- 1Department of Psychiatry, Bellvitge University Hospital-IDIBELL, Barcelona, Spain
- 2Ciber Fisiopatología Obesidad y Nutrición (CIBERObn), Instituto de Salud Carlos III, Madrid, Spain
- 3Department of Psychobiology and Methodology, Autonomous University of Barcelona, Barcelona, Spain
- 4Department of Clinical Sciences, School of Medicine, University of Barcelona, Barcelona, Spain
- 5Psychiatry and Mental Health Group, Neurosciences Programme, Instituto de Investigación Biomédica de Bellvitge (IDIBELL), Barcelona, Spain
- 6Department of Psychiatry and Child Study Center, Yale University School of Medicine, New Haven, CT, United States
- 7Connecticut Council on Problem Gambling, Wethersfield, CT, United States
- 8Connecticut Mental Health Center, New Haven, CT, United States
- 9Department of Neuroscience, Yale University, New Haven, CT, United States
- 10Department of Clinical Sciences Lund, Psychiatry, Faculty of Medicine, Lund University, Lund, Sweden
- 11Region Skåne, Gambling Disorder Unit, Malmö, Sweden
- 12Department of Public Health, Mental Health and Perinatal Nursing, School of Nursing, University of Barcelona, Barcelona, Spain
- 13Ciber Salut Mental (CIBERSAM), Instituto de Salud Carlos III, Madrid, Spain
Background and Aims: COVID-19 pandemic and confinement have represented a challenge for patients with gambling disorder (GD). Regarding treatment outcome, dropout may have been influenced by these adverse circumstances. The aims of this study were: (a) to analyze treatment dropout rates in patients with GD throughout two periods: during and after the lockdown and (b) to assess clinical features that could represent vulnerability factors for treatment dropout.
Methods: The sample consisted of n=86 adults, mostly men (n=79, 91.9%) and with a mean age of 45years old (SD=16.85). Patients were diagnosed with GD according to DSM-5 criteria and were undergoing therapy at a Behavioral Addiction Unit when confinement started. Clinical data were collected through a semi-structured interview and protocolized psychometric assessment. A brief telephone survey related to COVID-19 concerns was also administered at the beginning of the lockdown. Dropout data were evaluated at two moments throughout a nine-month observational period (T1: during the lockdown, and T2: after the lockdown).
Results: The risk of dropout during the complete observational period was R=32/86=0.372 (37.2%), the Incidence Density Rate (IDR) ratio T2/T1 being equal to 0.052/0.033=1.60 (p=0.252). Shorter treatment duration (p=0.007), lower anxiety (p=0.025), depressive symptoms (p=0.045) and lower use of adaptive coping strategies (p=0.046) characterized patients who abandoned treatment during the lockdown. Briefer duration of treatment (p=0.001) and higher employment concerns (p=0.044) were highlighted in the individuals who dropped out after the lockdown. Treatment duration was a predictor of dropout in both periods (p=0.005 and p<0.001, respectively).
Conclusion: The present results suggest an impact of the COVID-19 pandemic on treatment dropout among patients with GD during and after the lockdown, being treatment duration a predictor of dropout. Assessing vulnerability features in GD may help clinicians identify high-risk individuals and enhance prevention and treatment approaches in future similar situations.
Introduction
The COVID-19 pandemic, declared as a public health emergency by the World Health Organization [World Health Organization (WHO), 2020], has affected more than 200 countries around the world since the first case appeared in Wuhan (China) in December 2019 (Yuki et al., 2020). Spain has been considered one of the most affected countries by this pandemic since the early stages. Its first case of infection was reported within the first months of 2021, and since then, the number of cases has continued to increase. Along with other European countries, such as Italy and France, Spain has presented one of the highest mortality rates due to the pandemic (Ceylan, 2020).
To control pandemic spread, confinement and other restrictive measures have been stated everywhere (Hale et al., 2020), being social distancing, disruption of daily routines, and economic and employment uncertainty reported as some of the most frequent COVID-19-related concerns (Chew et al., 2020). This context alongside with health worries due to the pandemic has led to an increase in anxiety and depressive symptoms among general population (Islam et al., 2020; Wang et al., 2020). In this vein, people with a mental illness, such as gambling disorder (GD), have been considered at particularly high risk of suffering the psychological impact of the COVID-19 pandemic (González-Sanguino et al., 2020; Özdin and Bayrak Özdin, 2020; Rajkumar, 2020).
GD is defined as engaging in a repeated compulsive gambling behavior, accompanied by unsuccessful efforts to stop the behavior and uncontrollable urges to keep gambling, which leads to considerable distress and impairment. This gambling behavior tends to persist over time despite negative consequences on the person’s physical, mental, social, or financial well-being [American Psychiatry Association (APA), 2013]. GD was included within the “substance-related and addictive disorders” category in the fifth edition of the Diagnostic and Statistical Manual of Mental Disorders [DSM-5; American Psychiatry Association (APA), 2013]. It has been considered the most prevalent behavioral addiction, with a lifetime prevalence ranging from 1.1 to 10.6% (Calado and Griffiths, 2016). In Europe, epidemiological studies have shown that gambling problems range from 0.3 to 3.3% (Dirección General de Ordenación del Juego (DGOJ) 2015).
In the face of environmental changes, maladaptive coping strategies and behaviors, such as those related to gambling activity, could be used to cope with psychological distress (Asmundson and Taylor, 2020; Rajkumar, 2020; Fernández-Aranda et al., 2020a; Avena et al., 2021), specifically in individuals with higher vulnerability (e.g., those with a mental illness; Fernández-Aranda et al., 2020a; Baenas et al., 2020).
During confinement, the use of technologies has extended to more aspects of daily life. Hence, excessive engagement in specific online activities, such as gambling, may lead to severe problems and increases the risk of a disordered or addictive use (King et al., 2020; Mestre-Bach et al., 2020). These maladaptive behaviors are usually presented in combination with social isolation and boredom, factors which have been also linked to confinement and previously associated with the pathogenesis of GD (Ledgerwood and Petry, 2006; Mercer and Eastwood, 2010). On the other hand, the use of gambling has been also stated as a mechanism to deal with adverse economic circumstances in prior financial crises (Olason et al., 2015; Economou et al., 2019). Similarly, economic difficulties due to pandemic and confinement might represent a risk factor that could favor gambling activity. Moreover, some works have described a risk profile for engaging in gambling behavior during confinement (being young male regular gambler with high alcohol use prior to confinement; Håkansson, 2020a; Emond et al., 2021; Hodgins and Stevens, 2021). In the non-clinical study by Price (2020), online gambling modality was associated with the subgroup of gamblers at a higher risk of developing GD, characterized by greater psychological impact due to pandemic and substance use while gambling. In this line, gambling behavior prior to the pandemic predicted gambling activity during confinement, mainly at the expense of online gambling (Gainsbury et al., 2020; Håkansson, 2020b; Emond et al., 2021; Hodgins and Stevens, 2021).
With reference to the potential of the pandemic worsening gambling problems (King et al., 2020; Marsden et al., 2020), legal measures have been adopted by governments in an attempt to minimize these consequences. For instance, in Spain, advertising related to gambling has been limited (SBC News, 2020). Among other useful tools, a consensus guidance on how to avoid problematic use of the internet during the pandemic has been also published (Király et al., 2020). In this guidance, healthy general practices, such as those related to exercise or sleep hygiene were recommended, as well as specific tips regarding Internet use. Monitoring and regulating screen time exposure in children and adults, using digital well-being apps and analogue technical tools, keeping social contact with relatives and friends, or seeking professional help when needed were highly advised (Király et al., 2020).
Among people with GD, the challenges represented by the pandemic and confinement (Håkansson et al., 2020; King et al., 2020) were based on different vulnerability aspects (Allami et al., 2021; Hodgins and Stevens, 2021). For instance, higher levels of anxiety, depressive symptoms and hostility have been described in subjects with GD during confinement, in comparison with the general population (Salerno and Pallanti, 2021). In this context, gambling behavior could be conducted (and reinforced) to regulate emotions (Barrault et al., 2019), being a trigger for relapse in those patients who remained abstinent (Campbell, 2020). However, restrictive strategies and some policies adopted during confinement seemed to reduce gambling opportunities, specifically at offline locations, promoting abstinence (Donati et al., 2021). In this line, patients already in treatment for GD reported a positive attitude about restrictions due to pandemic and related to gambling activity, with improved social and familiar environments. This positive social reinforcement also contributed to maintain abstinence. Interestingly, large-scale migration from offline to online gambling has not been observed so far among patients with GD during confinement (Donati et al., 2021).
Works regarding GD treatment during confinement did not report an increase in treatment-seeking for GD, at least in the short term (Gunstone et al., 2020; Donati et al., 2021; Håkansson et al., 2021). When talking about GD treatment, cognitive-behavioral therapy (CBT) and group-based modalities have been widely demonstrated as effective options for the management of this disorder (Gooding and Tarrier, 2009; Cowlishaw et al., 2012; Jiménez-Murcia et al., 2015). Besides, internet-based treatments for GD demonstrated similar efficacy to traditional treatments (Hedman et al., 2012; Chakrabarti, 2015). Trying more flexible approaches to patient care in the face of pandemic situation (Marsden et al., 2020), tele-psychiatry has shown to be helpful (Hollander and Carr, 2020) and internet-based tools for GD have been used in some treatment facilities during confinement (Columb et al., 2020).
Within the treatment outcome in GD, dropout has been defined as leaving before the completion of a predetermined program (Milton et al., 2002; Robson et al., 2002). Despite a broad dropout range (14–50%) reported by previous national and international studies (Melville et al., 2007; Smith et al., 2010; Jimenez-Murcia et al., 2012), an average of around 26% has been agreed (Melville et al., 2007). Prior to confinement, a recent national study (Jiménez-Murcia et al., 2019) reported dropout rates of 32.4% among patients with GD regarding clinical trajectories based on GD severity among patients following a 12months manualized CBT program. Features such as patients’ medical or family issues, length of therapy, personality traits (e.g., perseverance, reward sensitivity, sensation-seeking), younger age, lower educational level, and neurocognitive variables, among others, have been studied as potential predictors of treatment dropout (Melville et al., 2007; Álvarez-Moya et al., 2011; Jiménez-Murcia et al., 2015; Mallorquí-Bagué et al., 2018, 2019; Mestre-Bach et al., 2019).
So far, most published studies concerning gambling activity during confinement focused on non-clinical populations (Auer et al., 2020; Brown and Hickman, 2020; Gunstone et al., 2020; Lindner et al., 2020; Zamboni et al., 2021). Scarce research on the effects of pandemic on GD treatment outcome and possible associated factors has been reported (Price, 2020; Donati et al., 2021). Furthermore, information related to initial stages of confinement provided a limited knowledge not only regarding its longer-term impact, but also in distinguishing its effects during time periods characterized by different levels of restrictive measures, such as the lockdown and the following months.
To the best of our knowledge, this is the first observational clinical study regarding the effect of the pandemic on GD treatment outcome related to dropout rates among clinical population. The longitudinal nature of the present work favors reporting data comparing two well-established periods of time across confinement (i.e., during and after the lockdown). Finally, the already existing involvement of these patients with the treatment Unit and a suitable observational period allow for evaluating clinical features possibly associated with dropout. Thus, the main aims of this study were to analyze treatment dropout rates in patients with GD in the two established periods, and assess clinical features that would be linked to treatment dropout in both periods.
Materials and Methods
Participants
The sample consisted of n=86 adults, mostly men (n=79, 91.9%) and with a mean age of 45years old (SD=16.85). Participants had been diagnosed according to DSM-5 criteria [American Psychiatry Association (APA), 2013] and were voluntarily recruited in the region of Catalonia (Spain), specifically at the Behavioral Addiction Unit within the Psychiatry Department of a Public University Hospital in Barcelona (Spain). All the patients recruited in the present study were already enrolled in the outpatient treatment program when confinement started. The mean duration of treatment at this point, considering therapy phase and follow-up was 14.3months (SD=9.2months) and around 10.5% (n=9) were not abstinent before the lockdown.
The outpatient treatment program offered in the Unit was based on a standardized protocol (Jiménez-Murcia et al., 2006). It has shown adequate effectiveness for GD in both short- and medium-terms, and has been widely described in previous Unit’s studies related to treatment of GD (Jiménez-Murcia et al., 2006, 2007, 2019; Jimenez-Murcia et al., 2012). It comprised (a) two initial baseline assessment visits, (b) CBT group therapy phase, and (c) a post-therapy follow-up. In the sessions prior to the treatment phase, a semi-structured clinical interview was conducted by expert psychologists and psychiatrists of our Unit with high experience in the field of behavioral addictions (SCID-5; First et al., 2015). Moreover, a protocolized psychometric assessment was also completed in a second session lasting about 90min (Jiménez-Murcia et al., 2006). The treatment phase was composed of 16 weekly sessions with a mean duration of 90min per session, conducted by an experienced clinical psychologist. The main treatment objective was training patients to put into practice CBT strategies to achieve full and definitive abstinence from gambling. Among other techniques, psychoeducation, stimulus control, response prevention, cognitive restructuring, reinforcement and self-reinforcement, skills training, and relapse-prevention techniques were used. Once completed, a post-treatment evaluation was performed, and a 24-month follow-up was initiated before being discharged from the Unit. The therapists recorded dropouts and relapses based on patients’ oral reports and written diaries and relatives’ collateral information. The presence of a co-therapist (e.g., partner, family member, and close friend) was highly recommended.
Contextual Setting Due to the Pandemic
The officially established COVID-19 lockdown period in the region of Catalonia (Spain) extended from March 14 to May 11, 2020. This period was characterized by a stay-at-home policy with measures such as the interruption of all non-essential work, academic, social and leisure activities outside. The coverage of basic products was guaranteed, as well as emergency medical assistance.
During the nine-month observational period of the present study, the described treatment schedule was maintained in each case. However, specific modifications were carried out due to the pandemic situation and restrictive measures. During the lockdown (i.e., March 14, 2020–May 11, 2020), face-to-face treatment was adapted to virtual modality using internet-based tools. Tutorial information was provided to guarantee accessibility and a proper use. Eventually, the presence of the co-therapist was also ensured. After the lockdown, usual face-to-face treatment was resumed (i.e., after May 11, 2020).
Procedure
All the assessments apart from the Brief Telephone Survey related to COVID-19 (Behavioral Addictions Unit, Psychiatry Department, University Hospital, Barcelona, Spain, 2020) were part of the standardized assessment within the Unit’s treatment program previously described (Jiménez-Murcia et al., 2006), and contemplated in other studies related to treatment of GD (Jiménez-Murcia et al., 2006, 2007, 2019; Jimenez-Murcia et al., 2012).
The Brief Telephone Survey related to COVID-19 was obtained in the first therapeutic contact with each patient within the first 2months of confinement in Spain (i.e., lockdown period), mostly at the beginning of the lockdown. Over the nine-month observational period of this study, information regarding dropout (bivariant dropout/non-dropout) was collected by reviewing therapists’ clinical reports. Two dropout periods were distinguished: (T1) between March 14 and May 11 (i.e., during the lockdown), and (T2) between May 12 and December 31, 2020 (i.e., after the lockdown).
Assessments
At Baseline
South Oaks Gambling Screen (SOGS; Lesieur and Blume, 1987): a 20-item instrument for screening for past-year gambling problems and related negative consequences. The total score obtained as the sum of the scored items has been used as a measure of problem-gambling severity, with a score of 5 or more suggestive of “probable pathological gambling.” The Spanish validation of the scale achieved very good psychometric results (test–retest reliability R=0.98, internal consistency α=0.94 and convergent validity R=0.92; Echeburúa et al., 1994). The internal consistency for this scale in the study sample was good (α=0.74).
Diagnostic Questionnaire for Pathological Gambling According to DSM criteria (Stinchfield, 2003): a self-report questionnaire with 19 items coded in a binary scale (yes-no), used for diagnosing GD according to the DSM-IV-TR [American Psychiatry Association (APA), 2000] and DSM-5 [American Psychiatry Association (APA), 2013] criteria. The Spanish adaptation of the questionnaire obtained satisfactory psychometric properties (Cronbach’s alpha α=0.81 for a population-based sample and α=0.77 for a clinical sample; Jiménez-Murcia et al., 2009). The internal consistency for this scale in the study sample was good (α=0.83).
Symptom Checklist-90-Revised (SCL-90-R; Derogatis, 1990): a 90-item self-report questionnaire measured on an ordinal 3-point scale. It evaluates a broad range of psychological problems and psychopathology, based on nine primary symptom dimensions (Somatization, Obsession-Compulsion, Interpersonal Sensitivity, Depression, Anxiety, Hostility, Phobic Anxiety, Paranoid Ideation, and Psychoticism). It includes three global indices (global severity index, positive symptom distress index, and total positive symptom). The validation of the scale in a Spanish population (Derogatis, 2002) obtained a mean internal consistency of α=0.75. The internal consistency in the study was between good (α=0.77 for paranoid ideation) to excellent (α=0.98 for global indexes).
Temperament and Character Inventory-Revised (TCI-R; Cloninger, 1999): a questionnaire with 240-items scored on a 5-point Likert scale and measuring personality derived from three character dimensions (Self-Directedness, Cooperativeness, and Self-Transcendence) and four temperament dimensions (Harm Avoidance, Novelty Seeking, Reward Dependence, and Persistence). Evaluation of the Spanish revised version (Gutiérrez-Zotes et al., 2004) had an internal consistency of α=0.87. We obtained verbal consent from the author for using the TCI-R questionnaire in a public non-profit hospital and exclusively linked to the field of research. This questionnaire was administered in its Spanish adaptation, in which the original author participated (Gutiérrez-Zotes et al., 2004). The internal consistency in the study was between good (α=0.72 for novelty seeking) to excellent (α=0.90 for persistence).
Other variables: Additional data (e.g., socio-demographic, and socio-economic) were measured. They were collected in a semi-structured face-to-face clinical interview as described elsewhere (Jiménez-Murcia et al., 2006). Disorder-related variables such as the age of onset and duration of GD were also assessed. Some variables were updated during the lockdown period as part of the Brief Telephone Survey, as it was described below.
At Lockdown
Brief Telephone Survey (Behavioral Addictions Unit, Psychiatry Department, University Hospital, Barcelona, Spain, 2020). A binary qualitative scale (yes/no) was employed to answer most of the points although some questions were open-ended. The different sections of the survey are described as follows:
a. Socio-demographics in the lockdown situation: (1) employment status, (2) working during confinement, (3) being confined and since when with reference to the beginning of the lockdown period, (4) affected close people by COVID-19, and (5) the presence of social support.
b. GD behaviors in the lockdown situation: (1) increased, reduced, or maintained GD symptoms versus abstinence state and (2) the presence of new symptoms related to GD not referred before the lockdown (e.g., different type of gambling modality).
c. COVID-19-related concerns in the lockdown situation: (1) fear of infection, (2) uncertain future, (3) worries about employment, and (4) difficulties in accessing to treatment.
d. Psychological state due to the pandemic (1) pandemic-related anxiety at psychic, emotional, motor, and cognitive dimensions (e.g., headaches, tachycardia, dizziness, nervousness, avoidance behaviors, recurrent thoughts, intrusions, and anticipation) and (2) the presence of depressive-symptoms in the lockdown situation (e.g., presence of hopelessness, pessimism, discouragement, sleepiness, indifference, lack of illusion, crying, social/communicative isolation, and passive thoughts of death).
e. Questions about other items, such as (1) boredom, (2) frequent arguments with cohabitants, family, friends, and (3) receiving and looking for continuous information on COVID-19 were also included.
f. Finally, coping strategies during confinement were evaluated in two ways: (1) as the presence or absence of both adaptive and maladaptive mechanisms (yes/no) and (2) the kind of strategies used, categorizing the coping mechanisms in five dimensions for adaptive strategies (i.e., social contact, leisure, sporting activities, daily routines, and academic/work activity) and three related to maladaptive ones (i.e., obtaining too much COVID-19-related information; behaviors related to GD and other maladaptive behaviors, such as substance use).
Statistical Analysis
Statistical analysis was conducted with Stata17 for Windows (StateCorp, 2021). First, the incidence density rate (IDR, also called the person-time incidence rate) of dropout and relapse was estimated, and comparison between the periods T1 (i.e., during the lockdown) and T2 (i.e., after the lockdown) were performed. IDR is the measure of the frequency with which the event occurs (in the study dropout and gambling-episodes) over a specified period. The denominator of this estimation is the product of the person-time of the at-risk sample, and therefore this measurement is not dependent on the duration of the observational period.
Next, chi-square tests (χ2) were evaluated comparisons between groups for categorical variables, and T-TEST for quantitative measures. For these analyses, Cohen’s h coefficients measured effect sizes for proportion comparisons and Cohen’s d for mean comparisons (null effect size was considered for values |h|<0.20 or |d|<0.20, low-poor for |h|>0.20 or |d|>0.20, moderate-medium for |h|>0.50 or |d|>0.50, and large-high for |h|>0.80 or |d|>0.80; Cohen, 1988; Kelley and Preacher, 2012). In addition, the Finner’s-method (a family-wise error rate stepwise procedure, a less stringent approach than a conservative Bonferroni correction) was used to control Type-I error related to multiple comparisons (Finner and Roters, 2001).
Third, logistic regressions explored significant contributors for the risk of dropout during the observational period, considering as potential predictors socio-demographic variables (sex, age, marital status, education, employment, and social position index), contextual variables during COVID-19-related confinement, GD-related measures at baseline/prior to the confinement (age of onset and duration of gambling problems, and problem-gambling severity), personality measures (TCI-R scores) and psychological distress at baseline (SCL-90R GSI). These analyses used a stepwise method to automatically select significant statistical predictors separately for the risk of dropout during the lockdown (i.e., T1) and after the lockdown (i.e., T2).
Results
Characteristics of the Sample
Most participants were men (91.9%), employed (53.5%) and within mean-low to low social position indexes (75.5%). Many were single (44.4%) or married (43.0%), with primary (45.3%) or secondary (40.7%) education levels. The mean age was 45.0years (SD=16.9), mean age of onset of GD was 31.8years (SD=12.7) and mean duration of GD was 6.2years (SD=7.8). The most frequently acknowledged preferred gambling form was non-strategic [46.5%, followed by strategic (33.7%), and mixed (19.8%)], and preferred gambling locations was through offline platforms (66.3%). Table 1 includes the complete description for the variables of the study measured at baseline, prior to the COVID-19-related confinement.
Environmental Contextual Factors During COVID-19-Related Confinement
Table 2 includes the frequency distribution of the variables assessing contextual environment and personal measures during the COVID-19-related confinement. The percentage of participants reporting working was 25.6%, while 89.5% lived with other people, and 5.8% indicated an affected close people by COVID-19.
The percentage of participants reporting COVID-19-related concerns was 67.4%. Most patients employed adaptive reactions (74.4%), while 22.1% reported maladaptive reactions. The presence of anxiety symptoms was reported by 25.6% of the participants, and depressive symptoms by 19.8%. Family conflicts due to confinement were reported by 9.3% of participants.
Factors Contributing to Dropout During the Observational Period
The risk of dropout during the complete observational period was R=32/86=0.372 (37.2%), and the IDR was 0.045 per person month (4.5 patients dropped out per 100 participants-month). Considering separately the two periods of time of the observational period (Figure 1), IDR increased from 0.033 (3.3 individuals dropped out per 100 participants-month) at T1 to 0.052 (5.2 dropped out per 100 participants-month) at T2, the IDR ratio being equal to 0.052/0.033=1.60 (p=0.252).
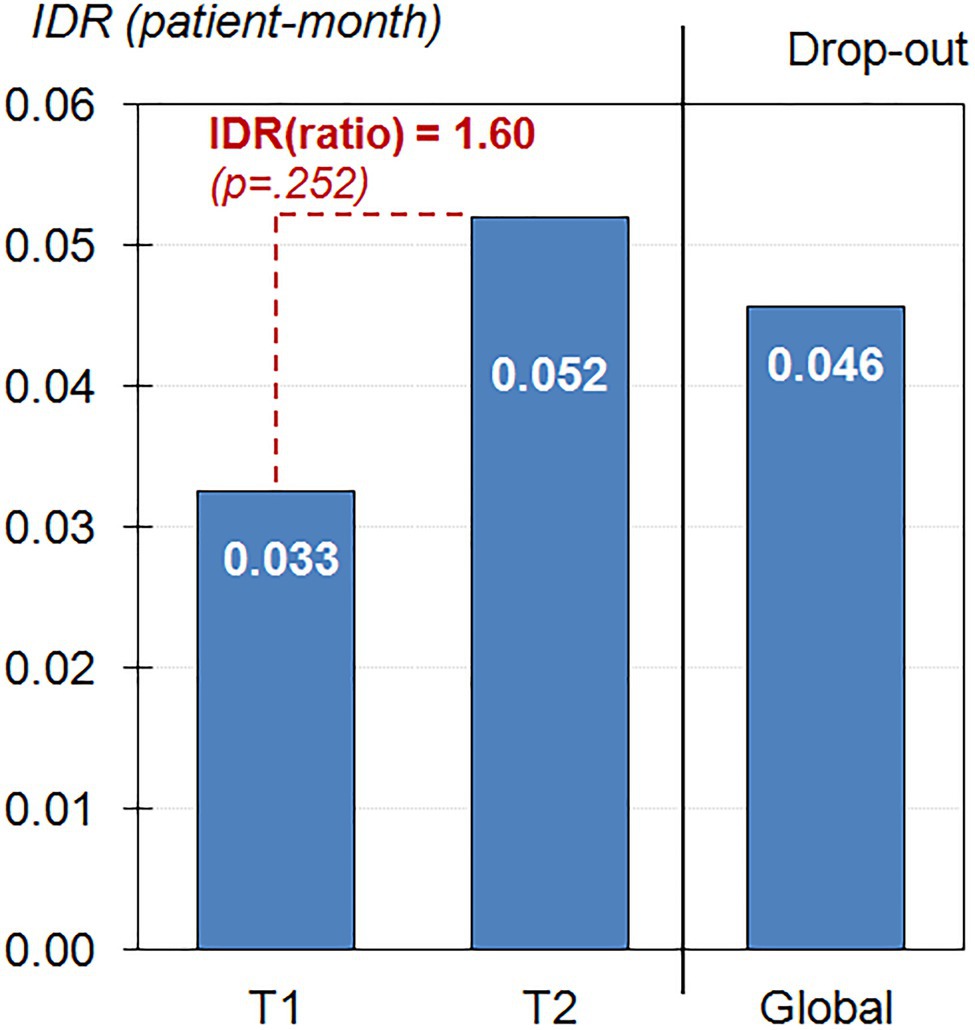
Figure 1. Incidence Density Rate (IDR) of Dropout during the nine-month observational period (n=86).
Figure 2 shows the survival functions (Kaplan–Meier product-limit estimator) for dropouts, considering the period from the regional COVID-19-related the lockdown and the end of the observational period (March 2020–December 2020). The curve in the upper part of the figure (continuous-line) represents the cumulative survival function, and the lower curve (dash-line) the inverse 1-cumulative survival function. The cumulate survival function estimates the proportion of patients surviving in treatment (without dropout) for certain amounts of time. The inverse curve (1-cumulative survival function) plots the cumulate proportion of dropouts during the observational period. The shape of the cumulate survival function, in the form of regular jumps/rectangles during the period March–July suggested that most dropouts were observed during this period, (concretely, 31.4% of participants had abandoned during these months). The remaining 5.8% of dropouts were registered during the following months (August–December).
The frequency of relapses was very low during the complete observational period. Figure 3 contains the bar-chart with the IDR of gambling episodes in the study. The lowest IDR was registered during the lockdown period (1.2 patients reported relapses per 100 participants-month), followed by the post-lockdown stage (1.3 patients reported relapses per 100 participants-month). Compared with the period prior to when confinement started (IDR=0.037 at baseline, 3.7 relapses per 100 participants-month), the probability of relapses during the lockdown was 3.11 times lower (p=0.049), and after the lockdown the probability of relapses was 2.87 times lower (p=0.041). No statistical differences was observed comparing the IDR during and after the lockdown (p=0.941).
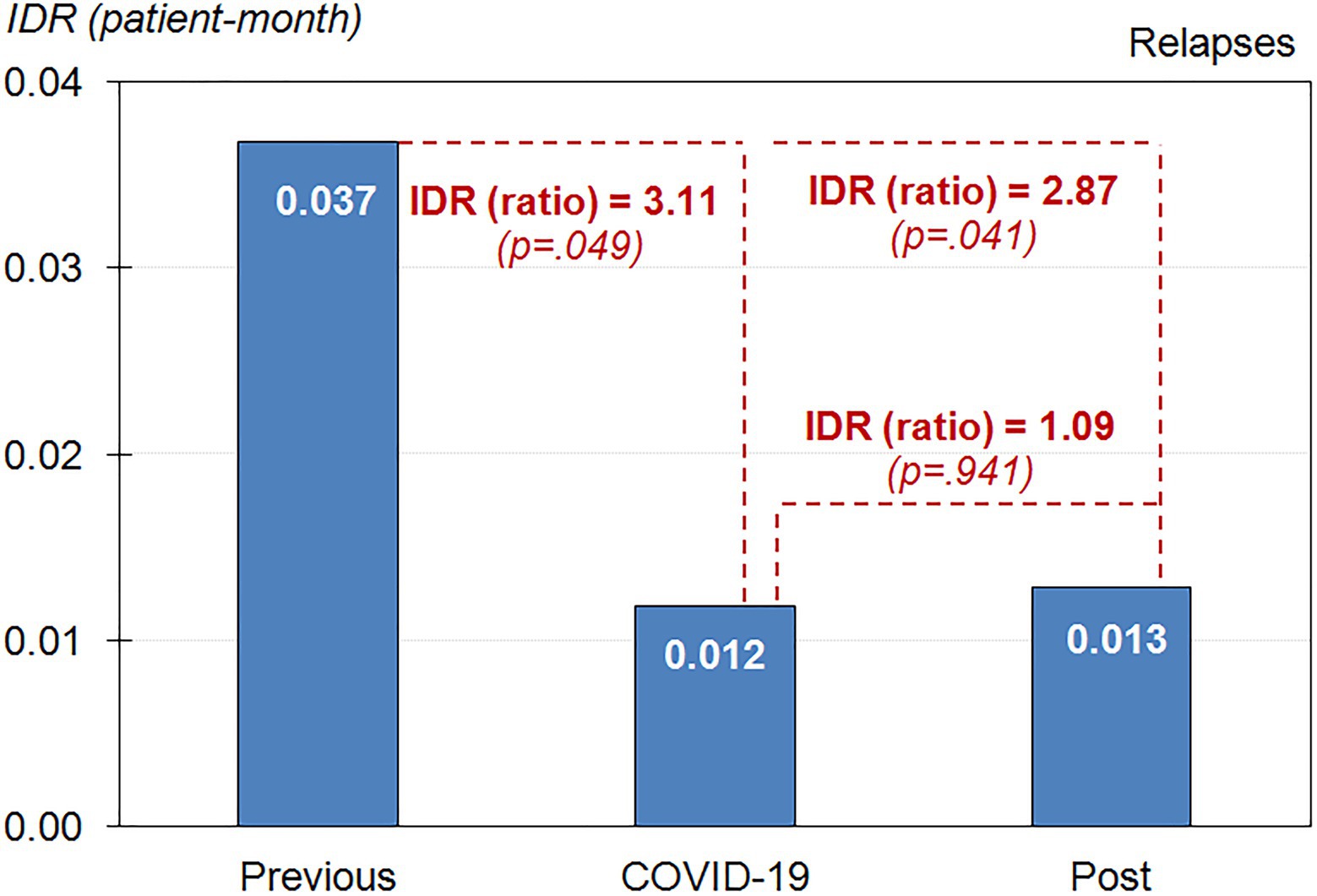
Figure 3. Incidence Density Rate (IDR) of relapse rates during the nine-month observational period (n=86).
Table 3 shows the bivariate comparisons between the groups defined based on the dropouts (non-dropout versus dropout) for the variables of the study (socio-demographics, gambling related measures, clinical profile, and those variables collected via the Brief Telephone Survey). Separate analyses were performed for the T1 and T2 stages. Variables related to the increase in the risk of dropout during T1 were male sex, higher education levels, higher social position indexes, lower SCL-90R anxiety and psychotic scores, lower use of adaptive and non-adaptive reactions, and lower level of anxiety and depression symptoms due to pandemic. Online gambling was a protective factor for dropout during T1. Variables contributing to increase the risk of dropout during T2 were shorter duration of treatment and more concerns related to employment status during the lockdown.
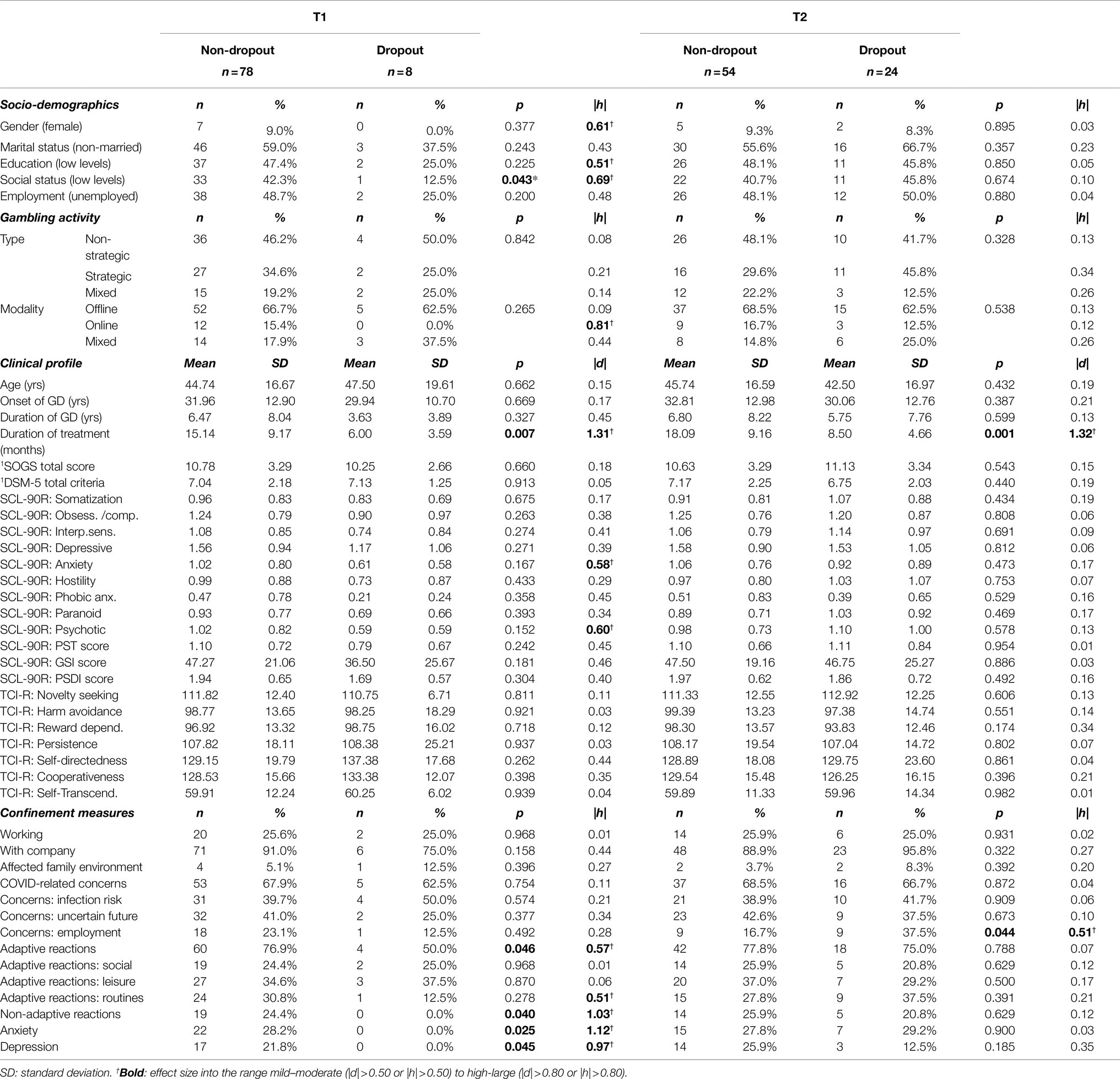
Table 3. Association between socio-demographics and dropout during two periods of the COVID-19 pandemic: during (T1) and after (T2) the lockdown.
Table 4 contains the final models obtained in the stepwise logistic regression models, considering the risk of dropout as criterion and as potential predictors the socio-demographic variables, the gambling related measures at baseline, clinical state at baseline and during the lockdown, as well as the contextual factors during the lockdown. The three final models obtained considering separately the stages T1 and T2, and the entire observational period, retained as the only significant predictor the duration of the GD treatment: the shorter the duration of treatment, the higher the likelihood of dropout.
Discussion
The present study was designed to explore the impact of the COVID-19 pandemic and confinement on treatment adherence among patients with GD. For this purpose, we evaluated dropout rates both during and after the lockdown. The relationship between clinical variables and dropout was also explored in both periods.
Most patients of the sample were male and employed, and their main gambling problem involved offline gambling. This socio-demographic and clinical profile is similar to the characterization reported in previous studies of our Unit (Jiménez-Murcia et al., 2007, 2015). Regarding treatment outcome, the risk of dropout over the nine-month observational period reached similar values to those mentioned in the literature (Melville et al., 2007) and described before confinement started (Jiménez-Murcia et al., 2019). A slightly lower mean incidence of dropout was found during the lockdown in comparison with the following months although without reaching statistical significance. The restrictive characteristics of the lockdown has allowed for greater stimulus control, especially related to offline gambling activity. Consequently, the reduction of gambling opportunities, improved social/familiar environments (Donati et al., 2021), and the maintenance of an active treatment adapted to the context might be considered as protective factors (e.g., reassessing the situation and seeking alternative methods of treatment and prevention; Lee and Rovers, 2008; Côté et al., 2020).
Lower levels of anxiety and depressive symptoms due to pandemic and higher socio-economic positions at baseline were stated among the individuals who dropped out during the lockdown. These findings possibly suggest that those patients with better socio-economic background prior to confinement and lower referred psychological impact due to pandemic (in terms of anxiety and depressive symptoms) may have been more likely to discontinue treatment, already from the initial stages of confinement. Also, a lower use of adaptive coping strategies during the lockdown was also described in patients who dropped out in comparison to those who remained in treatment. In contrast, patients with greater insight about their gambling problem and more urgent needs for professional help may have been more likely to remain in treatment, even if the need was not directly related to gambling behavior (i.e., social support, anxiety, and depressive symptoms). Several individuals who remained in treatment reported using non-adaptive strategies during the lockdown (such as concerns related to COVID-19 and gambling behavior). In this sense, we hypothesize that treatment attendance may represent an adaptive mechanism to deal with emotional distress. Hence, treatment should focus on coping with emotions as a helpful approach to tackle gambling problems in the face of similar future adverse circumstances (Estévez et al., 2021).
During the lockdown, a more flexible approach to treatment was employed (Marsden et al., 2020), using Internet-based tools therapeutic strategies (Columb et al., 2020). This approach may have influenced treatment adherence in patients who were already in treatment for GD, from two different perspectives. On the one hand, online therapy was more accessible to people who had previously found it difficult to attend (American Psychological Association, 2015). Moreover, the relationship between therapist and patient in the case of virtual therapy has been described as good as for in-person therapy (Bergman and Kelly, 2021), allowing the therapeutic alliance to be preserved in these exceptional circumstances. On the other hand, the absence of in-person activities, a lack of peer-to-peer social and emotional connections, inequities in Internet access, and online distractions could represent challenges for adapting to shifting from face-to-face to online therapy. These issues have been previously reported in virtual therapeutic approaches in other mental disorders during confinement, such as in substance use disorders and eating disorders (Fernández-Aranda et al., 2020b; Bergman and Kelly, 2021). In the present study, although changes in the treatment approach did not seem to have detrimental effects on treatment adherence, some of the patients who dropped out may have presented some of the described difficulties. Pandemic situation highlights the need to efficiently adapt the available treatment strategies to these exceptional circumstances to ensure therapeutic continuity. In this vein, customizing proven treatment strategies to virtual formats has been a useful tool, preserving the initial therapeutic objectives.
After the lockdown, restrictive measures were progressively reduced (e.g., related to “stay-at-home” measures, social distancing, and diminished mobility) and the treatment program in its original format was reinstated. Dropout was associated with more employment concerns due to the pandemic. In this line, the financial pressure of job insecurity and unemployment could motivate individuals to use gambling as a mechanism to earn money and manage debts (van Schalkwyk et al., 2020; Salerno and Pallanti, 2021). Also, previous financial crises have shown the possibility of engaging in gambling behaviors to attempt economic restitution (Olason et al., 2015; Economou et al., 2019). One plausible explanation for our results could be that those patients reporting work instability and difficult financial situations due to the lockdown could have relapsed and abandoned treatment. Another possible rationale could be that, coinciding with the easing of restrictions after the lockdown, some patients may find it difficult to juggle work and treatment, prioritizing the search and maintenance of a job over treatment. While many of these notions are currently speculative, they warrant direct examination in future studies.
The survival analysis showed that most dropouts were concentrated in the first months of this period, especially between May and July 2020. These months in Spain could be considered as a transition period between the measures taken during the lockdown and the beginning of the gradual phasing out of these measures. It was characterized by an increased uncertainty about the return to daily routines (e.g., work routines), the ubiquity of social contact and health concerns related to COVID-19. The general instability of this phase, along with the reopening of the gambling establishments, made these first months after lockdown a particular period of high vulnerability for relapse and for treatment dropout. Furthermore, some of the patients who abandoned treatment during the first months of confinement were in the early stages of the treatment phase. These results are in line with previous studies which described a predominant tendency to dropout at the start of the treatment or after the first few sessions (Jiménez-Murcia et al., 2015).
Going one step further, mean incidence of relapse for gambling behavior was significantly decreased during and after lockdown in comparison with mean rates prior to the confinement started. However, there were not differences in relapse rates between during and after the lockdown. Most patients reported mainly gambling offline and living with others during the nine-month observational period. A high abstinence from gambling of around 75% has previously been described in patients who were already in CBT treatment and/or in the follow-up phase (Jiménez-Murcia et al., 2007; Cowlishaw et al., 2012). In the context of pandemic, our results also agreed with recent literature that pointed out higher abstinence rate during confinement (Avanzi et al., 2020). In line with Donati et al. (2021), restrictive measures due to pandemic, social support and some policies related to gambling activity adopted during confinement, have promoted gambling abstinence by reducing offline gambling opportunities.
Finally, an association between treatment duration and dropout was found during and after lockdown. Specifically, the shorter the duration of treatment, the higher the risk of dropout during and after the lockdown. Furthermore, and according to the predictive analysis model, the duration of the treatment would be a predictive factor of dropout. Length of therapy has been positively related to treatment outcome in previous studies (Orlinsky et al., 1994). In this sense, achieving a strong therapeutic alliance with the patient early in treatment is important for improving adherence to therapy (Gómez-Peña et al., 2012). Therefore, patients with weaker therapeutic links and lower durations of treatment may have found it more challenging to preserve treatment adherence in the face of similar adverse situations.
Strengths and Limitations
The strengths of the study included a clinical sample of patients already engaged with our treatment Unit. Moreover, we used clinical records and assessments to evaluate patients rather than just self-report measures. The longitudinal nature of this work allowed reporting treatment outcomes related to data over the course of 9months. Limitations included the modest sample size, limited geographic location, and the absence of a control group for comparison and multiple qualitative assessments. Some clinical and socio-demographic variables were collected prior to the onset of the lockdown (i.e., at the baseline evaluation prior to treatment) and their possible changes due to the pandemic were updated in the short-term during confinement. However, the influence of new changes in these variables in the middle-long term should be contemplated in future research. Moreover, upcoming studies would benefit from including quantitative validated scales and evaluating changes over longer time periods in GD symptomatology and associated factors, throughout different stages of the COVID-19 pandemic in multiple jurisdictions and cultures.
Conclusion
In conclusion, the present findings provide data on the treatment dropout in clinical population diagnosed with GD and potential contributing factors during confinement. In this line, duration of treatment could be a possible predictor of dropout in the face of adverse external situations. These results have relevance for identifying potentially high-risk subjects and optimizing individualized early interventions in the setting of future similar adverse circumstances.
Data Availability Statement
Individuals may inquire with Dr. Jiménez-Murcia regarding availability of the data as there is ongoing studies using the data. To avoid overlapping research efforts, Dr. Jiménez-Murcia will consider a request on a case-by-case basis.
Ethics Statement
The present study was conducted in accordance with the latest version of the Declaration of Helsinki. The Clinical Research Ethics Committee of the first author’s Public University Hospital gave approval before confinement, as most of the data used in this study was part of the baseline 308 assessment protocol prior to the start of treatment and previously used in the Unit’s studies related to treatment (Ref: PR329/19). We added the brief telephone survey to this protocol at the beginning of confinement. Signed informed consent was obtained from all participants.
Author Contributions
IB, EC, ME, and SJ-M contributed to the development of the study concept and design and aided with interpretation of data and the writing of the manuscript. RG performed the statistical analysis. IB, MG-P, LM, SR, AP-G, BM-M, EV-M, and SJ-M aided with data collection. FF-A, SJ-M, MP, AH, and JM revised the manuscript and provided substantial comments. FF-A and SJ-M obtained funding. All authors contributed to the article and approved the submitted version.
Funding
CERCA Programme/Generalitat de Catalunya gave institutional support. This work was additionally supported by a grant from the Ministerio de Ciencia, Innovación y Universidades (grant RTI2018-101837-B-100). The research was funded by the Delegación del Gobierno para el Plan Nacional sobre Drogas (2017I067 and 2019I47), Instituto de Salud Carlos III (ISCIII) (PI17/01167) and co-funded by FEDER funds/European Regional Development Fund (ERDF), a way to build Europe. CIBEROBN and CIBERSAM are both initiatives of ISCIII. IB was partially supported by a Post-Residency Grant from Research Committee of the University Hospital of Bellvitge (HUB; Barcelona, Spain) 2020–2021. The funders had no role in the study design, data collection and analysis, decision to publish or preparation of the manuscript.
Conflict of Interest
AH holds a position at Lund University, Sweden, sponsored by the state-owned Swedish gambling operator AB Svenska Spel and has funding from the research council of the same organization and from the research councils of the Swedish state-owned alcohol monopoly Systembolaget AB and the Swedish sports federation. MP notes the following disclosures. He has: consulted for and advised Game Day Data, the Addiction Policy Forum, AXA, Idorsia, and Opiant/Lakelight Therapeutics; received research support from the Veteran’s Administration, Mohegan Sun Casino, and the National Center for Responsible Gaming (on the International Center for Responsible Gambling); participated in surveys, mailings, or telephone consultations related to addictions, impulse-control disorders or other health topics; consulted for law offices and the federal public defender’s office in issues related to impulse-control and addictive disorders; provided clinical care in the Connecticut Department of Mental Health and Addiction Services Problem Gambling Services Program; performed grant reviews for the National Institutes of Health and other agencies; edited journals and journal sections; given academic lectures in grand rounds, CME events and other clinical/scientific venues; and generated books or chapters for publishers of mental health texts.
The remaining authors declare that the research was conducted in the absence of any commercial or financial relationships that could be construed as a potential conflict of interest.
Publisher’s Note
All claims expressed in this article are solely those of the authors and do not necessarily represent those of their affiliated organizations, or those of the publisher, the editors and the reviewers. Any product that may be evaluated in this article, or claim that may be made by its manufacturer, is not guaranteed or endorsed by the publisher.
Acknowledgments
We thank CERCA Programme/Generalitat de Catalunya, Instituto de Salud Carlos III (ICSIII) and Instituto de Investigación Biomédica de Bellvitge (IDIBELL).
References
Allami, Y., Hodgins, D. C., Young, M., Brunelle, N., Currie, S., Dufour, M., et al. (2021). A meta-analysis of problem gambling risk factors in the general adult population. Addiction 116, 2968–2977. doi: 10.1111/add.15449
Álvarez-Moya, E. M., Ochoa, C., Jiménez-Murcia, S., Aymamí, M. N., Gómez-Peña, M., Fernández-Aranda, F., et al. (2011). Effect of executive functioning, decision-making and self-reported impulsivity on the treatment outcome of pathologic gambling. J. Psychiatry Neurosci. 36, 165–175. doi: 10.1503/jpn.090095
American Psychiatry Association (APA) (2000). Diagnostic and Statistical Manual of Mental Disorders. 4th Edn. Washington, DC: American Psychiatric Association.
American Psychiatry Association (APA) (2013). Diagnostic and Statistical Manual of Mental Disorders. 5th Edn. Washington DC: American Psychiatric Association, doi: 10.1176/appi.books.9780890425596
American Psychological Association. (2015). What you Need to Know Before Choosing Online Therapy. Available at: https://www.apa.org/topics/online-therapy
Asmundson, G. J. G., and Taylor, S. (2020). Coronaphobia: fear and the 2019-nCoV outbreak. J. Anxiety Disord. 70. doi: 10.1016/j.janxdis.2020.102196
Auer, M., Malischnig, D., and Griffiths, M. D. (2020). Gambling before and during the COVID-19 pandemic among European regular sports bettors: an empirical study using behavioral tracking data. Int. J. Ment. Health Addict., 1–8. doi: 10.1007/s11469-020-00327-8
Avanzi, M., Calabrese, A., and Cabrini, S. (2020). Il Disturbo da Gioco d’Azzardo (DGA) al tempo della pandemia di COVID-19: Il punto di vista dei SerDP. Alea Bull 8, 13–17.
Avena, N. M., Simkus, J., Lewandowski, A., Gold, M. S., and Potenza, M. N. (2021). Substance use disorders and behavioral addictions during the COVID-19 pandemic and COVID-19-related restrictions. Front. Psych. 12. doi: 10.3389/fpsyt.2021.653674
Baenas, I., Caravaca-Sanz, E., Granero, R., Sánchez, I., Riesco, N., Testa, G., et al. (2020). COVID-19 and eating disorders during confinement: analysis of factors associated with resilience and aggravation of symptoms. Eur. Eat. Disord. Rev. 28, 855–863. doi: 10.1002/erv.2771
Barrault, S., Mathieu, S., Brunault, P., and Varescon, I. (2019). Does gambling type moderate the links between problem gambling, emotion regulation, anxiety, depression and gambling motives. Int. Gambl. Stud. 19, 54–68. doi: 10.1080/14459795.2018.1501403
Bergman, B. G., and Kelly, J. F. (2021). Online digital recovery support services: An overview of the science and their potential to help individuals with substance use disorder during COVID-19 and beyond. J. Subst. Abus. Treat. 120:108152. doi: 10.1016/j.jsat.2020.108152
Brown, R., and Hickman, A. (2020). “Changes in online gambling during the COVID-19 pandemic,” in Statistical Bulletin no. 25 (Canberra: Australian Institute of Criminology).
Calado, F., and Griffiths, M. D. (2016). Problem gambling worldwide: An update and systematic review of empirical research (2000-2015). J. Behav. Addict. 5, 592–613. doi: 10.1556/2006.5.2016.073
Campbell, C. (2020). Coronavirus: Lockdown leaves addicts ‘close to relapse’. Available at: https://www.bbc.com/news/uk-northern-ireland-52811931
Ceylan, Z. (2020). Estimation of COVID-19 prevalence in Italy, Spain, and France. Sci. Total Environ. 729:138817. doi: 10.1016/j.scitotenv.2020.138817
Chakrabarti, S. (2015). Usefulness of telepsychiatry: A critical evaluation of videoconferencing-based approaches. World J. Psychiatry 5:286. doi: 10.5498/wjp.v5.i3.286
Chew, Q. H., Wei, K. C., Vasoo, S., Chua, H. C., and Sim, K. (2020). Narrative synthesis of psychological and coping responses towards emerging infectious disease outbreaks in the general population: practical considerations for the COVID-19 pandemic. Singap. Med. J. 61, 350–356. doi: 10.11622/smedj.2020046
Cloninger, C. R. (1999). The Temperament and Character Inventory—Revised. St Louis, MO, USA: Center for Psychobiology of Personality, Washington University.
Cohen, J. (1988). Statistical Power Analysis for the Behavioral Sciences (2nd ed.). Hillsdale, NJ: Lawrence Earlbaum Associates.
Columb, D., Hussain, R., and O’Gara, C. (2020). Addiction psychiatry and COVID-19: impact on patients and service provision. Ir. J. Psychol. Med. 37, 164–168. doi: 10.1017/ipm.2020.47
Côté, M., Tremblay, J., Jiménez-Murcia, S., Fernàndez-Aranda, F., and Brunelle, N. (2020). How can partners influence the gambling habits of their gambler spouse? J. Gambl. Stud. 36, 783–808. doi: 10.1007/s10899-019-09917-1
Cowlishaw, S., Merkouris, S., Dowling, N., Anderson, C., Jackson, A., and Thomas, S. (2012). Psychological therapies for pathological and problem gambling. Cochrane Database Syst. Rev. 11. doi: 10.1002/14651858.CD008937.pub2
Derogatis, L. R. (1990). SCL-90-R: Symptom Checklist-90-R. Administration, Scoring and Procedures Manuall—II for the Revised Version. Clinical Psychometric Research.
Dirección General de Ordenación del Juego (DGOJ) (2015). Estudio sobre prevalencia, comportamiento y características de los usuarios de juegos de azar en España. Retrieved 05 Sep 2018 from Dirección General de Ordenación del juego website. Available at: https://www.ordenacionjuego.es/es/estudios-informes
Donati, M. A., Cabrini, S., Capitanucci, D., Primi, C., Smaniotto, R., Avanzi, M., et al. (2021). Being a gambler during the covid-19 pandemic: A study with italian patients and the effects of reduced exposition. Int. J. Environ. Res. Public Health 18, 1–19. doi: 10.3390/ijerph18020424
Echeburúa, E., Baez, C., Fernández-Montalvo, J., and Páez, D. (1994). Cuestionario de Juego Patológico de South Oaks (SOGS): validación española. Análisis y Modif. la Conduct. 20, 769–791.
Economou, M., Souliotis, K., Malliori, M., Peppou, L. E., Kontoangelos, K., Lazaratou, H., et al. (2019). Problem gambling in Greece: prevalence and risk factors during the financial crisis. J. Gambl. Stud. 35, 1193–1210. doi: 10.1007/s10899-019-09843-2
Emond, A., Nairn, A., Collard, S., and Hollén, L. (2021). Gambling by young adults in the UK during COVID-19 lockdown. J. Gambl. Stud. 17, 1–13. doi: 10.1007/s10899-021-10029-y
Estévez, A., Jáuregui, P., Lopez-Gonzalez, H., Mena-Moreno, T., Lozano-Madrid, M., Macia, L., et al. (2021). The severity of gambling and gambling related cognitions as predictors of emotional regulation and coping strategies in adolescents. J. Gambl. Stud. 37, 483–495. doi: 10.1007/s10899-020-09953-2
Fernández-Aranda, F., Casas, M., Claes, L., Bryan, D. C., Favaro, A., Granero, R., et al. (2020a). COVID-19 and implications for eating disorders. Eur. Eat. Disord. Rev. 28, 239–245. doi: 10.1002/erv.2738
Fernández-Aranda, F., Munguía, L., Mestre-Bach, G., Steward, T., Etxandi, M., Baenas, I., et al. (2020b). COVID isolation eating scale (CIES): analysis of the impact of confinement in eating disorders and obesity—A collaborative international study. Eur. Eat. Disord. Rev. 28, 871–883. doi: 10.1002/erv.2784
Finner, H., and Roters, M. (2001). On the false discovery rate and expected type I errors. Biom. J. 43, 985–1005. doi: 10.1002/1521-4036(200112)43:8<985::AID-BIMJ985>3.0.CO;2-4
First, M. B., Williams, J. B. W., Karg, R. S., and Spitzer, R. L. (2015). Structured Clinical Interview for DSM-5 Research Version. Washington D.C: American Psychiatric Association.
Gainsbury, S., Swanton, T., Burgess, M., and Blaszczynski, A. (2020). Impacts of the COVID-19 shutdown on gambling patterns in Australia: consideration of problem gambling and psychological distress. J. Addict. Med. 15, 468–476. doi: 10.1097/ADM.0000000000000793
Gómez-Peña, M., Penelo, E., Granero, R., Fernández-Aranda, F., Álvarez-Moya, E., Santamaría, J. J., et al. (2012). Correlates of motivation to change in pathological gamblers completing cognitive-behavioral group therapy. J. Clin. Psychol. 68, 732–744. doi: 10.1002/jclp.21867
González-Sanguino, C., Ausín, B., Castellanos, M. Á., Saiz, J., López-Gómez, A., Ugidos, C., et al. (2020). Mental health consequences during the initial stage of the 2020 coronavirus pandemic (COVID-19) in Spain. Brain Behav. Immun. 87, 172–176. doi: 10.1016/j.bbi.2020.05.040
Gooding, P., and Tarrier, N. (2009). A systematic review and meta-analysis of cognitive-behavioural interventions to reduce problem gambling: hedging our bets? Behav. Res. Ther. 47, 592–607. doi: 10.1016/j.brat.2009.04.002
Gunstone, B., Gosschalk, K., Joyner, O., Diaconu, A., and Sheikh, M. (2020). The impact of the COVID19 lockdown on gambling behaviour, harms and demand for treatment and support.
Gutiérrez-Zotes, J. A., Bayón, C., Montserrat, C., Valero, J., Labad, A., Cloninger, C. R., et al. (2004). Temperament and character inventory revised (TCI-R). Standardization and normative data in a general population sample. Actas Esp. Psiquiatr. 32, 8–15.
Håkansson, A. (2020a). Changes in gambling behavior during the COVID-19 pandemic—a web survey study in Sweden. Int. J. Environ. Res. Public Health 17, 1–16. doi: 10.3390/ijerph17114013
Håkansson, A. (2020b). Impact of COVID-19 on online gambling – a general population survey during the pandemic. Front. Psychol. 11:568543. doi: 10.3389/fpsyg.2020.568543
Håkansson, A., Åkesson, G., Grudet, C., and Broman, N. (2021). No apparent increase in treatment uptake for gambling disorder during ten months of the covid-19 pandemic—analysis of a regional specialized treatment unit in Sweden. Int. J. Environ. Res. Public Health 18, 1–10. doi: 10.3390/ijerph18041918
Håkansson, A., Fernández-Aranda, F., Menchón, J. M., Potenza, M. N., and Jiménez-Murcia, S. (2020). Gambling during the COVID-19 crisis – a cause for concern. J. Addict. Med. 14, e10–e12. doi: 10.1097/ADM.0000000000000690
Hale, T., Petherick, A., Phillips, T., and Webster, S. (2020). Variation in Government Responses to COVID-19” Version 3.0. Blavatnik School of Government Working Paper. Available at: www.bsg.ox.ac.uk/covidtracker
Hedman, E., Ljótsson, B., and Lindefors, N. (2012). Cognitive behavior therapy via the internet: A systematic review of applications, clinical efficacy and cost-effectiveness. Expert Rev. Pharmacoeconomics Outcomes Res. 12, 745–764. doi: 10.1586/erp.12.67
Hodgins, D. C., and Stevens, R. M. G. (2021). The impact of COVID-19 on gambling and gambling disorder: emerging data. Curr. Opin. Psychiatry 34, 332–343. doi: 10.1097/YCO.0000000000000709
Hollander, J. E., and Carr, B. G. (2020). Virtually perfect? Telemedicine for Covid-19. N. Engl. J. Med. 382, 1679–1681. doi: 10.1056/NEJMp2003539
Islam, M. S., Ferdous, M. Z., and Potenza, M. N. (2020). Panic and generalized anxiety during the COVID-19 pandemic among Bangladeshi people: an online pilot survey early in the outbreak. J. Affect. Disord. 276, 30–37. doi: 10.1016/j.jad.2020.06.049
Jiménez-Murcia, S., Álvarez-Moya, E. M., Granero, R., Aymami, M. N., Gómez-Peña, M., Jaurrieta, N., et al. (2007). Cognitive-behavioral group treatment for pathological gambling: analysis of effectiveness and predictors of therapy outcome. Psychother. 36, 165–175. Res. doi: 10.1080/10503300601158822
Jimenez-Murcia, S., Aymamí, N., Gómez-Peña, M., Santamaría, J. J., Álvarez-Moya, E., Fernández-Aranda, F., et al. (2012). Does exposure and response prevention improve the results of group cognitive-behavioural therapy for male slot machine pathological gamblers? Br. J. Clin. Psychol. 51, 54–71. doi: 10.1111/j.2044-8260.2011.02012.x
Jiménez-Murcia, S., Aymamí-Sanromà, M., Gómez-Pena, M., Álvarez-Moya, E., and Vallejo, J. (2006). Protocols de tractament cognitivoconductual pel joc patològic i d’altres addiccions no tòxiques [Protocols of cognitive-behaviour therapy for pathological gambling and other behavioural addictions] University Hospital of Bellvitge, Barcelona, Spain.
Jiménez-Murcia, S., Granero, R., Fernández-Aranda, F., Arcelus, J., Aymamí, M. N., Gómez-Peña, M., et al. (2015). Predictors of outcome among pathological gamblers receiving cognitive behavioral group therapy. Eur. Addict. Res. 21, 169–178. doi: 10.1159/000369528
Jiménez-Murcia, S., Granero, R., Fernández-Aranda, F., Aymamí, N., Gómez-Peña, M., Mestre-Bach, G., et al. (2019). Developmental trajectories of gambling severity after cognitive-behavioral therapy. Eur. Psychiatry 60, 28–40. doi: 10.1016/j.eurpsy.2019.04.001
Jiménez-Murcia, S., Stinchfield, R., Álvarez-Moya, E., Jaurrieta, N., Bueno, B., Granero, R., et al. (2009). Reliability, validity, and classification accuracy of a spanish translation of a measure of DSM-IV diagnostic criteria for pathological gambling. J. Gambl. Stud. 25, 93–104. doi: 10.1007/s10899-008-9104-x
Kelley, K., and Preacher, K. J. (2012). On effect size. Psychol. Methods 17, 137–152. doi: 10.1037/a0028086
King, D. L., Delfabbro, P. H., Billieux, J., and Potenza, M. N. (2020). Problematic online gaming and the COVID-19 pandemic. J. Behav. Addict. 9, 184–186. doi: 10.1556/2006.2020.00016
Király, O., Potenza, M. N., Stein, D. J., King, D. L., Hodgins, D. C., Saunders, J. B., et al. (2020). Preventing problematic internet use during the COVID-19 pandemic: consensus guidance. Compr. Psychiatry 100. doi: 10.1016/j.comppsych.2020.152180
Ledgerwood, D. M., and Petry, N. M. (2006). Psychological experience of gambling and subtypes of pathological gamblers. Psychiatry Res. 144, 17–27. doi: 10.1016/j.psychres.2005.08.017
Lee, B. K., and Rovers, M. (2008). ‘“Bringing torn lives together again”’: Efects of the frst congruence couple therapy training application to clients in pathological gambling. Int. Gambl. Stud. 8, 113–129. doi: 10.1080/14459790701878137
Lesieur, H. R., and Blume, S. B. (1987). The south oaks gambling screen (SOGS): a new instrument for the identification of pathological gamblers. Am. J. Psychiatry 144, 1184–1188. doi: 10.1176/ajp.144.9.1184
Lindner, P., Forsström, D., Jonsson, J., Berman, A. H., and Carlbring, P. (2020). Transitioning between online gambling modalities and decrease in total gambling activity, but no indication of increase in problematic online gambling intensity during the first phase of the COVID-19 outbreak in Sweden: a Time Series Forecast Study. Front. Public Heal. 8:554542. doi: 10.3389/fpubh.2020.554542
Mallorquí-Bagué, N., Mestre-Bach, G., Lozano-Madrid, M., Fernandez-Aranda, F., Granero, R., Vintró-Alcazaz, C., et al. (2018). Trait impulsivity and cognitive domains involving impulsivity and compulsivity as predictors of gambling disorder treatment response. Addict. Behav. 89, 169–176. doi: 10.1016/j.addbeh.2018.07.006
Mallorquí-Bagué, N., Vintró-Alcaraz, C., Verdejo-García, A., Granero, R., Fernández-Aranda, F., Magaña, P., et al. (2019). Impulsivity and cognitive distortions in different clinical phenotypes of gambling disorder: profiles and longitudinal prediction of treatment outcomes. Eur. Psychiatry 61, 9–16. doi: 10.1016/j.eurpsy.2019.06.006
Marsden, J., Darke, S., Hall, W., Hickman, M., Holmes, J., Humphreys, K., et al. (2020). Mitigating and learning from the impact of COVID-19 infection on addictive disorders. Addiction 115, 1007–1010. doi: 10.1111/add.15080
Melville, K. M., Casey, L. M., and Kavanagh, D. J. (2007). Psychological treatment dropout among pathological gamblers. Clin. Psychol. Rev. 27, 944–958. doi: 10.1016/j.cpr.2007.02.004
Mercer, K. B., and Eastwood, J. D. (2010). Is boredom associated with problem gambling behaviour? It depends on what you mean by “boredom.”. Int. Gambl. Stud. 10, 91–104. doi: 10.1080/14459791003754414
Mestre-Bach, G., Blycker, G. R., and Potenza, M. N. (2020). Pornography use in the setting of the COVID-19 pandemic. J. Behav. Addict. 9, 181–183. doi: 10.1556/2006.2020.00015
Mestre-Bach, G., Steward, T., Granero, R., Fernández-Aranda, F., del Pino-Gutiérrez, A., Mallorquí-Bagué, N., et al. (2019). The predictive capacity of DSM-5 symptom severity and impulsivity on response to cognitive-behavioral therapy for gambling disorder: a 2-year longitudinal study. Eur. Psychiatry 55, 67–73. doi: 10.1016/j.eurpsy.2018.09.002
Milton, S., Crino, R., Hunt, C., and Prosser, E. (2002). The effect of compliance-improving interventions on the cognitive-behavioural treatment of pathological gambling. J. Gambl. Stud. 18, 207–229. doi: 10.1023/A:1015580800028
Olason, D. T., Hayer, T., Brosowski, T., and Meyer, G. (2015). Gambling in the mist of economic crisis: results from three national prevalence studies from Iceland. J. Gambl. Stud. 31, 759–774. doi: 10.1007/s10899-015-9523-4
Orlinsky, D. E., Grawe, K., and Parks, B. K. (1994). “Process and outcome in psychotherapy: Noch einmal,” in Handbook of Psychotherapy and Behavior Change. eds. A. E. Bergin and S. L. Garfield (John Wiley & Sons), 270–376.
Özdin, S., and Bayrak Özdin, Ş. (2020). Levels and predictors of anxiety, depression and health anxiety during COVID-19 pandemic in Turkish society: the importance of gender. Int. J. Soc. Psychiatry 66, 504–511. doi: 10.1177/0020764020927051
Price, A. (2020). Online gambling in the midst of COVID-19: a nexus of mental health concerns, substance use and financial stress. Int. J. Ment. Health Addict., 1–18. doi: 10.1007/s11469-020-00366-1
Rajkumar, R. P. (2020). COVID-19 and mental health: A review of the existing literature. Asian J. Psychiatr. 52:102066. doi: 10.1016/j.ajp.2020.102066
Robson, E., Edwards, J., Smith, G., and Colman, I. (2002). Gambling decisions: an early intervention program for problem gamblers. J. Gambl. Stud. 18, 235–255. doi: 10.1023/A:1016888705033
Salerno, L., and Pallanti, S. (2021). COVID-19 related distress in gambling disorder. Front. Psych. 12. doi: 10.3389/fpsyt.2021.620661
SBC News. (2020). Spain Order ‘Social Shield’ to Fast Track Gambling Advertising Window. Available at: https://sbcnews.co.uk/europe/2020/04/01/spain-orders-social-shield-to-fast-trackgambling-advertising-window/SBCNews
Smith, D., Harvey, P., Battersby, M., Pols, R., Oakes, J., and Baigent, M. (2010). Treatment outcomes and predictors of drop out for problem gamblers in South Australia: A cohort study. Aust. N. Z. J. Psychiatry 44, 911–920. doi: 10.3109/00048674.2010.493502
Stinchfield, R. (2003). Reliability, validity, and classification accuracy of a measure of DSM-IV diagnostic criteria for pathological gambling. Am. J. Psychiatry 160, 180–182. doi: 10.1176/appi.ajp.160.1.180
van Schalkwyk, M. C. I., Heetham, D., Reeves, A., and Petticreew, M. (2020). We must take urgent action to avoid an increase in problem gambling and gambling related harms. BMJ. Available at: https://blogs.bmj.com/bmj/2020/04/06/covid-19-we-must-take-urgent-action-to-avoid-an-increase-in-problem-gambling-and-gambling-related-harms
Wang, C., Pan, R., Wan, X., Tan, Y., Xu, L., Ho, C. S., et al. (2020). Immediate psychological responses and associated factors during the initial stage of the 2019 coronavirus disease (COVID-19) epidemic among the general population in China. Int. J. Environ. Res. Public Health 17. doi: 10.3390/ijerph17051729
World Health Organization (WHO). (2020). #HealthyAtHome – Mental Health. Available at: https://www.who.int/news-room/campaigns/connecting-the-world-to-combat-coronavirus/healthyathome/healthyathome—mental-health
Yuki, K., Fujiogi, M., and Koutsogiannaki, S. (2020). COVID-19 pathophysiology: A review. Clin. Immunol. 215:108427. doi: 10.1016/j.clim.2020.108427
Keywords: COVID-19, confinement, gambling disorder, dropout, coping
Citation: Baenas I, Etxandi M, Codina E, Granero R, Fernández-Aranda F, Gómez-Peña M, Moragas L, Rivas S, Potenza MN, Håkansson A, del Pino-Gutiérrez A, Mora-Maltas B, Valenciano-Mendoza E, Menchón JM and Jiménez-Murcia S (2021) Does Confinement Affect Treatment Dropout Rates in Patients With Gambling Disorder? A Nine-Month Observational Study. Front. Psychol. 12:761802. doi: 10.3389/fpsyg.2021.761802
Edited by:
Tobias Hayer, University of Bremen, GermanyReviewed by:
Davinia M. Resurrección, Universidad Loyola Andalucía, SpainJuan Francisco Rodríguez-Testal, Sevilla University, Spain
Copyright © 2021 Baenas, Etxandi, Codina, Granero, Fernández-Aranda, Gómez-Peña, Moragas, Rivas, Potenza, Håkansson, del Pino-Gutiérrez, Mora-Maltas, Valenciano-Mendoza, Menchón and Jiménez-Murcia. This is an open-access article distributed under the terms of the Creative Commons Attribution License (CC BY). The use, distribution or reproduction in other forums is permitted, provided the original author(s) and the copyright owner(s) are credited and that the original publication in this journal is cited, in accordance with accepted academic practice. No use, distribution or reproduction is permitted which does not comply with these terms.
*Correspondence: Susana Jiménez-Murcia, sjimenez@bellvitgehospital.cat