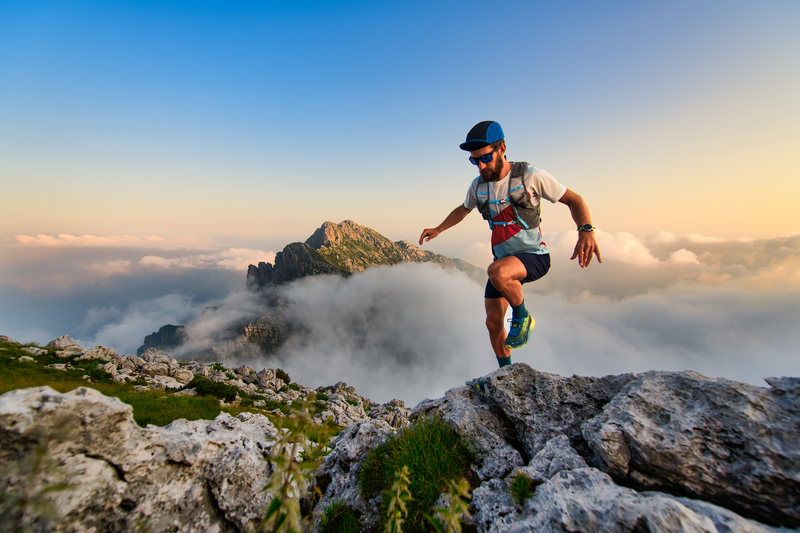
94% of researchers rate our articles as excellent or good
Learn more about the work of our research integrity team to safeguard the quality of each article we publish.
Find out more
BRIEF RESEARCH REPORT article
Front. Psychol. , 25 November 2021
Sec. Quantitative Psychology and Measurement
Volume 12 - 2021 | https://doi.org/10.3389/fpsyg.2021.767244
Differential Item Functioning (DIF) analysis is always an indispensable methodology for detecting item and test bias in the arena of language testing. This study investigated grade-related DIF in the General English Proficiency Test-Kids (GEPT-Kids) listening section. Quantitative data were test scores collected from 791 test takers (Grade 5 = 398; Grade 6 = 393) from eight Chinese-speaking cities, and qualitative data were expert judgments collected from two primary school English teachers in Guangdong province. Two R packages “difR” and “difNLR” were used to perform five types of DIF analysis (two-parameter item response theory [2PL IRT] based Lord’s chi-square and Raju’s area tests, Mantel-Haenszel [MH], logistic regression [LR], and nonlinear regression [NLR] DIF methods) on the test scores, which altogether identified 16 DIF items. ShinyItemAnalysis package was employed to draw item characteristic curves (ICCs) for the 16 items in RStudio, which presented four different types of DIF effect. Besides, two experts identified reasons or sources for the DIF effect of four items. The study, therefore, may shed some light on the sustainable development of test fairness in the field of language testing: methodologically, a mixed-methods sequential explanatory design was adopted to guide further test fairness research using flexible methods to achieve research purposes; practically, the result indicates that DIF analysis does not necessarily imply bias. Instead, it only serves as an alarm that calls test developers’ attention to further examine the appropriateness of test items.
It is self-evident that language tests should be fair to all the test takers, rather than favoring or disfavoring any test taker groups because of construct-irrelevant issues such as gender, age, and native languages. This, however, cannot always be guaranteed no matter how carefully tests are designed. Conscientious test developers are expected to provide research evidence for the quality of their tests including the absence of bias (Kunnan, 2017). A well-known method to address this problem is the Differential Item Functioning (DIF) analysis, which examines whether test items function differentially toward two testing groups after controlling for the ability level of the groups (Holland and Wainer, 1993). Numerous scholars and test standards have advocated this method for the purpose of detecting construct-irrelevant and biased test items and improving test validity and fairness (e.g., Camilli and Shepard, 1994; Kunnan, 1997, 2000, 2004, 2017; Xi, 2010; Martinková and Drabinová, 2018).
Empirical studies have conducted DIF analysis to detect problematic test items, providing evidence for test quality and fairness. Existing studies, however, have mainly focused on the DIF effect toward testing groups classified by native languages (Abbott, 2007), gender (Aryadoust et al., 2011; Grover and Ercikan, 2017), and age (Geranpayeh and Kunnan, 2007; Aryadoust, 2012; Banerjee and Papageorgiou, 2016; Oliver et al., 2018). It is commonly known that learners of the same age may be placed into different grades, meaning that their years of English learning are different. But the grade-related DIF, emphasizing years of receiving English education, in tests for young children has been under-researched. The grade DIF usually occurs due to the discrepancy of grade levels. In other words, since higher grade students tend to be more cognitively developed due to their extra years of receiving English education, it is speculated that they are likely to be favored in a test even when the overall ability of the higher and the lower grade students is controlled for (i.e., they are more likely to get the correct answer even if they have the same overall ability as the lower grade students). Therefore, the indispensability of grade cannot be neglected in that it might influence test takers’ test performance, and further challenge the fairness and validity of the assessment.
While DIF items can be easily detected due to the development of statistical methods and software, relevant studies have rarely provided sound explanations for the existence of DIF. Geranpayeh and Kunnan (2007) reported that existing studies mainly focus on detecting DIF items, rather than identifying DIF sources. Similarly, Zumbo (2007) also pointed out this problem and called for exploring the reasons for DIF. In fact, some studies have made efforts to answer this why question (e.g., Li et al., 2004; Yao and Chen, 2020). However, only a weak relationship was found between gender DIF and task types, but no convincing explanations could be provided (see detailed discussion in the next section).
Considering the above-mentioned reasons, this study aims to detect grade DIF in a test for children, the GEPT-Kids (listening section1), and to find out potential reasons for such DIF. The GEPT-Kids claims that it is designed for primary school students but does not specify for which grade (Language Training and Testing Center, LTTC, 2015; Kunnan and Liao, 2019). Hence, it ought to be fair to all the pupils, rather than functioning differentially toward different grades. However, this has not been supported by empirical research evidence, which will be addressed in the current study.
On account of the development of methods, empirical DIF studies have a relatively long history. Geranpayeh and Kunnan (2007) listed relevant studies in language testing from 1980 to 2005. Later, Kunnan (2017) continued to summarize DIF studies conducted from 1980 to 2017. Table 1 updates Kunnan’s (ibid) list by including more studies in the arena of language assessment during the same period. As the table shows, most of the existing DIF studies have placed the foci on L1 language, gender, age, and academic major. None of them have examined grade DIF, which, as argued in the introduction, is an influential factor for children due to years of English learning. In addition, tests for young children tend to be ignored in studies of this type. In terms of analytical methods, few of the existing studies used multiple methods. Therefore, their results may not be as precise or comprehensive as expected.
Another deficiency with existing studies is that they can hardly give convincing explanations for the DIF effect that they found. At the early stage, Angoff (1993) concluded that DIF results are often confounding because it cannot be explained the reasons that some perfectly reasonable items are flagged. This question is still not well solved today, even though researchers have tried to explain DIF items. There are mainly two approaches to investigating DIF reasons: exploratory and confirmatory approaches. The exploratory approach detects DIF items first with statistical methods and then asks content experts to look for possible reasons for the DIF effect. Geranpayeh and Kunnan (2007) adopted this approach and proposed some reasons for DIF (e.g., the multidimensionality of test items). However, the reliability of explanations based on content experts’ experience and speculation sometimes is in doubt. The confirmatory approach is theory-based and hypothesis-driven. It first proposes hypotheses based on relevant literature, then detects DIF items, and finally checks the correctness of the hypotheses. For example, Li et al. (2004) attempted to find out the relationship between test items characteristics and gender DIF by coding test items based on Ibarra’s (2001) multi-context theory and Gallagher’s (1998) cognitive structure analysis approach. Correlation analyses between coded DIF index and detected DIF index suggested that multi-context theory-based approach could predict gender DIF more effectively (cf. Li et al., 2004). While this study shows that test items with certain characteristics tend to favor a particular group of test takers, and it inherently does not explain why such a relationship exists or why some test items with the same characteristics do not favor any group. Potential DIF sources are fruitful and reasons for DIF may vary across items. The confirmatory approach, however, tends to confine its focus to a particular reason and overgeneralizes this reason to all the items.
By contrast, the exploratory approach is open to any reasonable explanations and treats each question as a unique item with its own DIF sources. Therefore, the exploratory approach is considered to be more appropriate in exploring DIF sources in the current study. Since expert judgment alone does not give reliable reasons, Ercikan et al. (2010) used verbal report protocols of test takers to confirm DIF sources identified by experts. Such triangulation is supposed to improve the reliability of the study.
In view of the research gaps identified above and the merits and demerits of different research methods, the current study aims to examine grade-related DIF in the GEPT-Kids (listening section) through different analytical methods and explore the potential DIF sources through expert judgment and post-test interviews. To achieve these purposes, the following research questions are raised to guide the research.
(1) Are there any items in GEPT-Kids Listening exhibiting DIF toward different grade groups (Grade 5 and Grade 6)?
(2) What are the possible reasons for the detected grade DIF?
To address the research questions, a mixed-methods sequential explanatory design was adopted in this study with two phases (Creswell et al., 2003; Ivankova et al., 2006). A quantitative DIF analysis was conducted to identify potential grade DIF items in GEPT-Kids listening (phase I). Then, qualitative expert judgment was conducted to find out the potential reasons for the detected grade DIF (phase II).
Quantitative data used in this study were 791 pupils’ test scores in a GEPT-Kids listening test. The 791 participants (Grade 5 = 398; Grade 6 = 393) were English as a Foreign Language (EFL) learners from eight Chinese-speaking cities (i.e., Beijing, Shanghai, Guangzhou, Hong Kong, Macau, Taipei, Taichung, and Kaohsiung). The number of male (N = 399) and female (N = 392) students is similar. All the participants started learning English in Grade 1, and their first language is Chinese and second language is English. The GEPT-Kids listening test paper included four parts and 25 multiple-choice questions in total, with each item carrying one point. The test aims to test pupils’ understanding of common words, phrases, and simple sentences used in familiar topics that pupils may encounter in their daily life and school context (LTTC, 2015). The data were collected in a GEPT-Kids related project funded by LTTC2 in 2016–2017. The research assistants in this project went to the above-mentioned cities and administered the test in a Grade 5 and a Grade 6 class in each city. Then, the test papers were scored, and the results were input. The current study has gained permission from the LTTC to use relevant data.
Qualitative data were gained from expert judgment by two primary school English teachers in China. They both passed the Test for English Majors-Band 8 (TEM-8) and have been teaching English in the primary school in Guangdong province for more than ten years. Additionally, they are experienced in developing and designing tests for pupils. Firstly, the two teachers were asked to read the whole test paper carefully and judge whether certain items may favor Grade 5 and Grade 6 students and why. Then, they were informed which items were flagged as DIF items and tried to find out potential reasons. The two teachers sent their thoughts and opinions to the researchers during their analysis.
The quantitative data (i.e., test result) were analyzed by the Statistical Package for the Social Sciences (SPSS) v.24.0 and the RStudio. Firstly, SPSS v.24.0 was used to conduct a descriptive analysis of the test result. Mean and point-biserial correlation coefficients (item discrimination) were calculated to present an overview of the test result. Then, RStudio v.3.6.1 (R Studio Team, 2019) was used to conduct DIF analyses. Even though many DIF methods are available, different methods show different results and consensus has not been achieved on which method is the best. A feasible solution is to use different methods and examine all the results, in which way we may get closer to the truth that we are looking for Zumbo (2007); Zumbo et al. (2015). Also, even though various software has been developed for those DIF methods, such as the simultaneous item bias test (SIBTEST, Shealy and Stout, 1993), Differential Item Functioning Analysis System (DIFAS, Penfield, 2005, 2013), BILOG-MG (Zimowski, 1998), Item Response Theory for Patient-reported Outcomes (IRTPRO, Cai et al., 2011), not all of them are free, and more essentially, able to apply different methods [see Liu et al. (2019) published on Frontiers in Psychology for specific DIF methods discussion]. In terms of accessibility and flexibility, RStudio is an ideal tool, which is open to the public and allows the installation of various R packages to perform different kinds of analysis. Therefore, RStudio was chosen to conduct multiple DIF analyses. In RStudio, two R packages “difR” (Magis et al., 2010) and “difNLR” (Drabinová and Martinková, 2017; Hladka and Martinkova, 2020) were used to perform five types of DIF analysis (two-parameter [2PL] IRT based Lord’s chi-square and Raju’s area tests, MH, LR, and NLR DIF methods) on the test result. As different methods may flag different DIF items, the above-mentioned methods were taken to make the analysis as exhaustive as possible. Besides, ShinyItemAnalysis package (Martinková and Drabinová, 2018) was used to display item characteristic curves (ICCs) in RStudio to give a direct visual presentation about which test group the DIF items favor. Default settings were used for all the DIF analyses, interested readers may consult the manuals of related R packages online.
In the DIF analyses, it was hypothesized that Grade 6 students might be favored while Grade 5 students might be disfavored. The rationale is that for young children, grades are very likely to influence their test performance because different grade students vary in learning curriculums, psychological status, and other aspects. Even when the overall levels of different grade students are controlled for, Grade 6 students still tend to be favored (i.e., more likely to get the correct answer) because they are more cognitively developed, having larger working memory capacity and better comprehension of language, gaining more exposure to English and tests, and having been learning English for one more year. Since all of these developmental features seem to advantage higher grade students, in this study, Grade 6 was set as the reference group and Grade 5 as the focal group (the group at the risk of being disfavored, see Jiao and Chen, 2014).
The qualitative data were the two teachers’ self-reported thoughts during the analysis of the test paper. Their thoughts were summarized and reported below.
Table 2 presents the descriptive statistics of the test performance data, which show the item difficulty and item discrimination. The mean of test items ranges from 0.52 to 0.98 for Grade 5 and from 0.65 to 0.98 for Grade 6. The highest mean for both Grade 5 and Grade 6 students lies in L9 and L12, and the lowest mean lies in L21. This result implies that students from both performed well on items L9 and L12, but poorly on item L21. The mean of most items is above 0.80, indicating that the test might be too easy for the test takers. In terms of item discrimination, most items have a discrimination level above 0.30 (McCowan and McCowan, 1999), indicating that they can discriminate test takers to some extent. To be more specific, high scorers are more likely to answer the items correctly, while low scorers are more likely to answer the items incorrectly. However, five items (L1, L3, L4, L5, and L9) may not be able to discriminate test takers at different levels because their corrected item-total correlation is too low (<0.30, McCowan and McCowan, 1999).
Table 3 summarizes DIF items flagged by different methods. From the table, the nonlinear regression test is the most sensitive method, detecting the most DIF items (14 items, over half of the total 25 items). On the other hand, 2PL IRT based Lord’s chi-square test and MH test are the least sensitive methods, detecting the fewest DIF items (four items). Five methods altogether flagged 16 items (i.e., L2, L3, L4, L6, L7, L9, L13, L15, L16, L17, L19, L20, L21, L22, L23, and L25) with potential DIF effect, which represent 64% of the total items. Among which, item L15 was flagged as DIF for the most times (four times). All these results were significant at 0.05 level (p < 0.05) and the effect sizes for the flagged items were moderate or large.
The ICCs of the flagged 16 items show details about their DIF effect, which is displayed in Table 4. According to the table, four items have little DIF effect. The little effect represents that the test items are detected as DIF items, but the effect is minimal or even negligible. Also, six items favor Grade 6 students; four items favor Grade 5 students; and two items have non-uniform DIF effect (i.e., favoring one group at lower ability level and favoring the other group at higher ability level). Due to the space limit, not all the ICCs can be presented here; only an example of each DIF type is presented below.
The ICCs show that the DIF effect of L6, L7, L16, and L17 is minimal. Taking L16 as an instance (see Figure 1), the two lines of the reference group and the focal group are almost identical, indicating that test takers at different levels have almost the same probability to get the correct answer. A striking feature is that most of the items, as predicted, favor Grade 6 students. As L3 in Figure 2 shows, at the lower ability level, the reference group (Grade 6) has a higher probability to get the correct answer. Surprisingly, there are four items favoring grade 5 students. For example, Figure 3 shows that at the lower ability level, the focal group (Grade 5) students are more likely to get the correct answer. Besides, L15 and L21 present a complex non-uniform DIF effect. As Figure 4 shows, at lower ability level, the focal group is more likely to get the correct answer, while at higher ability level, the reference group has a higher probability to get the correct answer.
Expert judges were used to corroborate the findings from the DIF study. Without being told which items were identified in the DIF analysis, the two teachers who took part in the study could not identify any problems related to the grade factor with the test items. They did not think that any items would favor students in Grade 5 or Grade 6. After being told which items were flagged, they tried to come up with reasonable explanations for particular students being favored on certain items. They suggested that four items that may cause DIF: L20, L21, L22, and L25. For example, L21 asks what is Nina going to do this evening? with three options: A. See Dr. Li; B. Watch a ball game; C. Stay at home. The possible reason that they could think of is that be going to structure is not taught until the second term of Grade 5. Grade 5 students might have not learned this structure yet at the time of the data collection. Therefore, Grade 6 students might be favored on these four items with the be going to structure. Nevertheless, no plausible explanations could be given for other flagged items. For example, L2 requires test takers to look at a picture (a pair of socks) and judge whether what they hear (This is a pair of socks.) is the same as the picture. In the teachers’ opinion, test items like this one was considered fair to Grade 5 or Grade 6 students, because the topic and language were familiar to students in both grades, although these items were flagged as DIF items in the DIF analyses.
This study used a sequential explanatory mixed-methods design to detect DIF items in GEPT-Kids Listening. Different methods identified different potential DIF items, which may undermine the reliability of the analysis. Some researchers (e.g., Ferne and Rupp, 2007) recommend using multiple methods to validate DIF results. This study found that cross-validation may not be achieved through this way. Even though the five methods detected many potential DIF items, there were no items flagged by all of the five methods. The true value of using multiple DIF methods might be that they can detect potential DIF items to a larger extent and remind test developers to further examine those items. In addition, a potential limitation of research methods was that this study did not use correction for multiple comparisons in methods where DIF is tested item by item. Future DIF studies may try to use correction for multiple comparisons when they adopt multiple methods to conduct DIF analyses.
Similar to many of the previous studies (e.g., Uiterwijk and Vallen, 2005; Geranpayeh and Kunnan, 2007; Song et al., 2015), this study did not find convincing reasons for the DIF effect or any evidence of bias with the DIF items. It was suspected that L20, L21, L22, and L25 may favor Grade 6 because they contain be going to structure which is not taught until in the second term of Grade 5. This speculation makes sense to some extent. However, it does not explain why L21 exhibits non-uniform DIF, rather than favoring Grade 6 only. In addition, the two teachers’ speculation was solely based on their teaching experience in Guangdong province, so it may not be appropriate for this explanation to be generalized to other areas. Besides, there is no golden standard, i.e., criteria or rubrics, for teachers to appraise the suitability of test items in terms of grade. Moreover, even if the be going to structure is the reason for certain items favoring Grade 6, it does not mean that those items are biased, because language knowledge is a construct-relevant issue. The Grade 5 students have not learned this structure is not the problem with the test items themselves. In GEPT-Kids Listening, no evidence of bias could be found with the DIF items, which suggests that those items are not problematic. It is to be hoped that further research using expert judgment could consider containing more experts to share their opinions and feedback.
Even though this study detected many DIF items through statistical analyses, it should be noted that being flagged as DIF items does not necessarily mean that those items are biased (Angoff, 1993). Since DIF sources cannot be identified in this and many other studies, it might be reasonable to arrive at the conclusion that the result of DIF analysis only serves as an alarm that calls test developers’ attention to further examine the appropriateness of test items. When further examination cannot find evidence of bias with the test items, a verdict might be made that the test is free of bias. On the other hand, given the current study only lays emphasis on the grade factor, other variables such as gender and region are advocated to be included in further DIF research to present more comprehensive and representative research outcomes.
To sum up, the current study may both contribute to the test fairness to achieve its sustainable development: methodologically, robust mixed-methods research was adopted to guide further research using more flexible methods; practically, the study suggests test developers pay more attention to the test bias so that fairer test items could be developed, which may enhance the validity and reliability of the test and the bring about beneficial consequences to the educational system and practices or even the society.
The datasets presented in this article are not readily available because, due to the policy of LTTC, the data is not public. Requests to access the datasets should be directed to Rachel Wu, cnd1QGx0dGMubnR1LmVkdS50dw==.
The studies involving human participants were reviewed and approved by the Language Training and Testing Center (LTTC). Written informed consent to participate in this study was provided by the participants’ legal guardian/next of kin.
LL was responsible for the manuscript draft. DY helped with data analyses and corresponding issues. Both authors contributed to the article and approved the submitted version.
The authors declare that the research was conducted in the absence of any commercial or financial relationships that could be construed as a potential conflict of interest.
All claims expressed in this article are solely those of the authors and do not necessarily represent those of their affiliated organizations, or those of the publisher, the editors and the reviewers. Any product that may be evaluated in this article, or claim that may be made by its manufacturer, is not guaranteed or endorsed by the publisher.
We want to express our great gratitude to Prof. Antony John Kunnan for his guidance. Besides, our special thanks go to Dr. Jessica Wu and Dr. Rachel Wu from LTTC for granting the data. Without their help, we could not have had this manuscript published.
Abbott, M. L. (2007). A confirmatory approach to differential item functioning on an ESL reading assessment. Language Testing 24, 7–36. doi: 10.1177/0265532207071510
Alderman, D. L., and Holland, P. W. (1981). Item Performance Across Native Language Groups on the TOEFL (TOEFL Research Report No.9). Princeton, NJ: Educational Testing Service.
Alderson, J. C., and Urquhart, A. H. (1985). The effect of students’ academic discipline on their performance on ESP reading tests. Language Testing 2, 192–204. doi: 10.1177/026553228500200207
Allalouf, A., and Abramzon, A. (2008). Constructing better second language assessments based on differential item functioning analysis. Language Assess. Quart. 5, 120–141. doi: 10.1080/15434300801934710
Angoff, W. (1993). “Perspective on differential item functioning methodology,” in Differential Item Functioning, eds P. W. Holland and H. Wainer (Lawrence Erlbaum Associates), 3–24.
Aryadoust, V. (2012). Differential item functioning in while-listening performance tests: the case of the international english language testing system (IELTS) listening module. Int. J. Listen. 26, 40–60. doi: 10.1080/10904018.2012.639649
Aryadoust, V., Goh, C. C., and Kim, L. O. (2011). An investigation of differential item functioning in the MELAB listening test. Language Assess. Quarte. 8, 361–385. doi: 10.1080/15434303.2011.628632
Banerjee, J., and Papageorgiou, S. (2016). What’s in a topic? Exploring the interaction between test-taker age and item content in high-stakes testing. Int. J. Listen. 30, 8–24. doi: 10.1080/10904018.2015.1056876
Brown, J. D. (1993). What are the characteristics of natural cloze tests? Language Testing 10, 93–116. doi: 10.1177/026553229301000201
Brown, J. D. (1999). The relative importance of persons, items, subtests and languages to TOEFL test variance. Language Testing 16, 217–238. doi: 10.1177/026553229901600205
Cai, L., Du Toit, S. H. C., and Thissen, D. (2011). IRTPRO: Flexible, Multidimensional, Multiple Categorical IRT Modeling [Computer Software]. Chicago: Scientific Software International.
Camilli, G., and Shepard, L. (1994). Methods for Identifying Biased Test Items. Thousand Oaks, CA: Sage Publications.
Chen, Z., and Henning, G. (1985). Linguistic and cultural bias in language proficiency tests. Language Testing 2, 155–163. doi: 10.1177/026553228500200204
Creswell, J. W., Tashakkori, A., Jensen, K. D., and Shapley, K. L. (2003). “Teaching mixed methods research: practices, dilemmas, and challenges,” in Handbook of Mixed Methods in Social and Behavioral Research, eds A. Tashakkori and C. Teddlie (Sage Publications), 91–110.
Drabinová, A., and Martinková, P. (2017). Detection of differential item functioning with non linear regression: non-IRT approach accounting for guessing. J. Educ. Measure. 54, 498–517. https://ur.booksc.eu/book/67571848/ea23fb
Ercikan, K., Arim, R., Law, D., Domene, J., Gagnon, F., and Lacroix, S. (2010). Application of think aloud protocols for examining and confirming sources of differential item functioning identified by expert reviews. Educ. Measure. Issues Practice 29, 24–35. doi: 10.1111/j.1745-3992.2010.00173.x
Ferne, T., and Rupp, A. A. (2007). A synthesis of 15 years of research on DIF in language testing: methodological advances, challenges, and recommendations. Language Assess. Quart. 4, 113–148. doi: 10.1080/15434300701375923
Gallagher, A. M. (1998). Gender and antecedents of performance in mathematics testing. Teachers College Record 100, 297–314.
Geranpayeh, A., and Kunnan, A. J. (2007). Differential item functioning in terms of age in the certificate in advanced english examination*. Language Assess. Quart. 4, 190–222. doi: 10.1080/15434300701375758
Ginther, A., and Stevens, J. (1998). “Language background, ethnicity, and the internal construct validity of the advanced placement Spanish language examination,” in Validation in Language Assessment, ed. A. J. Kunnan (Lawrence Erlbaum), 169–194.
Grover, R. K., and Ercikan, K. (2017). For which boys and which girls are reading assessment items biased against? Detection of differential item functioning in heterogeneous gender populations. Appl. Measure. Educ. 30, 178–195. doi: 10.1080/08957347.2017.1316276
Hale, G. A. (1988). Student major field and text content: interactive effects on reading comprehension in the test of english as a foreign language. Language Testing 5, 49–61. doi: 10.1177/026553228800500104
Harding, L. (2012). Accent, listening assessment and the potential for a shared-L1advantage: A DIF perspective. Language Testing 29, 163–180. doi: 10.1177/0265532211421161
Hladka, A., and Martinkova, P. (2020). DifNLR: generalized logistic regression models for DIF and DDF detection. Journal 12, 300–323.
Holland, P. W., and Wainer, H. (1993). Differential Item Functioning. Mahwah: Lawrence Erlbaum Associates, Inc.
Ivankova, N. V., Creswell, J. W., and Stick, S. (2006). Using mixed-methods sequential explanatory design: from theory to practice. Field Methods 18, 3–20. doi: 10.1177/1525822X05282260
Jiao, H., and Chen, Y. F. (2014). “Differential item and testlet functioning analysis,” in The Companion to Language Assessment, ed. A. J. Kunnan (Wiley), 1282–1300.
Kim, M. (2001). Detecting DIF across the different language groups in a speaking test. Language Testing 18, 89–114. doi: 10.1177/026553220101800104
Kim, Y. H., and Jang, E. E. (2009). Differential functioning of reading subskills on the OSSLT for L1 and ELL students: a multidimensionality model-based DBF/DIF approach. Language Learning 59, 825–865.
Kunnan, A. J. (1990). DIF in native language and gender groups in an ESL placement test. Tesol. Quart. 24, 741–746.
Kunnan, A. J. (1992). An investigation of a criterion-referenced test using G-theory, and factor and cluster analyses. Language Testing 9, 30–49. doi: 10.1177/026553229200900104
Kunnan, A. J. (1995). Test Taker Characteristics and Test Performance: A Structural Modeling Approach. Cambridge: Cambridge University Press.
Kunnan, A. J. (1997). “Connecting validation and fairness in language testing,” in Current Developments and Alternatives in Language Assessment, eds A. Huhta, V. Kohonen, L. Kurki-Suomo, and S. Luona (University of Jyväylä), 85–105.
Kunnan, A. J. (2000). “Fairness and justice for all,” in Fairness and Validation in Language Assessment, ed. A. J. Kunnan (Cambridge University Press), 1–13.
Kunnan, A. J. (2004). “Test fairness,” in European Year of Languages Conference Papers, Barcelona, Spain, eds M. Milanovic and C. Weir (Cambridge University Press), 27–48.
Kunnan, A. J., and Liao, L. (2019). Modeling the relationships among young learners’ self-assessment, learning attitude, and language test performance. J. Asia TEFL 16, 701–710.
Li, Y., Cohen, A. S., and Ibarra, R. A. (2004). Characteristics of mathematics items associated with gender DIF. Int. J. Testing 4, 115–136. doi: 10.1207/s15327574ijt0402_2
Liu, Y., Yin, H., Xin, T., Shao, L., and Yuan, L. (2019). A comparison of differential item functioning detection methods in cognitive diagnostic models. Front. Psychol. 10:1–9.
Lowenberg, P. (2000). “Non-native varieties and issues of fairness in testing english as a world language,” in Fairness and Validation in Language Assessment: Selected Papers From the 19th Language Testing Research Colloquium, Orlando, Florida, ed. A. J. Kunnan (Cambridge University Press), 43–60.
LTTC (2015). The Test Design of GEPT Kids. Available online at: https://www.geptkids.org.tw/ENHome/design#testContent (assessed October 1, 2021).
Magis, D., Béland, S., Tuerlinckx, F., and De Boeck, P. (2010). A general framework and an R package for the detection of dichotomous differential item functioning. Behav. Res. Methods 42, 847–862. doi: 10.3758/BRM.42.3.847
Martinková, P., and Drabinová, A. (2018). Shiny item analysis for teaching psychometrics and to enforce routine analysis of educational tests. R J. 10, 503–515.
McCowan, R. J., and McCowan, S. C. (1999). Item Analysis for Criterion-Referenced Tests. New York: Center for Development of Human Services.
Norton, B., and Stein, P. (1998). “Why the “monkeys passage” bombed: tests, genres, and teaching,” in Validation in Language Assessment, ed. A. J. Kunnan (Cambridge University Press), 231–249.
Ockey, G. J. (2007). Investigating the validity of math word problems for english language learners with DIF. Language Assess. Quart. 4, 149–164.
Oliver, M., Lawless, R., Robin, F., and Bridgeman, B. (2018). An exploratory analysis of differential item functioning and its possible sources in a higher education admissions context. Appl. Measure. Educ. 31, 1–16. doi: 10.1080/08957347.2017.1391258
Oltman, P. K., Stricker, L. J., and Barrows, T. S. (1988). Native language, english proficiency, and the structure of the test of english as a foreign language, ETS. Res. Rep. Ser. 1, 1–36. doi: 10.1002/j.2330-8516.1988.tb00282.x
Pae, T. I. (2004). DIF for examinees with different academic backgrounds. Language Testing 21, 53–73. doi: 10.1191/0265532204lt274oa
Pae, T.-I., and Park, G.-P. (2006). Examining the relationship between differential item functioning and differential test functioning. Language Testing 23, 475–496. doi: 10.1191/0265532206lt338oa
Penfield, R. (2005). DIFAS: differential item functioning analysis system. Appl. Psychol. Measure. 29, 150–151. doi: 10.1177/0146621603260686
Penfield, R. D. (2013). DIFAS 4.0: User’s Manual. Manuscript in Preparation. Available online at: https://soe.uncg.edu/wp-content/uploads/2015/12/DIFASManual_V5.pdf (assessed October 1, 2021).
Roever, C. (2007). DIF in the assessment of second language pragmatics. Language Assess. Quart. 4, 165–189.
R Studio Team (2019). RStudio: Integrated Development for R. Boston, MA. Available online at: http://www.rstudio.com/ (accessed October 18, 2021).
Ryan, K. E., and Bachman, L. F. (1992). Differential item functioning on two tests of EFL proficiency. Language Testing 9, 11–29. doi: 10.1177/026553229200900103
Sasaki, M. (1991). A comparison of two methods for detecting differential I item functioning in an ESL placement test. Language Testing 8, 95–111. doi: 10.1177/026553229100800201
Schohamy, E., and Inbar, O. (1991). Validation of listening comprehension tests: the effect of text and question type. Language Testing 8, 23–40. doi: 10.1177/026553229100800103
Shealy, R., and Stout, W. (1993). A model-based standardization approach that separates true bias/DIF from group ability differences and detects test bias/DTF as well as item bias/DIF. Psychometrika 58, 159–194. doi: 10.1007/BF02294572
Shohamy, E. (1984). Does the testing method make a difference? the case of reading comprehension. Language Testing 1, 147–170. doi: 10.1177/026553228400100203
Song, X., Cheng, L., and Klinger, D. (2015). DIF investigations across groups of gender and academic background in a large-scale high-stakes language test. Papers Language Testing Assess. 4, 97–124.
Swinton, S. S., and Powers, D. E. (1980). Factor analysis of the test of english as a foreign language for several language groups. ETS Res. Rep. Ser. 2, 1–79. doi: 10.1002/j.2333-8504.1980.tb01229.x
Takala, S., and Kaftandjieva, F. (2000). Test fairness: a DIF analysis of an L2 vocabulary test. Language Testing 17, 323–340. doi: 10.1177/026553220001700303
Uiterwijk, H., and Vallen, T. (2005). Linguistic sources of item bias for second generation immigrants in dutch tests. Language Testing 22, 211–234. doi: 10.1191/0265532205lt301oa
Yao, D., and Chen, K. (2020). Gender-related differential item functioning analysis on an ESL test. J. Language Testing Assess. 3, 5–19. doi: 10.23977/langta.2020.030102
Zeidner, M. (1986). Are scholastic aptitude tests in Israel BIASED towards Arab college student candidates? Higher Educ. 15, 507–522.
Zeidner, M. (1987). Essay versus multiple-choice type classroom exams: the student’s perspective. J. Educ. Res. 80, 352–358. doi: 10.1080/00220671.1987.10885782
Zimowski, M. F. (1998). BILOG-MG: Multiple-Group IRT Analysis and Test Maintenance for Binary Items. Chicago: Scientific Software International.
Zumbo, B. D. (2007). Three generations of DIF analyses: considering where it has been, where it is now, and where it is going. Language Assess. Quart. 4, 223–223. doi: 10.1080/15434300701375832
Keywords: grade, DIF, GEPT-Kids, listening, mixed-methods approach
Citation: Liao L and Yao D (2021) Grade-Related Differential Item Functioning in General English Proficiency Test-Kids Listening. Front. Psychol. 12:767244. doi: 10.3389/fpsyg.2021.767244
Received: 30 August 2021; Accepted: 18 October 2021;
Published: 25 November 2021.
Edited by:
Alexander Robitzsch, IPN – Leibniz Institute for Science and Mathematics Education, GermanyReviewed by:
Patrícia Martinková, Institute of Computer Science (ASCR), CzechiaCopyright © 2021 Liao and Yao. This is an open-access article distributed under the terms of the Creative Commons Attribution License (CC BY). The use, distribution or reproduction in other forums is permitted, provided the original author(s) and the copyright owner(s) are credited and that the original publication in this journal is cited, in accordance with accepted academic practice. No use, distribution or reproduction is permitted which does not comply with these terms.
*Correspondence: Don Yao, eWI4NzcxMEB1bS5lZHUubW8=
†These authors have contributed equally to this work
Disclaimer: All claims expressed in this article are solely those of the authors and do not necessarily represent those of their affiliated organizations, or those of the publisher, the editors and the reviewers. Any product that may be evaluated in this article or claim that may be made by its manufacturer is not guaranteed or endorsed by the publisher.
Research integrity at Frontiers
Learn more about the work of our research integrity team to safeguard the quality of each article we publish.