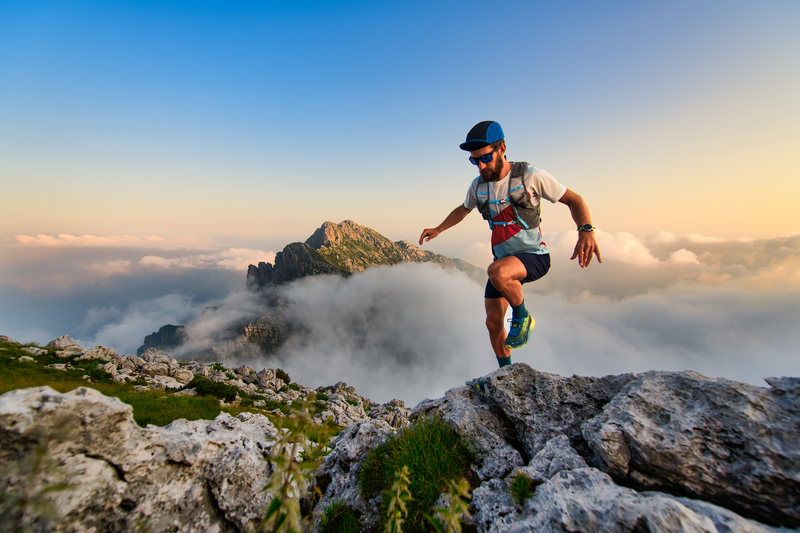
95% of researchers rate our articles as excellent or good
Learn more about the work of our research integrity team to safeguard the quality of each article we publish.
Find out more
ORIGINAL RESEARCH article
Front. Psychol. , 04 May 2021
Sec. Psychopathology
Volume 12 - 2021 | https://doi.org/10.3389/fpsyg.2021.676763
This article is part of the Research Topic Insomnia: A Heterogenic Disorder Often Comorbid With Other Disorders and Diseases View all 9 articles
Objective: Co-morbid insomnia and sleep apnea (COMISA) is a common and debilitating condition that is more difficult to treat compared to insomnia or sleep apnea-alone. Emerging evidence suggests that cognitive behavioral therapy for insomnia (CBTi) is effective in patients with COMISA, however, those with more severe sleep apnea and evidence of greater objective sleep disturbance may be less responsive to CBTi. Polysomnographic sleep study data has been used to predict treatment response to CBTi in patients with insomnia-alone, but not in patients with COMISA. We used randomized controlled trial data to investigate polysomnographic predictors of insomnia improvement following CBTi, versus control in participants with COMISA.
Methods: One hundred and forty five participants with insomnia (ICSD-3) and sleep apnea [apnea-hypopnea index (AHI) ≥ 15] were randomized to CBTi (n = 72) or no-treatment control (n = 73). Mixed models were used to investigate the effect of pre-treatment AHI, sleep duration, and other traditional (AASM sleep macrostructure), and novel [quantitative electroencephalography (qEEG)] polysomnographic predictors of between-group changes in Insomnia Severity Index (ISI) scores from pre-treatment to post-treatment.
Results: Compared to control, CBTi was associated with greater ISI improvement among participants with; higher AHI (interaction p = 0.011), less wake after sleep onset (interaction p = 0.045), and less N3 sleep (interaction p = 0.005). No quantitative electroencephalographic, or other traditional polysomnographic variables predicted between-group ISI change (all p > 0.09).
Discussion: Among participants with COMISA, higher OSA severity predicted a greater treatment-response to CBTi, versus control. People with COMISA should be treated with CBTi, which is effective even in the presence of severe OSA and objective sleep disturbance.
Chronic insomnia and obstructive sleep apnea (OSA) are the two most common sleep disorders and frequently co-occur within the same patient (The American Academy of Sleep Medicine, 2014; Sweetman et al., 2017a). Chronic insomnia is characterized by frequent difficulties initiating sleep, maintaining sleep, and/or early morning awakenings from sleep, and associated daytime functional impairments, which persist for at least 3 months (The American Academy of Sleep Medicine, 2014). OSA is characterized by frequent narrowing (hypopnea) or collapse (apnea) of the upper airway during sleep, resulting in reduced oxygen saturation, frequent cortical arousals, and daytime sleepiness and fatigue. The clinical index of OSA is the apnea-hypopnea index (AHI) which reflects the average number of respiratory events per hour of sleep. Moderate and severe OSA are commonly defined by an AHI of 15–29, and ≥30 events/h of sleep, respectively. Insomnia and OSA both increase risk of mental health problems (Peppard et al., 2006; Baglioni et al., 2011), and result in substantial societal costs through reduced productivity and quality of life, and high healthcare utilization (Ozminkowski et al., 2007; Wickwire et al., 2016; Natsky et al., 2020).
Co-morbid insomnia and sleep apnea (COMISA) is a common and debilitating condition (Luyster et al., 2010; Sweetman et al., 2017a, 2019a). For example, approximately 30–50% of patients with OSA report clinically significant insomnia symptoms, while 30–40% of patients with insomnia fulfill diagnostic criteria for OSA when assessed via overnight polysomnography (Zhang et al., 2019; Sweetman et al., 2019a). Compared to patients with insomnia-alone, or OSA-alone, patients with COMISA experience greater impairment to sleep (Bianchi et al., 2013), mood (Lang et al., 2017), daytime functioning (Krakow et al., 2001; Smith et al., 2004; Sweetman et al., 2017b), and quality of life (Sweetman et al., 2017a, 2019a; Tasbakan et al., 2018).
Despite the high prevalence and morbidity associated with COMISA, the most effective treatment approach is unknown. Continuous positive airway pressure (CPAP) therapy is the first-line treatment for moderate and severe OSA (Epstein et al., 2009). However, patients with COMISA show lower initial acceptance and long-term use of CPAP, compared to patients with OSA alone (Suraiya and Lavie, 2006; Wickwire et al., 2010; Pieh et al., 2012; Sweetman et al., 2017a; Philip et al., 2018). On the other hand, cognitive behavioral therapy for insomnia (CBTi) is the recommended “first-line” treatment for insomnia (Schutte-Rodin et al., 2008; Qaseem et al., 2016). Although CBTi leads to a significant overall reduction in insomnia symptoms among patients with COMISA (Fung et al., 2014; Sweetman et al., 2017b, 2019b; Alessi et al., 2020; Ong et al., 2020), the efficacy of CBTi is variable between patients, with up to 65% of COMISA patients failing to achieve remission of insomnia by post-CBTi (Sweetman et al., 2017b). Therefore, it is important to identify which patients with COMISA are likely to respond to CBTi, to guide tailored treatment approaches (Sweetman et al., 2017a).
Polysomnographic sleep study data have previously been used to predict response to CBTi in patients with insomnia (Bathgate et al., 2017; Miller et al., 2018; Kalmbach et al., 2020). For example, studies have reported that shorter sleep duration (Bathgate et al., 2017), lower objective sleep efficiency (Kalmbach et al., 2020; percentage of time in bed spent asleep), shorter sleep onset latency (Troxel et al., 2013), specific quantitative electroencephalography (qEEG) parameters (Krystal and Edinger, 2010), and co-morbid OSA (Sweetman et al., 2017b) are associated with a reduced response to CBTi.
In the context of COMISA, it is possible that patients who have more severe OSA (higher AHI), worse sleep macrostructure (less sleep time, sleep efficiency), or lighter and more fragmented sleep (more light sleep, higher arousal index, and modified sleep microstructure) before treatment may be less responsive to CBTi. This information is critical to guide the most appropriate treatment approach for patients with COMISA. For example, if patients with insomnia symptoms and severe OSA are minimally responsive to CBTi, it may be possible to expedite CPAP therapy to manage the OSA, before commencing CBTi. Alternatively, if CBTi is found to be effective in the presence of more severe OSA, it may be possible to treat such patients with CBTi to improve insomnia and potentially increase use of CPAP therapy (Sweetman et al., 2019b; Alessi et al., 2020; Ong et al., 2020). Therefore, we aimed to use randomized controlled trial (RCT) data to investigate the effect of pre-treatment polysomnographic sleep and respiratory data on insomnia improvements following CBTi, versus control in participants with COMISA.
This study used data from a previously reported RCT investigating the effect of a 4-session CBTi program, versus no-treatment control, on subsequent acceptance and use of CPAP therapy in participants with COMISA (Sweetman et al., 2019b). As reported previously (Sweetman et al., 2019b), the CBTi group reported a greater average reduction in the ISI from pre-treatment to post-treatment, compared to the control group. This exploratory study aimed to investigate whether any polysomnographic data before treatment predicted change in insomnia symptoms from pre-treatment to post-treatment, in the CBTi group, versus the control group. Mixed model analyses were used to investigate the interaction effect between pre-treatment polysomnographic predictors, group (CBTi, control) and time (pre-treatment, post-treatment) on self-reported insomnia symptoms. No participants were using CPAP therapy during this study phase. Participants with previous CPAP use were not excluded, provided that CPAP had not been used for at least 3 months before the pre-treatment assessment. 10 of 72 participants in the CBTi group and 8 of 73 participants in the control group reported previous CPAP use (Fisher’s exact p = 0.624).
Participant screening and recruitment have been described previously (Sweetman et al., 2019b), and are illustrated in Figure 1. Briefly, two Australian outpatient sleep clinics screened 2,870 patients, of who 145 people with untreated insomnia [Psychologist diagnosis; ICSD-3 criteria (The American Academy of Sleep Medicine, 2014)] and OSA (Sleep physician diagnosis; AHI ≥ 15 according to full overnight sleep study) were randomized between November 2013 and April 2016.
Figure 1. Flow diagram indicating participant screening, randomization and follow-up. ISI, insomnia severity index; OSA50, obstructive sleep apnea 50 questionnaire; AHI, apnea–hypopnea index/h; and CPAP, continuous positive airway pressure.
Following completion of pre-treatment questionnaires, sleep diaries, and overnight polysomnography, participants were randomly allocated to a CBTi group or to a control group. A manualized CBTi program including four 45-min sessions was administered by registered or provisional psychologists trained in administration of this CBTi intervention.
Session 1 included sleep education (two-process model of sleep regulation), and bedtime restriction therapy. Session 2 included sleep misperception feedback (Polysomnography versus sleep diary parameters). Session 3 included information about insomnia, hyper-arousal, and cognitive therapy. Session 4 was used to review the previous weeks of therapy and for relapse prevention. Participants completed sleep diaries and an Epworth Sleepiness Scale during each session. CBTi did not include any information about OSA or CPAP therapy.
Adequate treatment fidelity and effectiveness of CBTi, versus control has been reported previously (Sweetman et al., 2019b). Participants randomized to the control condition received no treatment between pre-treatment and post-treatment.
Participants completed a single home-based diagnostic polysomnographic study before randomization (Somté, Compumedics, Australia). Participants attended sleep laboratories on the evening of sleep studies to be set up with recording equipment by trained technicians. Polysomnography studies included electrode placement to record two electroencephalographic channels (C3–M2, C4–M1), two chin-electromyogram channels, two electrooculogram channels, two leg-electromyogram channels, a nasal cannula to record nasal pressure, a oro-nasal thermistor, a finger pulse oximeter, and chest and abdominal bands to record respiratory effort. As participants were free to choose their time in bed parameters in their home environment, there was no systematic restriction of time in bed on the night of the polysomnography study. Studies were scored by trained technicians according to AASM 2007 “alternate” criteria (Iber et al., 2007; Ruehland et al., 2009). Three main categories of polysomnographic predictors were investigated. Firstly, sleep apnea severity predictors included; AHI, arousal index, and nadir oxygen saturation. Secondly, we examined traditional AASM sleep parameters and sleep architecture predictors including; total sleep time, sleep efficiency, sleep onset latency, wake after sleep onset, and total sleep time in each stage (N1–3, REM).
Finally, we examined qEEG predictors, including absolute and relative non-rapid eye movement sleep and rapid eye movement sleep beta, alpha, theta, and delta power, and N2 K-complex density. Briefly, absolute power of the beta, alpha, theta, and delta power was calculated in 5-s non-overlapping EEG segments using multi-taper based (Prerau et al., 2017) fast Fourier transform as reported previously (Scott et al., 2020). The mean absolute power in NREM epochs was then calculated for each frequency band. Epochs with an absolute maximum amplitude of 400 μv were considered noisy. Our choice of rejection threshold for noisy epochs is inevitably somewhat arbitrary, but was chosen to exceed the largest slow wave EEG events of interest during sleep; which have a negative maximum amplitude of around 200 to 300 μv (Massimini et al., 2004). We have previously reproduced more complex noise rejection algorithms (D’Rozario et al., 2015). However, the difference between the current threshold (maximum amplitude of 400 μv) and these more complex algorithms was very small (around 4 min over the full night). Thus, we opted for the simpler threshold (max 400 μv) for this study since it is more easily implemented for others to reproduce. K-complexes were scored according to previously published method (Lechat et al., 2020) and K-complex density was defined as the number of K-complexes occurrence divided by the time spent in N2 sleep. Although no frontal electrodes were included in our PSG montage, this K-complex density algorithm has previously been validated based on C3 electrode placement that was used in the current study.
All polysomnographic variables were retained as continuous predictors to preserve information that is otherwise lost by splitting continuous data into two dichotomous groups (Altman and Royston, 2006). However, as a sensitivity analysis, and to inform clinical utility of the effect of OSA severity on treatment-response, OSA severity was also investigated by dividing participants into two groups reflecting standard clinical cut-offs for moderate (AHI < 30) versus severe OSA (AHI ≥ 30). Similarly, polysomnographic sleep duration was also investigated by dividing participants into those with short (<6 h), versus normal sleep duration (≥6 h) according to previously established categories (Bathgate et al., 2017).
The Insomnia Severity Index (ISI) was the primary outcome measure. The ISI is a 7-item self-report measure of insomnia severity which has been used extensively in insomnia-treatment research (Bastien et al., 2001). Scores range from 0 to 28, with higher scores indicating more severe insomnia symptoms. A reduction of ≥8 points was used to define “responders” (Sweetman et al., 2019b), and a post-treatment threshold of ≥15 was used to indicate “clinically significant insomnia” (Bastien et al., 2001; Morin et al., 2011; Gagnon et al., 2013).
Data were analyzed with IBM SPSS (Version 25) on an intention-to-treat basis. Mixed models were used to examine interaction effects between pre-treatment polysomnographic predictors, group (CBTi, control) and time (pre-treatment, post-treatment) on the ISI. Binary logistic regression was used to investigate the effect of pre-treatment polysomnographic predictors on rates of “clinically significant insomnia” by post-treatment. A small amount of missing data and patterns of missing data have been reported previously (two participants in the CBTi group and one participant in the control group withdrew before post-treatment; Figure 1; Sweetman et al., 2019b). Descriptive statistics are reported with standard deviations (pre-treatment data), and 95% confidence intervals (CIs; change data) and an alpha significance level of <0.05 was used.
Data were extracted for qEEG analysis from both electroencephalogram channels (C3–M2, C4–M1). These were initially retained as independent predictors (e.g., EEG1 beta power, EEG2 beta power, etc.). As no significant predictors were observed when analyzing qEEG power of separate channels, the average qEEG power of both channels was calculated and reported in the analyses below.
Participants included 145 people with COMISA (Age M = 58.2, sd = 9.9; 55% male). There were no between-group differences in any socio-demographic, questionnaire, or polysomnographic variables at pre-treatment, including the proportion of participants with severe OSA (AHI ≥ 30), or the proportion of participants with normal sleep duration (sleep duration ≥6 h; see Table 1).
As reported previously (Sweetman et al., 2019b), the CBTi group experienced a greater ISI reduction from pre-treatment (M = 18.5, ±95%CI = 1.3) to post-treatment (M = 12.2, ±95%CI = 1.4) compared to the control group (pre-treatment M = 18.0, ±95%CI = 1.3; post-treatment M = 16.6, ±95%CI = 1.4; and interaction p < 0.001). This pattern of results was similar when investigating each polysomnography predictor of treatment response (below). Therefore, the two-way (group by time) analyses are not reported for each model.
Interaction effects between continuous polysomnography variables, group and time on the ISI are displayed in Table 2. There was a three-way interaction effect between pre-treatment AHI (continuous), group (CBTi, versus control), and time (pre-treatment, versus post-treatment) on the ISI [Figure 2; F(1,134) = 6.7, interaction p = 0.011]. Higher pre-treatment AHI was associated a greater reduction of insomnia severity in the CBTi group, but a smaller reduction of insomnia severity in the control group from pre-treatment to post-treatment. Put differently, among participants with more severe OSA (e.g., AHI = 60, solid darker lines; Figure 2), control participants experienced very little ISI reduction, while CBTi participants experienced a large ISI reduction by post-treatment. However, among participants with less severe OSA (e.g., AHI = 15, dashed lines; Figure 2), there was less difference in reduction of ISI between the CBTi and control group by post-treatment. As pre-treatment AHI was retained as a continuous predictor in this model, these AHI values were chosen for illustrative purposes (Figure 2).
Table 2. Effect of continuous polysomnography parameters on ISI change during CBTi, versus control, represented as beta coefficient (±95%CI).
Figure 2. Modeled data illustrating the effect of pre-treatment AHI, group (CBTi, control), and time (pre-treatment, post-treatment) on the Insomnia Severity Index (95%CI). Pre-treatment AHI was retained as a continuous predictor and data are plotted for pre-treatment AHI values of 15 and 60 for each group according to model intercept, slopes and interaction effects. Compared to control, higher pre-treatment AHI was associated with greater insomnia severity reduction during CBTi.
This effect was supported in a sensitivity analysis of the interaction between AHI severity level (<30, versus ≥30), group, and time on the ISI (interaction p = 0.028; Supplementary Figure 1). Among participants with severe OSA, those in the control group showed no reduction of ISI by post-treatment (M reduction = 0.25, ±95%CI = 1.7, p = 0.77, and Cohen’s d = 0.05), while those in the CBTi group showed a large ISI reduction (M reduction = 7.0, ±95%CI = 1.7, p < 0.001, and Cohen’s d = 1.4). Among participants with moderate OSA, significant ISI reduction by post-treatment was observed in both the control (M reduction = 2.6, ±95%CI = 1.7, p = 0.002, and Cohen’s d = 0.5) and CBTi groups (M reduction = 5.7, ±95%CI = 1.6, p < 0.001, and Cohen’s d = 1.1). There was no effect of pre-treatment arousal index [continuous; F(1,133) = 2.4, interaction p = 0.121], or nadir oxygen saturation [continuous; F(1,133) <0.1, interaction p = 0.943] on between-group change in the ISI by post-treatment.
When examining only participants in the CBTi group, there were no interaction effects of pre-treatment AHI [F(1,67) = 1.5, interaction p = 0.229], arousal index [F(1,67) = 0.2, interaction p = 0.626], or nadir oxygen saturation [F(1,67) = 0.1, interaction p = 0.754] (all retained as continuous predictors) on change in the ISI by post-treatment.
Several three-way interactions between group, time, and polysomnography sleep parameters (retained as continuous variables) on ISI were performed. Compared to control, greater ISI reduction in the CBTi group was predicted by less objective wake after sleep onset [F(1,134) = 4.1, interaction p = 0.045; Figure 3], and less time spent in N3 sleep [F(1,135) = 8.2, interaction p = 0.005; Figure 4]. There was no effect of pre-treatment polysomnographic total sleep time [F(1,133) = 2.8, interaction p = 0.098; Figure 5], sleep efficiency [F(1,137) <0.1, interaction p = 0.881], sleep onset latency [F(1,145) = 0.5, interaction p = 0.488], or time spent in N1 [F(1,133) <0.1, interaction p = 0.840], N2 [F(1,134) <0.1, interaction p = 0.811], or REM sleep [F(1,136) = 3.7, interaction p = 0.056; see Supplementary Figure 2] on reduction of ISI scores during treatment between the CBTi versus control group.
Figure 3. Modeled data illustrating the effect of pre-treatment wake after sleep onset (WASO; min), group (CBTi, control), and time (pre-treatment, post-treatment) on the Insomnia Severity Index (CI). Pre-treatment WASO was retained as a continuous predictor and data are plotted according to intercept, slopes and interaction effects. Compared to control, lower pre-treatment WASO predicted greater insomnia severity reduction in the CBTi group.
Figure 4. Modeled data illustrating the effect of pre-treatment N3 sleep (min), group (CBTi, control), and time (pre-treatment, post-treatment) on the Insomnia Severity Index (CI). Pre-treatment N3 sleep was retained as a continuous predictor and data are plotted according to intercept, slopes and interaction effects. Compared to control, less N3 sleep time predicted greater insomnia severity reduction in the CBTi group.
Figure 5. Modeled data illustrating the effect of pre-treatment total sleep time, group (CBTi, control), and time (pre-treatment, post-treatment) on the Insomnia Severity Index (95%CI; not statistically significant). Pre-treatment sleep time was retained as a continuous predictor and data are plotted according to intercept, slopes and interaction effects.
When total sleep time was dichotomized into short (<6 h) versus normal (≥6 h) sleep duration, there was no three-way interaction effect of sleep duration group, intervention-group and time on the ISI (p = 0.341). Indeed, among participants in the control group, there was no significant ISI reduction by post-treatment in the short (M reduction = 1.38, 95%CI = 1.7, p = 0.11, and Cohen’s d = 0.3) or normal sleep duration groups (M reduction = 1.49, 95%CI = 1.7, p = 0.08, and Cohen’s d = 0.3). Among participants in the CBTi group, there was a significant ISI reduction by post-treatment in both the short (M reduction = 7.30, 95%CI = 1.9, p < 0.001, and Cohen’s d = 1.4) and normal sleep duration groups (M reduction = 5.76, 95%CI = 1.7, p < 0.001, and Cohen’s d = 1.1).
When analyses were restricted to only participants in the CBTi group, there was a significant interaction effect between polysomnographic total sleep duration (continuous) and time [F(1,67) = 4.1, interaction p = 0.047; see Figure 5], and sleep onset latency and time (interaction p = 0.012) on the ISI. Shorter sleep duration (see slopes for CBTi group in Figure 5) and longer sleep onset latency before treatment (i.e., greater sleep impairment) were associated with a greater reduction of ISI scores from pre-treatment to post-treatment. When sleep duration was dichotomized into short (<6 h) versus normal sleep duration (≥6 h), the interaction between sleep duration and time on ISI among participants in the CBTi group was no longer significant (p = 0.251). There was no effect of continuous polysomnographic wake after sleep onset (interaction p = 0.435), sleep efficiency (interaction p = 0.174), or time spent in any sleep stage (N1–3, REM; all interaction p ≥ 0.11) on reduction of ISI scores during treatment in the CBTi group.
Finally, qEEG predictors of treatment-response to CBTi were also investigated in a series of exploratory analyses. There was no effect of pre-treatment absolute or ratio (relative to power across other bands) non-rapid eye movement sleep beta, alpha, theta, or delta power, or N2 K-complex density on reduction of ISI scores between groups (all retained as continuous predictors; all p > 0.09). Similarly, there was no effect of rapid eye movement sleep beta, alpha, theta, or delta power on reduction of ISI scores between groups (all retained as continuous predictors; all p > 0.30).
When analyses were restricted to participants in the CBTi group, there were no significant interaction effects between any non-rapid eye movement or rapid eye movement sleep absolute qEEG predictors and time on ISI scores (all retained as continuous predictors; all p ≥ 0.10).
Among participants in the CBTi group, binary logistic regression was used to investigate the association of pre-treatment polysomnographic data with rates of insomnia response (≥8 point reduction by post-treatment), and “clinically significant insomnia” (ISI ≥ 15) by post-treatment.
Participants in the CBTi group reported lower rates of “clinically significant insomnia” at post-treatment (34.8%), compared to participants in the control group (65.2%; χ2 = 12.5, p < 0.001). There was a statistically significant, albeit small, association between pre-treatment non-rapid eye movement sleep absolute beta power and “clinically significant insomnia” classification at post-treatment (exponentiated B = 0.99; p = 0.048). No other pre-treatment polysomnographic variables were associated with rates of clinically significant insomnia by post-treatment (all p ≥ 0.13). Among those in the CBTi group, there was no difference in rates of clinically significant insomnia between participants with moderate (40%) versus severe OSA (29.4%) by post-treatment (χ2 = 0.85, p = 0.11).
Participants in the CBTi group were more likely to show a positive insomnia response from pre-treatment to post-treatment (ISI reduction ≥ 8; 46.4%), compared to participants in the control group (6.1%; χ2 = 28.0, p < 0.001). There was a statistically significant, albeit small, association between pre-treatment sleep efficiency and rates of insomnia response in the CBTi group (exponentiated B = 0.96, p = 0.046). No other polysomnography variables before treatment were associated with rates of insomnia response in the CBTi group (all p > 0.05). Among those in the CBTi group, there was no difference in rates of “insomnia response” between participants with moderate (37.1%) versus severe OSA (55.9%) by post-treatment (χ2 = 2.4, p = 0.12).
The main finding of this study was that among participants with COMISA, we found that more severe OSA before treatment was associated with a greater reduction of insomnia severity during CBTi, versus control. Polysomnographic sleep duration, other measures of sleep fragmentation (arousal index, time spent in light sleep), and qEEG sleep parameters before treatment did not predict a reduced response to CBTi, versus control. This suggests that CBTi is effective in the presence of severe OSA, and in participants who commence treatment with greater objective sleep disturbance.
Although it was hypothesized that participants with COMISA who have more severe OSA and evidence of greater objective sleep disturbance may be less responsive to CBTi, these data indicate that CBTi is an effective treatment among such participants. Theoretically, among patients with more severe OSA, clinicians may conceptualize the insomnia as a secondary manifestation of the untreated OSA. Indeed, respiratory events and sleep fragmentation may result in awakenings that precipitate insomnia symptoms in some patients with OSA. Previous studies have also demonstrated that CPAP therapy improves insomnia symptoms among some COMISA patients, including those with more severe OSA (Björnsdóttir et al., 2013; Glidewell et al., 2014). However, the present data indicate that greater OSA severity does not predict a reduced response to CBTi among people with both disorders.
In fact, participants with more severe OSA a reported greater reduction of insomnia severity following CBTi, versus control. This three-way interaction effect was largely due to variability in changes in insomnia severity over time in the control group, while the CBTi group showed more homogenous improvement of insomnia across different OSA severity levels (Figure 2). Among control participants, this variable effect of pre-treatment AHI on reduction of insomnia may result from a natural reduction of insomnia severity over time or gradual regression to the mean among participants with less severe OSA, while participants with more severe OSA experienced more stable insomnia severity between pre-treatment and post-treatment. Given that un-treated insomnia symptoms showed little natural variation over time among participants with more severe OSA, it is recommended that clinicians consider referring such patients for CBTi to improve insomnia and potentially increase subsequent CPAP use (Sweetman et al.,2019a,b).
Previous studies have investigated the effect of short objective sleep duration (i.e., total sleep time < 6 h) and objective sleep efficiency on treatment-response to CBTi in patients with insomnia (Bathgate et al., 2017; Galbiati et al., 2019; Kalmbach et al., 2020). It has been postulated that insomnia patients with short objective sleep duration may experience an insomnia phenotype characterized by underlying biological mechanisms, rather than psychological/behavioral mechanisms that may be less responsive to CBTi (Vgontzas et al., 2013). In the current COMISA sample, polysomnographic sleep duration and sleep efficiency did not predict response to CBTi, versus control. When analyses were restricted to only participants in the CBTi group, short sleep duration and longer sleep onset latency predicted greater ISI reduction during CBTi (e.g., see CBTi group in Figure 5). Although these findings potentially indicate a greater response to CBTi among participants with greater objective sleep impairment before treatment, these interaction effects were no longer statistically significant when comparing with changes across time in the control group. It is likely that the underlying mechanisms proposed to underpin the short sleep duration phenotype differ between people with insomnia-alone and those with COMISA (Bianchi et al., 2013; Vgontzas et al., 2013). For example, among COMISA participants, it is possible that sleep duration is partially dependent on frequent respiratory events causing arousals, and awakenings, in addition to physiological arousal processes. The “short sleep duration” insomnia phenotype requires further investigation in the context of COMISA.
We observed that participants with less objective wake after sleep onset, and less time spent in N3 sleep experienced a greater reduction of insomnia severity following CBTi, versus control. Similar to the three-way interaction of AHI, group and time on insomnia severity, these interaction effects also largely resulted from variability in changes in insomnia severity in the control group, but homogenous reduction in the CBTi group. Finally, we conducted exploratory analyses to investigate if any novel qEEG parameters predicted treatment-response to CBTi, but observed no notable or statistically significant predictors.
Although this study had a number of strengths including the use of standardized measures and interventions, and only a small amount of missing data (Sweetman et al., 2019b), there are important limitations that warrant consideration. Firstly, this was a secondary analysis of existing RCT data, which was not specifically powered to identify polysomnographic predictors of treatment-response to CBTi. However, as previous studies with smaller sample sizes have detected statistically significant effects of objective sleep duration (Bathgate et al., 2017), sleep efficiency (Kalmbach et al., 2020), sleep onset latency (Troxel et al., 2013), and qEEG predictors (Krystal and Edinger, 2010) on response to CBTi in patients with insomnia, it is unlikely that our exploratory study was under-powered to detect an effect of these specific polysomnographic predictors on treatment-response.
Secondly, only a single night of polysomnographic data was collected before treatment, which may have been impacted by “first night effects,” or night-to-night variability in objective sleep and respiratory parameters. Given the high costs associated with polysomnography, it may be possible for future studies to utilize novel and less expensive measures of objective sleep such as wearable wrist-worn or electroencephalography devices (e.g., actigraphy, portable EEG monitoring devices) capable of measuring sleep over several consecutive nights to produce more stable estimates of key sleep parameters (Arnal et al., 2020).
Finally, the generalizability of this study is restricted to participants with at least moderate OSA (AHI ≥ 15). As this study did not include insomnia participants with no OSA or mild OSA, it was not possible to determine whether the presence/absence of sleep apnea (or mild severity OSA) is predictive of treatment response to CBTi. A previous study investigating the effectiveness of CBTi between patients with insomnia-alone and COMISA (mild-severe OSA) reported that although both groups showed significant improvement of insomnia during CBTi, those with COMISA reported a slightly smaller response to CBTi by 3-month follow-up (Sweetman et al., 2017b). Instead, the present study targeted an important clinical question related to the most effective treatment approach in patients with both chronic insomnia and moderate/severe OSA.
Emerging evidence suggests that CBTi may be an effective (Sweetman et al., 2019b; Alessi et al., 2020; Ong et al., 2020) and safe (Sweetman et al., 2020) overall treatment for COMISA, however, it is important to identify which patients are most responsive to CBTi, and which patients are less responsive to CBTi and require other initial treatments (e.g., CPAP). Contrary to our hypothesis, this study found that higher OSA severity before treatment was associated with a greater insomnia improvement following CBTi, compared with control. Furthermore, objective short sleep duration, and qEEG parameters did not predict a reduced response to CBTi, versus control in participants with COMISA. Compared to control, CBTi was associated with greater ISI improvement among participants with less objective wake after sleep onset, and less N3 sleep before treatment. This suggests that people with COMISA should be referred for CBTi, regardless of OSA severity or objective sleep disturbance before treatment.
The datasets presented in this article are not readily available because participants did not provide informed consent for sharing of trial data.
The studies involving human participants were reviewed and approved by the Southern Adelaide Clinical Human Research Ethics Committee (Southern Adelaide Local Health Network, Adelaide, Australia), the Human Research Ethics Committee (The Prince Charles Hospital, Brisbane, Australia), the Queensland University of Technology Human Research Ethics Committee, and the External Request Evaluation Committee (Services Australia). The patients/participants provided their written informed consent to participate in this study.
AS: study design, data collection, management, and manuscript writing and analysis. BL: extraction of qEEG data and manuscript drafting. PC: study design, management, and manuscript drafting. SS: study design, management, and manuscript drafting. NA: study design and management. AO’G: data collection and management. ND: data collection and management. RDMc: study design, management, and manuscript drafting. LL: study design, management, and manuscript drafting. All authors have approved this manuscript.
This research was funded by an Australian Government National Health and Medical Research Council grant (1049591; Treating insomnia co-morbid with obstructive sleep apnoea: A randomized controlled clinical effectiveness trial).
RDMc reports research funding support from Philips Respironics and research equipment donations from ResMed and Air Liquide. PC received salary support via an Australian Research Council Future Fellowship (FT120100510), reports research funding support from Philips Respironics via the CRC for Alertness, Safety and Productivity, and equipment support from Philips Respironics and Air Liquide. LL has received funding support from Re-timer (Re-timer Pty Ltd, Adelaide, Australia).
The remaining authors declare that the research was conducted in the absence of any commercial or financial relationships that could be construed as a potential conflict of interest.
The authors would like to acknowledge the following people for their contributions to this study. Jan Robinson, Denzil Paul, Assoc. Prof. Ching Li Chai-Coetzer, and Dr. James Douglas for oversight and management of study, and data collection. Dr. Ashliegh Perry, Dr. Neralie Cain, Melissa Wilson, Dr. Sara Winter, and Dr. Lynette Buller for delivering the CBTi sessions, Laura Bandick, Michaela O’Keefe, Hiro Tojo, and Sharn Rowland for administering CPAP education and setups, Dr. Emer Van Ryswyk, Cassandra Pattinson, and Dr. Alicia Allan for managing recruitment and home studies, Tim Jarryd, Alistair Edwards, Henry Scown, Hayden Ng, Dr. Gorica Micic, Dr. Kalina Rossa, Dr. Sherrie-Anne Kaye, and Dr. Luisa Roeder for assistance with sleep studies, Carl Downey for scoring sleep studies, Air Liquide for supplying CPAP equipment and assisting with CPAP setups, and the NHMRC for funding this research.
The Supplementary Material for this article can be found online at: https://www.frontiersin.org/articles/10.3389/fpsyg.2021.676763/full#supplementary-material
Alessi, C. A., Fung, C. H., Dzierzewski, J. M., Fiorentino, L., Stepnowsky, C., Rodriguez Tapia, J. C., et al. (2020). Randomized controlled trial of an integrated approach to treating insomnia and improving use of positive airway pressure therapy in veterans with comorbid insomnia disorder and obstructive sleep apnea. Sleep 44:zsaa235.
Altman, D. G., and Royston, P. (2006). The cost of dichotomising continuous variables. BMJ 332:1080.
Arnal, P. J., Thorey, V., Debellemaniere, E., Ballard, M. E., Bou Hernandez, A., Guillot, A., et al. (2020). The dreem headband compared to polysomnography for EEG signal acquisition and sleep staging. Sleep 43:zsaa097.
Baglioni, C., Battagliese, G., Feige, B., Spiegelhalder, K., Nissen, C., Voderholzer, U., et al. (2011). Insomnia as a predictor of depression: a meta-analytic evaluation of longitudinal epidemiological studies. J. Affect. Disord. 135, 10–19. doi: 10.1016/j.jad.2011.01.011
Bastien, C. H., Vallières, A., and Morin, C. M. (2001). Validation of the insomnia severity index as an outcome measure for insomnia research. Sleep Med. 2, 297–307. doi: 10.1016/s1389-9457(00)00065-4
Bathgate, C. J., Edinger, J. D., and Krystal, A. D. (2017). Insomnia patients with objective short sleep duration have a blunted response to cognitive behavioral therapy for insomnia. Sleep 40:zsw012.
Bianchi, M., Williams, K. L., McKinney, S., and Ellenbogen, J. M. (2013). The subjective-objective mismatch in sleep perception among those with insomnia and sleep apnea. J. Sleep Res. 22, 557–568. doi: 10.1111/jsr.12046
Björnsdóttir, E., Janson, C., Sigurdsson, J. F., Gehrman, P., Perlis, M., Juliusson, S., et al. (2013). Symptoms of insomnia among patients with obstructive sleep apnea before and after two years of postivie airway pressure treatment. Sleep 36, 1901–1909. doi: 10.5665/sleep.3226
D’Rozario, A. L., Dungan, G. C., Banks, S., Liu, P. Y., Wong, K. K., Killick, R., et al. (2015). An automated algorithm to identify and reject artefacts for quantitative EEG analysis during sleep in patients with sleep-disordered breathing. Sleep Breath. 19, 607–615. doi: 10.1007/s11325-014-1056-z
Epstein, L. J., Kristo, D., Strollo, P. J., Friedman, N., Malhotra, A., Patil, S. P., et al. (2009). Clinical guideline for the evaluation, management and long-term care of obstructive sleep apnea in adults: adult obstructive sleep apnea task force of the American Academy of Sleep Medicine. J. Clin. Sleep Med. 5, 263–276. doi: 10.5664/jcsm.27497
Fung, C. H., Martin, J. L., Dzierzewski, J. M., Josephson, K., Jouldjian, S., Rodriguez Tapia, J., et al. (2014). Untreated mild sleep disordered breathing does not reduce the efficacy of cognitive behaivoral therapy for insomnia in older adults. Sleep 37:A203.
Gagnon, C., Bélanger, L., Ivers, H., and Morin, C. M. (2013). Validation of the insomnia severity index in primary care. J. Am. Board Fam. Med. 26, 701–710. doi: 10.3122/jabfm.2013.06.130064
Galbiati, A., Sforza, M., Fasiello, E., Castronovo, V., and Ferini-Strambi, L. (2019). Impact of phenotypic heterogeneity of insomnia on the patients’ response to cognitive-behavioral therapy for insomnia: current perspectives. Nat. Sci. Sleep 11:367. doi: 10.2147/nss.s198812
Glidewell, R. N., Renn, B. N., Roby, E., and Orr, W. C. (2014). Predictors and patterns of insomnia symptoms in OSA before and after PAP therapy. Sleep Med. 15, 899–905. doi: 10.1016/j.sleep.2014.05.001
Iber, C., Ancoli-Israel, S., Chesson, A., and Quan, S. (2007). The AASM Manual for the Scoring of Sleep and Associated Events: Rules, Terminology and Technical Specification. Westchester, IL: American Academy of Sleep Medicine.
Kalmbach, D., Cheng, P., Roth, T., Sagong, C., and Drake, C. (2020). Objective sleep disturbance is associated with poor response to cognitive and behavioral treatments for insomnia in postmenopausal women. Sleep Med. 73, 82–92. doi: 10.1016/j.sleep.2020.04.024
Krakow, B., Melendrez, D., Ferreira, E., Clark, J., Warner, T. D., Sisley, B., et al. (2001). Prevalence of insomnia symptoms in patients with sleep-disordered breathing. Chest 120, 1923–1929. doi: 10.1378/chest.120.6.1923
Krystal, A. D., and Edinger, J. D. (2010). Sleep EEG predictors and correlates of the response to cognitive behavioral therapy for insomnia. Sleep 33, 669–677. doi: 10.1093/sleep/33.5.669
Lang, C. J., Appleton, S. L., Vakulin, A., McEvoy, R. D., Wittert, G. A., Martin, S. A., et al. (2017). Co−morbid OSA and insomnia increases depression prevalence and severity in men. Respirology 22, 1407–1415. doi: 10.1111/resp.13064
Lechat, B., Hansen, K., Catcheside, P., and Zajamsek, B. (2020). Beyond K-complex binary scoring during sleep: probabilistic classification using deep learning. Sleep 43:zsaa077.
Luyster, F. S., Buysse, D. J., and Strollo, P. J. (2010). Comorbid insomnia and obstructive sleep apnea: challenges for clinical practice and research. J. Clin. Sleep Med. 6, 196–204. doi: 10.5664/jcsm.27772
Massimini, M., Huber, R., Ferrarelli, F., Hill, S., and Tononi, G. (2004). The sleep slow oscillation as a traveling wave. J. Neurosci. 24, 6862–6870. doi: 10.1523/jneurosci.1318-04.2004
Miller, C. B., Espie, C. A., Bartlett, D. J., Marshall, N. S., Gordon, C. J., and Grunstein, R. R. J. (2018). Acceptability, tolerability, and potential efficacy of cognitive behavioural therapy for insomnia disorder subtypes defined by polysomnography: a retrospective cohort study. Sci. Rep. 8:6664.
Morin, C. M., Belleville, G., Bélanger, L., and Ivers, H. (2011). The insomnia severity index: psychometric indicators to detect insomnia cases and evaluate treatment response. Sleep 34, 601–608. doi: 10.1093/sleep/34.5.601
Natsky, A., Vakulin, A., Chai-Coetzer, C., Lack, L., McEvoy, R., Lovato, N., et al. (2020). Economic evaluation of cognitive behavioural therapy for insomnia (CBT-I) for improving health outcomes in adult populations: a systematic review. Sleep Med. Rev. 54:101351. doi: 10.1016/j.smrv.2020.101351
Ong, J. C., Crawford, M. R., Dawson, S. C., Fogg, L. F., Turner, A. D., Wyatt, J. K., et al. (2020). A randomized controlled trial of CBT-I and PAP for obstructive sleep apnea and comorbid insomnia: main outcomes from the MATRICS study. Sleep 43:zsaa041.
Ozminkowski, R. J., Wang, S., and Walsh, J. K. (2007). Direct and indirect costs of untreated insomnia in adults in the United States. Sleep 30, 263–273. doi: 10.1093/sleep/30.3.263
Peppard, P. E., Szklo-Coxe, M., Hla, K. M., and Young, T. (2006). Longitudinal association of sleep-related breathing disorder and depression. Arch. Intern. Med. 166, 1709–1715. doi: 10.1001/archinte.166.16.1709
Philip, P., Bioulac, S., Altena, E., Morin, C. M., Ghorayeb, I., Coste, O., et al. (2018). Specific insomnia symptoms and self-efficacy explain CPAP compliance in a sample of OSAS patients. PLoS One 13:e0195343. doi: 10.1371/journal.pone.0195343
Pieh, C., Bach, M., Popp, R., Jara, C., Crönlein, T., Hajak, G., et al. (2012). Insomnia symptoms influence CPAP compliance. Sleep Breath. 17, 99–104. doi: 10.1007/s11325-012-0655-9
Prerau, M. J., Brown, R. E., Bianchi, M. T., Ellenbogen, J. M., and Purdon, P. L. (2017). Sleep neurophysiological dynamics through the lens of multitaper spectral analysis. Physiology 32, 60–92. doi: 10.1152/physiol.00062.2015
Qaseem, A., Kansagara, D., Forciea, M. A., Cooke, M., and Denberg, T. D. (2016). Management of chronic insomnia disorder in adults: a clinical practice guideline from the American College of Physicians. Ann. Intern. Med. 165, 125–133. doi: 10.7326/m15-2175
Ruehland, W. R., Rochford, P. D., O’Donoghue, F. J., Pierce, R. J., Singh, P., and Thorton, A. T. (2009). The new AASM criteria for scoring hypopneas: impact on the apnea hypopnea index. Sleep 32, 150–157. doi: 10.1093/sleep/32.2.150
Schutte-Rodin, S., Broch, L., Buysse, D., Dorsey, C., and Sateia, M. (2008). Clinical guideline for the evaluation and management of chronic insomnia in adults. J. Clin. Sleep Med. 4, 487–504. doi: 10.5664/jcsm.27286
Scott, H., Lechat, B., Lovato, N., and Lack, L. (2020). Correspondence between physiological and behavioural responses to vibratory stimuli during the sleep onset period: a quantitative electroencephalography analysis. J. Sleep Res. e13232. (in press). doi: 10.1111/jsr.13232
Smith, S., Sullivan, K., Hopkins, W., and Douglas, J. (2004). Frequency of insomnia report in patients with obstructive sleep apnoea hypopnea syndrome (OSAHS). Sleep Med. 5, 499–456.
Suraiya, S., and Lavie, P. (2006). Sleep onset insomnia in sleep apnea patients: influence on acceptance of nCPAP treatment. Sleep Med. 7(Suppl.), S85.
Sweetman, A., Lack, L., and Bastien, C. (2019a). Co-Morbid Insomnia and Sleep Apnea (COMISA): prevalence, consequences, methodological considerations, and recent randomized controlled trials. Brain Sci. 9:371. doi: 10.3390/brainsci9120371
Sweetman, A., Lack, L., Catcheside, P., Antic, N., Smith, S., Chai-Coetzer, C. L., et al. (2019b). Cognitive and behavioral therapy for insomnia increases the use of continuous positive airway pressure therapy in obstructive sleep apnea participants with co-morbid insomnia: a randomized clinical trial. Sleep 42, zsz178.
Sweetman, A., Lack, L. C., Catcheside, P. G., Antic, N. A., Chai-Coetzer, C., Smith, S., et al. (2017a). Developing a successful treatment for co-morbid insomnia and sleep apnoea. Sleep Med. Rev. 33, 28–38. doi: 10.1016/j.smrv.2016.04.004
Sweetman, A., Lack, L. C., Lambert, S., Gradisar, M., and Harris, J. (2017b). Does co-morbid obstructive sleep apnea impair the effectiveness of cognitive and behavioral therapy for insomnia? Sleep Med. 39, 38–46. doi: 10.1016/j.sleep.2017.09.003
Sweetman, A., McEvoy, R., Smith, S., Catcheside, P., Antic, N., Chai-Coetzer, C., et al. (2020). The effect of cognitive and behavioral therapy for insomnia on week-to-week changes in sleepiness and sleep parameters in insomnia patients with co-morbid moderate and severe sleep apnea: a randomized controlled trial. Sleep 43:zsaa002.
Tasbakan, M. S., Gunduz, C., Pirildar, S., and Basoglu, O. K. (2018). Quality of life in obstructive sleep apnea is related to female gender and comorbid insomnia. Sleep Breath. 22, 1013–1020. doi: 10.1007/s11325-018-1621-y
The American Academy of Sleep Medicine (2014). International Classification of Sleep Disorders (ICSD-3), Diagnostic and Coding Manual, 3rd Edn. Westchester, IL: The American Academy of Sleep Medicine.
Troxel, W. M., Conrad, T. S., Germain, A., and Buysse, D. J. (2013). Predictors of treatment response to brief behavioral treatment of insomnia (BBTI) in older adults. J. Clin. Sleep Med. 9, 1281–1289. doi: 10.5664/jcsm.3270
Vgontzas, A. N., Fernandez-Mendoza, J., Liao, D., and Bixler, E. O. (2013). Insomnia with objective short sleep duration: the most biologically severe phenotype of the disorder. Sleep Med. Rev. 17, 241–254. doi: 10.1016/j.smrv.2012.09.005
Wickwire, E. M., Shaya, F. T., and Scharf, S. M. (2016). Health economics of insomnia treatments: the return on investment for a good night’s sleep. Sleep Med. Rev. 30, 72–82. doi: 10.1016/j.smrv.2015.11.004
Wickwire, E. M., Smith, M. T., Birnbaum, S., and Collop, N. A. (2010). Sleep maintenance insomnia complaints predict poor CPAP adherence: a clinical case series. Sleep Med. 11, 772–776. doi: 10.1016/j.sleep.2010.03.012
Keywords: COMISA, chronic insomnia, sleep disordered breathing, obstructive sleep apnea, Polysomnogram, precision medicine, CBTi, qEEG
Citation: Sweetman A, Lechat B, Catcheside PG, Smith S, Antic NA, O’Grady A, Dunn N, McEvoy RD and Lack L (2021) Polysomnographic Predictors of Treatment Response to Cognitive Behavioral Therapy for Insomnia in Participants With Co-morbid Insomnia and Sleep Apnea: Secondary Analysis of a Randomized Controlled Trial. Front. Psychol. 12:676763. doi: 10.3389/fpsyg.2021.676763
Received: 06 March 2021; Accepted: 13 April 2021;
Published: 04 May 2021.
Edited by:
Susanna Jernelöv, Karolinska Institutet (KI), SwedenReviewed by:
Andrea Galbiati, Vita-Salute San Raffaele University, ItalyCopyright © 2021 Sweetman, Lechat, Catcheside, Smith, Antic, O’Grady, Dunn, McEvoy and Lack. This is an open-access article distributed under the terms of the Creative Commons Attribution License (CC BY). The use, distribution or reproduction in other forums is permitted, provided the original author(s) and the copyright owner(s) are credited and that the original publication in this journal is cited, in accordance with accepted academic practice. No use, distribution or reproduction is permitted which does not comply with these terms.
*Correspondence: Alexander Sweetman, YWxleGFuZGVyLnN3ZWV0bWFuQGZsaW5kZXJzLmVkdS5hdQ==
†Deceased
‡These authors share senior authorship
Disclaimer: All claims expressed in this article are solely those of the authors and do not necessarily represent those of their affiliated organizations, or those of the publisher, the editors and the reviewers. Any product that may be evaluated in this article or claim that may be made by its manufacturer is not guaranteed or endorsed by the publisher.
Research integrity at Frontiers
Learn more about the work of our research integrity team to safeguard the quality of each article we publish.