain. For. 10:1243. doi: 10.3390/su10041243
Wang, X. H., and Hu, S. L. (2022). Does university-industry collaboration improve the technological innovation performance of manufacturing firms?An empirical study based on propensity score matching method. J. Technol. Econ. 41, 30–43.
Wang, L. P., and Luan, H. M. (2019). Organization distance, value co-creation, and industry-university-research collaborative innovation performance. Chin. J. Manag. 16, 704–711.
Wang, Y. Y., Yuan, C. H., Zhang, S. M., and Wang, R. Q. (2021). Moderation in all things: industry-university-research alliance portfolio configuration and SMEs' innovation performance in China. J. Small Bus. Manag. 60, 1516–1544. doi: 10.1080/00472778.2020.1867735
Wang, X. X., Zhu, G. L., and Wang, Z. (2022). Build the nest and a good bridge: the relationship among knowledge coupling, trust and organizational learning in industry-academia-research cooperation. Sci. Technol. Prog. Policy. 39, 58–66. doi: 10.6049/kjjbydc.CZ202107118
Wu, Y., Gu, X., Tu, Z., and Zhang, Z. (2022). System dynamic analysis on industry-university-research institute synergetic innovation process based on knowledge flow. Scientometrics 127, 1317–1338. doi: 10.1007/s11192-021-04244-y
Wu, A. H., Wang, Z., and Chen, S. (2017). Impact of specific investments, governance mechanisms and behaviors on the performance of cooperative innovation projects. Int. J. Proj. Manag. 35, 504–515. doi: 10.1016/j.ijproman.2016.12.005
Xie, G., Li, W. Y., Tian, H. Y., and Li, W. H. (2018). Co-evolution of intermediary-firm interaction and Firm's absorptive capability—based on the case study of technology small firm. Soft Sci. 32, 88–91+96. doi: 10.13956/j.ss.1001-8409.2018.10.20
Xu, H. B., and Gao, X. Y. (2006). The mechanism that interpersonal trust facilitates knowledge transfer in organizational settings: an integrative framework. Nankai Bus. Rev. 5, 99–106. doi: 10.3969/j.issn.1008-3448.2006.05.016
Yan, H. Y., Bao, X. Z., and He, Q. (2017). Social network analysis of innovation of industry-university-research cooperation in chemical industry (based on China patent licensing data). Bulg. Chem. Commun. 49, 98–103.
Yang, D. S., Bian, W. X., and Zhang, Y. A. (2008). Game analysis on the selection for university-industry cooperative innovation. Sci. Res. Manage. 29, 29–31+35. doi: 10.19571/j.cnki.1000-2995.2008.s1.009
Yang, N., Du, W., Mei, F., Zhang, Y., Du, J., and Sharif, S. M. F. (2022). Impact of partner heterogeneity on innovation performance through interaction behaviour in Chinese complex product R&D industries. Int. J. Technol. Manag. 89, 232–252. doi: 10.1504/ijtm.2022.123740
Ye, C. S., and Chen, C. M. (2022). Industry-university research collaboration, knowledge absorptive capacity and Enterprise innovation performance. Sci. Technol. Manage. Res. 42, 184–194. doi: 10.3969/j.issn.1000-7695.2022.3.023
Zhang, S., and Chen, C. (2022). Impact of technological enterprises' innovation climate on innovation performance—the mediating role of knowledge absorptive capacity. Sci. Res. Manag. 43, 113–120. doi: 10.19571/j.cnki.1000-2995.2022.06.012
Zhang, X. F., Chen, G. H., and Hai, B. L. (2019). Financing constraints, government subsidies and performance of industry-university-institute collaborative innovation. Stud. Sci. Sci. 37, 1529–1536. doi: 10.16192/j.cnki.1003-2053.2019.08.020
Zhang, D. F., and Shen, J. (2019). Organizational proximity, knowledge transfer, big data adoption, and of industry-university-research collaborative innovation performance: a moderated mediation examination. J. Shanghai Univ. Int. Bus. Econ. 26, 49–58. doi: 10.16060/j.cnki.issn2095-8072.2019.06.005
Zhang, S. M., Yuan, C. H., and Han, C. (2020). Industry-university-research alliance portfolio size and firm performance: the contingent role of political connections. Res. Policy 45, 1505–1534. doi: 10.1007/s10961-020-09778-6
Zhang, S. M., Yuan, C. H., and Han, C. (2021). Industry-university-research alliance portfolio partner diversity and firm innovation performance: moderating roles of business environment and state ownership. J. Ind. Eng. Eng. Manage. 35, 51–60. doi: 10.13587/j.cnki.jieem.2021.04.005
Zhang, S. M., Zhang, Y. F., and Yuan, C. H. (2022). How partner geographic concentration in industry-university-research alliance portfolios influences focal firms′innovation performance: analyses of mediating and moderating effects. J. Ind. Eng. Eng. 838, 1–13. doi: 10.13587/j.cnki.jieem.2023.02.004
Zhao, J., Dong, L., and Xi, X. (2019). Research on the strategic alliance innovation system evolution mechanism: the perspective of knowledge flow. Cluster Comput. J. Netw. Software Tools Appl. 22, 9209–S9227. doi: 10.1007/s10586-018-2113-3
Zhao, S., and Ma, J. (2021). Halo or curse: why domain-relevant SkillsImpede creativity?—modification and expansion of Amabile's classical theory. Nankai Bus. Rev. 6, 1–25.
Zhou, X. (2022). Moderating effect of structural holes on absorptive capacity and knowledge-innovation performance: empirical evidence from Chinese firms. Sustain. For. 14:5821. doi: 10.3390/su14105821
Keywords: industry–university–research cooperation, cooperative trust, cooperative communication, knowledge absorptive capacity, cooperative innovation performance
Citation: Meng X, Di K, Su H, Jin X, Lv W, Huang X, Wu C and Fan L (2023) The relationship between the interactive behavior of industry–university–research subjects and the cooperative innovation performance: The mediating role of knowledge absorptive capacity. Front. Psychol. 13:1077614. doi: 10.3389/fpsyg.2022.1077614
Received: 23 October 2022; Accepted: 09 December 2022;
Published: 05 January 2023.
Edited by:
Shi Yin, Agricultural University of Hebei, ChinaReviewed by:
Francesca Loia, University of Naples Federico II, ItalyCopyright © 2023 Meng, Di, Su, Jin, Lv, Huang, Wu and Fan. This is an open-access article distributed under the terms of the Creative Commons Attribution License (CC BY). The use, distribution or reproduction in other forums is permitted, provided the original author(s) and the copyright owner(s) are credited and that the original publication in this journal is cited, in accordance with accepted academic practice. No use, distribution or reproduction is permitted which does not comply with these terms.
*Correspondence: Xiulan Meng, ✉ bXhsYW45MEAxNjMuY29t
Disclaimer: All claims expressed in this article are solely those of the authors and do not necessarily represent those of their affiliated organizations, or those of the publisher, the editors and the reviewers. Any product that may be evaluated in this article or claim that may be made by its manufacturer is not guaranteed or endorsed by the publisher.
Research integrity at Frontiers
Learn more about the work of our research integrity team to safeguard the quality of each article we publish.