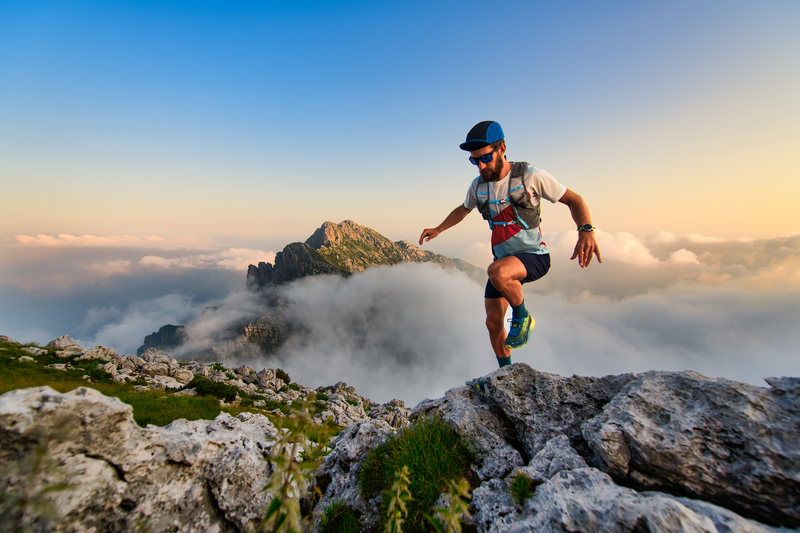
94% of researchers rate our articles as excellent or good
Learn more about the work of our research integrity team to safeguard the quality of each article we publish.
Find out more
CONCEPTUAL ANALYSIS article
Front. Psychol. , 28 December 2021
Sec. Health Psychology
Volume 12 - 2021 | https://doi.org/10.3389/fpsyg.2021.782866
This article is part of the Research Topic Digital Health Interventions for Psychological and Behavioral Changes During the COVID-19 Pandemic View all 9 articles
The COVID-19 pandemic has adverse consequences on human psychology and behavior long after initial recovery from the virus. These COVID-19 health sequelae, if undetected and left untreated, may lead to more enduring mental health problems, and put vulnerable individuals at risk of developing more serious psychopathologies. Therefore, an early distinction of such vulnerable individuals from those who are more resilient is important to undertake timely preventive interventions. The main aim of this article is to present a comprehensive multimodal conceptual approach for addressing these potential psychological and behavioral mental health changes using state-of-the-art tools and means of artificial intelligence (AI). Mental health COVID-19 recovery programs at post-COVID clinics based on AI prediction and prevention strategies may significantly improve the global mental health of ex-COVID-19 patients. Most COVID-19 recovery programs currently involve specialists such as pulmonologists, cardiologists, and neurologists, but there is a lack of psychiatrist care. The focus of this article is on new tools which can enhance the current limited psychiatrist resources and capabilities in coping with the upcoming challenges related to widespread mental health disorders. Patients affected by COVID-19 are more vulnerable to psychological and behavioral changes than non-COVID populations and therefore they deserve careful clinical psychological screening in post-COVID clinics. However, despite significant advances in research, the pace of progress in prevention of psychiatric disorders in these patients is still insufficient. Current approaches for the diagnosis of psychiatric disorders largely rely on clinical rating scales, as well as self-rating questionnaires that are inadequate for comprehensive assessment of ex-COVID-19 patients’ susceptibility to mental health deterioration. These limitations can presumably be overcome by applying state-of-the-art AI-based tools in diagnosis, prevention, and treatment of psychiatric disorders in acute phase of disease to prevent more chronic psychiatric consequences.
The COVID-19 pandemic has created enormous stressors across the globe by elevating rates of anxiety, depression, posttraumatic stress disorder (PTSD), or even suicidal behaviors (Bäuerle et al., 2020; Sherman et al., 2020; Wu T. et al., 2020; Cheung et al., 2021; Czeisler et al., 2021). The inadequate number of psychiatrists, lack of predictive biomarkers (Walker et al., 2017), and human subjectivity in assessments can further aggravate these problems, leading to a surge in the incidence and prevalence of mental health disorders across the globe. Therefore, urgent actions on extensive monitoring of mental health are needed, particularly concerning prediction and early treatment of individuals who were exposed to a high level of COVID-19-related distress, especially if they exhibit low overall stress resilience (Riehm et al., 2021) and other specific vulnerabilities (Li et al., 2020; Tuman, 2021). Recognition and identification of such high-risk individuals in the early stages of acute stress is extremely important to prevent the development of more serious long-term mental health disorders. The proposed predictive methodology presented in this article is based on appropriate multimodal stimuli, corresponding multimodal neuro-psycho-physiological features and their AI-based integration and analysis. A new generation of AI-based tools emerging in psychiatry (Ćosić et al., 2020a,b) might facilitate prediction of risk of chronic psychopathology (Karstoft et al., 2015; Schultebraucks et al., 2020a) and detection of suicidal ideation (Just et al., 2017), enable automated delivery of treatment (Fitzpatrick et al., 2017), help understand crucial ingredients of psychotherapy (Ewbank et al., 2020), as well as destigmatize reporting of mental health symptoms as virtual human interviewers (Lucas et al., 2017). Therefore, whether in prediction, prevention, diagnosis or treatment, AI-based technologies exhibit transformational potential to shift a self-report, conversation-, and observation-based qualitative system in psychiatry toward a more data/information-rich and quantitative one, even if several challenges still exist along this path (Graham et al., 2019; Rainey and Erden, 2020). A major strength of statistical and AI methods is their ability to identify specific non-obvious patterns beyond human computational capabilities within highly heterogeneous multimodal sets of data relevant for mental health assessment (Eyre et al., 2016; Garcia-Ceja et al., 2018), which is essential for early detection of ex-COVID-19 patients at high risk of mental health deterioration. Diverse forms of data for inclusion into such heterogeneous data sets have been encountered in several publications on AI in mental health (Eyre et al., 2016; Garcia-Ceja et al., 2018; Graham et al., 2019): various rating scales used by patients and clinicians, electronic health records (EHRs), brain imaging data, genomics, blood biomarkers, data collected while using smartphones, textual posts from social media platforms, speech and language audio data, facial video data, multiple peripheral physiological signals etc. Assuming that mentally vulnerable individuals affected by COVID-19 pandemic vs. resilient individuals have different profile of neuro-psycho-physiological indicators together with differences in personality traits and psycho-behavioral status, a comprehensive AI-based predictive approach should lead to better detection of high-risk ex-COVID-19 patients (Ramos-Lima et al., 2020) and more effective prevention strategies. The following sections present the concept of prediction of potential chronic mental health diseases among ex-COVID-19 patients, which includes comprehensive psychological, neurophysiological, semantic, acoustic, and facial/oculometric measurements and features, as well as the concept of mental health disorder prevention using AI methods.
The main objective of the proposed methodology for prediction of potential mental health disorders among ex-COVID-19 patients is to extend clinical interview and self-report information with more objective metrics. Therefore, this section provides an overview of a variety of psychological, speech-based, neurophysiological, as well as facial/oculometric features as potential predictors of mental health deterioration in the setting of post-COVID clinics. Such comprehensive data obtained through extended screening procedures of ex-COVID-19 patients in post-COVID clinics (Rubin, 2020; Walter, 2021), analyzed with AI-based tools of unsupervised machine learning methods (such as multivariate correlation analyses, clustering, and principal component analysis), as well as a range of traditional supervised machine learning methods (such as artificial neural networks, support vector machines and contemporary deep learning supervised approaches) can significantly improve prediction accuracy. A variety of AI-based tools which are already being used in research on prediction of adverse mental health outcomes from diverse data sets, are covered in the subsection on “Multimodal data fusion: statistical and ML analysis.”
Understanding that self-report psychological screening is also important, we will briefly discuss these instruments for prediction of chronic psychopathology. A wide variety of self-report questionnaires, many of which have already been employed in COVID-19-related mental health research, is potentially applicable within the proposed prediction methodology:
• Questionnaires regarding overall mental health and wellbeing, like the General Health Questionnaire (GHQ-12) (Gao et al., 2004; Pierce et al., 2020; Veer et al., 2021) and the Short Form Health Survey questionnaires (SF-36 and SF-12) (Ware and Sherbourne, 1992; Ware et al., 1996; Chen et al., 2020; Zhang S. X. et al., 2020).
• Questionnaires regarding stress resilience as an important protective factor against chronic psychopathology, e.g., the Connor-Davidson Resilience Scale (Connor and Davidson, 2003; Cai et al., 2020) and the Brief Resilience Scale (Smith et al., 2008; Riehm et al., 2021), as well as questionnaires targeting other related protective or risk factors, like hardiness (Eschleman et al., 2010; Rezaei and Vakilian, 2021), distress tolerance (Simons and Gaher, 2005; Liu N. et al., 2020), appraisal style (Kalisch et al., 2015; Veer et al., 2021), self-control (Maloney et al., 2012; Li et al., 2020), trait anxiety (Speilberger and Vagg, 1984; Lin et al., 2020), anxiety sensitivity (Deacon et al., 2003; Warren et al., 2021), and type D personality (Denollet, 2005; Tuman, 2021).
• Questionnaires regarding preexisting vulnerability to psychopathology that stems from adverse experiences encountered in life prior to pandemic, such as Life Events Checklist (Gray et al., 2004; Marco et al., 2020), Adverse Childhood Experiences Questionnaire (ACE-Q) (Felitti et al., 1998; Zarse et al., 2019; McCarthy et al., 2021), and the Trauma History Questionnaire (Hooper et al., 2011; Kolacz et al., 2020).
• Questionnaires closely aligned with diagnostic criteria for specific mental health disorders, like PTSD Checklist for DSM-5 (PCL-5) (Blevins et al., 2015; Liu N. et al., 2020), Impact of Event Scale–Revised (Weiss, 2004; Wang et al., 2020), Beck Depression Inventory (Beck et al., 1961; Di Tella et al., 2020), Generalized Anxiety Disorder scale (GAD-7) (Spitzer et al., 2006; Bäuerle et al., 2020). These questionnaires are an essential part of any future predictive algorithm, since a very high score on any of them suggests an immediate risk that the disorder is already emerging or present.
• Questionnaires regarding general distress experienced by ex-COVID-19 patients during the pandemic, like K10 Kessler Psychological Distress Scale (Andrews and Slade, 2001; Janiri et al., 2020), Perceived Stress Scale (Roberti et al., 2006; Eubank et al., 2021), and Peritraumatic Dissociative Experiences Questionnaire (Marmar et al., 1997; Ranieri et al., 2021).
• Questionnaires on COVID-19-specific distress, which are either adapted from existing illness-related questionnaires, like Brief Illness Perception Questionnaire (Broadbent et al., 2006; Hubbard et al., 2021) or newly developed, e.g., Fear of COVID-19 Scale (Ahorsu et al., 2020; Bitan et al., 2020), Post-COVID-19 Functional Status Scale (Klok et al., 2020), and COVID-19 Peritraumatic Distress Index (CPDI) (Qiu et al., 2020).
Finally, self-reports regarding the presence of a previously diagnosed psychopathology (Asmundson et al., 2020) would be important in cases where mental health history is not otherwise available, since it is known that depression and anxiety occur more commonly in patients with previous psychiatric diagnosis (Hao et al., 2020). In selection of questionnaires in line with this brief review, it is important to minimize their overlap, while favoring all of those with strong scientific evidence as risk assessment instruments, to acquire efficiently the most valuable psychological features for prediction of future psychopathology.
The potential of human speech patterns in detection and prediction of psychopathology has already been demonstrated in several research works (Cummins et al., 2015; Marmar et al., 2019; Schultebraucks et al., 2020b). Therefore, this section discusses speech features that are the most promising for application in risk assessment of ex-COVID-19 patients. A wide range of methods to elicit speech features for assessment and prediction of mental health disorders include: vocal exercises; reading neutral or emotionally charged words, sentences or passages, with or without mood induction by pictures or videos; as well as free-form speaking while describing emotionally arousing events or during interviews conducted by humans or virtual agents (Cummins et al., 2015). Due to prior experiences that free-form speech might offer better prediction performance compared to a read passage (Cummins et al., 2015), free-form speech elicitation methods might be preferable for ex-COVID-19 patients. While free-form speech collection during time-consuming disorder-specific diagnostic interviews can provide valuable speech information, e.g., in PTSD assessment (Marmar et al., 2019), it would require excessive time to conduct multiple diagnostic interviews for a broader range of COVID-19-relevant mental health problems, like generalized anxiety, depression, PTSD and suicidal ideation/behavior. Accordingly, for elicitation of speech in ex-COVID-19 patients, it might be more appropriate to use: (a) relevant modules of shorter structured clinical interviews, like M.I.N.I. (Sheehan et al., 1998; Winkler et al., 2020); (b) semi-structured interviews on COVID-19 experiences, which have been applied in qualitative research during the pandemic (Gillard et al., 2021; McKinlay et al., 2021); (c) COVID-19-related adaptations of very brief time-limited interviews, like the 3-min interview from Schultebraucks et al. (2020b); or (d) COVID-19-related concretizations of broad interview formats that are generally structured around 5W and H questions, e.g., (Dilts, 1990).
More accurate prediction of potential psychiatric disorders among ex-COVID-19 patients would benefit from AI-based natural language processing (NLP) of free-form speech to make distinctions between stress vulnerable vs. stress resilient ex-COVID-19 patients. Such linguistic information and related unstructured data might be of great importance as valuable clinical information to identify relevant keywords for early detection of various mental health problems. NLP can capture patterns of irrational or distorted language associated with patterns of specific psychopathologies, like patient’s mental distortions, biases, core beliefs, negative wording, and compensatory strategies. Using NLP techniques, we can uncover patterns of how specific psychopathologies are reflected in language, and related semantic and acoustic features across time, what might be helpful in post-COVID clinical settings. From a technical perspective, all answers, comments and observations during the interview are continuously monitored and serve as a basis for real-time, and subsequently off-line, NLP analysis. To support this, an automated conversion of microphone-recorded speech to text is performed in the background.
Many studies analyze speech content in terms of linguistic measures such as word frequencies, lexical diversity, narrative coherence, sentiment of speech content and others, using them as features to classify major depressive disorder (MDD) (Cummins et al., 2011, 2015; Alghowinem et al., 2013; Calvo et al., 2017; Schultebraucks et al., 2020b) and PTSD (Scherer et al., 2015; He et al., 2017; Schultebraucks et al., 2020b). Schultebraucks et al. (2020b) showed that the most important features for predicting PTSD and MDD status were the NLP features, while the features from other groups (face, acoustic and movement) also showed high potential. Features were calculated using the Linguistic Inquiry and Word Count (LIWC) 2015 dictionary (Pennebaker et al., 2015) and DeepSpeech (Hannun et al., 2014) from free-form speech responses to a brief interview 1 month after the trauma. Predictive importance ranking was done using SHAP (SHapley Additive exPlanation). The most important predictor for PTSD was NLP LIWC “self-assured,” while for MDD, it was age, followed by NLP LIWC “workhorse.”
LIWC is a widely used software tool in mental health projects (Calvo et al., 2017). It is composed of more than 6,000 words, word stems and selected emoticons and calculates approximately 90 output variables for each text input, i.e., word count, summary language variables (e.g., authenticity, emotional tone), general descriptor categories (e.g., words per sentence), word categories tapping psychological constructs (e.g., affect, cognition, biological processes), informal language markers (e.g., assents, fillers, swear words, netspeak) and others (Pennebaker et al., 2015). In addition to LIWC, authors often use other linguistic tools such as N-gram language models, emotional thesauri like WordNet-affect (Strapparava and Valitutti, 2004) and normative databases such as the Affective Norm for English Words (ANEW) (Bradley and Lang, 1999).
A study was done on the topic of wording trend analysis and other NLP analyzes in 15 of the world’s largest mental health support groups found on Reddit (e.g., r/schizophrenia, r/SuicideWatch, r/Depression) during the initial stage of the COVID-19 pandemic (Low et al., 2020). Beside the LIWC features, the authors also included sentiment metrics, basic word and syllable counts, punctuation, readability metrics, term frequency–inverse document frequency (TF-IDF) ngrams and manually built lexicons about suicidality, economic stress, isolation, substance use, domestic stress and guns. Although the paper is not directly related to the prediction or diagnosis of mental health disorders in ex-COVID-19 patients, the presented textual features and conclusions of the analyses can be used as valuable information for tuning and improving such prediction and diagnosis models.
Most recent studies in the field of mental health-related NLP, as shown above, are still based on the analysis of keywords and statistical representations of words, although NLP has been elevated to the context-based semantic level of word representations in the last few years. The reason behind this is mainly due to the ease of interpretation of keyword-based features, while on the other hand, the context-based representation of words is more abstract.
In general, this semantic NLP progress can be shown through the following most important achievements: bag-of-words (word-count metrics that ignore grammatical structure) (Harris, 1954), word2vec (word embeddings—static representation of words in a semantic multidimensional space based on the context in which these words occur in a specific language) (Mikolov et al., 2013), and BERT (transformer-based language model that represents words and subwords through contextual embeddings in a way that they dynamically change their position in the semantic space depending on the specific environment, e.g., sentence, in which they are located) (Devlin et al., 2019).
Acoustic features of speech have widely been used as indirect markers of underlying physiological phenomena. These features are useful within the clinical interview paradigm, described at the beginning of this section, as speech production involves both simultaneous cognitive planning and complex motoric muscular actions (Cummins et al., 2015). Slight physiological and cognitive changes can produce noticeable acoustic changes (Scherer, 1986) in the perceived speech during such events. Examples of such changes were documented as far as 1921 (Kraepelin, 1921), where voices of depressed patients were described as low, slow, hesitant and monotonous (amongst other things). While some of those descriptions can easily be explained with singular features, e.g., lower speech fundamental frequency (sometimes called pitch) in the case of low voice, or lower number of voiced frames in an utterance (speech rate) and pauses between words in the case of slow voice, we often find more complex groups of features describing such phenomena as they can produce more information for such a varied process like speech. Such features can also help to identify other breathing abnormalities (e.g., Mukherjee et al., 2021a,b).
In our previous research we have focused on developing optimized stimulation paradigms for elicitation of multimodal responses related to stress resilience (Dropuljić et al., 2017; Ćosić et al., 2019b) and cognitive functioning estimation (Mijić et al., 2017, 2019). Acoustic feature sets were related to: (a) fundamental frequency, an estimate of the base harmonic that the vibrating vocal cords are producing during vocalization; RMS energy, a signal-processing-based estimate of the energy of the sound recorded by the microphone; (b) formant frequencies (f1–f4) and mel-frequency-cepstral-coefficients which describe the spectral and cepstral behavior of the recorded utterances; (c) jitter and shimmer, representing voice perturbations; and (d) number of voiced segments per second and mean voiced/unvoiced segment lengths in seconds, which are related to speech rate.
Predefined experimental paradigms (e.g., Dropuljić et al., 2017; Ćosić et al., 2019b) may be applicable for collecting speech features that would be helpful in identifying ex-COVID-19 patients suffering from post-COVID-19-related psychopathology. However, we believe that using utterances from clinical interviews would be more beneficial as it enables a more “close-nit” integration with semantic features and free-form speaking has evident advantages when collecting speech data in the context of psychopathologies (Cummins et al., 2015). Afshan et al. (2018) describe a particularly similar usecase, where depressed vs. normal speech was successfully classified using speech recordings of 1688 clinical interviews (735 depressive and anxiety disorders, 953 healthy). The used feature sets greatly overlapped with the ones we used in our stress resilience and cognitive load estimation research, described in the previous paragraph. A comprehensive framework for analyzing depression in the context of voice features is presented in Cummins et al. (2015), alongside an exhaustive overview of papers that deal with particular voice features and how they relate to either the physiological or cognitive effects of depressive disorders. Such body of work provides the necessary interpretability needed in usecases where we deploy the above-described features as diagnostic tools for usecases presented in this paper. An important property of speech as a modality is that it can easily and reliably be collected within the paradigm proposed in this paper, and can serve within the multimodal framework, complementing other modalities.
In summary, speech as a modality within the clinical interview paradigm, and as a component of a larger set of paradigms would prove a suitable augmentation alongside semantic, neurophysiological, and facial/oculometric features. Acoustic speech analysis would be particularly valuable alongside semantic analysis of the clinical interview, for prediction of potential mental health disorders like depression and early identification of various mental health risks in ex-COVID-19 patients. Furthermore, acoustic speech analysis may potentially be used as a real-time analysis tool that provides valuable information about the dynamics of the patients’ emotional change during AI-based therapy described in section on Prevention, or a tool that helps show differences between resilient vs. vulnerable patients.
This section provides an overview of multimodal neurophysiological features as potential predictors of mental health deterioration in ex-COVID-19 patients. The main idea is a comprehensive set of carefully selected, elicited, and quantified neurophysiological features which can help identify those ex-COVID-19 patients who are at risk of developing post-COVID-19-related psychopathology, such as depression, anxiety, or PTSD (Liu C. H. et al., 2020; Mazza et al., 2020). These features are assessed during specific test paradigms and tasks which elicit corresponding multimodal responses and can be associated with patients’ neuro-psycho-physiological risk states, like acute stress, anxiety (Shaffer and Ginsberg, 2017; Arza et al., 2019), and cognitive decline (Lynch and Lachman, 2020); as well as protective traits, like stress resilience (Walker et al., 2017). Translation of earlier findings, particularly in the area of physiology-based resilience/vulnerability assessment (Ćosić et al., 2019a; Šarlija et al., 2021), as well as in the area of objective neurophysiological assessment of cognitive functioning (Kesedžić et al., 2020a), could facilitate the design of efficient and reliable methods for multimodal prediction of potentially vulnerable individuals among ex-COVID-19 patients.
Stress resilience can be defined as an ability or a process of maintaining normal psychological, physiological, and physical functioning when exposed to high levels of stress and trauma (Russo et al., 2012). Moreover, a review of various objective biomarkers of stress resilience by Walker et al. (2017), described resilience as the absence of trauma-related psychiatric disorder symptoms, i.e., PTSD, as well as of other disorders like depression (Edward, 2005; Schultebraucks et al., 2021). A primary vision highlighted in the review by Walker et al. (2017), as well by our research on multimodal physiology-based stress resilience assessment (Ćosić et al., 2019a; Šarlija et al., 2021), is the “early identification of individuals who are at risk of developing PTSD or depression” (Walker et al., 2017), which becomes particularly valuable in the face of the COVID-19 pandemic (Ćosić et al., 2020a; Liu C. H. et al., 2020; Mazza et al., 2020).
The deficits in a wide range of cognitive processes and/or dysfunction of the prefrontal cortex (PFC) and its connected circuitry are already associated with many mental disorders and neurological conditions (Friedman and Robbins, 2021). The cognitive decline in ex-COVID-19 patients, related to cognitive deficits (Hampshire et al., 2021), as well as global cognitive impairment, impairment in memory, attention, and executive function (Daroische et al., 2021), is one of the specific characteristics of mental health vulnerabilities among the ex-COVID-19 patients. Cognitive assessment of patients with recent COVID-19 infection through specialized clinics dedicated to further diagnostic assessment and tailored rehabilitation is recognized as a preventive measure of mental health deterioration (Liotta et al., 2020; Daroische et al., 2021).
Peripheral physiological features of interest are mainly related to resting autonomic nervous system (ANS) activity, like resting heart rate variability (HRV) and respiratory sinus arrhythmia (RSA); features of psychophysiological allostasis, like cardiac allostasis; as well as electromyogram- and electrodermal activity-based (EMG and EDA) acoustic startle response (ASR) features, like startle reactivity, startle habituation, and startle-modulation-related features, e.g., fear/anxiety-potentiated or prepulse inhibited startle response (Ćosić et al., 2019a; Šarlija et al., 2021). In the following paragraphs, we aim to expand on our prior research related to identification and assessment of various multimodal physiological features of stress resilience (Ćosić et al., 2019a; Šarlija et al., 2021) in the direction of potential discriminators of ex-COVID-19 patients which are either resilient or at-risk for the development of COVID-19-related psychopathology.
In our research on objectivization of stress resilience assessment (Ćosić et al., 2019a), several physiological features confirmed discriminative power between resilient and non-resilient mentally healthy individuals. These were related to: (a) resting RSA, which measures HRV in phase with inhalation and exhalation (Grossman and Taylor, 2007); (b) EMG-based startle reactivity, which measures the strength of reflexive defensive responding to an aversive unconditioned stimulus, i.e., abrupt, loud noise (Bradford et al., 2014); and (c) cardiac allostasis, which measures adaptive reaction to a stressful event, involving a vigorous cardiac response to stress coupled with a significant cardiac recovery in the aftermath (Souza et al., 2013). These three simple physiological features were also recognized as potential biomarkers of stress resilience according to an extensive review of relevant prior research on the psychophysiology of resilience (Walker et al., 2017).
This paragraph provides a brief overview of physiological features supported by evidence from prospective studies, which are in the context of the current paper considered the most relevant category of literature. A simple EDA-based measure of sympathetic activity, collected during a standard trauma interview in the immediate aftermath of a traumatic event, was shown to predict risk of developing PTSD (Hinrichs et al., 2019). Elevated heart rate following a traumatic event is a simple physiological feature that was associated with later development of warzone-related PTSD (Shalev et al., 1998; Dean et al., 2019). Both increased heart rate and reduced high frequency HRV (HF-HRV) predicted PTSD development following a trauma exposure in women (Seligowski et al., 2021). Another simple measure of resting vagally-mediated HRV, i.e., RMSSD, was negatively associated with development of depressive symptoms in healthy young subjects, over a period of 3 years (Carnevali et al., 2018). HF-HRV and RMSSD are, besides RSA, also features which are linked to resting autonomic nervous system activity. Startle reactivity, introduced above as a marker of resilience, as well as other ASR-related features, e.g., startle habituation, were also found to be predictive of PTSD development (Shalev et al., 2000; Pole et al., 2009; Risbrough et al., 2012, 2017). In the context of COVID-19, EMG-based startle reactivity, interpreted as a physiological marker of anxiety was increased in COVID-19 physicians compared to non-COVID-19 physicians, and was also predictive of later anxiety scores measured by GAD-7 (Dolev et al., 2021). A systematic literature review on the association between HRV reactivity to laboratory stress (which is similar to the concept of cardiac allostasis, mentioned in the paragraph above) and depression, states that none of reviewed studies have evaluated the prospective prediction of depressive symptoms (Hamilton and Alloy, 2016). The authors claim that this “will be important in differentiating whether HRV reactivity is a consequence or correlate of depression or a marker of vulnerability to future depression,” which is in line with our discussion on the importance of prospective research. Besides physiological features, other markers such as polygenic scores (Schultebraucks et al., 2021), cortisol (Galatzer-Levy et al., 2017), or measures of hippocampal activity (van Rooij et al., 2018, 2021) were also identified as predictive of mental health deterioration after major life stressors, but a review of such an expanded set of potential predictors exceeds the scope of this section. A vast amount of research was also done on various other physiological features as correlates or diagnostic markers of PTSD, depression, anxiety, etc. but are here omitted due to their already mentioned decreased relevance in comparison with prospective research.
A brief literature review presented above demonstrates the potential of simple yet informative physiological features like resting RSA or EMG-based startle reactivity, which can be elicited and assessed using a relatively short and generic stimulation and evaluation protocol (Ćosić et al., 2019a,b; Šarlija et al., 2021). Therefore, the proposed protocol for elicitation and assessment of physiological features in the prediction of mental health deterioration in ex-COVID-19 patients should include a resting period, a stress-inducing period with acoustic startle stimuli, and a post-stress recovery period, while the patients’ peripheral physiological responses are being continuously recorded.
Cognitive decline, which is observable in the PFC activation on a variety of cognitive tasks, is one of the characteristics of mental health vulnerabilities in ex-COVID-19 patients (Daroische et al., 2021; Hampshire et al., 2021). The prediction of vulnerable individuals among the ex-COVID-19 patients can be improved using brain monitoring techniques, and particularly features extracted from the PFC activation. The neuroanatomical connectivity of the PFC to most parts of the cortical and subcortical brain makes it well suited for participating in a number of neural networks and carrying out cognitive control operations in different functional domains (e.g., spatial, visual, and verbal) (Friedman and Robbins, 2021). The PFC is linked through its extensive association connections with distant and broadly dispersed parts of the association and limbic cortices and these interconnections with the amygdala, hypothalamus, midbrain, and pons represent important subcortical linkages of the extended prefrontal neural system.
Research on mental disorders using brain imaging techniques, including functional near-infrared spectroscopy (fNIRS), a simple, wearable, and low-cost brain imaging technique which enables PFC activation estimation, suggests that these disorders change the way the brain functions during different cognitive challenges. fNIRS was used to estimate the PFC activation on a variety of cognitive tasks in patients with PTSD (Matsuo et al., 2003; Tian et al., 2014; Yennu et al., 2016; Gramlich et al., 2017), panic disorder (Nishimura et al., 2007), social anxiety disorder (Yokoyama et al., 2015), and MDD (Nishizawa et al., 2019; Zhu et al., 2020). Differences between these groups of patients and healthy individuals were found, suggesting a hypoactivation of the PFC in individuals with PTSD compared to healthy controls in verbal fluency task (Matsuo et al., 2003), as well as hypoactivation in the left lateral PFC during the incongruent Stroop task (Yennu et al., 2016). A general conclusion from these studies is that PTSD patients experience PFC hypoactivation during cognitive tasks, but their PFC activation increases dramatically when the person encounters an object associated with tragic and traumatic events (Kim et al., 2017). A systematic review by Ho et al. (2020) on applications of fNIRS for MDD indicated that fNIRS consistently demonstrated attenuated cerebral hemodynamic changes in depressed compared to healthy individuals. Patients with MDD or panic disorder show hypoactivation in the PFC during cognitive tasks (Kim et al., 2017), while high-trait-anxiety individuals showed a reduced PFC activation during the cognitive task compared to those with low anxiety (Brugnera et al., 2017). In our research on cognitive functioning, we classified different cognitive states using neurophysiological signals (Kesedžić et al., 2020a), and showed differences in brain activation among individuals during cognitive tasks and discussed the applicability of such objective monitoring systems in a real-working environment (Kesedžić et al., 2020b). In addition to the research in patients with mental disorders and healthy controls, the fNIRS was used on ex-COVID-19 patients in the research of hypoxemia (Ferrari and Quaresima, 2020) as well as during presentation of olfactory stimuli (Ho et al., 2021).
In summary, the tasks used in research include verbal fluency tasks, Stroop tasks, mathematical tasks, neutral and fearful faces presentation, and pleasant, unpleasant, and neutral sounds. The proposed paradigm for ex-COVID-19 patients should include cognitive tasks, such as mathematical tasks, n-back task, or Stroop task. In addition to the standard tasks for the study of memory processing and decision making, such paradigms should consist of images, sounds, and videos related to COVID-19 traumatic experiences. These paradigms would likely show differences in PFC activation on different tasks and might predict possible future mental disorders in vulnerable ex-COVID-19 patients.
Distortions in facial and oculometric system have been reported in patients with various psychiatric disorders when compared to controls. These detected oculometric distortions can be categorized either as behavioral or as physiological depending on the extracted features. This section presents an overview of a limited number of studies that discuss prediction of psychiatric disorders from facial and/or oculometric features. This method is still in the discovery phase, but it has great potential, particularly due to non-invasiveness and inexpensiveness.
Behavioral changes include dysfunctions in oculomotor performance related to attention and fixation. Several studies have shown that attention deficit is a feature of psychiatric disorders such as depression, anxiety disorder, schizophrenia, and bipolar disorder (Bittencourt et al., 2013; Kleineidam et al., 2019). One often reported cognitive parameter for attention evaluation is saccadic eye movement. Patients with depression experienced shorter scanpath length in the free-viewing test, shorter duration of saccades and lower peak saccade velocity in the smooth pursuit test (Takahashi et al., 2021), as well as showed a greater latency, a reduction in movement precision, and a reduced peak velocity in the antisaccade task (Bittencourt et al., 2013; Li et al., 2016). Furthermore, individuals affected by PTSD and/or depression maintained their attention longer on the fearful, disgusted, and depressed expressions relative to the happy expression in comparison to controls (Sears et al., 2011; Armstrong et al., 2013; Lee and Lee, 2014; Powers et al., 2019). Additionally, PTSD patients demonstrated a greater number of initial eye fixations on the threat word and more orienting responses on all threatening and neutral words than controls (Felmingham et al., 2011; Powers et al., 2019).
Further, attention bias toward positive emotional faces studied in the laboratory has been shown to predict self-reported stress resilience (Thoern et al., 2016). We have developed and optimized general stimulation paradigms for elicitation of facial and eye gaze features for stress resilience assessment/prediction (Ćosić et al., 2016, 2019b).
Stress-induced physiological changes include dysfunctions in oculomotor performance exhibited in physiological parameters such as pupil diameter and spontaneous eye blink, which are modulated by ANS. Pupil diameter, modulated by the noradrenergic locus coeruleus (Rajkowski et al., 1993.), is reported to be larger in patients with PTSD exposed to threat-relevant stimuli and negatively valanced pictures (Kimble et al., 2010; Cascardi et al., 2015; Mckinnon et al., 2020). Previous studies have also indicated a relationship between spontaneous blink rate, a proxy of dopaminergic activity (Eckstein et al., 2017), and anxiety and/or depression (Mackintosh et al., 1983; Kojima et al., 2002; Al-gawwam and Benaissa, 2018).
Facial features that have been reported to be informative in classification of psychiatric disorders, especially depression, are facial expressions and face movement measures (Schultebraucks et al., 2020b; Stolicyn et al., 2020). Facial expressions used for prediction are in the literature described and supported by individual components of muscle movement, called Action Units (AUs) (Yao et al., 2021). There are 23 AUs all together, but some have stood out more than others in predicting psychopathological states (Gavrilescu and Vizireanu, 2019; Kumar et al., 2020). Beside facial expressions, face movement measures are also indicators of various disorders. A study by Jaiswal et al. (2019) included head pose in prediction of depression and anxiety, along with AUs and eye gaze but the discriminative power of the feature itself was not discussed.
The abovementioned studies did not include ex-COVID-19 patients, but the presented findings are useful in the context of prediction of psychopathological states in ex-COVID-19 patients, who reported increased symptoms of anxiety and depression and high risk of developing PTSD, sleep abnormalities, and cognitive impairments (Mazza et al., 2020; Wu C. et al., 2020).
The proposed protocol, based on the overview of the literature, should include clinical interview which would be video recorded and from which facial features then would be extracted. In addition to the recorded interview, the proposed protocol should also consist of oculometric paradigms such as antisaccade, free-viewing and smooth pursuit task, as well as images related to COVID-19 traumatic experiences, while the patients’ oculometric responses are being continuously recorded.
Based on the literature review and discussion of potential multimodal predictors of psychopathology in ex-COVID-19 patients, we first summarize the proposal of an experimental protocol for elicitation, acquisition, and assessment of the described multimodal data (Figure 1). The patients should first fill out a series of above listed self-report instruments. A relatively short structured clinical interview is suggested right after the self-report assessment. These two parts of the protocol can quickly and simply indicate the need of immediate psychiatric intervention in patients which are in the phase of emerging and evident psychopathology at the time of the screening. Furthermore, the clinical interview should be both audio and video recorded, similarly to a protocol recently used in deep-learning-based diagnosis of PTSD and depression following trauma (Schultebraucks et al., 2020b). The audio recording would therefore provide data for the extraction of various semantic and acoustic speech features, and the video recording would provide data for extraction of various video-based, i.e., facial/oculometric features. Patients which are classified as mentally healthy based on the self-report instruments and the clinical interview are administered the remainder of the experimental protocol, which should include: (a) a short and generic stimulation protocol for elicitation and assessment of physiological features of stress resilience (Ćosić et al., 2019a; Šarlija et al., 2021); and (b) a set of appropriate tasks for assessment of various fNIRS-based and attention-related features.
Figure 1. Illustration of time-synchronized multimodal input stimulation and multimodal output response of ex-COVID-19 patient during prediction protocol.
The potential of AI in alleviating the consequences of the COVID-19 pandemic on mental health has already been recognized (Ćosić et al., 2020a,b; Thenral and Annamalai, 2020). The comprehensive data obtained through the proposed screening protocol of ex-COVID-19 patients can be analyzed by AI-based tools of unsupervised ML methods (e.g., multivariate correlation analyses, clustering, and principal component analysis), as well as a range of supervised ML methods (e.g., artificial neural networks, support vector machines, deep-learning supervised approaches). Such technologies are already being used in forecasting COVID-19 cases (e.g., Lalmuanawma et al., 2020; Santosh, 2020), as well as in COVID-19-specific (e.g., Prout et al., 2020; Herbert et al., 2021) and more general mental health context related to prediction of stress- or trauma-related adverse mental health outcomes from different types of data. For example, traditional supervised learning methods have been applied on: (a) self-report psychological measures and variables collected in emergency room to predict PTSD and depression (Galatzer-Levy et al., 2014; Karstoft et al., 2015; Ziobrowski et al., 2021); (b) bio-physio-clinical-demographic features from EHRs augmented by acute stress self-report measures to predict PTSD course (Galatzer-Levy et al., 2017; Schultebraucks et al., 2020a); (c) clinical and demographic features from EHRs to identify veterans at high suicide risk (Kessler et al., 2017); and (d) polygenic scores to predict the trajectory of depression development (Schultebraucks et al., 2021). Unsupervised clustering methods and physiological startle-based measures have been used in the long-term prediction of adverse psychological outcomes among physicians treating COVID-19 or non-COVID-19 patients (Dolev et al., 2021). Deep learning methods applied on facial and speech data have been shown to successfully classify PTSD and depression following a trauma (Schultebraucks et al., 2020b). The mentioned examples, as well as a recent review of 33 prognostic and 16 diagnostic studies on application of ML in trauma-related mental health disorders (Ramos-Lima et al., 2020), highlight the ability of ML techniques to improve not only diagnosis, but also to enable prediction of negative trauma-related mental health outcomes, which is important in the context of the current work.
Besides establishing a comprehensive set of potentially predictive features (illustrated in Figure 2), how we label the data deserves particular attention. The hierarchical data acquisition protocol described in the previous section would also be accompanied by a complex hierarchical fuzzy labelling system, which includes, e.g., PTSD risk (“high,” “medium,” “low”), depression risk (“high,” “medium,” “low”), state anxiety (“high,” “medium,” “low”). This labelling process requires longitudinal prospective research years after the initial measurement, which is feasible within the framework of post-COVID-19 clinics. The hierarchical data acquisition protocol should additionally result with a hierarchical prediction and decision support system, where preventive actions can truly be taken in a timely manner.
Figure 2. Multimodal data acquisition as input for real-time and offline feature computation. Illustrated subset of selected features includes: General Health Questionnaire (GHQ-12), Connor-Davidson Resilience Scale (CD-RISC), COVID-19 Peritraumatic Distress Index (CPDI); NLP feature LIWC “sad,” voice fundamental frequency (F0), voice root mean square (RMS); respiratory sinus arrhythmia (RSA), EMG-based startle reactivity (SREMG), prefrontal cortex activity (PFCact); saccadic peak velocity (SPV), pupil dilation (PD), a feature related to facial action coding system (FACS).
Methods based on supervised ML, while maximizing classification/prediction accuracy, often sacrifice model explainability and rigorous statistical validation. Accordingly, the need to focus on explainability, transparency, as well as generalizability of the ML models applied in psychiatry, by means of external validation of the developed models, has been recognized (Shatte et al., 2019; Chandler et al., 2020; Ramos-Lima et al., 2020). For example, only 6 out of 28 studies reviewed in 2019 by Graham et al. included external validation. One generalizability-related challenge for AI in mental health is dealing with prediction models for rare disorders or events, e.g., suicides in particular, which can generate unacceptably high number of false positive predictions once deployed on a large scale in practice, even when exhibiting high accuracies, sensitivities and specificities (Belsher et al., 2019; Jiang et al., 2020; Machado et al., 2021). Explainability, as a key prerequisite for the clinical use of the developed ML methods (Tjoa and Guan, 2020), is important for reliable prediction, but also for personalized treatment and prevention of mental health deterioration in ex-COVID-19 patients. When mentioning explainability, people often refer to global explainability (Jha et al., 2021), which is useful for understanding the underlying mechanisms of mental health deterioration, e.g., what variables contribute most significantly to a given prediction. Besides such global explanations, in the context of health applications, the local explainability of the developed models is crucial, i.e., providing the information to the end user, in this case the mental health practitioners, on why a particular decision was made. Examples of local explainability methods are LIME (Ribeiro et al., 2016) and SHAP (Lundberg and Lee, 2017). Such explanations for risk assessments and predictions are needed for mental health practitioners to justify their reliability, as well as to better tailor the preventive action for a given patient (discussed in the next section), according to the main determinants of the predicted negative mental health outcome. A more extensive discussion on the potential of explainable AI (XAI) in addressing the complexity of mental health research, particularly related to human-computer interaction, is available in Roessner et al. (2021). Furthermore, these new AI methods in prediction and prevention of mental health disorders should take into account human-computer interaction design principles on ethical, fair, trustworthy, and human-centered AI, which is important in the context of mental healthcare/suicide prevention and beyond (Xu, 2019; Robert et al., 2020). Additionally, other challenges like ethics due to sensitive data assessment, social factors, suitable education and training of medical professionals, as well as lack of evidence for clinical and economic impact of using AI in clinical psychiatric practice, are discussed in the literature (Graham et al., 2019; Aung et al., 2021; Lee et al., 2021).
Timely and appropriate preventive interventions among high-risk ex-COVID-19 patients, before the onset of serious psychopathology, should be of paramount common interest (Billings et al., 2020; Duan and Zhu, 2020). A variety of digital technologies which may assist in this globally increasing problem, including AI-based tools, are being discussed in the literature (Bickman, 2020; Ćosić et al., 2020b; Maulik et al., 2020; Gargot et al., 2021). Along these lines, the proposal in this section is based on our prior research work on tools and methods for prevention and treatment of stress-related disorders (Popovic et al., 2006; Ćosić et al., 2007, 2010a,2013a; Popović et al., 2009). The proposed preventive approach facilitated by AI tools is based on our research on an adaptive system for multimodal stimulation and multimodal real-time neuro-psycho-physiological feedback loop in the context of cognitive-behavioral exposure therapy (Rothbaum and Schwartz, 2002) and stress inoculation training (Meichenbaum, 2017), and is closely related to the emotion regulation framework (Gross, 1998), emotion regulation therapy (Mennin, 2006; Renna et al., 2017, 2020), as well as cognitive restructuring (Clark, 2013). Real-time processing of an individual’s multimodal neuro-psycho-physiological features, e.g., those presented in the previous section, is a prerequisite for a closed-loop emotion regulation strategy in the context of exposure therapy and stress inoculation training (Hodges and Rothbaum, 1998; Ćosić et al., 2010a); a similar closed-loop approach for a specific phobia has been demonstrated by Bãlan et al. (2020). Closing the loop over a single feature is commonly found in bio/neurofeedback (Choi et al., 2011; Tan et al., 2011), but more complex multimodal feedback has been more recently investigated in the context of fatigue and anxiety (Chang et al., 2016; Apostolidis et al., 2021). A study by Chang et al. (2016) demonstrated multimodal biofeedback in AI-based driver fatigue detection and alerting system implemented on an embedded real-time digital signal processing hardware, using six HRV parameters extracted from ECG signal and classification of yawning and eye closure events from face recordings. A study by Apostolidis et al. (2021) presented an AI-based biofeedback system for anxiety awareness, using anxiety classification from galvanic skin response, heart rate, and skin temperature signals on a low-cost real-time processing board and displaying relaxing pictures in cases of high anxiety. Both studies introduced AI methods into the multimodal feedback loop, but still used only one or two modalities and a limited number of features. In the context of mental health disorder prevention, more modalities and features are required, which would then require an optimal feature selection, faster AI models, as well as a higher computational power with an emphasis on real-time performance. As modalities and input complexity increase, so do real-time processing device requirements (Adams and Marketing, 2002), including sufficient number of cores for parallel processing and intensive computing, adequate amount of memory, high-speed interfaces capable of moving large amounts of data, and cost efficiency.
In this context, multisensory and multimodal interaction between the AI-assisted therapist and the treated ex-COVID-19 patient is based on a variety of customized multisensory inputs, such as non-interactive visual and auditory stimuli, interactive virtual reality stimuli and digital serious games (Li et al., 2014; Lau et al., 2017), as well as comprehensive multimodal neuro-psycho-physiological feedback features. Closed-loop control strategies, which match selected multisensory stimuli to multimodal feedback in real-time using a broad range of AI-based algorithms, from fuzzy logic (Popovic et al., 2006; Ćosić et al., 2007) to machine learning (Ćosić et al., 2020b), are related to different emotion regulation, exposure, stress inoculation and cognitive restructuring methods (Popovic et al., 2006; Ćosić et al., 2007, 2010a, b, 2011, 2013a, b; Popović et al., 2009). Conceptual illustration of the integration of AI-based tools with interventions relying on various psychotherapeutic preventive strategies is presented in Figure 3.
Figure 3. Concept of the integration of AI-based tools and methods with various preventive intervention strategies.
Patient-personalized real-time multisensory stimuli generation uses semantically and emotionally annotated pictures, natural and artificial sounds, recorded speech, written text messages, video-clips and films or virtual reality synthetic environments, which reflects the underlying complexity of potential chronic psychopathology. Usage of existing and dedicated large databases of images and/or sounds, which are semantically (Deng et al., 2009) and emotionally annotated (Horvat, 2017) via AI-tools related to ontologies (Horvat et al., 2009, 2012, 2014) and automated semantic segmentation (Chen et al., 2018), should optimize the delivery of the most relevant semantic and emotional stimuli content to the ex-COVID-19 patient during the intervention course, which is adapted to the patient’s reactions in real time (Ćosić et al., 2010a). Real-time computation of multimodal neurophysiological, speech and facial/oculometric features, as well as real-time computation of optimal control multimodal closed-loop feedback stimuli is a particular software and hardware challenge. Efficient search of comprehensive stimuli databases and selection of semantically and emotionally optimal stimuli within therapeutic context and strategy also deserve particular attention.
Comprehensive patient monitoring during emotion regulation in a multisensory and multimodal closed-loop feedback paradigm, based on real-time tracking of multidimensional neuro-psycho-physiological features, better reflects the complexity of the problem of stress-induced psychopathologies than focusing exclusively on conscious thinking process or a dysregulation of a single physiological variable. It is expected that augmentation of the number and variety of features to be computed in real time for closed-loop regulation definitely exceeds the capacities of any mental health professional. The presented AI-based approach, if available in post-COVID clinics after its scientific validation, may significantly relieve the overburdened mental health system.
Future research on personalized AI-based multisensory stimulation and multimodal feedback regulation may lead to novel digital clinical tools for more comprehensive patient screening, monitoring and reducing therapist burden, which is in line with calls for development and integration of new technology-enabled models of care to gain substantial improvements in patients’ mental health (Iorfino et al., 2021). An AI-assisted closed-loop psychotherapeutic approach within COVID-19 pandemic may also assist in lower cost, better and faster stratification of ex-COVID-19 patients according to high-medium-low risk of chronic psychopathology. Stratification of risk using the AI-based prediction methods, supplemented with personalized face-to-face risk assessments as necessary, can facilitate matching of ex-COVID-19 individuals with the most appropriate preventive psychotherapeutic intervention in severely resource-limited psychotherapeutic practices. For example, high-risk individuals might require the most effective first-line interventions with extensive involvement of the psychotherapist, while persons with moderately elevated risk might undergo validated online preventive intervention programs with minimal or no psychotherapist involvement. Prediction of risk will benefit from AI tools, as proposed in this paper, keeping in mind the necessity of future work on trustworthiness and generalizability of the developed methods, as discussed in subsection 2.6. On the other hand, the preventive intervention prescribed to a particular ex-COVID-19 patient based on his/her stratified risk might or might not use AI, depending on the selected intervention from the pool of validated preventive approaches that are available in a particular region. The proposed AI-based predictive and preventive approach should be viewed as a part of the whole package of measures focusing on protection of mental health during COVID-19 pandemic, which also include wide-ranging efforts emphasizing the protective potential of, e.g., physical exercise, sleep, and finding the sense of meaning in life (De Jong et al., 2020; Zhang Y. et al., 2020; Rajkumar, 2021), in times of physical distancing, isolations and other negative psycho-socio-economic consequences of attempts to limit the spread and mortality of the virus.
The paper conceptually contributes to the accelerating state-of-the-art research in AI-based prediction of mental health disorders, particularly in ex-COVID-19 patients, calling for further coordinated large scale research efforts in prediction and prevention of mental health disorders, while integrating different categories of multidisciplinary data that have been shown promising in a growing number of separate research studies. The proposed AI-based methodology in prediction and prevention of negative psychological and behavioral consequences in ex-COVID-19 patients, potentially applied in newly established post-COVID clinics, deserves more attention. It expands traditionally used self-rating scales and psychiatric assessment questionnaires and interviews in diagnosis of mental health disorders with more objective multimodal neuro-psycho-physiological metrics based on state-of-the-art AI tools. The proposed prediction protocol may contribute to selection of post-COVID-stress most vulnerable individuals in immediate need for psychiatric treatment and psychological interventions. This approach might also be applied generally for all individuals who were exposed to higher levels of mental health risks during the COVID-19 pandemic and might assist in diagnostic process and in selection of the most appropriate preventive treatment strategies. Early prediction and effective treatment of ex-COVID-19 patients with the proposed methodology, particularly for most vulnerable individuals, is crucially important in prevention of widespread serious mental health diseases. The complexity of such interdisciplinary and multidisciplinary research deserves more global attention, particularly within the World Health Organization. Establishing a multinational interdisciplinary task force for policy design, planning and development of more advanced AI-based innovation in digital psychiatry may result in more successful and efficient strategies in coping with post-COVID overall mental health deterioration, leading to better, faster and more affordable global mental health services for all.
Written informed consent was obtained from the individual(s) for the publication of any potentially identifiable images or data included in this article.
KĆ conceptualized the idea and design of the research study and wrote the first draft of the manuscript. SP, MŠ, IK, MG, BD, and IM wrote sections of the manuscript. TJ and NH provided critical revision of the work. All authors contributed to manuscript revision, read, and approved the submitted version.
The authors declare that the research was conducted in the absence of any commercial or financial relationships that could be construed as a potential conflict of interest.
All claims expressed in this article are solely those of the authors and do not necessarily represent those of their affiliated organizations, or those of the publisher, the editors and the reviewers. Any product that may be evaluated in this article, or claim that may be made by its manufacturer, is not guaranteed or endorsed by the publisher.
Adams, L., and Marketing, S. (2002). Choosing the Right Architecture for Real-Time Signal Processing Designs. Texas Instruments, Document Number SPRA879. Dallas, TX: Texas Instrument.
Afshan, A., Guo, J., Park, S. J., Ravi, V., Flint, J., and Alwan, A. (2018). Effectiveness of voice quality features in detecting depression. Proc. Interspeech 2018, 1676–1680. doi: 10.1192/j.eurpsy.2021.2236
Ahorsu, D. K., Lin, C. Y., Imani, V., Saffari, M., Griffiths, M. D., and Pakpour, A. H. (2020). The fear of COVID-19 scale: development and initial validation. Int. J. Ment. Health Addict. 1–9. doi: 10.1007/s11469-020-00270-8 [Epub ahead of print].
Al-gawwam, S., and Benaissa, M. (2018). “Depression detection from eye blink features,” in Proceedings of the 2018 IEEE International Symposium on Signal Processing and Information Technology (ISSPIT) (Louisville, KY: IEEE), 388–392.
Alghowinem, S., Goecke, R., Wagner, M., Epps, J., Breakspear, M., and Parker, G. (2013). “Detecting depression: a comparison between spontaneous and read speech,” in Proceedings of the 2013 IEEE International Conference on Acoustics, Speech and Signal Processing (Vancouver, BC: IEEE), 7547–7551. doi: 10.1186/s12868-016-0283-6
Andrews, G., and Slade, T. (2001). Interpreting scores on the Kessler psychological distress scale (K10). Austr. N. Zeal. J. Public Health 25, 494–497. doi: 10.1111/j.1467-842x.2001.tb00310.x
Apostolidis, H., Papantoniou, G., and Tsiatsos, T. (2021). Deployment and dynamics of a biofeedback system for anxiety awareness during online examination activities. Appl. Sci. 11:756.
Armstrong, T., Bilsky, S. A., Zhao, M., and Olatunji, B. O. (2013). Dwelling on potential threat cues: an eye movement marker for combat-related PTSD. Depress. Anxiety 30, 497–502. doi: 10.1002/da.22115
Arza, A., Garzón-Rey, J. M., Lázaro, J., Gil, E., Lopez-Anton, R., de la Camara, C., et al. (2019). Measuring acute stress response through physiological signals: towards a quantitative assessment of stress. Med. Biol. Eng. Comp. 57, 271–287. doi: 10.1007/s11517-018-1879-z
Asmundson, G. J., Paluszek, M. M., Landry, C. A., Rachor, G. S., McKay, D., and Taylor, S. (2020). Do pre-existing anxiety-related and mood disorders differentially impact COVID-19 stress responses and coping? J. Anxiety Disord. 74:102271. doi: 10.1016/j.janxdis.2020.102271
Aung, Y. Y., Wong, D., and Ting, D. S. (2021). The promise of artificial intelligence: a review of the opportunities and challenges of artificial intelligence in healthcare. Br. Med. Bull. 139, 4–15. doi: 10.1093/bmb/ldab016
Bãlan, O., Moise, G., Moldoveanu, A., Leordeanu, M., and Moldoveanu, F. (2020). An investigation of various machine and deep learning techniques applied in automatic fear level detection and acrophobia virtual therapy. Sensors 20:496. doi: 10.3390/s20020496
Bäuerle, A., Teufel, M., Musche, V., Weismüller, B., Kohler, H., Hetkamp, M., et al. (2020). Increased generalized anxiety, depression and distress during the COVID-19 pandemic: a cross-sectional study in Germany. J. Public Health 42, 672–678. doi: 10.1093/pubmed/fdaa106
Beck, A. T., Ward, C. H., Mendelson, M., Mock, J., and Erbaugh, J. (1961). An inventory for measuring depression. Arch. Gen. Psychiatry 4, 561–571.
Belsher, B. E., Smolenski, D. J., Pruitt, L. D., Bush, N. E., Beech, E. H., Workman, D. E., et al. (2019). Prediction models for suicide attempts and deaths: a systematic review and simulation. JAMA Psychiatry 76, 642–651. doi: 10.1001/jamapsychiatry.2019.0174
Bickman, L. (2020). Improving mental health services: a 50-year journey from randomized experiments to artificial intelligence and precision mental health. Adm. Policy Ment. Health Ment. Health Servic. Res. 47, 795–843. doi: 10.1007/s10488-020-01065-8
Billings, J., Greene, T., Kember, T., Grey, N., El-Leithy, S., Lee, D., et al. (2020). Supporting hospital staff during COVID-19: early interventions. Occup. Med. 70, 327–329.
Bitan, D. T., Grossman-Giron, A., Bloch, Y., Mayer, Y., Shiffman, N., and Mendlovic, S. (2020). Fear of COVID-19 scale: psychometric characteristics, reliability and validity in the Israeli population. Psychiatr. Res. 289:113100.
Bittencourt, J., Velasques, B., Teixeira, S., Basile, L. F., Salles, J. I., Nardi, A. E., et al. (2013). Saccadic eye movement applications for psychiatric disorders. Neuropsychiatr. Dis. Treat. 9:1393. doi: 10.2147/NDT.S45931
Blevins, C. A., Weathers, F. W., Davis, M. T., Witte, T. K., and Domino, J. L. (2015). The posttraumatic stress disorder checklist for DSM-5 (PCL-5): development and initial psychometric evaluation. J. Traumat. Stress 28, 489–498. doi: 10.1002/jts.22059
Bradford, D. E., Kaye, J. T., and Curtin, J. J. (2014). Not just noise: individual differences in general startle reactivity predict startle response to uncertain and certain threat. Psychophysiology 51, 407–411. doi: 10.1111/psyp.12193
Bradley, M. M., and Lang, P. J. (1999). Affective Norms for English Words (Anew): Instruction Manual and Affective Ratings. Technical Report C-1, The Center for Research in Psychophysiology. Gainesville, FL: University of Florida.
Broadbent, E., Petrie, K. J., Main, J., and Weinman, J. (2006). The brief illness perception questionnaire. J. Psychosomat. Res. 60, 631–637.
Brugnera, A., Zarbo, C., Adorni, R., Compare, A., and Sakatani, K. (2017). Cortical and autonomic stress responses in adults with high versus low levels of trait anxiety: a pilot study. Adv. Exp. Med. Biol. 977, 127–132. doi: 10.1007/978-3-319-55231-6_17
Cai, W., Lian, B., Song, X., Hou, T., Deng, G., and Li, H. (2020). A cross-sectional study on mental health among health care workers during the outbreak of corona virus disease 2019. Asian J. Psychiatry 51:102111. doi: 10.1016/j.ajp.2020.102111
Calvo, R. A., Milne, D. N., Hussain, M. S., and Christensen, H. (2017). Natural language processing in mental health applications using non-clinical texts. Nat. Lang. Eng. 23, 649–685.
Carnevali, L., Thayer, J. F., Brosschot, J. F., and Ottaviani, C. (2018). Heart rate variability mediates the link between rumination and depressive symptoms: a longitudinal study. Int. J. Psychophysiol. 131, 131–138. doi: 10.1016/j.ijpsycho.2017.11.002
Cascardi, M., Armstrong, D., Chung, L., and Paré, D. (2015). Pupil response to threat in trauma-exposed individuals with or without PTSD. J. Traumat. Stress 28, 370–374. doi: 10.1002/jts.22022
Chandler, C., Foltz, P. W., and Elvevåg, B. (2020). Using machine learning in psychiatry: the need to establish a framework that nurtures trustworthiness. Schizophr. Bull. 46, 11–14. doi: 10.1093/schbul/sbz105
Chang, Y. L., Feng, Y. C., and Chen, O. T. C. (2016). “Real-time physiological and facial monitoring for safe driving,” in Proceedings of the 2016 38th Annual International Conference of the IEEE Engineering in Medicine and Biology Society (EMBC) (Orlando, FL: IEEE), 4849–4852. doi: 10.1109/EMBC.2016.7591813
Chen, K. Y., Li, T., Gong, F., Zhang, J. S., and Li, X. K. (2020). Predictors of health-related quality of life and influencing factors for COVID-19 patients, a follow-up at one month. Front. Psychiatry 11:668. doi: 10.3389/fpsyt.2020.00668
Chen, L. C., Hermans, A., Papandreou, G., Schroff, F., Wang, P., and Adam, H. (2018). “Masklab: instance segmentation by refining object detection with semantic and direction features,” in Proceedings of the IEEE Conference on Computer Vision and Pattern Recognition (Piscataway, NJ: IEEE), 4013–4022.
Cheung, T., Lam, S. C., Lee, P. H., Xiang, Y. T., and Yip, P. S. F. (2021). Global imperative of suicidal ideation in 10 countries amid the CoViD-19 pandemic. Front. Psychiatry 11:1558. doi: 10.3389/fpsyt.2020.588781
Choi, S. W., Chi, S. E., Chung, S. Y., Kim, J. W., Ahn, C. Y., and Kim, H. T. (2011). Is alpha wave neurofeedback effective with randomized clinical trials in depression? A pilot study. Neuropsychobiology 63, 43–51. doi: 10.1159/000322290
Clark, D. A. (2013). “Cognitive restructuring,” in The Wiley Handbook of Cognitive Behavioral Therapy, ed. D. Dozois (Hoboken, NJ: John Wiley & Sons, Inc), 1–22.
Connor, K. M., and Davidson, J. R. (2003). Development of a new resilience scale: the Connor-Davidson resilience scale (CD-RISC). Depress. Anxiety 18, 76–82.
Ćosić, K., Popović, S., Horvat, M., Kukolja, D., Dropuljić, B., Kostović, I., et al. (2011). “Virtual reality adaptive stimulation in stress resistance training,” in Proceedings of the RTO-MP-HFM-205 on Mental Health and Well-Being Across the Military Spectrum. Bergen.
Ćosić, K., Popović, S., Horvat, M., Kukolja, D., Dropuljić, B., Kovač, B., et al. (2013a). Computer-aided psychotherapy based on multimodal elicitation, estimation and regulation of emotion. Psychiatr. Danubina 25, 340–346.
Ćosić, K., Popović, S., Horvat, M., Kukolja, D., Dropuljić, B., Kovač, B., et al. (2013b). “Multimodal paradigm for mental readiness training and PTSD prevention,” in New Tools to Enhance Posttraumatic Stress Disorder Diagnosis and Treatment, ed. B. K. Wiederhold (Amsterdam: IOS Press), 102–116.
Ćosić, K., Popović, S., Jovanovic, T., Kukolja, D., and Slamić, M. (2007). Physiology-driven adaptive VR system: technology and rationale for PTSD treatment. Ann. Rev. CyberTher. Telemed. 5, 179–191.
Ćosić, K., Popović, S., Kostović, I., and Judaš, M. (2010b). Virtual reality adaptive stimulation of limbic networks in the mental readiness training. Stud. Health Technol. Inform. 154, 14–19.
Ćosić, K., Popović, S., Kukolja, D., Dropuljić, B., Ivanec, D., and Tonković, M. (2016). Multimodal analysis of startle type responses. Comput. Methods Programs Biomed. 129, 186–202. doi: 10.1016/j.cmpb.2016.01.002
Ćosić, K., Popović, S., Kukolja, D., Horvat, M., and Dropuljić, B. (2010a). Physiology-driven adaptive virtual reality stimulation for prevention and treatment of stress related disorders. Cyber Psychol. Behav. Soc. Netw. 13, 73–78. doi: 10.1089/cyber.2009.0260
Ćosić, K., Popović, S., Šarlija, M., and Kesedžić, I. (2020a). Impact of human disasters and COVID-19 pandemic on mental health: potential of digital psychiatry. Psychiatr. Danubina 32, 25–31. doi: 10.24869/psyd.2020.25
Ćosić, K., Popović, S., Šarlija, M., Kesedžić, I., and Jovanovic, T. (2020b). Artificial intelligence in prediction of mental health disorders induced by the COVID-19 pandemic among health care workers. Croat. Med. J. 61:279. doi: 10.3325/cmj.2020.61.279
Ćosić, K., Popović, S., Šarlija, M., Mijić, I., Kokot, M., Kesedžić, I., et al. (2019b). New tools and methods in selection of air traffic controllers based on multimodal psychophysiological measurements. IEEE Access 7, 174873–174888.
Ćosić, K., Šarlija, M., Ivkovic, V., Zhang, Q., Strangman, G., and Popović, S. (2019a). Stress resilience assessment based on physiological features in selection of air traffic controllers. IEEE Access 7, 41989–42005.
Cummins, N., Epps, J., Breakspear, M., and Goecke, R. (2011). “An investigation of depressed speech detection: features and normalization,” in Proceedings of the 12th Annual Conference of the International Speech Communication Association, Florence.
Cummins, N., Scherer, S., Krajewski, J., Schnieder, S., Epps, J., and Quatieri, T. F. (2015). A review of depression and suicide risk assessment using speech analysis. Speech Commun. 71, 10–49.
Czeisler, M. É, Lane, R. I., Wiley, J. F., Czeisler, C. A., Howard, M. E., and Rajaratnam, S. M. (2021). Follow-up survey of US adult reports of mental health, substance use, and suicidal ideation during the COVID-19 pandemic, September 2020. JAMA Netw. Open 4:e2037665. doi: 10.1001/jamanetworkopen.2020.37665
Daroische, R., Hemminghyth, M. S., Eilertsen, T. H., Breitve, M. H., and Chwiszczuk, L. J. (2021). Cognitive impairment after COVID-19—a review on objective test data. Front. Neurol. 12:699582. doi: 10.3389/fneur.2021.699582
De Jong, E. M., Ziegler, N., and Schippers, M. C. (2020). From shattered goals to meaning in life: life crafting in times of the COVID-19 pandemic. Front. Psychol. 11:577708. doi: 10.3389/fpsyg.2020.577708
Deacon, B. J., Abramowitz, J. S., Woods, C. M., and Tolin, D. F. (2003). The anxiety sensitivity index-revised: psychometric properties and factor structure in two nonclinical samples. Behav. Res. Ther. 41, 1427–1449. doi: 10.1016/s0005-7967(03)00065-2
Dean, K. R., Hammamieh, R., Mellon, S. H., Abu-Amara, D., Flory, J. D., Guffanti, G., et al. (2019). Multi-omic biomarker identification and validation for diagnosing warzone-related post-traumatic stress disorder. Mol. Psychiatry 25, 3337–3349. doi: 10.1038/s41380-019-0496-z
Deng, J., Dong, W., Socher, R., Li, L. J., Li, K., and Fei-Fei, L. (2009). “Imagenet: a large-scale hierarchical image database,” in Proceedings of the 2009 IEEE Conference on Computer Vision and Pattern Recognition (Miami, FL: IEEE), 248–255. doi: 10.1109/TMI.2016.2528162
Denollet, J. (2005). DS14: standard assessment of negative affectivity, social inhibition, and type D personality. Psychosomat. Med. 67, 89–97. doi: 10.1097/01.psy.0000149256.81953.49
Devlin, J., Chang, M. W., Lee, K., and Toutanova, K. (2019). “BERT: pre-training of deep bidirectional transformers for language understanding,” in Proceedings of the 2019 Conference of the North American Chapter of the Association for Computational Linguistics: Human Language Technologies (Minneapolis, MIN: Association for Computational Linguistics), 4171–4186.
Di Tella, M., Romeo, A., Benfante, A., and Castelli, L. (2020). Mental health of healthcare workers during the COVID-19 pandemic in Italy. J. Eval. Clin. Pract. 26, 1583–1587.
Dolev, T., Zubedat, S., Brand, Z., Bloch, B., Mader, E., Blondheim, O., et al. (2021). Physiological parameters of mental health predict the emergence of post-traumatic stress symptoms in physicians treating COVID-19 patients. Transl. Psychiatr. 11, 1–9. doi: 10.1038/s41398-021-01299-6
Dropuljić, B., Mijić, I., Petrinović, D., Jovanovic, T., and Ćosić, K. (2017). Vocal analysis of acoustic startle responses. IEEE Trans. Audio Speech Lang. Process. 26, 318–329.
Duan, L., and Zhu, G. (2020). Psychological interventions for people affected by the COVID-19 epidemic. Lancet Psychiatry 7, 300–302. doi: 10.1016/S2215-0366(20)30073-0
Eckstein, M. K., Guerra-Carrillo, B., Singley, A. T. M., and Bunge, S. A. (2017). Beyond eye gaze: what else can eyetracking reveal about cognition and cognitive development? Dev. Cogn. Neurosci. 25, 69–91. doi: 10.1016/j.dcn.2016.11.001
Edward, K. L. (2005). Resilience: a protector from depression. J. Am. Psychiatr. Nurses Assoc. 11, 241–243.
Eschleman, K. J., Bowling, N. A., and Alarcon, G. M. (2010). A meta-analytic examination of hardiness. Int. J. Stress Manag. 17:277. doi: 10.1371/journal.pone.0069526
Eubank, J. M., Burt, K. G., and Orazem, J. (2021). Examining the psychometric properties of a refined perceived stress scale during the COVID-19 pandemic. J. Prev. Interv. Commun. 49, 179–192. doi: 10.1080/10852352.2021.1908873
Ewbank, M. P., Cummins, R., Tablan, V., Bateup, S., Catarino, A., Martin, A. J., et al. (2020). Quantifying the association between psychotherapy content and clinical outcomes using deep learning. JAMA Psychiatry 77, 35–43. doi: 10.1001/jamapsychiatry.2019.2664
Eyre, H. A., Singh, A. B., and Reynolds, C. III (2016). Tech giants enter mental health. World Psychiatry 15:21. doi: 10.1002/wps.20297
Felitti, V. J., Anda, R. F., Nordenberg, D., Williamson, D. F., Spitz, A. M., Edwards, V., et al. (1998). Relationship of childhood abuse and household dysfunction to many of the leading causes of death in adults: the adverse childhood experiences (ACE) Study. Am. J. Prevent. Med. 14, 245–258.
Felmingham, K. L., Rennie, C., Manor, B., and Bryant, R. A. (2011). Eye tracking and physiological reactivity to threatening stimuli in posttraumatic stress disorder. J. Anxiety Disord. 25, 668–673. doi: 10.1016/j.janxdis.2011.02.010
Ferrari, M., and Quaresima, V. (2020). Hypoxemia in COVID-19: cerebral oximetry should be explored as a warning indicator for mechanically ventilated adults with COVID-19. Respir. Res. 21, 1–2. doi: 10.1186/s12931-020-01530-w
Fitzpatrick, K. K., Darcy, A., and Vierhile, M. (2017). Delivering cognitive behavior therapy to young adults with symptoms of depression and anxiety using a fully automated conversational agent (Woebot): a randomized controlled trial. JMIR Ment. Health 4:e19. doi: 10.2196/mental.7785
Friedman, N. P., and Robbins, T. W. (2021). The role of prefrontal cortex in cognitive control and executive function. Neuropsychopharmacology 47, 72–89. doi: 10.1038/s41386-021-01132-0
Galatzer-Levy, I. R., Karstoft, K. I., Statnikov, A., and Shalev, A. Y. (2014). Quantitative forecasting of PTSD from early trauma responses: a machine learning application. J. Psychiatr. Res. 59, 68–76. doi: 10.1016/j.jpsychires.2014.08.017
Galatzer-Levy, I. R., Ma, S., Statnikov, A., Yehuda, R., and Shalev, A. Y. (2017). Utilization of machine learning for prediction of post-traumatic stress: a re-examination of cortisol in the prediction and pathways to non-remitting PTSD. Transl. Psychiatry 7:e1070. doi: 10.1038/tp.2017.38
Gao, F., Luo, N., Thumboo, J., Fones, C., Li, S. C., and Cheung, Y. B. (2004). Does the 12-item general health questionnaire contain multiple factors and do we need them? Health Qual. Life Outcomes 2, 1–7. doi: 10.1186/1477-7525-2-63
Garcia-Ceja, E., Riegler, M., Nordgreen, T., Jakobsen, P., Oedegaard, K. J., and Tørresen, J. (2018). Mental health monitoring with multimodal sensing and machine learning: a survey. Pervasive Mob. Comput. 51, 1–26. doi: 10.1016/j.pmcj.2018.09.003
Gargot, T., Kisand, H., Miguel, A., Tanyeri, S., Soron, T. R., Serene, B., et al. (2021). Preventing post traumatic stress disorder in the general population induced by trauma during the COVID pandemic: a simple brief intervention based on cognitive science that could be delivered digitally. Eur. J. Trauma Dissociation 5:100193. doi: 10.1016/j.ejtd.2020.100193
Gavrilescu, M., and Vizireanu, N. (2019). Predicting depression, anxiety, and stress levels from videos using the facial action coding system. Sensors 19:3693. doi: 10.3390/s19173693
Gillard, S., Dare, C., Hardy, J., Nyikavaranda, P., Olive, R. R., Shah, P., et al. (2021). Experiences of living with mental health problems during the COVID-19 pandemic in the UK: a coproduced, participatory qualitative interview study. Soc. Psychiatry Psychiatr. Epidemiol. 56, 1447–1457.
Graham, S., Depp, C., Lee, E. E., Nebeker, C., Tu, X., Kim, H. C., et al. (2019). Artificial intelligence for mental health and mental illnesses: an overview. Curr. Psychiatry Rep. 21, 1–18. doi: 10.1007/s11920-019-1094-0
Gramlich, M. A., Neer, S. M., Beidel, D. C., Bohil, C. J., and Bowers, C. A. (2017). A functional near-infrared spectroscopy study of trauma-related auditory and olfactory cues: posttraumatic stress disorder or combat experience? J. Trauma. Stress 30, 656–665. doi: 10.1002/jts.22239
Gray, M., Litz, B., Hsu, J., and Lombardo, T. (2004). Psychometric properties of the life events checklist. Assessment 11, 330–341. doi: 10.1177/1073191104269954
Gross, J. J. (1998). The emerging field of emotion regulation: an integrative review. Rev. Gen. Psychol. 2, 271–299.
Grossman, P., and Taylor, E. W. (2007). Toward understanding respiratory sinus arrhythmia: relations to cardiac vagal tone, evolution and biobehavioral functions. Biol. Psychol. 74, 263–285. doi: 10.1016/j.biopsycho.2005.11.014
Hamilton, J. L., and Alloy, L. B. (2016). Atypical reactivity of heart rate variability to stress and depression across development: systematic review of the literature and directions for future research. Clin. Psychol. Rev. 50, 67–79. doi: 10.1016/j.cpr.2016.09.003
Hampshire, A., Trender, W., Chamberlain, S. R., Jolly, A. E., Grant, J. E., Patrick, F., et al. (2021). Cognitive deficits in people who have recovered from COVID-19. EClinicalMedicine 39:101044. doi: 10.1016/j.eclinm.2021.101044
Hannun, A., Case, C., Casper, J., Catanzaro, B., Diamos, G., Elsen, E., et al. (2014). Deep speech: scaling up end-to-end speech recognition. arXiv [Preprint]. arXiv:1412.5567
Hao, F., Tan, W., Jiang, L., Zhang, L., Zhao, X., Zou, Y., et al. (2020). Do psychiatric patients experience more psychiatric symptoms during COVID-19 pandemic and lockdown? A case-control study with service and research implications for immunopsychiatry. Brain Behav. Immun. 87, 100–106.
He, Q., Veldkamp, B. P., Glas, C. A., and de Vries, T. (2017). Automated assessment of patients’ self-narratives for posttraumatic stress disorder screening using natural language processing and text mining. Assessment 24, 157–172. doi: 10.1177/1073191115602551
Herbert, C., El Bolock, A., and Abdennadher, S. (2021). How do you feel during the COVID-19 pandemic? A survey using psychological and linguistic self-report measures, and machine learning to investigate mental health, subjective experience, personality, and behaviour during the COVID-19 pandemic among university students. BMC Psychol. 9:90. doi: 10.1186/s40359-021-00574-x
Hinrichs, R., van Rooij, S. J., Michopoulos, V., Schultebraucks, K., Winters, S., Maples-Keller, J., et al. (2019). Increased skin conductance response in the immediate aftermath of trauma predicts PTSD risk. Chron. Stress 3:2470547019844441. doi: 10.1177/2470547019844441
Ho, C. S., Lim, L. J., Lim, A. Q., Chan, N. H., Tan, R. S., Lee, S. H., et al. (2020). Diagnostic and predictive applications of functional near-infrared spectroscopy for major depressive disorder: a systematic review. Front. Psychiatry 11:378. doi: 10.3389/fpsyt.2020.00378
Ho, R. C., Sharma, V. K., Tan, B. Y., Ng, A. Y., Lui, Y. S., Husain, S. F., et al. (2021). Comparison of brain activation patterns during olfactory stimuli between recovered COVID-19 patients and healthy controls: a functional near-infrared spectroscopy (fNIRS) study. Brain Sci. 11:968. doi: 10.3390/brainsci11080968
Hodges, L. F., and Rothbaum, B. O. (1998). U.S. Patent No. 5,807,114. Washington, DC: U.S. Patent and Trademark Office.
Hooper, L. M., Stockton, P., Krupnick, J., and Green, B. L. (2011). The development, use, and psychometric properties of the Trauma history questionnaire. J. Loss Trauma 16, 258–283.
Horvat, M. (2017). “A brief overview of affective multimedia databases,” in Proceedings of the Central European Conference on Information and Intelligent Systems Faculty of Organization and Informatics, Varazdin, 3–9.
Horvat, M., Bogunović, N., and Ćosić, K. (2014). STIMONT: a core ontology for multimedia stimuli description. Multimed. Tools Appl. 73, 1103–1127.
Horvat, M., Grbin, A., and Gledec, G. (2012). WNtags: a web-based tool for image labeling and retrieval with lexical ontologies in Advances in Knowledge-Based and Intelligent Information and Engineering Systems eds M. Graña, C. Toro, J. Posada, R. J. Howlett, and L.C. Jain (Amsterdam: IOS Press) 585–594.
Horvat, M., Popović, S., Bogunović, N., and Ćosić, K. (2009). “Tagging multimedia stimuli with ontologies,” in Proceedings of the 32nd International Convention on Information & Communication Technology Electronics & Microelectronics (MIPRO) (Piscataway, NJ: IEEE), 203–208.
Hubbard, G., den Daas, C., Johnston, M., and Dixon, D. (2021). Sociodemographic and psychological risk factors for anxiety and depression: findings from the Covid-19 health and adherence research in Scotland on mental health (CHARIS-MH) cross-sectional survey. Int. J. Behav. Med. 28, 788–800. doi: 10.1007/s12529-021-09967-z
Iorfino, F., Occhipinti, J. A., Skinner, A., Davenport, T., Rowe, S., Prodan, A., et al. (2021). The impact of technology-enabled care coordination in a complex mental health system: a local system dynamics model. J. Med. Internet Res. 23:e25331. doi: 10.2196/25331
Jaiswal, S., Song, S., and Valstar, M. (2019). “Automatic prediction of depression and anxiety from behaviour and personality attributes,” in Proceedings of the 2019 8th International Conference on Affective Computing and Intelligent Interaction (ACII) (Cambridge: IEEE), 1–7.
Janiri, D., Kotzalidis, G. D., Giuseppin, G., Molinaro, M., Modica, M., Montanari, S., et al. (2020). Psychological distress after Covid-19 recovery: reciprocal effects with temperament and emotional dysregulation. An exploratory study of patients over 60 years of age assessed in a post-acute care service. Front. Psychiatry 11:590135. doi: 10.3389/fpsyt.2020.590135
Jha, I. P., Awasthi, R., Kumar, A., Kumar, V., and Sethi, T. (2021). Learning the mental health impact of COVID-19 in the United States with explainable artificial intelligence: observational study. JMIR Ment. Health 8:e25097. doi: 10.2196/25097
Jiang, T., Gradus, J. L., and Rosellini, A. J. (2020). Supervised machine learning: a brief primer. Behav. Ther. 51, 675–687. doi: 10.1016/j.beth.2020.05.002
Just, M. A., Pan, L., Cherkassky, V. L., McMakin, D. L., Cha, C., Nock, M. K., et al. (2017). Machine learning of neural representations of suicide and emotion concepts identifies suicidal youth. Nat. Hum. Behav. 1, 911–919. doi: 10.1038/s41562-017-0234-y
Kalisch, R., Müller, M. B., and Tüscher, O. (2015). A conceptual framework for the neurobiological study of resilience. Behav. Brain Sci. 38:e92. doi: 10.1017/S0140525X1400082X
Karstoft, K. I., Galatzer-Levy, I. R., Statnikov, A., Li, Z., and Shalev, A. Y. (2015). Bridging a translational gap: using machine learning to improve the prediction of PTSD. BMC Psychiatry 15:30. doi: 10.1186/s12888-015-0399-8
Kessler, R. C., Hwang, I., Hoffmire, C. A., McCarthy, J. F., Petukhova, M. V., Rosellini, A. J., et al. (2017). Developing a practical suicide risk prediction model for targeting high-risk patients in the Veterans health Administration. Int. J. Methods Psychiatr. Res. 26:e1575. doi: 10.1002/mpr.1575
Kesedžić, I., Božek, J., Radoš, M., Popović, S., and Ćosić, K. (2020b). “Air traffic control competencies measurements based on functional near-infrared spectroscopy,” in Proceedings of the 2020 43rd International Convention on Information, Communication and Electronic Technology (MIPRO) (Opatija: IEEE), 243–248. doi: 10.23919/MIPRO48935.2020.9245242
Kesedžić, I., Šarlija, M., Božek, J., Popović, S., and Ćosić, K. (2020a). Classification of cognitive load based on neurophysiological features from functional near-infrared spectroscopy and electrocardiography signals on n-back task. IEEE Sens. J. 21, 14131–14140. doi: 10.1109/JSEN.2020.3038032
Kim, H. Y., Seo, K., Jeon, H. J., Lee, U., and Lee, H. (2017). Application of functional near-infrared spectroscopy to the study of brain function in humans and animal models. Mol. Cells 40, 523–532. doi: 10.14348/molcells.2017.0153
Kimble, M. O., Fleming, K., Bandy, C., Kim, J., and Zambetti, A. (2010). Eye tracking and visual attention to threating stimuli in veterans of the Iraq war. J. Anxiety Disord. 24, 293–299. doi: 10.1016/j.janxdis.2009.12.006
Kleineidam, L., Frommann, I., Ruhrmann, S., Klosterkötter, J., Brockhaus-Dumke, A., Wölwer, W., et al. (2019). Antisaccade and prosaccade eye movements in individuals clinically at risk for psychosis: comparison with first-episode schizophrenia and prediction of conversion. Eur. Arch.psychiatr. Clin. Neurosci. 269, 921–930. doi: 10.1007/s00406-018-0973-4
Klok, F. A., Boon, G. J., Barco, S., Endres, M., Geelhoed, J. M., Knauss, S., et al. (2020). The Post-COVID-19 functional status scale: a tool to measure functional status over time after COVID-19. Eur. Respir. J. 56:2001494. doi: 10.1183/13993003.01494-2020
Kojima, M., Shioiri, T., Hosoki, T., Sakai, M., Bando, T., and Someya, T. (2002). Blink rate variability in patients with panic disorder: new trial using audiovisual stimulation. Psychiatr. Clin. Neurosci. 56, 545–549. doi: 10.1046/j.1440-1819.2002.01052.x
Kolacz, J., Dale, L. P., Nix, E. J., Roath, O. K., Lewis, G. F., and Porges, S. W. (2020). Adversity history predicts self-reported autonomic reactivity and mental health in US residents during the COVID-19 pandemic. Front. Psychiatry 11:577728. doi: 10.3389/fpsyt.2020.577728
Kraepelin, E. (1921). Manic depressive insanity and paranoia. J. Nervous Ment. Dis. 53:350. doi: 10.1016/j.jad.2006.09.014
Kumar, S., Varshney, D., Dhawan, G., and Jalutharia, H. (2020). “Analysing the effective psychological state of students using facial features,” in Proceedings of the 2020 4th International Conference on Intelligent Computing and Control Systems (ICICCS) (Madurai: IEEE), 648–653.
Lalmuanawma, S., Hussain, J., and Chhakchhuak, L. (2020). Applications of machine learning and artificial intelligence for Covid-19 (SARS-CoV-2) pandemic: a review. Chaos Solitons Fractals 139:110059. doi: 10.1016/j.chaos.2020.110059
Lau, H. M., Smit, J. H., Fleming, T. M., and Riper, H. (2017). Serious games for mental health: are they accessible, feasible, and effective? A systematic review and meta-analysis. Front. Psychiatry 7:209. doi: 10.3389/fpsyt.2016.00209
Lee, E. E., Torous, J., De Choudhury, M., Depp, C. A., Graham, S. A., Kim, H. C., et al. (2021). Artificial intelligence for mental healthcare: clinical applications, barriers, facilitators, and artificial wisdom. Biol. Psychiatr. Cogn. Neurosci. Neuroimaging 6, 856–864. doi: 10.1016/j.bpsc.2021.02.001
Lee, J. H., and Lee, J. H. (2014). Attentional bias towards emotional facial expressions in survivors of dating violence. Cogn. Emotion 28, 1127–1136. doi: 10.1080/02699931.2013.867834
Li, J., Theng, Y. L., and Foo, S. (2014). Game-based digital interventions for depression therapy: a systematic review and meta-analysis. Cyberpsychol. Behav. Soc. Netw. 17, 519–527. doi: 10.1089/cyber.2013.0481
Li, J. B., Yang, A., Dou, K., and Cheung, R. Y. (2020). Self-control moderates the association between perceived severity of coronavirus disease 2019 (COVID-19) and mental health problems among the Chinese public. Int. J. Environ. Res. Public Health 17, 4820–4829. doi: 10.3390/ijerph17134820
Li, Y., Xu, Y., Xia, M., Zhang, T., Wang, J., Liu, X., et al. (2016). Eye movement indices in the study of depressive disorder. Shanghai Arch. Psychiatry 28:326. doi: 10.11919/j.issn.1002-0829.216078
Lin, Y., Hu, Z., Alias, H., and Wong, L. P. (2020). Knowledge, attitudes, impact, and anxiety regarding COVID-19 infection among the public in China. Front. Public Health 8:236. doi: 10.3389/fpubh.2020.00236
Liotta, E. M., Batra, A., Clark, J. R., Shlobin, N. A., Hoffman, S. C., Orban, Z. S., et al. (2020). Frequent neurologic manifestations and encephalopathy-associated morbidity in Covid-19 patients. Ann. Clin. Transl. Neurol. 7, 2221–2230. doi: 10.1002/acn3.51210
Liu, C. H., Zhang, E., Wong, G. T. F., and Hyun, S. (2020). Factors associated with depression, anxiety, and PTSD symptomatology during the COVID-19 pandemic: clinical implications for US young adult mental health. Psychiatry Res. 290:113172. doi: 10.1016/j.psychres.2020.113172
Liu, N., Zhang, F., Wei, C., Jia, Y., Shang, Z., Sun, L., et al. (2020). Prevalence and predictors of PTSS during COVID-19 outbreak in China hardest-hit areas: gender differences matter. Psychiatry Res. 287:112921. doi: 10.1016/j.psychres.2020.112921
Low, D. M., Rumker, L., Talkar, T., Torous, J., Cecchi, G., and Ghosh, S. S. (2020). Natural language processing reveals vulnerable mental health support groups and heightened health anxiety on reddit during covid-19: observational study. J. Med. Internet Res. 22:e22635. doi: 10.2196/22635
Lucas, G. M., Rizzo, A., Gratch, J., Scherer, S., Stratou, G., Boberg, J., et al. (2017). Reporting mental health symptoms: breaking down barriers to care with virtual human interviewers. Front. Robot. AI 4:51. doi: 10.3389/frobt.2017.00051
Lundberg, S. M., and Lee, S. I. (2017). “A unified approach to interpreting model predictions,” in Proceedings of the 31st International Conference on Neural Information Processing Systems (Long Beach, CA), 4768–4777.
Lynch, K. S., and Lachman, M. E. (2020). The effects of lifetime trauma exposure on cognitive functioning in midlife. J. Trauma. Stress 33, 773–782. doi: 10.1002/jts.22522
Machado, C. D. S., Ballester, P. L., Cao, B., Mwangi, B., Caldieraro, M. A., Kapczinski, F., et al. (2021). Prediction of suicide attempts in a prospective cohort study with a nationally representative sample of the US population. Psychol. Med. 1–12. doi: 10.1017/S0033291720004997 [Epub ahead of print].
Mackintosh, J. H., Kumar, R., and Kitamura, T. (1983). Blink rate in psychiatric illness. Br. J. Psychiatry 143, 55–57. doi: 10.1192/bjp.143.1.55
Maloney, P. W., Grawitch, M. J., and Barber, L. K. (2012). The multi-factor structure of the brief self-control scale: discriminant validity of restraint and impulsivity. J. Res. Pers. 46, 111–115.
Marco, C. A., Larkin, G. L., Feeser, V. R., Monti, J. E., Vearrier, L., et al. Acep Ethics (2020). Post-traumatic stress and stress disorders during the COVID-19 pandemic: survey of emergency physicians. J. Am. Coll. Emerg. Phys. Open 1, 1594–1601. doi: 10.1002/emp2.12305
Marmar, C. R., Brown, A. D., Qian, M., Laska, E., Siegel, C., Li, M., et al. (2019). Speech-based markers for posttraumatic stress disorder in US veterans. Depress. Anxiety 36, 607–616. doi: 10.1002/da.22890
Marmar, C. R., Weiss, D. S., and Metzler, T. J. (1997). “The Peritraumatic dissociative experiences questionnaire,” in Assessing Psychological Trauma and Posttraumatic Stress Disorder, eds J. P. Wilson and C. R. Marmar (New York, NY: The Guilford Press), 412–428.
Matsuo, K., Taneichi, K., Matsumoto, A., Ohtani, T., Yamasue, H., Sakano, Y., et al. (2003). Hypoactivation of the prefrontal cortex during verbal fluency test in PTSD: a near-infrared spectroscopy study. Psychiatry Res. Neuroimaging 124, 1–10. doi: 10.1016/s0925-4927(03)00093-3
Maulik, P. K., Thornicroft, G., and Saxena, S. (2020). Roadmap to strengthen global mental health systems to tackle the impact of the COVID-19 pandemic. Int. J. Ment. Health Syst. 14:57. doi: 10.1186/s13033-020-00393-4
Mazza, M. G., De Lorenzo, R., Conte, C., Poletti, S., Vai, B., Bollettini, I., et al. (2020). Anxiety and depression in COVID-19 survivors: role of inflammatory and clinical predictors. Brain Behav. Immun. 89, 594–600. doi: 10.1016/j.bbi.2020.07.037
McCarthy, E., DeViva, J. C., Na, P. J., and Pietrzak, R. H. (2021). New-onset and exacerbated insomnia symptoms during the COVID-19 pandemic in US military veterans: a nationally representative, prospective cohort study. J. Sleep Res. e13450. doi: 10.1111/jsr.13450 [Epub ahead of print].
McKinlay, A. R., Fancourt, D., and Burton, A. (2021). A qualitative study about the mental health and wellbeing of older adults in the UK during the COVID-19 pandemic. BMC Geriatr. 21:439. doi: 10.1186/s12877-021-02367-8
Mckinnon, A. I., Gray, N. S., and Snowden, R. J. (2020). Enhanced emotional response to both negative and positive images in post-traumatic stress disorder: evidence from pupillometry. Biol. Psychol. 154:107922. doi: 10.1016/j.biopsycho.2020.107922
Meichenbaum, D. (2017). “Stress inoculation training: a preventative and treatment approach” The Evolution of Cognitive Behavior Therapy eds P. M. Lehrer, R. L. Woolfolk, and W. E. Sime (New York, NY: Routledge), 101–124.
Mennin, D. S. (2006). Emotion regulation therapy: an integrative approach to treatment-resistant anxiety disorders. J. Contemp. Psychother. 36, 95–105.
Mijić, I., Šarlija, M., and Petrinović, D. (2017). “Classification of cognitive load using voice features: a preliminary investigation,” in Proceedings of the 2017 8th IEEE International Conference on Cognitive Infocommunications (CogInfoCom), (Debrecen: IEEE), 000345–000350.
Mijić, I., Šarlija, M., and Petrinović, D. (2019). “MMOD-COG: a database for multimodal cognitive load classification,” in Proceedings of the 2019 11th International Symposium on Image and Signal Processing and Analysis (ISPA) (Dubrovnik: IEEE), 15–20.
Mikolov, T., Sutskever, I., Chen, K., Corrado, G. S., and Dean, J. (2013). “Distributed representations of words and phrases and their compositionality,” in Proceedings of the Advances in Neural Information Processing Systems (Red Hook, NY: Curran Associates Inc), 3111–3119.
Mukherjee, H., Salam, H., and Santosh, K. C. (2021a). Lung health analysis: adventitious respiratory sound classification using filterbank energies. Int. J. Pattern Recog. Artif. Intell. 2157008. doi: 10.1142/S0218001421570081 [Epub ahead of print].
Mukherjee, H., Sreerama, P., Dhar, A., Obaidullah, S. M., Roy, K., Mahmud, M., et al. (2021b). Automatic lung health screening using respiratory sounds. J. Med. Syst. 45:19. doi: 10.1007/s10916-020-01681-9
Nishimura, Y., Tanii, H., Fukuda, M., Kajiki, N., Inoue, K., Kaiya, H., et al. (2007). Frontal dysfunction during a cognitive task in drug-naive patients with panic disorder as investigated by multi-channel near-infrared spectroscopy imaging. Neurosci. Res. 59, 107–112. doi: 10.1016/j.neures.2007.05.016
Nishizawa, Y., Kanazawa, T., Kawabata, Y., Matsubara, T., Maruyama, S., Kawano, M., et al. (2019). fNIRS assessment during an emotional stroop task among patients with depression: replication and extension. Psychiatry Investig. 16:80. doi: 10.30773/pi.2018.11.12.2
Pennebaker, J. W., Boyd, R. L., Jordan, K., and Blackburn, K. (2015). The Development and Psychometric Properties of LIWC2015. Austin, TX: University of Texas at Austin.
Pierce, M., Hope, H., Ford, T., Hatch, S., Hotopf, M., John, A., et al. (2020). Mental health before and during the COVID-19 pandemic: a longitudinal probability sample survey of the UK population. Lancet Psychiatry 7, 883–892. doi: 10.1016/S2215-0366(20)30308-4
Pole, N., Neylan, T. C., Otte, C., Henn-Hasse, C., Metzler, T. J., and Marmar, C. R. (2009). Prospective prediction of posttraumatic stress disorder symptoms using fear potentiated auditory startle responses. Biol. Psychiatry 65, 235–240. doi: 10.1016/j.biopsych.2008.07.015
Popović, S., Horvat, M., Kukolja, D., Dropuljić, B., and Cosić, K. (2009). Stress inoculation training supported by physiology-driven adaptive virtual reality stimulation. Stud. Health Technol. Inf. 144, 50–54.
Popovic, S., Slamic, M., and Cosic, K. (2006). “Scenario self-adaptation in virtual reality exposure therapy for posttraumatic stress disorder,” in Novel Approaches to the Diagnosis and Treatment of Posttraumatic Stress Disorder, ed. M. J. Roy (Amsterdam: IOS Press), 135–147.
Powers, A., Fani, N., Murphy, L., Briscione, M., Bradley, B., Tone, E. B., et al. (2019). Attention bias toward threatening faces in women with PTSD: eye tracking correlates by symptom cluster. Eur. J. Psychotraumatol. 10:1568133. doi: 10.1080/20008198.2019.1568133
Prout, T. A., Zilcha-Mano, S., Aafjes-van Doorn, K., Békés, V., Christman-Cohen, I., Whistler, K., et al. (2020). Identifying predictors of psychological distress during COVID-19: a machine learning approach. Front. Psychol. 11:586202. doi: 10.3389/fpsyg.2020.586202
Qiu, J., Shen, B., Zhao, M., Wang, Z., Xie, B., and Xu, Y. (2020). A nationwide survey of psychological distress among Chinese people in the COVID-19 epidemic: implications and policy recommendations. Gen. Psychiatry 33:e100213.
Rainey, S., and Erden, Y. J. (2020). Correcting the brain? The convergence of neuroscience, neurotechnology, psychiatry, and artificial intelligence. Sci. Eng. Ethics 26, 2439–2454. doi: 10.1007/s11948-020-00240-2
Rajkowski, J., Kubiak, P., and Aston-Jones, G. (1993). Correlations between locus coeruleus (LC) neural activity, pupil diameter and behavior in monkey support a role of LC in attention. Soc. Neurosci. 19:974.
Rajkumar, R. P. (2021). Sleep, physical activity and mental health during the COVID-19 pandemic: complexities and opportunities for intervention. Sleep Med. 77:307. doi: 10.1016/j.sleep.2020.10.004
Ramos-Lima, L. F., Waikamp, V., Antonelli-Salgado, T., Passos, I. C., and Freitas, L. H. M. (2020). The use of machine learning techniques in trauma-related disorders: a systematic review. J. Psychiatr. Res. 121, 159–172. doi: 10.1016/j.jpsychires.2019.12.001
Ranieri, J., Guerra, F., and Di Giacomo, D. (2021). Predictive risk factors for post-traumatic stress symptoms among nurses during the Italian acute COVID-19 outbreak. Health Psychol. Rep. 9, 180–185.
Renna, M. E., Fresco, D. M., and Mennin, D. S. (2020). Emotion regulation therapy and its potential role in the treatment of chronic stress-related pathology across disorders. Chron. Stress 4:2470547020905787. doi: 10.1177/2470547020905787
Renna, M. E., Quintero, J. M., Fresco, D. M., and Mennin, D. S. (2017). Emotion regulation therapy: a mechanism-targeted treatment for disorders of distress. Front. Psychol. 8:98. doi: 10.3389/fpsyg.2017.00098
Rezaei, S., and Vakilian, M. (2021). The mediating role of illness perception in the relationship between psychological hardiness and the health status of covid-19 (coronavirus disease) survived patients. Health Psychol. Res. 9:24839.
Ribeiro, M. T., Singh, S., and Guestrin, C. (2016). ““Why should i trust you?” Explaining the predictions of any classifier,” in Proceedings of the 22nd ACM SIGKDD International Conference on Knowledge Discovery and Data Mining (New York, NY: ACM), 1135–1144.
Riehm, K. E., Brenneke, S. G., Adams, L. B., Gilan, D., Lieb, K., Kunzler, A. M., et al. (2021). Association between psychological resilience and changes in mental distress during the COVID-19 pandemic. J. Affect. Disord. 282, 381–385. doi: 10.1016/j.jad.2020.12.071
Risbrough, V. B., Baker, D. G., Nievergelt, C. M., Litz, B., Nash, W. P., Perez, J., et al. (2012). Prospective assessment of psychophysiological risk factors for PTSD. Eur. J. Psychotraumatol. 3, (Suppl. 1) doi: 10.3402/ejpt.v3i0.19473
Risbrough, V., Geyer, M., Acheson, D., Minassian, A., Maihofer, A., Baker, D., et al. (2017). 88. Identification of psychophysiological markers of PTSD risk and potential use as intermediate phenotypes. Biol. Psychiatry 81:S37.
Robert, L. P. Jr., Bansal, G., and Lütge, C. (2020). ICIS 2019 SIGHCI workshop panel report: human–computer interaction challenges and opportunities for fair, trustworthy and ethical artificial intelligence. AIS Trans. Hum. Comp. Interact. 12, 96–108.
Roberti, J. W., Harrington, L. N., and Storch, E. A. (2006). Further psychometric support for the 10-item version of the perceived stress scale. J. Coll. Couns. 9, 135–147.
Roessner, V., Rothe, J., Kohls, G., Schomerus, G., Ehrlich, S., and Beste, C. (2021). Taming the chaos? Using eXplainable artificial intelligence (XAI) to tackle the complexity in mental health research. Eur. Child Adolesc. Psychiatry 30, 1143–1146. doi: 10.1007/s00787-021-01836-0
Rothbaum, B. O., and Schwartz, A. C. (2002). Exposure therapy for posttraumatic stress disorder. Am. J. Psychother. 56, 59–75.
Rubin, R. (2020). As their numbers grow, COVID-19 “long haulers” stump experts. JAMA 324, 1381–1383. doi: 10.1001/jama.2020.17709
Russo, S. J., Murrough, J. W., Han, M. H., Charney, D. S., and Nestler, E. J. (2012). Neurobiology of resilience. Nat. Neurosci. 15, 1475–1484.
Santosh, K. C. (2020). AI-driven tools for coronavirus outbreak: need of active learning and cross-population train/test models on multitudinal/multimodal data. J. Med. Syst. 44:93. doi: 10.1007/s10916-020-01562-1
Šarlija, M., Popović, S., Jagodić, M., Jovanovic, T., Ivkovic, V., Zhang, Q., et al. (2021). Prediction of task performance from physiological features of stress resilience. IEEE J. Biomed. Health Inf. 25, 2150–2161. doi: 10.1109/JBHI.2020.3041315
Scherer, K. R. (1986). Vocal affect expression: a review and a model for future research. Psychol. Bull. 99:143.
Scherer, S., Lucas, G. M., Gratch, J., Rizzo, A. S., and Morency, L.-P. (2015). Self-reported symptoms of depression and PTSD are associated with reduced vowel space in screening interviews. IEEE Trans. Affect. Comput. 7, 59–73.
Schultebraucks, K., Choi, K. W., Galatzer-Levy, I. R., and Bonanno, G. A. (2021). Discriminating heterogeneous trajectories of resilience and depression after major life stressors using polygenic scores. JAMA Psychiatry 78, 744–752. doi: 10.1001/jamapsychiatry.2021.0228
Schultebraucks, K., Shalev, A. Y., Michopoulos, V., Grudzen, C. R., Shin, S. M., Stevens, J. S., et al. (2020a). A validated predictive algorithm of post-traumatic stress course following emergency department admission after a traumatic stressor. Nat. Med. 26, 1084–1088. doi: 10.1038/s41591-020-0951-z
Schultebraucks, K., Yadav, V., Shalev, A. Y., Bonanno, G. A., and Galatzer-Levy, I. R. (2020b). Deep learning-based classification of posttraumatic stress disorder and depression following trauma utilizing visual and auditory markers of arousal and mood. Psychol. Med. 1–11. doi: 10.1017/S0033291720002718 [Epub ahead of print].
Sears, C. R., Newman, K. R., Ference, J. D., and Thomas, C. L. (2011). Attention to emotional images in previously depressed individuals: an eye-tracking study. Cogn. Ther. Res. 35, 517–528. doi: 10.1016/j.jad.2021.04.063
Seligowski, A. V., Steuber, E. R., Hinrichs, R., Reda, M. H., Wiltshire, C. N., Wanna, C. P., et al. (2021). A prospective examination of sex differences in posttraumatic autonomic functioning. Neurobiol. Stress 15:100384. doi: 10.1016/j.ynstr.2021.100384
Shaffer, F., and Ginsberg, J. P. (2017). An overview of heart rate variability metrics and norms. Front. Public Health 5:258. doi: 10.3389/fpubh.2017.00258
Shalev, A. Y., Peri, T., Brandes, D., Freedman, S., Orr, S. P., and Pitman, R. K. (2000). Auditory startle response in trauma survivors with posttraumatic stress disorder: a prospective study. Am. J. Psychiatry 157, 255–261. doi: 10.1176/appi.ajp.157.2.255
Shalev, A. Y., Sahar, T., Freedman, S., Peri, T., Glick, N., Brandes, D., et al. (1998). A prospective study of heart rate response following trauma and the subsequent development of posttraumatic stress disorder. Arch. Gen. Psychiatry 55, 553–559. doi: 10.1001/archpsyc.55.6.553
Shatte, A. B., Hutchinson, D. M., and Teague, S. J. (2019). Machine learning in mental health: a scoping review of methods and applications. Psychol. Med. 49, 1426–1448. doi: 10.1017/S0033291719000151
Sheehan, D. V., Lecrubier, Y., Sheehan, K. H., Amorim, P., Janavs, J., Weiller, E., et al. (1998). The mini-international neuropsychiatric interview (MINI): the development and validation of a structured diagnostic psychiatric interview for DSM-IV and ICD-10. J. Clin. Psychiatry 59, 22–33.
Sherman, A. C., Williams, M. L., Amick, B. C., Hudson, T. J., and Messias, E. L. (2020). Mental health outcomes associated with the COVID-19 pandemic: prevalence and risk factors in a southern US state. Psychiatry Res. 293, 113476–113483. doi: 10.1016/j.psychres.2020.113476
Simons, J. S., and Gaher, R. M. (2005). The distress tolerance scale: development and validation of a self-report measure. Motiv. Emot. 29, 83–102.
Smith, B. W., Dalen, J., Wiggins, K., Tooley, E., Christopher, P., and Bernard, J. (2008). The brief resilience scale: assessing the ability to bounce back. Int. J. Behav. Med. 15, 194–200. doi: 10.1080/10705500802222972
Souza, G. G. L., Magalhaes, L. N., Da Cruz, T. A. R., Mendonça-De-Souza, A. C. F., Duarte, A. F. A., Fischer, N. L., et al. (2013). Resting vagal control and resilience as predictors of cardiovascular allostasis in peacekeepers. Stress 16, 377–383. doi: 10.3109/10253890.2013.767326
Speilberger, C. D., and Vagg, P. R. (1984). Psychometric properties of the STAI: a reply to Ramanaiah, Franzen, and Schill. J. Pers. Assess. 48, 95–97. doi: 10.1207/s15327752jpa4801_16
Spitzer, R. L., Kroenke, K., Williams, J. B., and Löwe, B. (2006). A brief measure for assessing generalized anxiety disorder: the GAD-7. Arch. Internal Med. 166, 1092–1097. doi: 10.1001/archinte.166.10.1092
Stolicyn, A., Steele, J. D., and Seriès, P. (2020). Prediction of depression symptoms in individual subjects with face and eye movement tracking. Psychol. Med. 1–9. doi: 10.1017/S0033291720003608 [Epub ahead of print].
Strapparava, C., and Valitutti, A. (2004). “WordNet-affect: an affective extension of WordNet,” in Proceedings of the LREC 2004-4th IInternational Conference on Language Resources and Evaluation, Vol. 4, Lisbon, 1083–1086.
Takahashi, J., Hirano, Y., Miura, K., Morita, K., Fujimoto, M., Yamamori, H., et al. (2021). Eye movement abnormalities in major depressive disorder. Front. Psychiatry 12:673443. doi: 10.3389/fpsyt.2021.673443
Tan, G., Dao, T. K., Farmer, L., Sutherland, R. J., and Gevirtz, R. (2011). Heart rate variability (HRV) and posttraumatic stress disorder (PTSD): a pilot study. Appl. Psychophysiol. Biofeedback 36, 27–35. doi: 10.1007/s10484-010-9141-y
Thenral, M., and Annamalai, A. (2020). Telepsychiatry and the role of artificial intelligence in mental health in post-COVID-19 India: a scoping review on opportunities. Indian J. Psychol. Med. 42:428. doi: 10.1177/0253717620952160
Thoern, H. A., Grueschow, M., Ehlert, U., Ruff, C. C., and Kleim, B. (2016). Attentional bias towards positive emotion predicts stress resilience. PLoS One 11:e0148368. doi: 10.1371/journal.pone.0148368
Tian, F., Yennu, A., Smith-Osborne, A., Gonzalez-Lima, F., North, C. S., and Liu, H. (2014). Prefrontal responses to digit span memory phases in patients with post-traumatic stress disorder (PTSD): a functional near infrared spectroscopy study. Neuroimage Clin. 4, 808–819. doi: 10.1016/j.nicl.2014.05.005
Tjoa, E., and Guan, C. (2020). A survey on explainable artificial intelligence (XAI): toward medical XAI. IEEE Trans. Neural Netw. Learn. Syst. 32, 4793–4813. doi: 10.1109/TNNLS.2020.3027314
Tuman, T. C. (2021). The effect of type D personality on anxiety, depression and fear of COVID-19 disease in healthcare workers. Arch. Environ. Occup. Health 1–8. doi: 10.1080/19338244.2021.1952152 [Epub ahead of print].
van Rooij, S. J., Ravi, M., Ely, T. D., Michopoulos, V., Winters, S. J., Shin, J., et al. (2021). Hippocampal activation during contextual fear inhibition related to resilience in the early aftermath of trauma. Behav. Brain Res. 408:113282. doi: 10.1016/j.bbr.2021.113282
van Rooij, S. J., Stevens, J. S., Ely, T. D., Hinrichs, R., Michopoulos, V., Winters, S. J., et al. (2018). The role of the hippocampus in predicting future posttraumatic stress disorder symptoms in recently traumatized civilians. Biol. Psychiatry 84, 106–115. doi: 10.1016/j.biopsych.2017.09.005
Veer, I. M., Riepenhausen, A., Zerban, M., Wackerhagen, C., Puhlmann, L. M., Engen, H., et al. (2021). Psycho-social factors associated with mental resilience in the Corona lockdown. Transl. Psychiatry 11:67. doi: 10.1038/s41398-020-01150-4
Walker, F. R., Pfingst, K., Carnevali, L., Sgoifo, A., and Nalivaiko, E. (2017). In the search for integrative biomarker of resilience to psychological stress. Neurosci. Biobehav. Rev. 74, 310–320. doi: 10.1016/j.neubiorev.2016.05.003
Walter, K. (2021). An inside look at a post–COVID-19 clinic. JAMA 325, 2036–2037. doi: 10.1001/jama.2021.2426
Wang, C., Pan, R., Wan, X., Tan, Y., Xu, L., McIntyre, R. S., et al. (2020). A longitudinal study on the mental health of general population during the COVID-19 epidemic in China. Brain Behav. Immun. 87, 40–48.
Ware, J. Jr., Kosinski, M., and Keller, S. D. (1996). A 12-Item short-form health survey: construction of scales and preliminary tests of reliability and validity. Med. Care 34, 220–233. doi: 10.1097/00005650-199603000-00003
Ware, J. E. Jr., and Sherbourne, C. D. (1992). The MOS 36-item short-form health survey (SF-36): I. Conceptual framework and item selection. Med. Care 30, 473–483.
Warren, A. M., Zolfaghari, K., Fresnedo, M., Bennett, M., Pogue, J., Waddimba, A., et al. (2021). Anxiety sensitivity, COVID-19 fear, and mental health: results from a United States population sample. Cogn. Behav. Ther. 50, 204–216. doi: 10.1080/16506073.2021.1874505
Weiss, D. S. (2004). “The impact of event scale-revised,” in Assessing Psychological Trauma and PTSD, eds J. P. Wilson and T. M. Keane (New York, NY: The Guilford Press), 168–189.
Winkler, P., Formanek, T., Mlada, K., Kagstrom, A., Mohrova, Z., Mohr, P., et al. (2020). Increase in prevalence of current mental disorders in the context of COVID-19: analysis of repeated nationwide cross-sectional surveys. Epidemiol. Psychiatr. Sci. 29:e173. doi: 10.1017/S2045796020000888
Wu, C., Hu, X., Song, J., Yang, D., Xu, J., Cheng, K., et al. (2020). Mental health status and related influencing factors of COVID-19 survivors in Wuhan, China. Clin. Transl. Med. 10:e52. doi: 10.1002/ctm2.52
Wu, T., Jia, X., Shi, H., Niu, J., Yin, X., Xie, J., et al. (2020). Prevalence of mental health problems during the COVID-19 pandemic: a systematic review and meta-analysis. J. Affect. Disord. 281, 91–98. doi: 10.1016/j.jad.2020.11.117
Xu, W. (2019). Toward human-centered AI: a perspective from human-computer interaction. Interactions 26, 42–46.
Yao, L., Wan, Y., Ni, H., and Xu, B. (2021). Action unit classification for facial expression recognition using active learning and SVM. Multimed. Tools Appl. 80, 1–15.
Yennu, A., Tian, F., Smith-Osborne, A., Gatchel, R. J., Woon, F. L., and Liu, H. (2016). Prefrontal responses to Stroop tasks in subjects with post-traumatic stress disorder assessed by functional near infrared spectroscopy. Sci. Rep. 6:30157. doi: 10.1038/srep30157
Yokoyama, C., Kaiya, H., Kumano, H., Kinou, M., Umekage, T., Yasuda, S., et al. (2015). Dysfunction of ventrolateral prefrontal cortex underlying social anxiety disorder: a multi-channel NIRS study. Neuroimage Clin. 8, 455–461.
Zarse, E. M., Neff, M. R., Yoder, R., Hulvershorn, L., Chambers, J. E., and Chambers, R. A. (2019). The adverse childhood experiences questionnaire: two decades of research on childhood trauma as a primary cause of adult mental illness, addiction, and medical diseases. Cogent Med. 6:1581447.
Zhang, S. X., Liu, J., Jahanshahi, A. A., Nawaser, K., Yousefi, A., Li, J., et al. (2020). At the height of the storm: healthcare staff’s health conditions and job satisfaction and their associated predictors during the epidemic peak of COVID-19. Brain Behav. Immun. 87, 144–146.
Zhang, Y., Zhang, H., Ma, X., and Di, Q. (2020). Mental health problems during the COVID-19 pandemics and the mitigation effects of exercise: a longitudinal study of college students in China. Int. J. Environ. Res. Public Health 17:3722. doi: 10.3390/ijerph17103722
Zhu, Y., Jayagopal, J. K., Mehta, R. K., Erraguntla, M., Nuamah, J., McDonald, A. D., et al. (2020). Classifying major depressive disorder using fNIRS during motor rehabilitation. IEEE Trans. Neural Syst. Rehabil. Eng. 28, 961–969. doi: 10.1109/TNSRE.2020.2972270
Ziobrowski, H. N., Kennedy, C. J., Ustun, B., House, S. L., Beaudoin, F. L., An, X., et al. (2021). Development and validation of a model to predict posttraumatic stress disorder and major depression after a motor vehicle collision. JAMA Psychiatry 78, 1228–1237. doi: 10.1001/jamapsychiatry.2021.2427
Keywords: artificial intelligence, mental health disorders, prediction and prevention, ex-COVID-19 patients, semantic/acoustic features, neurophysiological features, facial/oculometric features
Citation: Ćosić K, Popović S, Šarlija M, Kesedžić I, Gambiraža M, Dropuljić B, Mijić I, Henigsberg N and Jovanovic T (2021) AI-Based Prediction and Prevention of Psychological and Behavioral Changes in Ex-COVID-19 Patients. Front. Psychol. 12:782866. doi: 10.3389/fpsyg.2021.782866
Received: 24 September 2021; Accepted: 02 December 2021;
Published: 28 December 2021.
Edited by:
Jinhui Li, Jinan University, ChinaReviewed by:
K. C. Santosh, University of South Dakota, United StatesCopyright © 2021 Ćosić, Popović, Šarlija, Kesedžić, Gambiraža, Dropuljić, Mijić, Henigsberg and Jovanovic. This is an open-access article distributed under the terms of the Creative Commons Attribution License (CC BY). The use, distribution or reproduction in other forums is permitted, provided the original author(s) and the copyright owner(s) are credited and that the original publication in this journal is cited, in accordance with accepted academic practice. No use, distribution or reproduction is permitted which does not comply with these terms.
*Correspondence: Siniša Popović, c2luaXNhLnBvcG92aWNAZmVyLmhy
Disclaimer: All claims expressed in this article are solely those of the authors and do not necessarily represent those of their affiliated organizations, or those of the publisher, the editors and the reviewers. Any product that may be evaluated in this article or claim that may be made by its manufacturer is not guaranteed or endorsed by the publisher.
Research integrity at Frontiers
Learn more about the work of our research integrity team to safeguard the quality of each article we publish.