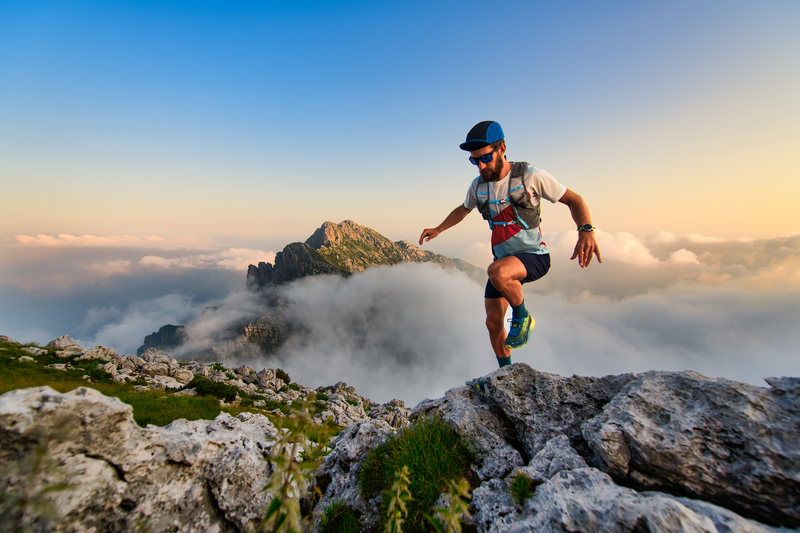
94% of researchers rate our articles as excellent or good
Learn more about the work of our research integrity team to safeguard the quality of each article we publish.
Find out more
METHODS article
Front. Psychol. , 04 February 2022
Sec. Emotion Science
Volume 12 - 2021 | https://doi.org/10.3389/fpsyg.2021.800657
This article is part of the Research Topic Methods and Applications in Emotion Science View all 9 articles
Android robots capable of emotional interactions with humans have considerable potential for application to research. While several studies developed androids that can exhibit human-like emotional facial expressions, few have empirically validated androids’ facial expressions. To investigate this issue, we developed an android head called Nikola based on human psychology and conducted three studies to test the validity of its facial expressions. In Study 1, Nikola produced single facial actions, which were evaluated in accordance with the Facial Action Coding System. The results showed that 17 action units were appropriately produced. In Study 2, Nikola produced the prototypical facial expressions for six basic emotions (anger, disgust, fear, happiness, sadness, and surprise), and naïve participants labeled photographs of the expressions. The recognition accuracy of all emotions was higher than chance level. In Study 3, Nikola produced dynamic facial expressions for six basic emotions at four different speeds, and naïve participants evaluated the naturalness of the speed of each expression. The effect of speed differed across emotions, as in previous studies of human expressions. These data validate the spatial and temporal patterns of Nikola’s emotional facial expressions, and suggest that it may be useful for future psychological studies and real-life applications.
Emotional interactions with other people are important for wellbeing (Keltner and Kring, 1998) but difficult to investigate in controlled laboratory experiments. While numerous psychological studies have presented pre-recorded photographs or videos of emotional expressions to participants and reported interesting findings regarding the psychological processes underlying emotional interactions (e.g., Dimberg, 1982), this method may lack the liveliness of real interactions, thus reducing ecological validity (Shamay-Tsoory and Mendelsohn, 2019; Hsu et al., 2020). Other studies used confederates as interaction partners and tested live emotional interactions (e.g., Vaughan and Lanzetta, 1980), but this strategy can lack rigorous control of confederates’ behaviors (Bavelas and Healing, 2013; Kuhlen and Brennan, 2013). Androids—that is, humanoid robots that exhibit appearances and behaviors that closely resemble those of humans (Ishiguro and Nishio, 2007)—could become an important tool for testing live face-to-face emotional interactions with rigorous control.
To implement emotional interaction in androids, the androids’ facial expressions must be carefully developed. Psychological studies have verified that facial expressions play a key role in transmitting information about emotional states in humans (Mehrabian, 1971). Studies of facial expressions developed methods for objectively evaluating facial actions (for a review, see Ekman, 1982), and the Facial Action Coding System (FACS; Ekman and Friesen, 1978; Ekman et al., 2002) is among the most refined of these methods. Based on observations of thousands of facial expressions in natural settings, together with a series of controlled psychological experiments, researchers identified the sets of facial action units (AUs) in the FACS corresponding to prototypical expressions of six basic emotions (Ekman and Friesen, 1975; Friesen and Ekman, 1983). For example, happy expressions involve an AU set consisting of the cheek raiser (AU 6) and lip corner puller (AU 12); surprised expressions involve the inner and outer brow raisers (AUs 1 and 2, respectively), the upper lid raiser (AU 5), and the jaw drop (AU 25). Numerous studies testing the recognition of photographs of facial expressions created based on this system verified that the expressions were recognized as the target emotional expressions above chance level across various cultures (e.g., Ekman and Friesen, 1971; for a review, see Ekman, 1993). Furthermore, the researchers described how the temporal aspects of dynamic emotional facial expressions are informative (Ekman and Friesen, 1975), which was supported by several subsequent experimental studies (for reviews, see Krumhuber et al., 2016; Dobs et al., 2018; Sato et al., 2019a). For example, Sato and Yoshikawa (2004) tested the naturalness ratings of dynamic changes in facial expressions and found that expressions that changed too slowly were generally rated as unnatural. Additionally, the effects of changing speeds differed across emotions, where fast and slow changes were regarded as relatively natural for surprised and sad expressions, respectively. Collectively, these psychological findings specify the spatial and temporal patterns of facial actions associated with facial expressions of emotions. Based on such findings, researchers have developed and validated novel research tools, including emotional facial expressions of virtual agents (Roesch et al., 2011; Krumhuber et al., 2012; Ochs et al., 2015). Virtual agents are promising tools to investigate emotional interactions with high ecological validity and control (Parsons, 2015; Pan and Hamilton, 2018). Androids may be comparably useful in this respect, and also have the unique advantage of being physically present (Li, 2015). If androids’ facial expressions can be developed and validated based on psychological evidence, they will constitute an important research tool for investigating emotional interactions.
However, although numerous studies have developed androids for emotional interactions (Kobayashi and Hara, 1993; Kobayashi et al., 2000; Minato et al., 2004, 2006, 2007; Weiguo et al., 2004; Ishihara et al., 2005; Matsui et al., 2005; Berns and Hirth, 2006; Blow et al., 2006; Hashimoto et al., 2006, 2008; Oh et al., 2006; Sakamoto et al., 2007; Lee et al., 2008; Takeno et al., 2008; Allison et al., 2009; Lin et al., 2009, 2016; Kaneko et al., 2010; Becker-Asano and Ishiguro, 2011; Ahn et al., 2012; Mazzei et al., 2012; Tadesse and Priya, 2012; Cheng et al., 2013; Habib et al., 2014; Yu et al., 2014; Asheber et al., 2016; Glas et al., 2016; Marcos et al., 2016; Faraj et al., 2021; Nakata et al., 2021; Table 1), few have empirically validated the androids that were developed. First, no study validated androids’ AUs coded using FACS (Ekman and Friesen, 1978; Ekman et al., 2002). Second, no study sufficiently demonstrated recognition of the six basic emotions conveyed by androids’ facial expressions. Many androids’ facial expressions were reportedly insufficiently developed to exhibit all six basic emotions (e.g., Minato et al., 2004). While several studies developed androids capable of exhibiting the six basic emotions, and recruited naïve participants to label the facial expressions, most did not statistically evaluate the accuracy (e.g., Kobayashi and Hara, 1993). One study conducted a statistical analysis that did not reveal significantly high level of recognition of disgust and fear (Berns and Hirth, 2006). Another study testing five basic emotions failed to observe better-than-chance recognition of fear (Becker-Asano and Ishiguro, 2011). Finally, no study systematically validated whether androids can show dynamic changes in facial expressions like humans. Only a few studies reported that incorporating the dynamic patterns of human facial expressions into an androids’ facial expressions led to high naturalness ratings of facial expressions during laughter (Ishi et al., 2019) and vocalized surprise (Ishi et al., 2017).
To resolve the issues described above, we developed an android head, called Nikola, and validated its facial actions and emotional expressions. Nikola has 35 actuators, designed to implement AUs relevant to prototypical facial expressions based on psychological evidence (Ekman and Friesen, 1975, 1978; Friesen and Ekman, 1983; Ekman et al., 2002). The temporal patterns of the actions can be programmed at a resolution of milliseconds. We conducted a series of psychological studies to validate Nikola’s emotional facial expressions. In Study 1, we applied FACS coding to Nikola’s single AUs, which underlie appropriate emotional facial expressions. In Study 2, we evaluated emotional recognition accuracy based on the spatial patterns of Nikola’s emotional facial expressions through an emotion labeling task. In Study 3, we evaluated the temporal patterns of Nikola’s dynamic facial expressions through a naturalness rating task.
Here, we used FACS coding for Nikola’s single facial actions. We expected that AUs specifically associated with the facial expressions corresponding to the six basic emotions to be produced.
Nikola was developed for the purpose of studying emotional interaction with humans. Currently, only the head and neck are complete; the body parts are under construction. It is human-like in appearance, similar to a male human child; it resembles a child to promote natural interactions with both adults and children. It is about 28.5 cm high and weighs about 4.6 kg. It has 35 actuators: 29 for facial muscle actions, 3 for head movement (roll, pitch, and yaw rotation), and 3 for eyeball control (pan movements of the individual eyeballs and tilt movements of both eyeballs). The facial and head movements are driven by pneumatic (air) actuators, which create safe, silent, and human-like motions (Ishiguro and Nishio, 2007; Minato et al., 2007). The pneumatic actuators are controlled by an air pressure control valve. The entire surface, except for the back of the head, is covered in a soft silicone skin. Video cameras are mounted inside the left and right eyeballs. Nikola is not a stand-alone system; the control valves, air compressor, and computer for controlling the actuators and sensor information processing are external.
The facial muscle actuators’ locations were selected to produce as many AUs as possible, specifically those associated with emotional facial expressions (Ekman and Friesen, 1975, 1978; Friesen and Ekman, 1983; Ekman et al., 2002), together with the information provided by previously constructed androids (Minato et al., 2004, 2006, 2007; Matsui et al., 2005; Glas et al., 2016). Specifically, we designed Nikola to produce the following AUs corresponding to the emotional expressions associated with six basic emotions: 1 (inner brow raiser), 2 (outer brow raiser), 4 (brow lowerer), 5 (upper lid raiser), 6 (cheek raiser), 7 (lid tightener), 10 (upper lip raiser), 12 (lip corner puller), 15 (lip corner depressor), 20 (lip stretcher), 25 (lips part), and 26 (jaw drop). Although AUs 9 (nose wrinkler), 17 (chin raiser), and 23 (lip tightener) are reportedly relevant to prototypical facial expressions (Ekman and Friesen, 1975; Friesen and Ekman, 1983), these AUs were not implemented owing to the technical limitations of the silicone skin. AUs 14 (dimpler), 16 (lower lip depressor), 18 (lip pucker), 22 (lip funneler), and 43 (eyes closed) were also designed to implement other communication-related facial actions (e.g., speech and blinking).
We programmed Nikola to exhibit AUs on an individual basis. A certified FACS coder scored the AUs from the neutral status to the action apex using FACS (Ekman et al., 2002). When the AU was detected, the coder evaluated it according to five discrete levels of intensity (A: trace, B: slight, C: marked/pronounced, D: severe, and E: extreme/maximum) according to FACS guidelines (Ekman et al., 2002). The coder could view the sequence repeatedly by adjusting the program settings. The Supplementary Material provides video clips of these AUs.
The AUs produced by Nikola are illustrated in Figure 1, and the results of the FACS coding are presented in Table 2. Figure 1 demonstrates that Nikola is capable of performing each AU. It was difficult to distinguish between AUs 6 (cheek raiser) and 7 (lid tightener), but the eyes’ outer corners were slightly lowered in AU 6. The maximum intensity of the AUs ranged from A (e.g., AU 12) to E (e.g., AU 26).
Figure 1. Illustrations of the facial action units (AUs) produced by the android Nikola. For AU 25, AU 25 + 26 is shown.
Our results demonstrated that Nikola was capable of producing each AU based on manual FACS coding performed by a certified FACS coder. The results are consistent with several earlier studies’ findings that androids could exhibit AUs designed based on FACS (e.g., Kobayashi and Hara, 1993), but none of these studies involved evaluation by certified FACS coders. The coder found it difficult to differentiate AUs 6 (cheek raiser) and 7 (lid tightener). This is in line with earlier findings that androids struggled to replicate z-vector movements, including wrinkles and tension, compared with human expressions (Ishihara et al., 2021), owing to the physical constraints of artificial skin materials. The results of our intensity evaluation revealed that some AUs’ maximum intensities were not realized. This resulted from technical limitations, such as an insufficient number of actuators and skin materials. Collectively, the data suggest that Nikola can produce AUs associated with prototypical facial expressions, albeit with limited intensity.
Next, we devised prototypical facial expressions for Nikola reflecting six basic emotions and asked naïve participants to label photographs of these expressions, as in earlier psychological studies using photographs of human facial expressions as stimuli (Sato et al., 2002, 2009; Kubota et al., 2003; Uono et al., 2011; Okada et al., 2015). Because earlier studies of human expression stimuli consistently demonstrated emotion recognition above the level of chance, as well as differences across emotions (such as lower recognition rates for angry, disgusted, and fearful expressions than happy, sad, and surprised expressions), we expected such patterns to be seen with respect to emotion recognition of Nikola’s facial expressions.
Thirty adult Japanese participants participated in this study (18 females; mean ± SD age, 36.0 ± 7.2 years). The sample size was determined based on an a priori power analysis using G*Power software ver. 3.1.9.2 (Faul et al., 2007). Assuming an α level of 0.008 (i.e., 0.05 Bonferroni-corrected for six tests), a power of 0.80, and a strong effect size (d = 0.8) based on an earlier study (Sato et al., 2002), the results indicated that 23 participants were required for a one-sample t-test. Participants were recruited through web advertisements distributed via CrowdWorks (Tokyo, Japan). After the procedures had been explained, all participants provided written informed consent to participate in the study, which was approved by the Ethics Committee of RIKEN. The experiment was performed in accordance with the Declaration of Helsinki.
Six photographs of facial expressions depicting the six basic emotions (anger, disgust, fear, happiness, sadness, and surprise) produced by Nikola were used as stimuli (Figure 2). The facial expressions were produced by activating the AUs according to the Emotional Facial Action Coding System (EMFACS; Friesen and Ekman, 1983). The activated AUs included 4, 5, 7, and 23 for anger; 15 for disgust; 1, 2, 4, 5, 7, 20, and 26 for fear; 6 and 12 for happiness; 1, 4, and 15 for sadness; and 1, 2, 5, and 26 for surprise. The facial expressions of the six basic emotions were photographed using a digital web camera (HD1080P; Logicool, Tokyo, Japan). The photographs were cropped to 630 horizontal × 720 vertical pixels.
Figure 2. Illustrations of the facial expressions of six basic emotions produced by the android Nikola.
The experiment was conducted via the Qualtrics online platform (Seattle, WA, United States). A label-matching paradigm was used, as in an earlier study (Sato et al., 2002). The photographs of Nikola’s facial expressions of the six basic emotions were presented on the monitor individually, and verbal labels for the six basic emotions were presented below each photograph. Participants were asked to select the label that best described the emotion shown in each photograph. No time limits were set, and no feedback on performance was provided. An image of each emotional expression was presented twice, pseudo-randomly, resulting in a total of 12 trials for each participant. Prior to the experiment, the participants performed two practice trials.
The data were analyzed using JASP 0.14.1 software (JASP Team, 2020). Accuracy percentages for emotion recognition were tested for the difference from chance (i.e., 16.7%) using one-sample t-tests (two-tailed) with the Bonferroni correction; the alpha level was divided by the number of tests performed (i.e., 6). The emotion recognition accuracy data were also subjected to repeated-measures analysis of variance (ANOVA) with emotion as a factor to test for differences among emotions. The assumption of sphericity was confirmed using Mauchly’s sphericity test (p > 0.10). Multiple comparisons were performed using Ryan’s method. All results were considered statistically significant at p < 0.05.
One-sample t-tests revealed that the accuracy percentage of emotionapl expression recognition for all emotion categories (Figure 3) was greater than chance, t(29) = 2.88, 4.74, 3.74, 14.58, 10.64, and 28.11; Bonferroni-corrected p = 0.042, 0.000, 0.007, 0.000, 0.000, and 0.000; Cohen’s d = 0.90, 1.24, 1.04, 3.27, 2.44, and 6.23 for anger, disgust, fear, happiness, sadness, and surprise, respectively.
Figure 3. Mean (±SE) accuracy percentages for the recognition of six emotions in facial expressions in Study 2.
The ANOVA with emotion as a factor for recognition accuracy revealed a significant main effect of emotion, F(5, 145) = 15.94, p = 0.000, η2p = 0.36. Multiple comparisons indicated that surprised, sad, and happy expressions were recognized with greater accuracy than disgusted, fearful, and angry expressions, t(245) > 3.21, p < 0.005.
Our findings indicated that the emotion recognition accuracy of Nikola’s facial expressions for all six basic emotions was above chance level. These results are consistent with earlier studies reporting that participants could recognize emotions from the facial expressions of androids, although the studies either did not determine whether recognition accuracy was better than chance (e.g., Kobayashi and Hara, 1993) or failed to find significantly higher recognition than chance for some emotions (Berns and Hirth, 2006; Becker-Asano and Ishiguro, 2011). Additionally, the results revealed differences in the accuracy of emotional recognition across emotional categories, with better recognition seen for happy, sad, and surprised expressions than for angry, disgusted, and fearful expressions. The results are consistent with earlier studies on emotion recognition using human facial expression stimuli (e.g., Uono et al., 2011). Compared with earlier studies using human stimuli, the overall emotion recognition percentage using photographs of Nikola as stimuli was low [e.g., 98.2 and 90.0% recognition accuracy for happy expressions of humans (Uono et al., 2011) and Nikola, respectively]. We speculate that this discrepancy was mainly attributable to low facial expression intensity for Nikola. Overall, the results indicate that Nikola can accurately exhibit emotional facial expressions of six basic emotions using a combination of AUs (Friesen and Ekman, 1983), although expression intensity is weak relative to human expressions.
In Study 3, we systematically changed the speed of Nikola’s dynamic facial expressions and asked naïve participants to evaluate the naturalness of the expressions’ speed, as in earlier psychological studies that used the dynamic stimuli of human facial expressions (Sato and Yoshikawa, 2004; Sato et al., 2013). Earlier studies that used human stimuli consistently reported that facial expressions that changed too slowly were generally rated as unnatural. Additionally, the effects of changing speeds differed across emotions, such that fast changes could be perceived as relatively natural for surprised expressions while slow changes were perceived as natural for sad expressions. We expected similar emotion-general and emotion-specific patterns for Nikola’s dynamic facial expressions.
Thirty adult Japanese participants took part in this study (19 females; mean ± SD age, 37.0 ± 7.4 years). As in Study 2, the sample size was determined based on an a priori power analysis using G*Power software ver. 3.1.9.2 (Faul et al., 2007). Assuming an α level of 0.05, a power of 0.80, and a medium effect size (f = 0.25), the results indicated that 24 participants were required for the planned trend analyses (four levels). Participants were recruited through web advertisements distributed via CrowdWorks (Tokyo, Japan). After the procedures had been explained, all participants provided written informed consent to participate in the study, which was approved by the Ethics Committee of RIKEN. The experiment was performed in accordance with the Declaration of Helsinki.
A total of 24 videotapes of dynamic facial expressions produced by Nikola, depicting six basic emotions (anger, disgust, fear, happiness, sadness, and surprise), from onset (neutral face) to action apex (full emotional expression) at four speeds (total durations of 250, 500, 1,000, and 2,000 ms) were used as stimuli (Figure 4). The four speed conditions used in previous studies (Sato and Yoshikawa, 2004; Sato et al., 2013) were also employed herein to allow comparison of the findings between humans and androids. The utility of these speeds was also supported by our preliminary encoding study (some data were reported in Sato et al., 2019b), in which we videotaped emotional facial expressions produced in response to various scenarios and found that most expressions were produced within 250–2,000 ms. Similar data (production durations of 220–1,540 ms) were reported by a different group (Fiorentini et al., 2012). A decoding study reported that the presentation of dynamic facial expressions for 180, 780, and 3,030 ms produced divergent free-response recognition of facial expressions (Kamachi et al., 2001). As in Study 2, the AUs of emotional facial expressions were determined according to EMFACS (Friesen and Ekman, 1983). All AUs were controlled simultaneously. The facial expressions were video-recorded using a digital web camera (HD1080P; Logitech, Tokyo, Japan). The Supplementary Material provides video clips of these dynamic facial expression stimuli.
Figure 4. Illustration of the dynamic facial expression stimuli used in Study 3. (Left) Nikola’s face changed from a neutral expression to one of six emotional expressions. (Right) Schematic illustration of the four speed conditions.
As in Study 2, the experiment was conducted via the online Qualtrics platform (Seattle, WA, United States). The naturalness of dynamic changes in emotional facial expressions was rated, as in an earlier study (Sato and Yoshikawa, 2004). In each trial, four video clips of Nikola’s facial expressions of one of six basic emotions, at different speeds, were presented on the monitor one by one. The speed conditions were presented in randomized order, and the interval between each clip was 1,500 ms. Participants were provided with the target emotion label and instructed to evaluate each clip in terms of the naturalness of the speed with which the particular emotion changed, using a 7-point scale ranging from 1 (not at all natural) to 7 (very natural). No time limits were set, and participants were allowed to view the sequence repeatedly (by clicking a button) until they were satisfied with their ratings. Each emotion condition was presented twice in pseudo-randomized order, resulting in a total of 12 trials for each participant. Prior to the experiment, participants performed two practice trials.
As in Study 2, the data were analyzed using JASP 0.14.1 software (JASP Team, 2020). The naturalness ratings were analyzed by repeated-measures ANOVA, with emotion (anger, disgust, fear, happiness, sadness, and surprise) and speed (total duration of 250, 500, 1,000, and 2,000 ms) as within-subjects factors. Because the assumption of sphericity was not met (Mauchly’s sphericity test, p < 0.05), the Huynh–Feldt correction was applied. Follow-up trend analyses were conducted on the effect of speed, to derive profiles of the changes in ratings across speed conditions. All results were considered statistically significant at p < 0.05.
The ANOVA for the naturalness ratings (Figure 5), with emotion and speed as within-subjects factors, revealed a significant main effect of speed, F(1.52, 44.14) = 12.62, p = 0.000, and η2p = 0.30. The interaction between emotion and speed was also significant, F(7.42, 215.30) = 9.45, p = 0.000, and η2p = 0.25. The main effect of emotion was not significant, F(3.05, 88.40) = 0.84, p = 0.476, and η2p = 0.03. Follow-up trend analyses of the main effect of speed indicated significant negative linear (i.e., faster changes were more natural) and quadratic (i.e., intermediate changes were the most natural) trends as a function of speed, t(87) = 3.98 and 4.68, respectively, ps = 0.000.
Figure 5. Mean (±SE) naturalness ratings for facial expressions of six emotions under the four speed conditions in Study 3.
For the significant interaction, simple trend analyses of the speed effect were conducted for each emotion. For anger, disgust, and fear, the linear and quadratic negative trends as a function of speed were significant, t(87) = 4.21, 5.09, 5.29, 2.01, 2.78, and 3.52; p = 0.000, 0.000, 0.000, 0.048, 0.006, and 0.000, for anger-linear, anger-quadratic, disgust-linear, disgust-quadratic, fear-linear, and fear-quadratic, respectively. Only the negative quadratic trend was significant for happiness, t(87) = 4.94, p = 0.000. For sadness, the positive linear (i.e., slower changes were more natural) and negative quadratic trends were significant, t(87) = 3.72 and 2.94, p = 0.000 and 0.004, respectively. For surprise, only the negative linear trend reached significance, t(87) = 6.67, p = 0.000.
The results indicated that the naturalness ratings for dynamic changes in Nikola’s emotional facial expressions generally decreased with reduced speed of change. The results also revealed differences across emotions; for example, the ratings linearly decreased and increased depending on speed for surprised and sad expressions, respectively. These results are consistent with those of earlier studies that used dynamic human facial expressions (Sato and Yoshikawa, 2004; Sato et al., 2013). The results are also in line with studies showing that an android exhibiting dynamic facial expressions with the same temporal patterns as human facial expressions was rated as more natural than an android that did not exhibit such expressions (Ishi et al., 2017, 2019). Our results demonstrate that the temporal aspects of Nikola’s facial expressions can transmit emotional messages, similar to those of humans.
In summary, the results of Study 1 confirmed that Nikola can produce AUs associated with prototypical facial expressions. Study 2 verified that Nikola can exhibit facial expressions of six basic emotions that can be accurately recognized by naïve participants. The results of Study 3 revealed that Nikola can exhibit dynamic facial expressions with temporal patterns that transmit emotional messages, as in human facial expressions. Collectively, these results support the validity of the spatial and temporal characteristics of the emotional facial expressions of our new android.
These results have practical implications. First, in terms of basic research, androids like Nikola represent important tools for psychological experiments examining face-to-face emotional interactions with high ecological validity and control. Several methods have been employed to conduct such experiments, each of which has specific advantages and disadvantages. Most studies in the literature have presented pre-recorded photographs or videos of others’ emotional expressions (e.g., Dimberg, 1982). Although this method provides a high level of control, its ecological validity is not particularly high (for a review, see Shamay-Tsoory and Mendelsohn, 2019); a recent study indicated that subjective and physiological responses to pre-recoded videos of facial expressions differed from those to live facial expressions (Hsu et al., 2020). Live emotional interactions between two participants are ecologically valid (e.g., Bruder et al., 2012; Riehle et al., 2017; Golland et al., 2019); however, such interactions are difficult to control, and the correlational nature of this approach makes it difficult to establish causality in terms of psychological mechanisms. Confederates are commonly used in social psychology (e.g., Vaughan and Lanzetta, 1980); although this approach has high ecological validity, serious disadvantages include difficulty in controlling confederates’ non-verbal behaviors (for reviews, see Bavelas and Healing, 2013; Kuhlen and Brennan, 2013). Interactions with virtual agents may promote both ecological validity and control (Parsons, 2015; Pan and Hamilton, 2018); however, virtual agents are obviously not physically present, which may limit ecological validity to some degree. Several studies have reported that physically present robots elicited greater emotional responses than virtual agents (e.g., Bartneck, 2003; Fasola and Mataric, 2013; Li et al., 2019; for a review, see Li, 2015). Taken together, our data suggest that androids like Nikola, which are human-like in appearance and facial expressions, and can physically coexist with humans, are valuable research tools for ecologically valid and controlled research on facial emotional interaction. Moreover, like several other advanced androids (e.g., Glas et al., 2016; Ishi et al., 2017, 2019), Nikola has the ability to talk with prosody, which can facilitate multimodal emotional interactions (Paulmann and Pell, 2011). Androids can also utilize advanced artificial intelligence (for reviews, see Krumhuber et al., 2021; Namba et al., 2021) to sense and analyze human facial expressions. We expect that androids will be a valuable tool in future psychological research on human emotional interaction.
Second, regarding future applications to real-life situations, our results suggest that androids like Nikola have the potential to transmit emotional messages to humans, and in turn promote human wellbeing. Android interactions may be useful in a wide range of situations, including elder care, behavioral interventions, counseling, nursing, education, information desks, customer service, and entertainment. For example, an earlier study has reported that a humanoid robot, which was controlled by manipulators and exhibited facial expressions of various emotions, was effective in comforting lonely older people (Hoorn et al., 2016). The researchers found that the robot satisfied users’ needs for emotional bonding as a social entity, while retaining a sense of privacy as a machine (Hoorn et al., 2016). With regard to behavioral interventions, several studies showed that children with autism spectrum disorder preferred robots and androids to human therapists (e.g., Adams and Robinson, 2011; for a review, see Scassellati, 2007). We expect that increasing their ability for emotional interactions would enhance androids’ value in future real-life applications.
Our results also have theoretical implications. Our findings could be regarded as constructive support for psychological theories that certain configurations of AUs can indicate emotional facial expressions (Ekman and Friesen, 1975) and that temporal patterns of facial expressions might transmit emotional information (Sato and Yoshikawa, 2004). Other ideas regarding human emotional interactions may also be verifiable through android experiments. The construction of effective android software and hardware requires that the mechanisms of psychological theories be elucidated. We expect that this constructivist approach to developing and testing androids (Ishiguro and Nishio, 2007; Minato et al., 2007) will be a useful methodology for understanding the psychological mechanisms underlying human emotional interaction.
Some limitations of this study should be acknowledged. First, as described above, the number and intensity of Nikola’s AUs is not comparable with those of humans owing to technical limitations related to the number of actuators and skin materials. Specifically, because silicone skin does not possess elastic qualities comparable with human skin (Cabibihan et al., 2009), creating natural wrinkles in Nikola’s face is difficult. Previous psychological studies have shown that nose wrinkling (i.e., AU 9) was associated with the recognition of disgust (Galati et al., 1997), while eye corner wrinkles (i.e., AU 6) improved the recognition of happy and sad expressions (Malek et al., 2019), suggesting the importance of wrinkles in emotional expressions. Future technical improvements will be required to realize richer and stronger emotional facial expressions.
Second, we used only controlled and explicit measures of the recognition of emotional facial expressions, including emotion labeling and naturalness ratings of speed changes; we did not measure automatic and/or reactive responses to facial expressions. Several previous studies have shown that emotional facial expressions induced stronger subjective (e.g., emotional arousal; Sato and Yoshikawa, 2007a) and physiological (e.g., activation of the sympathetic nervous system; Merckelbach et al., 1989) emotional reactions compared with non-facial stimuli. Other studies reported that observing emotional facial expressions automatically induced facial mimicry (e.g., Dimberg, 1982). Because Nikola’s eyeballs contain video cameras, it may be possible to videorecord participants’ faces to reveal externally observable facial mimicry, which cannot be accomplished in human confederates without specialized devices (Sato and Yoshikawa, 2007b). Investigation of these automatic and reactive measures represents a key avenue for future research.
Third, we only tested the temporal patterns of Nikola’s facial expressions in Study 3, by manipulating speed at four levels; thus, the optimal temporal characteristics of Nikola’s dynamic facial expressions remain to be identified. A previous psychophysical study has investigated this issue using generative approaches (Jack et al., 2014). The researchers presented participants with a large number of dynamic facial expressions of virtual agents with randomly selected AU sets and temporal parameters (e.g., acceleration) and asked them to identify the emotions being displayed. Mathematical modeling revealed the optimal spatial and temporal characteristics of facial expressions of various emotions. Research using similar data-driven approaches could reveal more fine-grained temporal, as well as spatial, characteristics of the dynamic facial expressions of Nikola.
Finally, although we constructed Nikola’s facial expressions according to basic emotion theory (Ekman and Friesen, 1975), the relationships between facial expressions and psychological states can be investigated from various perspectives. For example, Russell (1995, 1997) has proposed that facial expressions are associated not with basic emotions, but rather with core affective dimensions of valence and arousal. Fridlund and his colleagues proposed that facial expressions indicate not emotional states, but rather social messages (Fridlund, 1991; Crivelli and Fridlund, 2018). Investigation of these perspectives on facial expressions using androids is a key topic for future research.
The original contributions presented in the study are included in the article/Supplementary Material, further inquiries can be directed to the corresponding author.
The studies involving human participants were reviewed and approved by the RIKEN. The patients/participants provided their written informed consent to participate in this study.
WS and TM designed the research. WS, SNa, DY, SNi, and TM obtained the data. WS and SNa analyzed the data. WS, SNa, DY, SNi, CI, and TM wrote the manuscript. All authors contributed to the article and approved the submitted version.
SNi was employed by the company Nippon Telegraph and Telephone Corporation.
The remaining authors declare that the research was conducted in the absence of any commercial or financial relationships that could be construed as a potential conflict of interest.
All claims expressed in this article are solely those of the authors and do not necessarily represent those of their affiliated organizations, or those of the publisher, the editors and the reviewers. Any product that may be evaluated in this article, or claim that may be made by its manufacturer, is not guaranteed or endorsed by the publisher.
The authors thank Kazusa Minemoto and Saori Namba for their technical support.
The Supplementary Material for this article can be found online at: https://www.frontiersin.org/articles/10.3389/fpsyg.2021.800657/full#supplementary-material
Supplementary Figure 1 | Video clips of facial action units (AUs).
Supplementary Figure 2 | Video clips of the dynamic facial expression stimuli used in Study 3.
Supplementary Data 1 | Datasheet for Studies 2 and 3.
Adams, A., and Robinson, P. (2011). “An android head for social-emotional intervention for children with autism spectrum conditions,” in Proceedings of the 4th International Conference on Affective Computing and Intelligent Interaction, ACII 2011, Memphis, TN, doi: 10.1007/978-3-642-24571-8_19
Ahn, H. S., Lee, D. W., Choi, D., Lee, D. Y., Hur, M., and Lee, H. (2012). “Appropriate emotions for facial expressions of 33-DOFs android head EveR-4 H33,” in Proceedings of the 2012 IEEE RO-MAN: The 21st IEEE International Symposium on Robot and Human Interactive Communication, Paris, doi: 10.1109/ROMAN.2012.6343898
Allison, B., Nejat, G., and Kao, E. (2009). The design of an expressive humanlike socially assistive robot. J. Mech. Robot. 1:011001. doi: 10.1115/1.2959097
Asheber, W. T., Lin, C.-Y., and Yen, S. H. (2016). Humanoid head face mechanism with expandable facial expressions. Intern. J. Adv. Robot. Syst. 13:29. doi: 10.5772/62181
Bartneck, C. (2003). “Interacting with an embodied emotional character,” in Proceedings of the 2003 International Conference on Designing Pleasurable Products and Interfaces (DPPI2003), Pittsburgh, PA, doi: 10.1145/782896.782911
Bavelas, J., and Healing, S. (2013). Reconciling the effects of mutual visibility on gesturing: a review. Gesture 13, 63–92. doi: 10.1075/gest.13.1.03bav
Becker-Asano, C., and Ishiguro, H. (2011). Intercultural differences in decoding facial expressions of the android robot Geminoid F. J. Artific. Intellig. Soft Comput. Res. 1, 215–231.
Berns, K., and Hirth, J. (2006). “Control of facial expressions of the humanoid robot head ROMAN,” in Proceedings of the 2006 IEEE/RSJ International Conference on Intelligent Robots and Systems, Beijing, doi: 10.1109/IROS.2006.282331
Blow, M., Dautenhahn, K., Appleby, A., Nehaniv, C. L., and Lee, D. (2006). “The art of designing robot faces: dimensions for human-robot interaction,” in Proceedings of the HRI ’06: 1st ACM SIGCHI/SIGART Conference on Human-Robot Interaction, Salt Lake City, UT, doi: 10.1145/1121241.1121301
Bruder, M., Dosmukhambetova, D., Nerb, J., and Manstead, A. S. (2012). Emotional signals in nonverbal interaction: dyadic facilitation and convergence in expressions, appraisals, and feelings. Cogn. Emot. 26, 480–502. doi: 10.1080/02699931.2011.645280
Cabibihan, J. J., Pattofatto, S., Jomâa, M., Benallal, A., and Carrozza, M. C. (2009). Towards humanlike social touch for sociable robotics and prosthetics: comparisons on the compliance, conformance and hysteresis of synthetic and human fingertip skins. Intern. J. Soc. Robot. 1, 29–40. doi: 10.1007/s12369-008-0008-9
Cheng, L. C., Lin, C. Y., and Huang, C. C. (2013). Visualization of facial expression deformation applied to the mechanism improvement of face robot. Intern. J. Soc. Robot. 5, 423–439. doi: 10.1007/s12369-012-0168-5
Crivelli, C., and Fridlund, A. J. (2018). Facial displays are tools for social influence. Trends Cogn. Sci. 22, 388–399. doi: 10.1016/j.tics.2018.02.006
Dimberg, U. (1982). Facial reactions to facial expressions. Psychophysiology 19, 643–647. doi: 10.1111/j.1469-8986.1982.tb02516.x
Dobs, K., Bülthoff, I., and Schultz, J. (2018). Use and usefulness of dynamic face stimuli for face perception studies-a review of behavioral findings and methodology. Front. Psychol. 9:1355. doi: 10.3389/fpsyg.2018.01355
Ekman, P. (1982). “Methods for measuring facial action,” in Handbook of Methods in Nonverbal Behavior Research, eds K. R. Scherer and P. Ekman (Cambridge: Cambridge University Press), 45–90.
Ekman, P. (1993). Facial expression and emotion. Am. Psychol. 48, 384–392. doi: 10.1037//0003-066x.48.4.384
Ekman, P., and Friesen, W. V. (1971). Constants across cultures in the face and emotion. J. Pers. Soc. Psychol. 17, 124–129. doi: 10.1037/h0030377
Ekman, P., and Friesen, W. V. (1975). Unmasking the Face: A Guide to Recognizing Emotions from Facial Clues. Englewood Cliffs, NJ: Prentice-Hall.
Ekman, P., and Friesen, W. V. (1978). Facial Action Coding System: Consulting Psychologist. Palo Alto, CA: Consulting Psychologists Press.
Ekman, P., Friesen, W. V., and Hager, J. C. (2002). Facial Action Coding System. Salt Lake City, UT: Research Nexus, Network Research Information.
Faraj, Z., Selamet, M., Morales, C., Torres, P., Hossain, M., Chen, B., et al. (2021). Facially expressive humanoid robotic face. HardwareX 9:e00117. doi: 10.1016/j.ohx.2020.e00117
Fasola, S., and Mataric, M. J. (2013). A socially assistive robot exercise coach for the elderly. J. Hum. Robot Interact. 2, 3–32. doi: 10.5898/JHRI.2.2.Fasola
Faul, F., Erdfelder, E., Lang, A. G., and Buchner, A. (2007). G*Power 3: a flexible statistical power analysis program for the social, behavioral, and biomedical sciences. Behav. Res. Methods 39, 175–191. doi: 10.3758/bf03193146
Fiorentini, C., Schmidt, S., and Viviani, P. (2012). The identification of unfolding facial expressions. Perception 41, 532–555. doi: 10.1068/p7052
Fridlund, A. J. (1991). Evolution and facial action in reflex, social motive, and paralanguage. Biol. Psychol. 32, 3–100. doi: 10.1016/0301-0511(91)90003-y
Friesen, W., and Ekman, P. (1983). EMFACS-7: Emotional Facial Action Coding System. California: University of California.
Galati, D., Scherer, K. R., and Ricci-Bitti, P. E. (1997). Voluntary facial expression of emotion: comparing congenitally blind with normally sighted encoders. J. Pers. Soc. Psychol. 73, 1363–1379. doi: 10.1037/0022-3514.73.6.1363
Glas, D. F., Minato, C., Ishi, T., Kawahara, T., and Ishiguro, H. (2016). “ERICA: the ERATO intelligent conversational android,” in Proceedings of the 2016 25th IEEE International Symposium on Robot and Human Interactive Communication (RO-MAN), New York, NY, doi: 10.1109/ROMAN.2016.7745086
Golland, Y., Mevorach, D., and Levit-Binnun, N. (2019). Affiliative zygomatic synchrony in co-present strangers. Sci. Rep. 9:3120. doi: 10.1038/s41598-019-40060-4
Habib, A., Das, S. K., Bogdan, I., Hanson, D., and Popa, D. O. (2014). “Learning human-like facial expressions for android Phillip K. Dick,” in Proceedings of the 2014 IEEE International Conference on Automation Science and Engineering (CASE), New Taipei, doi: 10.1109/CoASE.2014.6899473
Hashimoto, T., Hiramatsu, S., and Kobayashi, H. (2008). “Dynamic display of facial expressions on the face robot made by using a life mask,” in Proceedings of the Humanoids 2008 - 8th IEEE-RAS International Conference on Humanoid Robots, Daejeon, doi: 10.1109/ICHR.2008.4756017
Hashimoto, T., Hitramatsu, S., Tsuji, T., and Kobayashi, H. (2006). “Development of the face robot SAYA for rich facial expressions,” in Proceedings of the 2006 SICE-ICASE, International Joint Conference, Busan, doi: 10.1109/SICE.2006.315537
Hoorn, J. F., Konijn, E. A., Germans, D. M., Burger, S., and Munneke, A. (2016). “The in-between machine: the unique value proposition of a robot or why we are modelling the wrong things,” in Proceedings of the 7th International Conference on Agents and Artificial Intelligence (ICAART), Lisbon, doi: 10.5220/0005251304640469
Hsu, C. T., Sato, W., and Yoshikawa, S. (2020). Enhanced emotional and motor responses to live versus videotaped dynamic facial expressions. Sci. Rep. 10:16825. doi: 10.1038/s41598-020-73826-2
Ishi, C. T., Minato, T., and Ishiguro, H. (2017). Motion analysis in vocalized surprise expressions and motion generation in android robots. IEEE Robot. Autom. Lett. 2, 1748–1754. doi: 10.1109/LRA.2017.2700941
Ishi, C. T., Minato, T., and Ishiguro, H. (2019). Analysis and generation of laughter motions, and evaluation in an android robot. APSIPA Trans. Signal Inform. Process. 8:e6. doi: 10.1017/ATSIP.2018.32
Ishiguro, H., and Nishio, S. (2007). Building artificial humans to understand humans. J. Artific. Organs 10, 133–142. doi: 10.1007/s10047-007-0381-4
Ishihara, H., Iwanaga, S., and Asada, M. (2021). Comparison between the facial flow lines of androids and humans. Front. Robot. AI 8:540193. doi: 10.3389/frobt.2021.540193
Ishihara, H., Yoshikawa, Y., and Asada, M. (2005). “Realistic child robot “Affetto” for understanding the caregiver-child attachment relationship that guides the child development,” in Proceedings of the 2011 IEEE International Conference on Development and Learning (ICDL), Frankfurt am Main, doi: 10.1109/DEVLRN.2011.6037346
Jack, R. E., Garrod, O. G., and Schyns, P. G. (2014). Dynamic facial expressions of emotion transmit an evolving hierarchy of signals over time. Curr. Biol. 24, 187–192. doi: 10.1016/j.cub.2013.11.064
Kamachi, M., Bruce, V., Mukaida, S., Gyoba, J., Yoshikawa, S., and Akamatsu, S. (2001). Dynamic properties influence the perception of facial expressions. Perception 30, 875–887. doi: 10.1068/p3131
Kaneko, K., Kanehiro, F., Morisawa, M., Miura, K., Nakaoka, S., Harada, K., et al. (2010). Development of cybernetic human “HRP-4C”-Project overview and design of mechanical and electrical systems. J. Robot. Soc. Jpn. 28, 853–864. doi: 10.7210/jrsj.28.853
Keltner, D., and Kring, A. M. (1998). Emotion, social function, and psychopathology. Rev. Gen. Psychol. 2, 320–342. doi: 10.1037//0021-843x.104.4.644
Kobayashi, H., and Hara, F. (1993). “Study on face robot for active human interface-mechanisms of face robot and expression of 6 basic facial expressions,” in Proceedings of the 1993 2nd IEEE International Workshop on Robot and Human Communication, Tokyo, doi: 10.1109/ROMAN.1993.367708
Kobayashi, H., Tsuji, T., and Kikuchi, K. (2000). “Study of a face robot platform as a kansei medium,” in Proceedings of the 2000 26th Annual Conference of the IEEE Industrial Electronics Society, Nagoya, doi: 10.1109/IECON.2000.973197
Krumhuber, E. G., Küster, D., Namba, S., and Skora, L. (2021). Human and machine validation of 14 databases of dynamic facial expressions. Behav. Res. Methods 53, 686–701. doi: 10.3758/s13428-020-01443-y
Krumhuber, E. G., Skora, L., Küster, D., and Fou, L. (2016). A review of dynamic datasets for facial expression research. Emot. Rev. 9, 280–292. doi: 10.1177/1754073916670022
Krumhuber, E. G., Tamarit, L., Roesch, E. B., and Scherer, K. R. (2012). FACSGen 2.0 animation software: generating three-dimensional FACS-valid facial expressions for emotion research. Emotion 12, 351–363. doi: 10.1037/a0026632
Kubota, Y., Quérel, C., Pelion, F., Laborit, J., Laborit, M. F., Gorog, F., et al. (2003). Facial affect recognition in pre-lingually deaf people with schizophrenia. Schizophr. Res. 61, 265–270. doi: 10.1016/s0920-9964(02)00298-0
Kuhlen, A. K., and Brennan, S. E. (2013). Language in dialogue: when confederates might be hazardous to your data. Psychon. Bull. Rev. 20, 54–72. doi: 10.3758/s13423-012-0341-8
Lee, D. W., Lee, T. G., So, B., Choi, M., Shin, E. C., Yang, K. W., et al. (2008). “Development of an android for emotional expression and human interaction,” in Proceedings of the 17th World Congress The International Federation of Automatic Control, Seoul, doi: 10.3182/20080706-5-KR-1001.2566
Li, J. (2015). The benefit of being physically present: a survey of experimental works comparing copresent robots, telepresent robots and virtual agents. Intern. J. Hum. Comput. Stud. 77, 23–37. doi: 10.1016/j.ijhcs.2015.01.001
Li, R., van Almkerk, M., van Waveren, S., Carter, E., and Leite, I. (2019). “Comparing human-robot proxemics between virtual reality and the real world,” in Proceedings of the 2019 14th ACM/IEEE International Conference on Human-Robot Interaction (HRI), Daegu, doi: 10.1109/HRI.2019.8673116
Lin, C., Huang, C., and Cheng, L. (2016). An expressional simplified mechanism in anthropomorphic face robot design. Robotica 34, 652–670. doi: 10.1017/S0263574714001787
Lin, C., Tseng, C., Teng, W., Lee, W., Kuo, C., Gu, H., et al. (2009). “The realization of robot theater: humanoid robots and theatric performance,” in Proceedings of the 2009 International Conference on Advanced Robotics, Munich.
Malek, N., Messinger, D., Gao, A. Y. L., Krumhuber, E., Mattson, W., Joober, R., et al. (2019). Generalizing Duchenne to sad expressions with binocular rivalry and perception ratings. Emotion 19, 234–241. doi: 10.1037/emo0000410
Marcos, S., Pinillos, R., García-Bermejo, J. G., and Zalama, E. (2016). Design of a realistic robotic head based on action coding system. Adv. Intellig. Syst. Comput. 418, 423–434. doi: 10.1007/978-3-319-27149-1_33
Matsui, D., Minato, T., MacDorman, F., and Ishiguro, H. (2005). “Generating natural motion in an android by mapping human motion,” in Proceedings of the 2005 IEEE/RSJ International Conference on Intelligent Robots and Systems, Edmonton, Edmonton, AB, doi: 10.1109/IROS.2005.1545125
Mazzei, D., Lazzeri, N., Hanson, D., and De Rossi, D. (2012). “HEFES: an hybrid engine for facial expressions synthesis to control human-like androids and avatars,” in Proceedings of the 2012 4th IEEE RAS & EMBS International Conference on Biomedical Robotics and Biomechatronics (BioRob), Rome, doi: 10.1109/BioRob.2012.6290687
Mehrabian, A. (1971). “Nonverbal communication,” in Proceedings of the Nebraska Symposium on Motivation, 1971, ed. J. K. Cole (Lincoln, NE: University of Nebraska Press), 107–161.
Merckelbach, H., van Hout, W., van den Hout, M. A., and Mersch, P. P. (1989). Psychophysiological and subjective reactions of social phobics and normals to facial stimuli. Behav. Res. Therapy 27, 289–294. doi: 10.1016/0005-7967(89)90048-x
Minato, T., Shimada, M., Ishiguro, H., and Itakura, S. (2004). Development of an android robot for studying human-robot interaction. Innov. Appl. Artific. Intellig. 3029, 424–434. doi: 10.1007/978-3-540-24677-0_44
Minato, T., Shimada, M., Itakura, S., Lee, K., and Ishiguro, H. (2006). Evaluating the human likeness of an android by comparing gaze behaviors elicited by the android and a person. Adv. Robot. 20, 1147–1163. doi: 10.1163/156855306778522505
Minato, T., Yoshikawa, T., Noda, T., Ikemoto, S., and Ishiguro, H. (2007). “CB2: a child robot with biomimetic body for cognitive developmental robotics,” in Proceedings of the 2007 7th IEEE-RAS International Conference on Humanoid Robots, Pittsburgh, PA, doi: 10.1109/ICHR.2007.4813926
Nakata, Y., Yagi, S., Yu, S., Wang, Y., Ise, N., Nakamura, Y., et al. (2021). Development of ‘ibuki’ an electrically actuated childlike android with mobility and its potential in the future society. Robotica 2021, 1–18. doi: 10.1017/S0263574721000898
Namba, S., Sato, W., Osumi, M., and Shimokawa, K. (2021). Assessing automated facial action unit detection systems for analyzing cross-domain facial expression databases. Sensors 21:4222. doi: 10.3390/s21124222
Ochs, M., Niewiadomski, R., and Pelachaud, C. (2015). “Facial expressions of emotions for virtual characters,” in The Oxford Handbook of Affective Computing, eds R. A. Calvo, S. K. D’Mello, J. Gratch, and A. Kappas (New York, NY: Oxford University Press), 261–272.
Oh, J. H., Hanson, D., Kim, W. S., Han, I. Y., Kim, J. Y., and Park, I. W. (2006). “Design of android type humanoid robot: Albert HUBO,” in Proceedings of the 2006 IEEE/RSJ International Conference on Intelligent Robots and Systems, Beijing, doi: 10.1109/IROS.2006.281935
Okada, T., Kubota, Y., Sato, W., Murai, T., Pellion, F., and Gorog, F. (2015). Common impairments of emotional facial expression recognition in schizophrenia across French and Japanese cultures. Front. Psychol. 6:1018. doi: 10.3389/fpsyg.2015.01018
Pan, X., and Hamilton, A. F. D. C. (2018). Why and how to use virtual reality to study human social interaction: the challenges of exploring a new research landscape. Br. J. Psychol. 109, 395–417. doi: 10.1111/bjop.12290
Parsons, T. D. (2015). Virtual reality for enhanced ecological validity and experimental control in the clinical, affective and social neurosciences. Front. Hum. Neurosci. 9:660. doi: 10.3389/fnhum.2015.00660
Paulmann, S., and Pell, M. D. (2011). Is there an advantage for recognizing multi-modal emotional stimuli. Motiv. Emot. 35, 192–201. doi: 10.1007/s11031-011-9206-0
Riehle, M., Kempkensteffen, J., and Lincoln, T. M. (2017). Quantifying facial expression synchrony in face-to-face dyadic interactions: temporal dynamics of simultaneously recorded facial EMG signals. J. Nonverb. Behav. 41, 85–102. doi: 10.1007/s10919-016-0246-8
Roesch, E., Tamarit, L., Reveret, L., Grandjean, D. M., Sander, D., and Scherer, K. R. (2011). FACSGen: a tool to synthesize emotional facial expressions through systematic manipulation of facial action units. J. Nonverb. Behav. 35, 1–16. doi: 10.1007/s10919-010-0095-9
Russell, J. A. (1995). Facial expressions of emotion: what lies beyond minimal universality? Psychol. Bull. 118, 379–391. doi: 10.1037/0033-2909.118.3.379
Russell, J. A. (1997). Core affect and the psychological construction of emotion. Psychol. Rev. 110, 145–172. doi: 10.1037/0033-295x.110.1.145
Sakamoto, D., Kanda, T., Ono, T., Ishiguro, H., and Hagita, N. (2007). “Android as a telecommunication medium with a human-like presence,” in Proceedings of the 2007 2nd ACM/IEEE International Conference on Human-Robot Interaction (HRI), Arlington, VA, doi: 10.1145/1228716.1228743
Sato, W., Hyniewska, S., Minemoto, K., and Yoshikawa, S. (2019a). Facial expressions of basic emotions in Japanese laypeople. Front. Psychol. 10:259. doi: 10.3389/fpsyg.2019.00259
Sato, W., Krumhuber, E. G., Jellema, T., and Williams, J. (2019b). Editorial: dynamic emotional communication. Front. Psychol. 10:2836. doi: 10.3389/fpsyg.2019.02836
Sato, W., Kubota, Y., Okada, T., Murai, T., Yoshikawa, S., and Sengoku, A. (2002). Seeing happy emotion in fearful and angry faces: qualitative analysis of the facial expression recognition in a bilateral amygdala damaged patient. Cortex 38, 727–742. doi: 10.1016/s0010-9452(08)70040-6
Sato, W., Uono, S., Matsuura, N., and Toichi, M. (2009). Misrecognition of facial expressions in delinquents. Child Adolesc. Psychiatry Ment. Health 3:27. doi: 10.1186/1753-2000-3-27
Sato, W., Uono, S., and Toichi, M. (2013). Atypical recognition of dynamic changes in facial expressions in autism spectrum disorders. Res. Autism Spectr. Disord. 7, 906–912. doi: 10.1016/j.bpsc.2020.09.006
Sato, W., and Yoshikawa, S. (2004). The dynamic aspects of emotional facial expressions. Cogn. Emot. 18, 701–710. doi: 10.1080/02699930341000176
Sato, W., and Yoshikawa, S. (2007a). Enhanced experience of emotional arousal in response to dynamic facial expressions. J. Nonverb. Behav. 31, 119–135. doi: 10.1007/s10919-007-0025-7
Sato, W., and Yoshikawa, S. (2007b). Spontaneous facial mimicry in response to dynamic facial expressions. Cognition 104, 1–18. doi: 10.1016/j.cognition.2006.05.001
Scassellati, B. (2007). “How social robots will help us to diagnose, treat, and understand autism,” in Robotics Research. Springer Tracts in Advanced Robotics, eds S. Thrun, H. Durrant-Whyte, and R. Brooks (Berlin: Springer), 552–563. doi: 10.1007/978-3-540-48113-3_47
Shamay-Tsoory, S. G., and Mendelsohn, A. (2019). Real-life neuroscience: an ecological approach to brain and behavior research. Perspect. Psychol. Sci. 14, 841–859. doi: 10.1177/1745691619856350
Tadesse, Y., and Priya, S. (2012). Graphical facial expression analysis and design method: an approach to determine humanoid skin deformation. J. Mech. Robot. 4:021010. doi: 10.1115/1.4006519
Takeno, J., Mori, K., and Naito, Y. (2008). “Robot consciousness and representation of facial expressions,” in Proceedings of the 2008 3rd International Conference on Sensing Technology, Taipei, doi: 10.1109/ICSENST.2008.4757170
Uono, S., Sato, W., and Toichi, M. (2011). The specific impairment of fearful expression recognition and its atypical development in pervasive developmental disorder. Soc. Neurosci. 6, 452–463. doi: 10.1080/17470919.2011.605593
Vaughan, K. B., and Lanzetta, J. T. (1980). Vicarious instigation and conditioning of facial expressive and autonomic responses to a model’s expressive display of pain. J. Pers. Soc. Psychol. 38, 909–923. doi: 10.1037//0022-3514.38.6.909
Weiguo, W., Qingmei, M., and Yu, W. (2004). “Development of the humanoid head portrait robot system with flexible face and expression,” in Proceedings of the 2004 IEEE International Conference on Robotics and Biomimetics, Shenyang, doi: 10.1109/ROBIO.2004.1521877
Keywords: android, emotional facial expression, dynamic facial expression, Facial Action Coding System, robot
Citation: Sato W, Namba S, Yang D, Nishida S, Ishi C and Minato T (2022) An Android for Emotional Interaction: Spatiotemporal Validation of Its Facial Expressions. Front. Psychol. 12:800657. doi: 10.3389/fpsyg.2021.800657
Received: 23 October 2021; Accepted: 21 December 2021;
Published: 04 February 2022.
Edited by:
Andrea Poli, Università degli Studi di Pisa, ItalyReviewed by:
Tanja S. H. Wingenbach, University Hospital Zurich, SwitzerlandCopyright © 2022 Sato, Namba, Yang, Nishida, Ishi and Minato. This is an open-access article distributed under the terms of the Creative Commons Attribution License (CC BY). The use, distribution or reproduction in other forums is permitted, provided the original author(s) and the copyright owner(s) are credited and that the original publication in this journal is cited, in accordance with accepted academic practice. No use, distribution or reproduction is permitted which does not comply with these terms.
*Correspondence: Wataru Sato, d2F0YXJ1LnNhdG8ueWFAcmlrZW4uanA=
Disclaimer: All claims expressed in this article are solely those of the authors and do not necessarily represent those of their affiliated organizations, or those of the publisher, the editors and the reviewers. Any product that may be evaluated in this article or claim that may be made by its manufacturer is not guaranteed or endorsed by the publisher.
Research integrity at Frontiers
Learn more about the work of our research integrity team to safeguard the quality of each article we publish.