- 1The First Affiliated Hospital, Xinjiang Medical University, Ürümqi, China
- 2Public Health and Preventive Medicine Post-Doctoral Mobile Station, Xinjiang Medical University, Ürümqi, China
- 3Xinjiang Clinical Research Center of Urogenital Diseases, Ürümqi, China
- 4College of Public Health, Xinjiang Medical University, Ürümqi, China
- 5Department of Sanitary Technology, Department of Public Health, Karamay Campus, Xinjiang Medical University, Karamay, China
- 6Secondary Supply Room, Disinfection Distribution Center of the First Affiliated Hospital of Xinjiang Medical University, Ürümqi, China
- 7Karamay Central Hospital Health Management Center, Karamay, China
- 8Department of Epidemiology and Health Statistics, College of Public Health, Xinjiang Medical University, Ürümqi, China
- 9Clinical Post-Doctoral Mobile Stations, Xinjiang Medical University, Ürümqi, China
Objectives: Use Bayes statistical methods to analyze the factors related to the working ability of petroleum workers in China and establish a predictive model for prediction so as to provide a reference for improving the working ability of petroleum workers.
Materials and methods: The data come from the health questionnaire database of petroleum workers in the Karamay region, Xinjiang, China. The database contains the results of a health questionnaire survey conducted with 4,259 petroleum workers. We established an unsupervised Bayesian network, using Node-Force to analyze the dependencies between influencing factors, and established a supervised Bayesian network, using mutual information analysis methods (MI) to influence factors of oil workers’ work ability. We used the Bayesian target interpretation tree model to observe changes in the probability distribution of work ability classification under different conditions of important influencing factors. In addition, we established the Tree Augmented Naïve Bayes (TAN) prediction model to improve work ability, make predictions, and conduct an evaluation.
Results: (1) The unsupervised Bayesian network shows that there is a direct relationship between shoulder and neck musculoskeletal diseases, anxiety, working age, and work ability, (2) The supervised Bayesian network shows that anxiety, depression, shoulder and neck musculoskeletal diseases (Musculoskeletal Disorders, MSDs), low back musculoskeletal disorders (Musculoskeletal Disorders, MSDs), working years, age, occupational stress, and hypertension are relatively important factors that affect work ability. Other factors have a relative impact on work ability but are less important.
Conclusion: Anxiety, depression, shoulder and neck MSDs, waist and back MSDs, and length of service are important influencing factors of work ability. The Tree Augmented Naïve Bayes prediction model has general performance in predicting workers’ work ability, and the Bayesian model needs to be deepened in subsequent research and a more appropriate forecasting method should be chosen.
Introduction
Work ability is a multifactorial concept, including workers’ health status, physical capacity, and psychosocial resources (Oellingrath et al., 2019). Work ability reflects the balanced relationship between work needs and productivity. Maintaining and improving the work ability of workers has a positive impact on promoting labor productivity and socioeconomic development. A decrease in work ability and an increase in absenteeism due to illness, decrease in work efficiency, and early retirement are increasing (Ng and Chan, 2018), and this loss of work ability will increase personal and socioeconomic burdens. Research evidence from the United States shows that the annual economic loss caused by loss of work ability is as high as $260 billion each year (Mitchell and Bates, 2011).
There are many factors affecting work ability (van den Berg et al., 2008). Some previous studies have shown that work ability is closely related to mental and psychological factors (Ruitenburgm et al., 2012). The research results of Gharibi et al. (2016) showed that the low work ability of Iranian workers was significantly related to work pressure. A research report by Sun, X, and others pointed out that the work ability of copper-nickel miners in Xinjiang decreased with the increase of job burnout; thus, reducing job burnout can improve the work ability of copper and nickel miners (Sun et al., 2020). There are also some research results showing that anxiety is closely related to work ability. Anxiety in the workplace is a long-term chronic mental health problem, which can lead to long-term sick leave and frequent absenteeism, resulting in loss of work ability (Muschalla, 2017). In a randomized controlled experimental study, the reduction of depressive symptoms could improve the work ability of workers (Hange et al., 2017). Some sociodemographic characteristics are also related to work ability. At present, the world is facing the problem of an aging labor force. The individual resources of elderly workers decrease with age, especially physical strength and certain cognitive abilities, while job needs remain the same or even increase. This leads to a reduction in work ability (Ilmarinen and Ilmarinen, 2015). In addition, sociodemographic characteristics, such as education level, marital status, obesity, and smoking, are also important factors influencing work performance (Qiaojun et al., 2017; Garzaro et al., 2019). At present, the research on the work ability of the professional population mainly involves teachers (Zuorong et al., 2015), medical staff (Xue, 2014), and manual workers (Yanxia et al., 2015). Among the manual workers in some special occupations, occupation-related characteristics play an important role in their health and work ability. For example, oil workers have unique work conditions. Their long-term shifts, irregular work and rest schedules, heavy workload, and long-term improper working postures cause long-term pain in the neck, shoulders, and lower back. Therefore, the muscles of petroleum workers, the morbidity rate of skeletal injuries (shoulder, neck, and lower back) are very high (Ning, 2016). Ge et al.’s study showed that the prevalence of musculoskeletal diseases among oil workers is as high as 84.7%, which not only affects their health but also causes long-term damage to their work ability (Ge et al., 2018). In addition, most of the workplaces of petroleum workers are in the Gobi Desert, so workers are extremely prone to mental and psychological problems, such as occupational stress, job burnout, anxiety, etc. These factors not only damage workers’ mental health but are also important factors that lead to reduced work ability (Brešić et al., 2007; Lee et al., 2017; Xiaoming et al., 2017; Xue, 2020).
The health of the occupational population has attracted much attention, and occupational health-related surveys and monitoring have gradually increased. In the process of occupational health surveying and monitoring, a large amount of information and data have been generated, but these data have not been well-exploited (Abad et al., 2019). Gerassis et al. (2018) believe that the application of new technological solutions based on artificial intelligence (AI) to occupational health databases has become a new strategy. Using big data to evaluate the results of occupational health surveys and monitoring can provide new models and ideas and explore the relationships between pieces of information to identify potential occupational health risk factors. Bayesian network is a data mining (or data-based machine learning) method that visualizes the relationship between variables as an interactive network. The result for each relationship is based on statistical conditional probability. All variables are represented by nodes. At each node, the probability distribution of the variable is defined by its relationship with the parent node, which is the root node from which all incoming vectors originate. Variables can directly or indirectly affect the outcome through other variables (Smith et al., 2018). The Bayesian network is widely used in the prediction of occupational accidents and the identification of potential factors affecting medical ability (Laaksonen et al., 2010; Mirzaei Aliabadi et al., 2018; Gerassis et al., 2019), but there is no research applying the Bayesian network model to the work ability of petroleum workers. The purpose of this study is to analyze the occupational health questionnaire database of oil workers using the Bayesian network assessment method, find the potential influencing factors and strong evidence of petroleum workers’ work ability, and establish a work ability prediction model through machine learning in order to formulate intervention measures and promote the work ability of oil workers while providing references.
Materials and methods
Data collection
The data comes from the 2015 oil worker health questionnaire database created in the Karamay region, Xinjiang, China. There are 4,391 copies of the questionnaire. After excluding 132 questionnaires with incomplete information, there were 4,259 complete data records of the petroleum worker health questionnaire.
Variable definitions
The variables in the health questionnaire can be divided into four categories. The first is demographic information: gender, age, ethnicity, marital status, and education level; the second is occupational characteristics: job title, income, shift status, the third category is behavioral factors: smoking, drinking, and Body Mass Index (BMI), and the fourth category is mental health and physical health: anxiety, depression, occupational stress, musculoskeletal conditions (lower back, neck, and shoulder), high blood pressure, and work ability status. In this database, occupational stress is evaluated by the Effort Reward Imbalance (ERI) questionnaire (Siegrist, 1996), and work ability is evaluated by the Work Ability Index (WAI). In the calculation of the WAI index score, work ability is divided into poor (7–27 score), good (28–36 score), and good (37–49 score) (Xiaoming et al., 2017). Musculoskeletal status is assessed using the Nordic Musculoskeletal Questionnaire (Tanaka et al., 2001), in which bone injury is defined as having symptoms of musculoskeletal injury in one or more parts within 1 year.
Data preprocessing
Data preprocessing and the conversion of variable forms are important steps prior to any statistical analysis. Because the Bayesian network is a non-parametric model, continuous variables need to be discredited, and all variables defined (see Appendix Table 1). For this study, missing data were deleted during preprocessing, so there is no missing data processing step.
Bayesian statistical analysis
Unsupervised Bayesian network
Bayesian networks express a set of variables and conditional dependencies via a Directed Acyclic Graph (DAG) (Conrady and Jouffe, 2013). This research first uses a database to establish an unsupervised Bayesian network. The unsupervised learning method represents the most accurate form of knowledge because there are no restrictions on the exploration of the potential relationships between variables in the research. The purpose of the unsupervised Bayesian network model is to discover the interdependence between a large number of variables without specifying the input or output nodes. In order to obtain the influence of the factors, it is necessary to calculate the node-force. The node-force parameter is derived from the sum of the arc force, and the concept of the arc force is based on information theory relative entropy, also known as Kullback–Leibler divergence (DKL) (Shannon, 1948). DKL is a measure of the asymmetry of the difference between two probability distributions, P and Q. In general, P represents the true distribution of the data, Q represents the theoretical distribution of the data, and DKL can be expressed as:
Supervised Bayesian network
This study established a supervised Bayesian network with work ability as the target variable. The purpose was to measure the importance of each factor to the target variable. In the supervised Bayesian network we first set the target variable according to the data and research purpose. In this research, the target variable is work ability. In order to minimize the complexity of the model, we use the Naïve Bayes algorithm to construct the supervised Bayesian network, so that mutual information is easier to calculate. Mutual information is the basic concept of information theory; it represents the amount of information held by each variable itself. Mutual information is defined as the marginal entropy of the target variable and the conditional entropy of a given target. The difference between the two variables is formally called mutual information (MI) (Conrady and Jouffe, 2015). Generally, the mutual information between two variables, X and Y, can be expressed with the following formula:
The MI value between work ability and each factor can determine which factors provide us with the greatest information gain; that is, the importance of each factor to work ability.
Tree augmented naïve Bayes prediction model
The disadvantage of the Naïve Bayes model is that it assumes that the features are independent of each other. However, in reality, there is no complete independence among the variables. The TAN model is a Semi-Naïve Bayes learning method (Friedman et al., 1997). It relaxes the independence assumption of the Naïve Bayes model by adopting a tree structure so that each attribute depends only on the class and another attribute. It can solve the problem of the interdependence of some attributes between conditions (Xiaolin, 2020).
The latest developments and applications of information theory have led to powerful algorithms and mechanisms, which have allowed for the optimization of Bayesian statistics in machine learning applications. According to Bayes’ theorem, Bayesian networks can provide a flexible graphical reasoning method based on the spread of uncertainty in the entire network. This study uses BayesiaLab 9.1 software to construct the Bayesian networks for probability inference and machine learning. Its artificial intelligence platform is a knowledge modeling environment that provides a variety of machine learning algorithm options, as well as an interactive environment for machine learning and knowledge discovery based on a broad set of algorithms in the Bayesian network paradigm.
Results
Analysis of the work ability index of petroleum workers
The average age of petroleum workers is 39.00 ± 8.60 years, and the average work ability index is 37.18 ± 5.86. Petroleum workers with low work ability account for 6.31% of the total petroleum worker population as shown in Table 1.
Unsupervised Bayesian model analyses of dependencies between variables
The unsupervised Bayesian model shows the relationship between variables, and the degree of dependence is proportional to the magnitude of the node-force. In this Bayesian network, we find that age, working age, and work ability are at the core of the network, as well as the starting point of other dependencies. Among them, marital status, working age, job title, and shift status are directly related to age, working age, education level, income level, working ability, and hypertension. There are four sub-nodes of work ability in the figure, namely shoulder and neck MSDs, anxiety, working age, and occupational stress, indicating that work ability is directly dependent on these four variables. The node-force shows the strength of the dependent relationship in the process of workers’ health survey. Among them, age, working age, gender, smoking, and shoulder and neck MSDs are the most influential factors in the survey process (see Figure 1).
Sensitivity analysis of supervised Bayesian network
A sensitivity analysis can rank the importance of factors affecting work ability. The supervised Naïve Bayes model with work ability as the target node shows that the MI between computing nodes can determine which factors provide the greatest information gain for work ability. The Bayesian Network graph is sorted clockwise according to the size of the information gain. Figure 2 shows the MI between all nodes and the target node, including anxiety, depression, shoulder and neck diseases, low back MSDs, and shoulder and neck MSDs. The MI value of the factor node and work ability is relatively large; that is, these five factors are the main influencing factors that affect work ability, and the other factors have relatively little influence on work ability (see Figure 2).
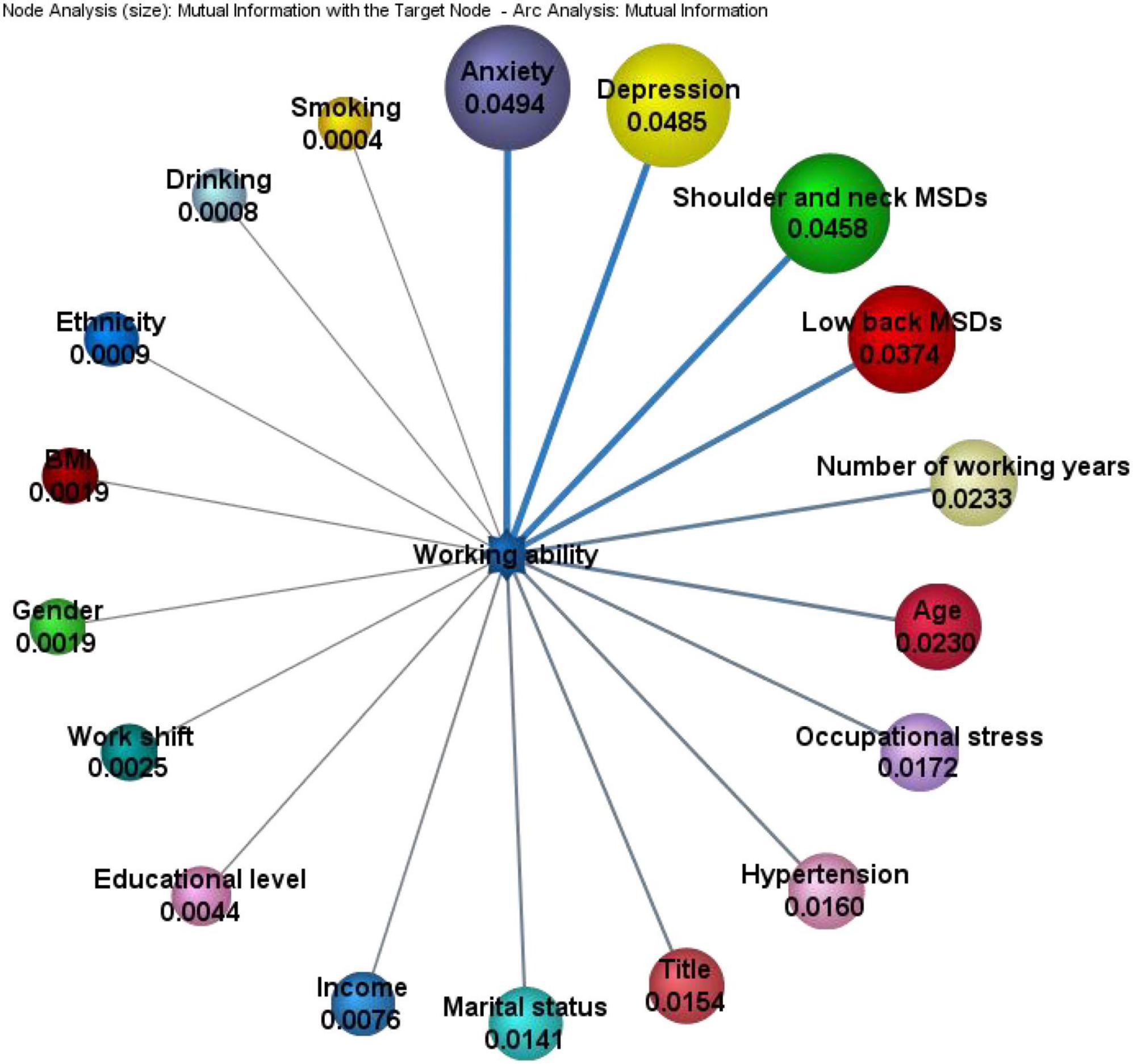
Figure 2. Sensitivity analysis of supervised Bayesian network. The numbers in the figure are calculated by MI. The sizes of the circles and the thickness of the lines are proportional to the importance of the influencing factors.
Bayesian probability interpretation tree models of work ability
The probabilistic interpretation tree model is a static tree structure that shows the influence of the different states of various factors on the probability distribution of the work ability state. The leftmost node in the tree (without any evidence set) shows the marginal probability distribution of the work ability. The probability distribution is the probability that each state of work ability occurs separately without any evidence. Considering the size of the graph, this study sets the maximum number of variables to four. The bottom panel of the leftmost node in the figure shows anxiety as the most important variable to be considered. The two branches that appear from the node represent the two MDs of the shoulder and neck. There are two different states, namely presence and absence, and we can see that when anxiety (Yes), depression (Yes), shoulder and neck MSDs (Yes), and low back MSDs (Yes) occur at the same time, the joint probability is 5.33%. At this time, the probability distribution of work ability considered high accounts for 12.37%, the probability distribution of work ability considered medium accounts for 61.16%, and that considered poor accounts for 26.46%. The joint probability of not having anxiety (No), depression (No), shoulder and neck MSDs (No), and waist and back MSDs (No) at the same time is 4.53%. The probability distribution of work ability considered high is 94.41%, the probability distribution of work ability considered medium is 5.24%, and the probability of work ability considered poor is The distribution accounts for 0.34% (see Figure 3).
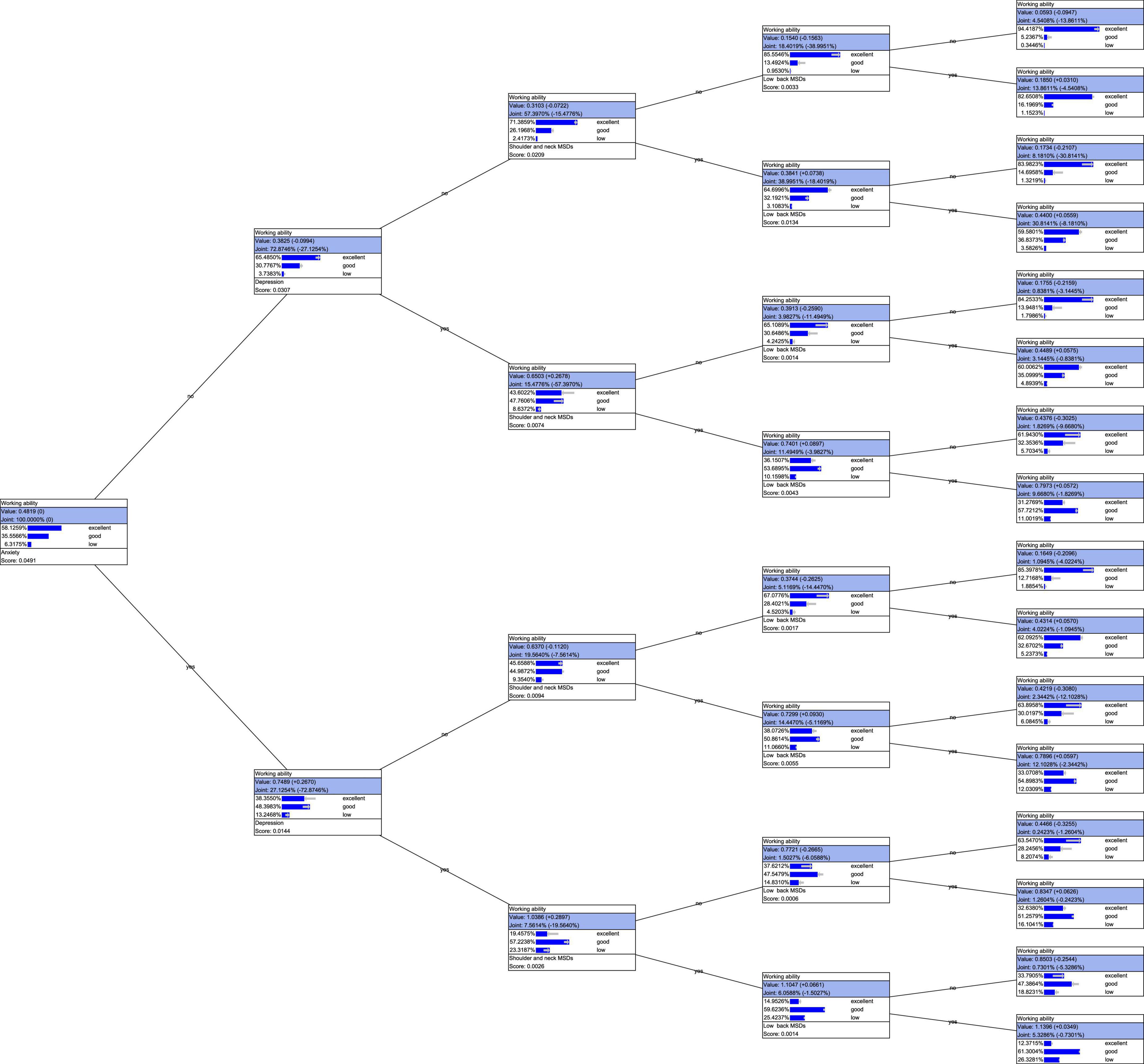
Figure 3. Bayesian probability interpretation tree model of work ability. In Figure 3, the arrows in the figure indicate the trends of the probability distribution changes, the joints in the tree are the joint probabilities, and the score is equal to the mutual information MI score.
Tree augmented naïve Bayes prediction model
It can be seen in Figure 1 that there is a mutual dependence between variables;, that is, variables are not independent of each other, so we use the TAN prediction model. In the model, the work ability is set as the target variable to be predicted. Since the target variable is a multi-categorical variable, we need to convert it into a binary variable. According to the literature review by Yajia et al. (2000), comprehensive work ability is measured by the average of the scores minus one standard deviation as the cut-off value. The comprehensive work ability score is divided into two groups. The cut-off value of this study is a score of 31. Those with a score of less than 31 are considered the work ability decline group, while those with a score greater than or equal to 31 are considered the normal work ability group. Other factors are regarded as influencing variables that affect the state of the target variable. Then, we use the TAN algorithm, automatically implemented by BayesiaLab, to establish a Bayesian prediction model.
Comprehensive forecasting ability analysis of the tree augmented naïve Bayes forecasting model
First, we divide the data into 10 equal parts, then dividing the data into a training set and a test set at a ratio of 8:2. In order to avoid the model becoming too complicated, we use the variable selection method in the TAN model. The model finally selects occupational stress, anxiety, depression, shoulder and neck MSDs, age, and hypertension, which were modeled as characteristic variables in the model. The comprehensive evaluation of the predictive ability was made based on the accuracy, reliability, and area under the Receiver Operating Characteristic (ROC) curve, as shown in Table 2.
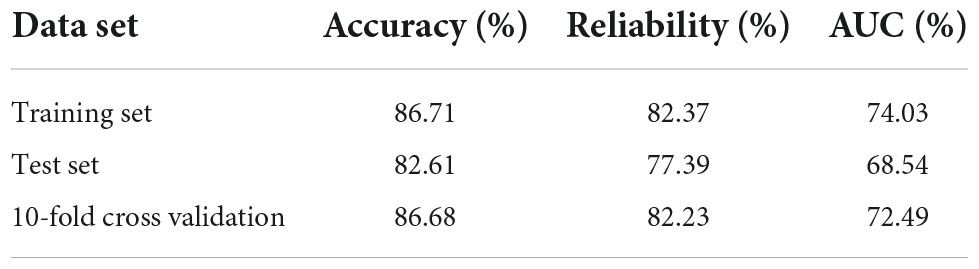
Table 2. Evaluation index analysis of the Bayesian forecasting model using different numbers of variables.
Discussion
With the growth of the world economy and the advancement of science and technology, the petroleum industry has developed rapidly and has taken a pivotal position in the world economy. The development of the petroleum industry has also made great contributions to China’s national economy. Karamay City, Xinjiang, China, is an industrialized city dominated by the petroleum industry, and its labor force accounts for 70.36% of the permanent population (Yang, 2014). Oil workers’ physical and mental health, as well as their work ability, directly affect the production safety and economic benefits of the oil industry, and it is particularly important to monitor the work ability of the professional population (El Fassi et al., 2013). Although the Karamay City Occupational Health and Hygiene Service Organization provides annual physical examinations and surveys for oil workers, the data generated by these physical examinations and surveys are difficult to make good use of. This research aims to explore the relationship between information related to work ability in health surveys by using Bayesian network assessment methods.
The Bayesian network model is helpful for revealing the complex network relationship among the factors related to work ability. The unsupervised Bayesian network used in this study shows that work ability is directly related to occupational stress, shoulder and neck musculoskeletal diseases, anxiety, and length of service. Studies have found that long-term occupational stress can cause changes in the behavior and functions of the occupational population (such as hypertension, headaches, and muscle tension), a weakened response to external stimuli, and decreased work ability and physiological functions (Mazloumi et al., 2012). Depression and anxiety are manifested as absenteeism, which leads to a decline in work ability (Muschalla, 2017). Musculoskeletal diseases are also a common cause of reduced work ability. This study shows that shoulder, neck, and lower back musculoskeletal diseases are relatively important factors influencing work ability by monitoring Bayesian networks and combining mutual information scores. The musculoskeletal system of oil workers is under high stress for a long time and cannot be relieved, which leads to neck and back pain among other musculoskeletal discomforts. These diseases cannot be treated in time so that work ability is also affected (Bugajska and Sagan, 2014; Ge et al., 2018). This study also further explained the influencing factors of work ability through the goal interpretation tree model. When there were no anxiety, depression, shoulder and neck MSDs, or low back MSDs, 94.41% of such oil workers had high work ability. Petroleum workers with poor work ability who did not have any of these conditions accounted for only 0.34%, but of the workers for whom these factors were present at the same time, 26.46% had poor work ability. This shows that these factors can explain the status of workers’ work ability to a significant extent. Anxiety and depression are important indicators of mental health. MSDs in the shoulders and neck and MSDs in the waist and back are important indicators of physical health. This also shows that the work ability of oil workers is affected by social, psychological, and physiological factors.
Working years (or natural age) is also an important factor affecting work ability. Although workers’ work experience and skills improve with the increase of working years, workers’ health and work ability gradually decline with age, especially in a job with high physical demand (Cadiz et al., 2019). It is worth mentioning that the results of this study show that the relationship between BMI and work ability is weak, which is contrary to the results reported in the literature by Andersen et al. (2017). Andersen et al. (2017) insist that obesity is associated with various diseases and functions. The decline is related to the decline. Overweight and obesity will lead to a decline in workers’ work ability. People engaged in sedentary work have a more obvious correlation between BMI and workability than those engaged in physical activities. The nature of the work of oil workers is mostly manual labor. This may be the reason for these inconsistent conclusions. In addition, this study also shows that there is no significant relationship between shifts and work ability, which is consistent with the research conclusion of Fischer et al. (2006), but there are also studies that have shown shifts and work ability being negatively correlated. Shift work may lead to burnout and work errors, which affect work ability (Elovainio et al., 2010). The relationships between BMI, shift work, and work ability need further research.
To predict the work ability of oil workers, this study established a TAN model. The accuracy of the TAN prediction model after 10-fold cross-validation was 86.68%, the reliability was 82.23%, and the AUC was 72.49%. The model has a good prediction accuracy performance, but the classification performance is average. One of the reasons for this may be that the sample imbalance causes the minority data to be predicted incorrectly or mistakenly regarded as noise and processed as such (Spelmen and Porkodi, 2018). The second reason may be that the work ability measured in this research is a multi-classification problem. Due to the imbalance of the sample, when evaluating the classification effect of the imbalanced data model, AUC is often more compatible and distinguishable than the classification accuracy (Feng et al., 2007), while AUC is suitable for evaluating two classification problems. In order to be able to use the AUC value, we divide work ability into two classification problems. This causes changes in the attributes of the sample and may affect the overall model performance. In addition, the data scale and category distribution also affect the model prediction, an important factor of ability (Xi and Kun, 2019).
The relationship between occupational stress and mental health among oilfield workers in an arid desert environment in the Xinjiang region of China was found in previous studies and revealed a causal relationship between occupational stress and psychological disorders, while the relationship between psychological disorders and genetic levels was further explored (Jiang et al., 2021). Workers in the mining, oil and gas extraction industries appear to have worse chronic health conditions than the general working population until retirement (Robinson et al., 2021), and we have found similar phenomena in our study, which deserve further attention. In Nigeria, where oil is the mainstay of the industry, almost all oil workers suffer from work-related musculoskeletal disorders (Omojunikanbi et al., 2022). It is recommended that health management training and occupational assessment programs be provided for workers in specialized industries. There is a need to develop measures to alleviate the occupational stress of oil workers in order to improve the mental health of this specific occupational group, as well as recommending ongoing attention to health status after retirement. This study uses Bayesian networks not only to visualize health management data, but also to build and validate tree-enhanced plain Bayesian models of work ability in relevant work scenarios, which reflect the correlation between the various factors in a more intuitive way. It is not limited to the occupational mental health management of oil workers, but can be further investigated in medical research, engineering information and other areas with the help of Bayesian predictive model selection and inference. This study also has certain limitations. First, the cross-sectional study cannot determine the order in which various factors and work ability occur. Second, the sample source is limited to Karamay City, Xinjiang, China, so the results may not necessarily represent oil workers nationwide. In the end, the imbalance of the sample will affect the overall performance of the prediction model. In the follow-up, we still need to conduct a more extensive investigation of the work ability of oil workers and conduct additional research on the prediction model. Details will be elaborated later. This study is reasonable and novel in applying Bayesian statistical methods to the information mining and predictive modeling tools of influencing factors related to oil workers’ work ability. The overview is reflected in the first application of Bayesian statistical methods to the study of oil workers’ work ability, as well as the information mining and visualization operations of related influencing factors and the development of predictive models, and in the methodological presentation of Bayesian statistical methods in the field of relevant public health and health research centered on oil workers.
Conclusion
This study shows that it is reasonable to apply Bayesian statistical methods to information mining and predictive models of factors related to the work ability of oil workers. Our research results have determined that anxiety, depression, MSDs in the shoulder and neck, MSDs in the waist and back, working age, and occupational stress are all relatively important factors influencing the work ability of oil workers. This provides new insights for improving the working ability of oil workers. Through the Bayesian network, the relationship between the various factors was visualized, the Tree Augmented Naïve Bayes (TAN) model of work ability was established, and the model evaluation was carried out. The results showed that the follow-up of the Bayesian prediction model selection and reasoning still needs to be studied in-depth to provide a scientific basis for establishing a more appropriate predictive model of work ability in the future.
The theoretical and practical implications of the study
The health of occupational groups is of great concern, and relevant questionnaires and monitoring for characteristic occupational groups are gradually increasing, while a large amount of information and data are generated in the process of occupational health surveys and monitoring, but these data have not been well-exploited. The aim of this study is to analyze the occupational health data of oil workers in the Karamay region of China through a Bayesian network evaluation method, to identify potential influencing factors and strong evidence of oil workers’ work capacity, and to build a predictive model of work capacity through machine learning, so as to provide some reference basis for government interventions and public health decisions at a later stage.
Strengths and limitations of this study
The study uses big data to evaluate the results of occupational health surveys and monitoring, which can provide new models and ideas, and explore the interrelationships of information to identify potential occupational health risk factors.
(1) Innovatively, the Bayesian network model is applied to the work ability of oil workers for the first time. Use mutual information analysis method (MI) and Bayesian target interpretation tree model to observe the changes in the probability distribution of work ability classification under different states of important influencing factors, and establish Tree Augment Naïve Bayes (TAN) prediction Model, predict and evaluate work ability.
(2) The purpose of this study is to analyze the occupational health questionnaire database of oil workers through Bayesian network assessment, find the potential influencing factors and strong evidences of oil workers’ working ability, and establish a working ability prediction model through machine learning to formulate interventions Measures and promotes the work ability of oil workers to provide a reference basis.
(3) The study is a cross-sectional study, so it is impossible to establish a causal relationship between factors related to work ability.
(4) The study is conducted in the oil-operating population of Karamay, a resource-based city in Northwest China, and due to special occupations This may affect the extrapolation of research conclusions.
Data availability statement
The original contributions presented in this study are included in the article/supplementary material, further inquiries can be directed to the corresponding authors.
Ethics statement
Written informed consent was obtained from the individual(s) for the publication of any potentially identifiable images or data included in this article. This study was reviewed by the Ethics Committee of Xinjiang Medical University (No. 2015006) and approved on June 26, 2015. Written informed consent to participate in this study was provided by the patient/participants’ or patient/participants legal guardian/next of kin.
Author contributions
NT and DZ designed this study. LX and YL collected and managed these data. DM completed the data analysis. HA and LX drafted the manuscript. NT checked and revised the manuscript. All authors have read and approved the final manuscript.
Funding
This study was supported by the Natural Science Foundation of Xinjiang Uygur Autonomous Region (No. 2020D01C158), Xinjiang Uygur Autonomous Region University Research Program Project (No. XJEDU2018Y029), and Public Health and Preventive Medicine–Speciality Subjects of Higher Education Institutions in the 14th Five-Year Plan, Xinjiang Uygur Autonomous Region Outstanding Postdoctoral Special Grant Program, Xinjiang Clinical Research Center of Urogenital Diseases.
Acknowledgments
We would like to thank Xinjiang Karamay Petroleum Administration and Karamay Central Hospital for their assistance in collecting data. At the same time, we also thank the oil workers who fully cooperated with this study.
Conflict of interest
The authors declare that the research was conducted in the absence of any commercial or financial relationships that could be construed as a potential conflict of interest.
Publisher’s note
All claims expressed in this article are solely those of the authors and do not necessarily represent those of their affiliated organizations, or those of the publisher, the editors and the reviewers. Any product that may be evaluated in this article, or claim that may be made by its manufacturer, is not guaranteed or endorsed by the publisher.
References
Abad, A., Gerassis, S., Saavedra, Á, Giráldez, E., García, J. F., and Taboada, J. (2019). A Bayesian assessment of occupational health surveillance in workers exposed to silica in the energy and construction industry. Environ. Sci. Pollut. Res. 26, 29560–29569. doi: 10.1007/s11356-018-2962-6
Andersen, L. L., Izquierdo, M., and Sundstrup, E. (2017). Overweight and obesity are progressively associated with lower work ability in the general working population: Cross-sectional study among 10,0adults. Int. Arch. Occup. Environ. Health 90, 779–787. doi: 10.1007/s00420-017-1240-0
Brešić, J., Knežević, B., Milošević, M., Tomljanović, T., Golubić, R., and Mustajbegović, J. (2007). Stress and work ability in oil industry workers[J]. Arch. Indust. Hyg. Toxicol. 58, 399–405. doi: 10.2478/v10004-007-0032-4
Bugajska, J., and Sagan, A. (2014). Chronic musculoskeletal disorders as risk factors for reduced work ability in younger and ageing workers. Int. J. Occup. Saf. Ergon. 20, 607–615. doi: 10.1080/10803548.2014.11077069
Cadiz, D. M., Brady, G., Rineer, J. R., and Truxillo, D. M. (2019). A review and synthesis of the work ability literature. Work Aging Retire. 5, 114–138. doi: 10.1093/workar/way010
Conrady, S., and Jouffe, L. (2013) Introduction to bayesian networks & bayesialab. Laval: Bayesia UAS, 1–31. doi: 10.13140/2.1.4737.6965
Conrady, S., and Jouffe, L. (2015). Bayesian networks and bayesia lab: A practical introduction for researchers. Laval: Bayesia USA, 15–25. ISBN:0996533303.
El Fassi, M., Bocquet, V., Majery, N., Lair, M. L., Couffignal, S., and Mairiaux, P. (2013). Work ability assessment in a worker population: Comparison and determinants of Work Ability Index and Work Ability score. BMC Public Health 13:305. doi: 10.1186/1471-2458-13-305
Elovainio, M., Kuusio, H., Aalto, A. M., Sinervo, T., and Heponiemi, T. (2010). Insecurity and shiftwork as characteristics of negative work environment: psychosocial and behavioural mediators. J. Adv. Nurs. 66, 1080–1091. doi: 10.1111/j.1365-2648.2010.05265.x
Feng, Q., Hui, L., Ze-kai, C., Shi-liu, R., and Li, C. (2007). Evaluating performance of multiple Bayes classifier based on AUC method(in Chinese). Comput. Eng. Des. 28, 5919–5920.
Fischer, F. M., Borges, F. N., Rotenberg, L., Latorre Mdo, R., Soares, N. S., Rosa, P. L., et al. (2006). Work ability of health care shift workers: What matters? Chronobiol. Int. 23, 1165–1179. doi: 10.1080/07420520601065083
Friedman, N., Geiger, D., and Goldszmidt, M. (1997). Bayesian network classifiers. Mach. Learn. 29, 131–163. doi: 10.1023/A:1007465528199
Garzaro, G., Sottimano, I., Di Maso, M., Bergamaschi, E., Coggiola, M., Converso, D., et al. (2019). Work ability among italian bank video display terminal operators: Socio-demographic, lifestyle, and occupational correlates. Int. J. Environ. Res. Public Health 16:1653. doi: 10.3390/ijerph16091653
Ge, H., Sun, X., Liu, J., and Zhang, C. (2018). The status of musculoskeletal disorders and its influence on the working ability of oil workers in Xinjiang, China. Int. J. Environ. Res. Public Health 15:842. doi: 10.3390/ijerph15050842
Gerassis, S., Abad, A., Saavedra, Á, García, J. F., and Taboada, J. (2018). Women’s occupational health: Improving medical protocols with artificial intelligence solutions[C]//proceedings of SAI intelligent systems conference. Cham: Springer, 1193–1199. doi: 10.1007/978-3-030-01057-7_88
Gerassis, S., Abad, A., Taboada, J., Saavedra, Á, and Giráldez, E. (2019). A comparative analysis of health surveillance strategies for administrative video display terminal employees. BioMed. Eng. OnLine 18:118. doi: 10.1186/s12938-019-0737-z
Gharibi, V., Mokarami, H., Taban, A., Yazdani Aval, M., Samimi, K., and Salesi, M. (2016). Effects of work-related stress on work ability index among Iranian workers. Saf. Health Work 7, 43–48. doi: 10.1016/j.shaw.2015.10.005
Hange, D., Ariai, N., Kivi, M., Eriksson, M. C., Nejati, S., and Petersson, E. L. (2017). The impact of internet-based cognitive behavior therapy on work ability in patients with depression–a randomized controlled study. Int. J. Gen. Med. 10:151. doi: 10.2147/IJGM.S129710
Ilmarinen, J., and Ilmarinen, V. (2015). “Work ability and aging,” in Facing the challenges of a multi-age workforce: A use-inspired approach, eds L. M. Finkelstein, D. M. Truxillo, F. Fraccaroli, and R. Kanfer (Milton Park: Routledge), 134–156.
Jiang, T., Wumaier, G., Li, X., Yang, X., and Liu, J. (2021). DRD2Research on the effects of occupational stress and the gene on the psychological health of workers in the Xinjiang desert oil field. Front. Psychiatry 12:737228. doi: 10.3389/fpsyt.2021.737228
Laaksonen, M., Pitkäniemi, J., Rahkonen, O., and Lahelma, E. (2010). Work arrangements, physical working conditions, and psychosocial working conditions as risk factors for sickness absence: Bayesian analysis of prospective data. Ann. Epidemiol. 20, 332–338. doi: 10.1016/j.annepidem.2010.02.004
Lee, S. A., Ju, Y. J., Han, K. T., Choi, J. W., Yoon, H. J., and Park, E. C. (2017). The association between loss of work ability and depression: A focus on employment status. Int. Arch. Occup. Environ. Health 90, 109–116. doi: 10.1007/s00420-016-1178-7
Mazloumi, A., Rostamabadi, A., Saraji, J. N., and Rahimi Foroushani, A. (2012). Work ability index (WAI) and its association with psychosocial factors in one of the petrochemical industries in Iran. J. Occup. Health 54, 112–118. doi: 10.1539/joh.11-0150-OA
Mirzaei Aliabadi M., Aghaei H., Kalatpour O., Soltanian A R., and Nikravesh A. (2020). Analysis of human and organizational factors that influence mining accidents based on Bayesian network. Int. J. Occup. Saf. Ergon. 26, 670–677. doi: 10.1080/10803548.2018.1455411
Mitchell, R. J., and Bates, P. (2011). Measuring health-related productivity loss. Popul. Health Manage. 14, 93–98. doi: 10.1089/pop.2010.0014
Muschalla, B. (2017). Is it a case of “work-anxiety” when patients report bad workplace characteristics and low work ability? J. Occup. Rehabil. 27, 106–114. doi: 10.1007/s10926-016-9637-2
Ng, J. Y. K., and Chan, A. H. S. (2018). The work ability of hong kong construction workers in relation to individual and work-related factors. Int. J. Environ. Res. Public Health 15:990. doi: 10.3390/ijerph15050990
Ning, T. (2016). The relationship between occupational stress and hypertension in Xinjiang arid desert oil workers(in Chinese). Xinjiang: Xinjiang Medical University.
Oellingrath, I. M., De Bortoli, M. M., Svendsen, M. V., and Fell, A. (2019). Lifestyle and work ability in a general working population in Norway: A cross-sectional study. BMJ Open 9:e026215. doi: 10.1136/bmjopen-2018-026215
Omojunikanbi, O. A., Akinpelu, A. O., and Ekechukwu, E. N. D. (2022). Prevalence, pattern and predictors of work-related musculoskeletal disorders among oil workers in Nigeria. Work 71, 151–163. doi: 10.3233/WOR-205005
Qiaojun, H., Jianbing, Z., Fengfeng, M., Zhilei, S., Junlong, H., Hongxia, L., et al. (2017). Effects of different educational level, age and body mass index on work ability in naval shipboard personnel. Occup. Health 33, 2831–2833.
Robinson, T., Sussell, A., Yeoman, K., Retzer, K., and Poplin, G. (2021). Health conditions in retired manual labor miners and oil and gas extraction workers: National Health Interview Survey, 2007-2017. Am. J. Indust. Med. 64, 118–126. doi: 10.1002/ajim.23195
Ruitenburgm, M. M., Frings-Dresen, M. H., and Sluiter, J. K. (2012). The prevalence of common mental disorders among hospital physicians and their association with self-reported work ability: A cross-sectional study. BMC Health Serv. Res. 12:292. doi: 10.1186/1472-6963-12-292
Shannon, C. E. (1948). A Mathematical theory of communication. Bell Syst. Tech. J. 27, 379–423. doi: 10.1002/j.1538-7305.1948.tb01338.x
Siegrist, J. (1996). Adverse health effects of high-effort/low-reward conditions. J. Occup. Health Psychol. 1:27. doi: 10.1037/1076-8998.1.1.27
Smith, R. L., Gallicchio, L. M., and Flaws, J. A. (2018). Understanding the complex relationships underlying hot flashes: A Bayesian network approach. Menopause 25, 182–190. doi: 10.1097/GME.0000000000000959
Spelmen, V. S., and Porkodi, R. (2018). A review on handling imbalanced data. 20International Conference on current trends towards converging technologies (ICCTCT), Piscataway, NJ: IEEE. 2018, 1–11. doi: 10.1109/ICCTCT.2018.8551020
Sun, X., Zhang, L., Zhang, C., Liu, J., and Ge, H. (2020). The status of job burnout and its influence on the working ability of copper-nickel miners in Xinjiang, China. BMC Public Health 20:3. doi: 10.1186/s12889-020-8245-4
Tanaka, S., Petersen, M., and Cameron, L. (2001). Prevalence and risk factors of tendinitis and related disorders of the distal upper extremity among US workers: comparison to carpal tunnel syndrome. Am. J. Indust. Med. 39, 328–335. doi: 10.1002/1097-0274(200103)39:3<328::AID-AJIM1021>3.0.CO;2-I
van den Berg, T., Elders, L., de Zwart, B., and Burdorf, A. (2008). The effects of work-related and individual factors on the work ability index: A systematic review. Occup. Environ. Med. 66, 211–220. doi: 10.1136/oem.2008.039883
Xi, C., and Kun, Z. (2019). Classifier learning method based on tree-augmented naïve Bayes(in Chinese). J. Electron. Inf. 41, 2001–2008.
Xiaolin, C. (2020). Personal credit evaluation based on Bayesian network model(in Chinese). Stat. Decis. 36, 153–155.
Xiaoming, M., Haili, K., Chunbo, S., Yue, L., Yangfan, W., Zhihua, L., et al. (2017). Lei Hongyan. Relationship between occupational stress and working ability of workers in a petroleum processing enterprise in high altitude area(in Chinese). Chin. J. Indust. Hyg. Occup. Dis. 35, 907–910.
Xue, L. (2014). Relevant research on medical staff’s occupational stress, occupational burnout and work ability in a 3-a-grade hospital of Xinjiang(in Chinese). Xinjiang: Xinjiang Medical University.
Xue, L. (2020). Study on the influence of job burnout and sleep quality on working ability of oil workers in Xinjiang(in Chinese). Xinjiang: Xinjiang Medical University.
Yajia L., Zhiming, W., Mianzhen, W., Chenglie, Z., Ilmarinen, J., Klockars, M., et al. (2000). The relationship between individual characteristics and common diseases and the working ability of middle-aged and elderly workers(in Chinese). J. West China Univ. Med. Sci. 31, 561–564.
Yang, H. (2014). Research on the change of Karamay city’s population status and its impact on economy and society(in Chinese). Karamay Acad. J. 4, 70–73.
Yanxia, Z., Yan, W., and Yulong, L. (2015). Research on the correlations between occupational stress and working ability among manual workers(in Chinese), Vol. 38. Xinjiang: Journal of Xinjiang Medical University, 500–503.
Zuorong, Z., Junling, Z., Mingming, H., Wang, K., Liu, J., Zhang, Y., et al. (2015). Status of job burnout and work ability of college teachers(in Chinese). Chin. J. Ind. Hyg. Occup. Dis. 33, 107–109.
Appendix
Keywords: Bayes evaluation, working ability, petroleum workers, stress, occupation
Citation: An H, Xu L, Liu Y, Ma D, Zhang D and Tao N (2022) Study on a Bayes evaluation of the working ability of petroleum workers in the Karamay region, Xinjiang, China. Front. Psychol. 13:1011137. doi: 10.3389/fpsyg.2022.1011137
Received: 03 August 2022; Accepted: 29 August 2022;
Published: 10 October 2022.
Edited by:
Jian Zhou, Nankai University, ChinaReviewed by:
Shankar S., Anna University, IndiaRana Yassir Hussain, University of Education Lahore, Pakistan
Sonia Kumari, Sukkur IBA University, Pakistan
Copyright © 2022 An, Xu, Liu, Ma, Zhang and Tao. This is an open-access article distributed under the terms of the Creative Commons Attribution License (CC BY). The use, distribution or reproduction in other forums is permitted, provided the original author(s) and the copyright owner(s) are credited and that the original publication in this journal is cited, in accordance with accepted academic practice. No use, distribution or reproduction is permitted which does not comply with these terms.
*Correspondence: Ning Tao, zflningning@sina.com; Dajun Zhang, 365388016@qq.com
†These authors have contributed equally to this work and share first authorship