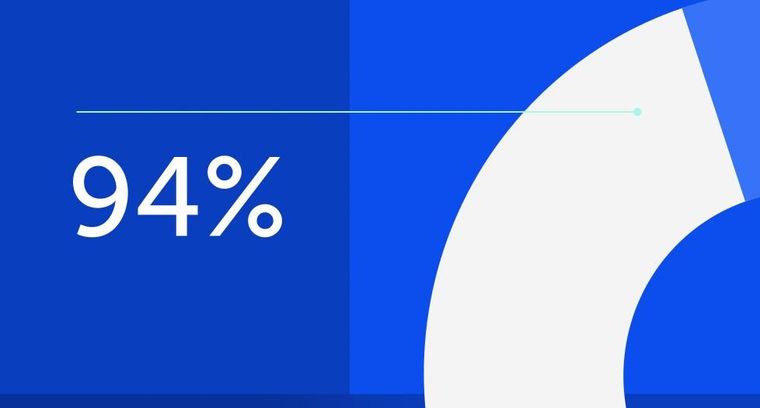
94% of researchers rate our articles as excellent or good
Learn more about the work of our research integrity team to safeguard the quality of each article we publish.
Find out more
ORIGINAL RESEARCH article
Front. Psychol., 22 January 2021
Sec. Movement Science
Volume 12 - 2021 | https://doi.org/10.3389/fpsyg.2021.636153
This article is part of the Research TopicFutsal research and challenges for sport developmentView all 23 articles
Introduction: The present study aimed to investigate the effects of two high-intensity interval training (HIIT) shuttle-run-based models, over 10 weeks on aerobic, anaerobic, and neuromuscular parameters, and the association of the training load and heart rate variability (HRV) with the change in the measures in young futsal players.
Methods: Eleven young male futsal players (age: 18.5 ± 1.1 years; body mass: 70.5 ± 5.7 kg) participated in this study. This pre-post study design was performed during a typical 10 weeks training period. HIIT sessions were conducted at 86% (HIIT86; n = 6) and 100% (HIIT100; n = 5) of peak speed of the FIET. Additionally, friendly and official matches, technical-tactical and strength-power training sessions were performed. Before and after the training period, all players performed the FIET, treadmill incremental, repeated sprint ability (RSA), sprint 15-m, and vertical jump tests (CMJ and SJ), and the HRV was measured. Training load (TL) was monitored using the session rating of perceived effort. Data analysis was carried out using Bayesian inference methods.
Results: The HIIT86 model showed clear improvements for the peak oxygen uptake (VO2peak), peak speed in the treadmill incremental test, first and second ventilatory thresholds, RSA best and mean times, CMJ, and SJ. The HIIT100 model presented distinct advances in VO2peak, peak speed in the treadmill incremental test, RSA mean time, and CMJ. Between HIIT models comparisons showed more favorable probabilities of improvement for HIIT86 than HIIT100 model in all parameters. TL data and HIIT models strongly explained the changes in the RSA mean and best times (R2 = 0.71 and 0.87, respectively), as well as HRV changes, and HIIT models explained positively VO2peak changes (R2 = 0.72). All other changes in the parameters were low to moderately explained.
Conclusion: The HIIT86 proved to be more effective for improving aerobic, RSA, and neuromuscular parameters than HIIT100 during a typical 10-week futsal training period. So, strength and conditioning specialists prescribing shuttle-run intermittent exercises at submaximal intensities can manage the individual acceleration load imposed on athlete increasing or decreasing either the set duration or the frequency of change of direction during HIIT programming.
Futsal is a team sport involving a complex range of high-intensity locomotor activities, requiring both aerobic and anaerobic fitness to cope with the multiple requirements of the match (Ribeiro et al., 2020). Research studies investigating this sport modality have increased significantly over the past two decades (Barbero-Alvarez et al., 2008; Castagna et al., 2009; De Oliveira Bueno et al., 2014; Caetano et al., 2015; Nakamura et al., 2020; Ribeiro et al., 2020), contributing to better understanding of the physical and skills requirements during the futsal match and organization of the training contents. Time motion analysis studies reported that during a single match, futsal players cover, on average, a total distance of 3000–4000 m, of which 8–13 and 5–9% are performed at high-speed running (HSR; >15.5 km/h) and sprinting (>18.3 km/h), respectively (Barbero-Alvarez et al., 2008; Castagna et al., 2009; De Oliveira Bueno et al., 2014). Repeated sprint sequences (RSS) with up to 2 to 3 sprints interspersed with 15 s of recovery are also frequently (∼80 occurrences per match) performed in futsal (Caetano et al., 2015). Similarly, the execution of deceleration and acceleration actions constitutes a critical part of the futsal players’ work-rate (Ribeiro et al., 2020). Thus, HSR episodes, sprints, RSS, changes of directions (COD), and acceleration and deceleration actions are among the main types of activities that players should be prepared to perform efficiently. This information may be of practical relevance for the design of suitable training programs in order to enhance the main physical capacities related to successful performance in futsal.
The training intensity is among the first training variables to be manipulated in most physical conditioning programs for athletes (Buchheit and Laursen, 2013). The definition of number and duration of sets performed per training session is also a key component to determine the total training volume (i.e., total work duration performed) (Buchheit and Laursen, 2013). Thus, any manipulation in these variables, in an isolated or combined manner, will be decisive to determine the magnitude of adaptations to training (Buchheit and Laursen, 2013). High-intensity interval training (HIIT) and repeated-sprint/sprint interval training (RST/SIT) are currently among the most frequently used training models in physical conditioning programs of team sports players (Buchheit et al., 2008; Ferrari-Bravo et al., 2008; Buchheit and Laursen, 2013; McGinley and Bishop, 2016). The current evidence comparing RST/SIT vs. HIIT models demonstrates conflicting results, with some studies showing reduced gains (Buchheit et al., 2008) and others superior gains (Ferrari-Bravo et al., 2008) in performance after RST/SIT compared to HIIT models. Other studies have examined the effects of two work-matched HIIT models performed at different training intensities (McGinley and Bishop, 2016; Viaño-Santasmarinas et al., 2018). For instance, Viaño-Santasmarinas et al. (2018) did not find any improvements in aerobic fitness indices and RSA performance after two work-matched, HIIT models, performed at 85 and 95% of the final speed obtained in the 30–15 Intermittent Fitness Test (VIFT) in professional handball players. This finding is in line with other research reporting no effect of training intensity on RSA performance outcomes following HIIT models (McGinley and Bishop, 2016). Thus, these two studies concluded that distinct training intensities during HIIT models produce similar performance enhancements when the same total exercise duration (i.e., isotime) is applied. While this isotime approach is a methodological strategy used in several studies to investigate the isolated effect of training intensity (McGinley and Bishop, 2016; Teixeira et al., 2018, 2019; Viaño-Santasmarinas et al., 2018), few studies have addressed the potential effects of distinct HIIT models varying in exercise intensity and total work duration on the athletic performance of team sport players (Ferrari-Bravo et al., 2008; Maggioni et al., 2019). Prior research studies investigating the integrative effects of these two key variables on the adaptive responses to distinct HIIT formats are limited to individual endurance sports athletes (Seiler et al., 2013).
Considering the multidirectional running pattern during futsal matches, HIIT strategies are usually composed of shuttle-runs in order to increase the specificity of these HIIT drills (Akenhead et al., 2014; Teixeira et al., 2018, 2019). In this sense, the simultaneous manipulation of the exercise intensity and total work duration during shuttle run HIIT models will have a direct influence on the magnitude and total number of acceleration and deceleration actions performed for each COD during a training session, respectively (Buchheit and Laursen, 2013; Akenhead et al., 2014). For instance, shuttle-run drills with a greater frequency of COD elicit an increased metabolic, cardiovascular, neuromuscular, and perceptual response in team sport athletes (Akenhead et al., 2014). Of interest, the number of COD performed during a short-term training period (5–6 weeks) was shown to be crucial to improve a variety of physical performance parameters in female team sport players (Sanchez-Sanchez et al., 2018; Teixeira et al., 2019). This research topic still needs further investigation, since the same effects observed in female athletes have not been noticed in male athletes (da Silva et al., 2015; Attene et al., 2016). Therefore, comparative studies examining the effects of two HIIT models at submaximal and maximal intensities with a contrasting total work duration in male futsal players could address practical questions on whether shuttle run HIIT programs, taking into account the physiological consequences of COD (Akenhead et al., 2014; Teixeira et al., 2018), would be more effective if performed at lower intensities with longer sets or at higher intensities with shorter set durations.
The dose-response relationship between the accumulated training load (TL) and performance adaptations is another relevant topic in the field of team sports, which deserves attention from coaches and sport scientists. The majority of studies examining the dose-response relationship have been primarily conducted with soccer and rugby players (Taylor et al., 2018; Daniels et al., 2019; Rabbani et al., 2019; Ellis et al., 2020). To date, few studies have investigated the dose-response relationship between TL and performance adaptations in futsal (Oliveira et al., 2013; Nakamura et al., 2020). Oliveira et al. (2013) did not find any association between TL and heart rate variability (HRV) and aerobic-anaerobic performance changes during seasonal training phases in professional futsal players. Differently, Nakamura et al. (2020) showed that accumulated TL negatively affected the physical and physiological adaptations of elite futsal players. Thus, further studies are still warranted to better understand the potential consequences of accumulated TL during the preseason phase on the subsequent changes in performance in futsal teams, especially in youth players.
The current study aimed to compare the effects of two shuttle run HIIT models performed at 86% (HIIT86) and 100% (HIIT100) of peak speed derived from the Futsal Intermittent Endurance Test (FIET, PSFIET) with a total work duration of 16 and 8 min, respectively, implemented over a period of 10 weeks, on the HRV, aerobic fitness, RSA, and neuromuscular performance of young male futsal players. A second aim of this study was to examine the dose-response relationships between accumulated TL and changes in physical and physiological measures. Based on previous studies (Sanchez-Sanchez et al., 2018; Teixeira et al., 2018, 2019) our hypothesis was that the HIIT model with more COD and longer set duration (HIIT86) would induce superior improvements on the selected physiological and physical measures than the model with less COD and shorter set duration performed at a higher intensity (HIIT100).
The inclusion criteria for the study were regular participation in, at least, 75% of the training sessions during the period of investigation, not suffering from injuries during the same period, and not taking any medication that could alter the outcome of this study. Eleven young male futsal players (mean ± standard deviation; age: 18.5 ± 1.1 years; body mass: 70.5 ± 5.7 kg; height: 1.78 ± 0.07 m) from the U-20 professional futsal team of the first division of Paraná state – Brazil took part in this study. None of the players suffered any injury during the study period and all of them attended more than 75% of the training sessions during the 10 weeks of training. All players and their guardians were informed about the procedures of the study and signed an informed consent form. This study was approved by the local research ethics committee (n° 93777318.0.0000.0121) in accordance with current national and international laws and regulations governing the use of human subjects (Declaration of Helsinki II).
A parallel 2-group longitudinal experimental study design was performed during 10 weeks from February to April of 2019 (5 weeks of pre-season and 5 weeks during the early in-season). During the study period, players were monitored over 105 training sessions, which were distributed into 16 sessions devoted to HIIT models (8 sessions for each group: HIIT100 and HIIT86) experimentally implemented for the purposes of this study, 23 sessions to develop strength-power characteristics, 56 sessions dedicated to futsal-specific technical-tactical skills, and 10 matches (3 friendly and 7 official matches; Figure 1A). Before and after the 10-week period, the following physical fitness tests were conducted on three separate days: first day (Monday): resting HRV and incremental treadmill test; second day (Wednesday): vertical jump (CMJ and SJ), straight 15-m sprint, and repeated sprint ability (RSA with COD: 8 x 10+20+10 m) tests; third day (Friday): FIET protocol (Figure 1B). Testing sessions were carried out in a laboratory and on an indoor futsal court, separated by 48 h between each session (Figure 1B), following the procedures of a previous study (Buchheit et al., 2008). Participants were allowed to drink water ad libitum during the field testing and training sessions. The internal training load of each player was monitored daily using the session rating of perceived exertion (s-RPE) during all training sessions and matches (Foster et al., 2001; Fanchini et al., 2016).
Figure 1. Experimental design with the number of each training/match session type in each week (A), and the testing sessions at pre and post 10 weeks of training (B). HIIT, high intensity interval training; HRV, heart rate variability; SJ, squat jump; CMJ, counter movement jump; RSA, repeated sprint ability test; FIET, Futsal Intermittent Endurance Test.
During the intervention period, two different shuttle-run HIIT models were applied based on the individual PSFIET of each player, and both training models were performed once a week. Due to the team’s training schedule, the HIIT sessions started only in the 2nd week and ended in the 9th week of training (last week before the 10th congested week: 2 matches within a 7-day period). All HIIT sessions were carried out before the tactical-technical sessions in the morning period. The HIIT86 model consisted of 4 sets of 4-min bouts performed at 86% of PSFIET with 3 min of passive recovery between the sets, whereas the HIIT100% model was composed of 8 sets of 60 s bouts at 100% of PSFIET with 45 s of passive recovery between the sets. Each bout was characterized by 15 s of running effort followed by 15 s of passive rest. Thus, players performed 8 and 2 repetitions of 15 s shuttle runs (with a COD every 3.75 s) during each set of the HIIT86% and HIIT100% models, respectively (Teixeira et al., 2018, 2019). Consequently, all players performed a total of 128 and 64 accelerations and 96 and 48 decelerations/COD actions per training session during the HIIT86% and HIIT100% models, respectively. The average running pace performed by the athletes between the start and return lines for each training model was dictated by a prerecorded audio cue, emitting beeps every 3.75 s (Speaker, Satellite, Taiwan). The distance covered by each athlete during the training sessions was individualized according to their respective PSFIET.
A progressive incremental exercise test was performed on a motorized treadmill (Imbramed ATL, Porto Alegre, Brazil). During the test the treadmill inclination was set at a 1% gradient with an initial speed of 9.0 km/h and then the treadmill speed was increased by 1.0 km/h every minute until volitional exhaustion (Kuipers et al., 2003). The peak speed (PSTREADMILL) was calculated according to procedures described elsewhere (Kuipers et al., 2003). Each participant was verbally encouraged to deliver maximum effort during the incremental test. Respiratory gasses were measured breath by breath during the test using a calibrated online metabolic system (K5; COSMED, Rome, Italy). For peak oxygen uptake (VO2peak), and first and second ventilatory threshold (VT1 and VT2, respectively) determination, all gas exchange data were filtered using K5 software (Omnia; COSMED, Rome, Italy) to discard outlier points. Subsequently, the data were reduced to means of 15 s for further analysis. The highest 15 s value of oxygen uptake (VO2) was considered as VO2peak. For VT1 and VT2 determination the ventilation/oxygen uptake (VE/VO2) and ventilation/carbon dioxide production (VE/VCO2) equivalents were used. The first abrupt increase in VE/VO2 without a concomitant increase in VE/VCO2 was considered the VT1 (Caiozzo et al., 1982), and the first abrupt increase in VE/VCO2 was considered the VT2 (McLellan, 1985). The speed at each threshold was determined.
The FIET consisted of shuttle-run bouts of 45 m (i.e., 3 × 15 m) performed at progressive speeds until voluntary exhaustion (Castagna and Barbero Álvarez, 2010). Every 45 m, players were allowed to actively rest for 10 s. After each 8 × 45 m bout, players passively rested for 30 s. The starting velocity was set at 9.0 km/h with speed increments of 0.33 km/h during the first 9 × 45 m bouts. After 9 × 45 m bouts, the increment changed to 0.20 km/h every 45 m until exhaustion. The speed was controlled by prerecorded audio cues (Speaker, Satellite, Taiwan). The test was finished when participants did not reach the front line in time with the beeps for 2 consecutive repetitions. The peak speed (i.e., PSFIET) reached at the end of the test by the athletes was reported as the performance criterion for the FIET.
Vertical jump height (cm) was determined using the counter movement jump (CMJ) and the squat jump (SJ). In the CMJ, the participants were instructed to execute a downward movement followed by a complete extension of the legs and were free to determine the countermovement amplitude to avoid changes in jumping coordination. In the SJ, the participants were required to remain in a static position with a 90° knee flexion angle for 3 s before jumping, without any preparatory movements. The CMJ and SJ were executed with the hands fixed on the hips. All jumps were performed on a contact platform (CEFISE, Brazil). A total of 3 attempts were allowed for each jump with a 45 s rest interval between attempts. The best CMJ and SJ attempts were used for further analysis (Bosco et al., 1983).
All players performed three maximal 15 m sprints with at least 2 min of passive rest between the three trials (Cronin and Hansen, 2005). Sprint time was recorded using a photocell system (Microgate, Italy) with timing gates placed at the 0 m (i.e., starting gate) and 15 m marks (i.e., finishing gates). All sprinting tests were conducted on an indoor futsal court, thus eliminating any potential effect of the environmental conditions. The best sprint time was retained for further analysis.
The 40-m RSA test consisted of 8 × 40 m sprints separated by 20 s of passive recovery (Baker et al., 1993). The athlete stared 0.5 m behind the start line and times were recorded electronically via photocells (Microgate, Italy). Before the RSA test, players performed a standardized 5-min warm-up of progressive runs and accelerations that were administered by the team’s physical trainer. Before starting, athletes were instructed to run as fast as possible between two lines placed 20 m apart, with the start/finish line (and the photocells) placed at the midpoint of the course. Each participant sprinted 10 m from the start/finish line to the end of the course, turned 180°, sprinted 20 m to the other end of the course, turned 180°, and sprinted 10 m back through the start/finish line. Following each sprint, the athlete decelerated and walked to the starting line in readiness for the subsequent sprint. Five seconds prior to the next sprint, the athletes assumed the starting position and a 3 s countdown was provided to commence again. The best (RSABEST) and mean sprint times (RSAMEAN) were recorded as the performance indices.
The resting HRV was obtained by time elapsed between two successive R-waves of the QRS signal of the heart rate (R-R intervals) using an RS800cx (Polar Electro, Finland) heart rate monitor. The resting HRV was recorded on Monday mornings at 7:00 a.m., before and after the 10-week period (Figure 1B). During the RR recordings, all players remained at rest for 5 min in the supine position following the standards set by the Task Force (Task Force, 1996). The first 2 min were excluded (signal stabilization), and the remaining 3 min were used to calculate the resting HRV indices. Correction of ectopic beats and/or erroneous signals was performed automatically using the manufacturer’s software (Kubios HRV Analysis, Finland) with a degree of correction < 3% for all recordings, and when necessary, manual correction of artifacts was performed. The resulting R-R intervals were examined in only 1 time-domain index (i.e., root mean square difference of successive normal R-R intervals [rMSSD]). The rMSSD has been reported to reflect vagal modulation and to be related to training-induced effects (Buchheit et al., 2009). The delta change (post – pre training values) in rMSSD (ΔrMSSD) was used for analysis.
The internal TL was measured using the s-RPE method (Foster et al., 2001). Thirty minutes after the completion of each training session and matches, players were requested to report RPE for the intensity of the training sessions and matches using a 0-100 point RPE scale proposed by Borg and Borg (2002) and recently validated by Fanchini et al. (2016). The 0-100 scale value reported by the players was divided by 10 (i.e., RPE: 75 ÷ 10 = 7.5), and this value was multiplied by session duration, in minutes, to calculate the TL of each training session and matches. When two training sessions were performed on the same day, the TL was summed to create the daily TL. During each training week, the daily TL was summed to create the total weekly TL.
The analysis was performed using established Bayesian inference methods. The physiological and performance data were analyzed as percentage deltas of pre-measure (Δ% = ((Post−Pre)/Pre)×100) (except for HRV that was analyzed in raw units). Analysis was performed using the linear modeling procedure, with the training models (i.e., HIIT100 and HIIT86), and the baseline measures centered to the mean of all study subjects included as fixed effects. Additionally, a dose-response analysis was used to verify the relationship of responses to training with the TL and HRV measures. Thus, the training models, delta in the HRV or training load measure, and interaction between training models × HRV/training load were inserted in the model as fixed effects. The Bayesian R2 was calculated as an estimate of the proportion of variance explained for new data (Gelman et al., 2019). Model fitting is performed using Markov Chain Monte Carlo (MCMC) methods, more specifically the No-U-Turn (NUTS) sampler implemented in Stan. Student t-distribution (df = 3, μ = 0, and σ = 10) priors were set to be non-informative, so that their influence on the estimates was relatively small (Gelman et al., 2008). Unless otherwise stated, all observed data are reported as means ± standard deviations (SD), and the posterior data as means with 90% highest density credible intervals (CIs) for pre to post changes, and medians with 90% equal tailed CIs for dose-response analysis. The CI represents there is a 90% probability that the parameter is contained within a 90% CI (Morey et al., 2016).
Inferences about the effects were made by interpreting the 90% CI in relation to the region of practical equivalence (ROPE). We specified our ROPE as 0.2 × between-subjects SD (Hopkins et al., 2009). Thus, the ROPEs for VO2peak, PSFIET, PSTREADMILL, VT2, VT1, RSABEST, RSAMEAN, sprint 15-m, CMJ, SJ, and HRV are ± 1.8%, ± 0.9%, ± 1.0%, ± 2.0%, ± 2.3%, ± 0.5%, ± 0.7%, ± 0.9%, ± 1.7%, ± 2.1%, and ± 6.1 ms, respectively. Therefore, an effect was deemed “trivial” when the two bounds of the 90% CI were within the ROPE. Conversely, when the CI overlapped the ROPE the effects were interpreted as “undecided” (Kruschke, 2018). When the two 90% CI bounds were out of the ROPE the effect was deemed as “beneficial” or “harmful”, when positive and negative, respectively; except for RSAMEAN, RSABEST, and Sprint 15-m where negative and positive effects were “beneficial” and “harmful”, respectively. Additionally, based on the posterior distributions, we calculated the probability (%) of the effect to be harmful/trivial/beneficial. Statistical analyses were performed using statistical software R (v4.0; R Core Team (2020), Vienna, Austria) and its graphical interface RStudio (v1.2.5). The package “brms” (Bürkner, 2017) allowing fitting of Bayesian multilevel models using “Stan” (Gelman et al., 2015) was used for analysis.
Descriptive statistics of observed data (mean ± standard deviation [range]) for aerobic, RSA, sprint, and vertical jump performances before (pre-) and after (post-training) the training period (10 weeks) in each HIIT model are presented in Table 1.
Table 1. Observed means ± SD (minimum – maximum) of physiological, and performance parameters of futsal players in each HIIT model pre and post ten weeks of training and the changes.
Changes for the parameters determined during the FIET and treadmill incremental tests are displayed in Figure 2. The HIIT100 model showed clear beneficial effects (i.e., the full 90% CI boundaries out of ROPE) for VO2peak and PSTREADMILL measurements. For the HIIT86 model, clear beneficial changes occurred in the VO2peak, PSTREADMILL, VT2, and VT1. For the HIIT100 model, VT2 and VT1 changes were well supported within the ROPE, nevertheless, these effects are inconclusive because they overlapped the lower and upper ROPE boundaries. For PSFIET, both HIIT models presented no clear improvements, although the probabilities were high for improvement (> 89%), low for trivial (< 8%), and negligible for impairment (< 2.6%). Between HIIT models comparisons showed more favorable probabilities in favor of HIIT86 than HIIT100 in all parameters; however, clearly more favorable changes for the HIIT86 model compared to the HIIT100 model were observed only for PSTREADMILL, VT2, and VT1 (probabilities > 96%).
Figure 2. Posterior density distributions and the respective means with 90% credible intervals of the pre to post changes in VO2peak, PSFIET, PSTREADMILL, VT2, and VT1 (upper to lower panels, respectively) in each HIIT model, and the comparison of changes between models. The effects are adjusted to baseline mean of all study subjects. Black points and error bars are the posterior mean change and 90% credible intervals, respectively. Vertical dashed lines are the lower and upper boundaries of the ROPE (i.e., 0.2× between subjects SD). The texts in each graph are the means (90% CI) and probabilities against ROPE.
Pre to post changes for RSAMEAN, RSABEST, 15-m sprint, CMJ, and SJ measures are summarized in Figure 3. The HIIT100 model showed clear beneficial effects for RSAMEAN and CMJ measurements, considerable probability of improvement for 15-m sprint time (84%), and inconclusive effects for RSABEST and SJ. The HIIT86 model showed clear beneficial effects for all anaerobic running measurements, except the 15-m sprint time, where a high probability of improvement (92.1%), small/moderate of being trivial (7.1%), and negligible of being harmful (0.8%) were observed. Between HIIT models comparisons showed clearly more favorable changes for the HIIT86 than HIIT100 model in the RSABEST and SJ measures (probabilities > 96%). All other effects between model were deemed inconclusive.
Figure 3. Posterior density distributions and the respective means with 90% credible intervals of the pre to post changes in RSABEST, RSAMEAN, Sprint 15-m, CMJ, and SJ (upper to lower panels, respectively) in each HIIT model, and the comparison of changes between models. The effects are adjusted to baseline mean of all study subjects. Black points and error bars are the posterior mean change and 90% credible intervals, respectively. Vertical dashed lines are the lower and upper boundaries of the ROPE (i.e., 0.2× between subjects SD). The texts in each graph are the means (90% CI) and probabilities against ROPE.
Pre to post change in the HRV revealed clear beneficial changes in both training models, with probabilities > 98.1% (Figure 4). However, no evidence of superiority of one HIIT model over the other was observed.
Figure 4. Posterior density distributions and the respective means with 90% credible intervals of the pre to post changes in HRV in each HIIT model, and the comparison of changes between models. Black points and error bars are the posterior mean change and 90% credible intervals, respectively. Vertical dashed lines are the lower and upper boundaries of the ROPE (i.e., 0.2× between subjects SD). The texts in each graph are the means (90% CI) and probabilities against ROPE.
The total weekly TLs for both HIIT86 (black bars) and HIIT100 (gray bars) models during each training week are presented in Figure 5A. The total accumulated TLs derived from (i) all training sessions and matches (#), (ii) all training sessions and matches without HIIT sessions ($), and (iii) HIIT sessions (∗) are presented in Figure 5B. For the entire training period, the total accumulated TL from all training sessions and matches showed a mean difference [90% CI] of −1836 [−8279, 4340] arbitrary units (a.u.) between HIIT86 and HIIT100 models, with a probability of 55.9% of the TL being lesser in the HIIT86 than HIIT100. The total accumulated TL of all training sessions and matches without HIIT was lesser in the HIIT86 than HIIT100 (−2671 [90% CI; −4137, 9004] a.u.), with a probability of TL being lesser in the HIIT86 of 76.5%. Contrarily, as expected, the total accumulated TL over the 8 HIIT sessions was two-fold higher in the HIIT86 than the HIIT100 model (difference: 778 [90% CI; 609, 941] a.u.), with 100% probability of being higher in the HIIT86 model.
Figure 5. Observed means ± SD of training load sum in each week (A) and the accumulated sum over 10 weeks of all training/match sessions [#], all training/match sessions without HIIT [$], and only HIIT sessions [*] (B). Bars and error bars are the mean and SD, respectively. a.u., arbitrary units.
Since the players followed the same training routine, with the exception of the HIIT sessions, the dose-response relationship between RPE-based TL and Δ rMSSD with changes in aerobic, RSA, 15-m sprint, and jump performances was carried out, adding HIIT models as a covariate in the final model. The regression outputs between total weekly TL (10-week average) and ΔrMSSD in addition to HIIT type with changes in performance measures are displayed in Figures 6, 7, respectively. Total weekly TL and HIIT model accounted for 25 to 87% of the variance (i.e., Bayesian R2) in aerobic fitness, RSA, and power-speed-related performance changes. The explained variance derived from regression models using ΔrMSSD and HIIT type as covariates ranged from 26 to 72%.
Figure 6. Posterior regression medians with 90% credible intervals between pre-to-post changes in VO2peak (A), PSFIET (B), VT2 (C), VT1 (D), PSTREADMILL (E), RSABEST (F), RSAMEAN (G), Sprint 15-m (H), CMJ (I), and SJ (J) for each HIIT model with training load. R2, Bayesian variance explained. Horizontal dashed lines are the lower and upper boundaries of the ROPE (i.e., 0.2× between subjects SD).
Figure 7. Posterior regression medians with 90% credible intervals between pre-to-post changes in VO2peak (A), PSFIET (B), VT2 (C), VT1 (D), PSTREADMILL (E), RSABEST (F), RSAMEAN (G), Sprint 15-m (H), CMJ (I), and SJ (J) for each HIIT model with the pre-to-post change in HRV. R2, Bayesian variance explained. Horizontal dashed lines are the lower and upper boundaries of the ROPE (i.e., 0.2× between subjects SD).
The current study aimed to compare the effects of two shuttle run-based HIIT models of varying intensity and total work duration (HITT86: 16 min vs. HITT100: 8 min) on aerobic, HRV, RSA, and neuromuscular performance outcomes in junior male futsal players. The dose-response relationship between RPE-based TL and changes in performance was also examined. The main findings of this study showed that after 10-weeks of futsal training: (i) the HIIT86 model was clearly more effective at improving the PSTREADMILL (Δ = 5.4%), VT2 (Δ = 10.0%), VT1 (Δ = 13.0%), RSABEST time (Δ = −3.5%), and SJ height (Δ = 13.7%) than the HIIT100; (ii) RPE-based TL in association with HIIT type explained 71% to 87% of the inter-individual variation in RSA performance changes, while the explained variance for the other parameters was smaller (25–59%); and (iii) changes in HRV along with HIIT type accounted for 72% of inter-individual variance in VO2peak changes following the training period.
Comparative studies examining the effectiveness of different training models are increasingly needed and recommended to help guide decision-making of strength and conditioning coaches during the planning of training programs with futsal players (Buchheit et al., 2008; Ferrari-Bravo et al., 2008; Sanchez-Sanchez et al., 2018). Training intensity and total work duration are two key variables commonly altered in order to increase the physical capacity of athletes (Buchheit and Laursen, 2013) and, therefore, they need to be well managed during HIIT programming. In the current study, the improvements in physical fitness indices were specific to training type. Our data indicated that the HIIT86 model clearly improved (i.e., full 90% CI out of ROPE) the majority of the physical and physiological parameters measured (9 out of 11 parameters; Figures 2, 3, 4) compared to the HIIT100 model (5 out of 11 parameters; Figures 2, 3, 4). In addition, the HIIT86 model induced larger improvements in aerobic, RSA, and neuromuscular performance outcomes than the HIIT100 model. The results presented herein suggest that the HIIT86 training, comprising longer sets at a lower intensity, was more effective to enhance performance than the HITT100 composed of shorter sets and more intense running efforts. Similarly, Buchheit et al. (2008) also reported greater performance adaptations after HIIT models performed at lower running intensities (i.e., close to 90–95% VIFT) in male adolescent handball players. Seiler et al. (2013) also showed that training intensity and total work duration influenced the magnitude of adaptive responses following distinct HIIT models (4 × 4 min; 4 × 8 min; 4 × 16 min) in trained cyclists. On the other hand, current studies published in the literature suggests that HIIT models of different training intensities induced similar performance enhancements in male adults (McGinley and Bishop, 2016; Viaño-Santasmarinas et al., 2018). Some differences between these studies should be addressed. For instance, the total work duration in our training design varied between training groups, while prior studies used an isotime approach (i.e., matched-work) only varying exercise intensity (Buchheit et al., 2008; Seiler et al., 2013; McGinley and Bishop, 2016; Viaño-Santasmarinas et al., 2018). In addition, the reference running speed used for training prescription also differed between the cited studies (Buchheit et al., 2008; Viaño-Santasmarinas et al., 2018). Thus, comparison between the current results and those observed in prior studies should be interpreted with caution due to differences in sample characteristics (e.g., chronological age), methodological issues, sport modality, training demands, and performance levels.
It is well known and accepted that aerobic fitness and RSA performance are two discriminant physical qualities of the competitive level in futsal (Álvarez et al., 2009; Pedro et al., 2013; Ayarra et al., 2018). Thus, training strategies targeting the development of these physical qualities simultaneously are essential. Our findings indicating the superiority of HIIT86 over HIIT100 at improving aerobic fitness, RSA, and vertical jump performance suggest that this training type (submaximal runs at 86% PSFIET and longer sets) should be preferentially used with young futsal players. The specific adaptations in physical performance following the HIIT86 and HITT100 models could potentially be related to differences in total work duration between the models (Teixeira et al., 2018, 2019). Due to its higher total running volume (16 vs. 8 min), the HIIT86 implies a greater number of COD performed in a single session (96 vs. 48 turns), increasing the total time that athletes spent accelerating per running bout compared to the HIIT100 model. Akenhead et al. (2014) showed that the number of turns and time spent accelerating (>± 1 m⋅s–2) are linearly related during shuttle run drills. Prior research has indicated that accumulated individual acceleration load is positively associated with changes in aerobic fitness and neuromuscular measures in professional soccer players (Clemente et al., 2019). Given that acute and chronic responses to training are dependent on acceleration load accumulated during multidirectional drills (Akenhead et al., 2014; Clemente et al., 2019), practitioners and coaches can increase or decrease the acceleration load accumulated by athlete during shuttle run-based HIIT varying either set duration as in this study or COD frequency per running bout (Sanchez-Sanchez et al., 2018; Teixeira et al., 2018, 2019). To date, to the best of our knowledge, this is the first study demonstrating that a HIIT model with more directional changes leads to superior gains in physical performance in a sample of aerobically well-trained male futsal players (VO2peak > 55 mL/kg/min at baseline). Previous studies published on this research topic did not show any further performance gain after different shuttle run training models varying the number of COD required per running bout in male soccer and basketball players (da Silva et al., 2015; Attene et al., 2016). Of interest, the same HIIT86 model used here was also applied in a sample of female futsal players (VO2peak: 47–49 mL/kg/min at baseline) (Teixeira et al., 2018, 2019). The current and prior studies (Teixeira et al., 2018, 2019) showed a greater improvement in PSTREADMILL and RSA performance after HIIT86 model. This demonstrates the consistency and effectiveness of this training model (HIIT86) to improve these physical qualities in age-matched male and female futsal players. At the same time, male and female futsal athletes of similar ages can display distinct neuromuscular performance adaptations (i.e., changes in SJ and CMJ height) following HIIT86, with male athletes in the current study being more responsiveness (Δ = 13–17%) than female athletes (Δ = 8–9%) in the study of Teixeira et al. (2018). Therefore, it should be highlighted that any generalization of our findings to other samples of futsal (male or female; young or adult) or other indoor team sports (e.g., basketball and handball) would be a hasty inference, since differences related to age, gender and sport demand in terms of workload and training content distribution during a typical training period could influence the effectiveness of this HIIT86 model in these other sports scenarios.
From the present results on RPE-based TL and changes in physical performance, it is possible to make inferences about the dose-response relationship during the training process. The present study suggests that TL in addition to training type (HIIT86 and HIIT100) accounted for a large portion of the inter-individual variance in RSA (71–87%), 15-m Sprint (46%), and vertical jump (40–47%) performance changes. A positive linear dose-response relationship between TL and changes in these performance measures was found for the HIIT86 model, while no (RSA performance) or negative (sprint and vertical jump) relationships were identified for the HIIT100 model. These findings are of practical relevance for practitioners and coaches. First, players in both HIIT86 and HIIT100 models with a similar total TL displayed a distinct adaptive response (especially for RSABEST and SJ performance), highlighting that the quality/specificity of the training stimuli is the most relevant component of the training process (Sanchez-Sanchez et al., 2018). In this case, the increased number of COD in the HIIT86 model (longer sets) may have been decisive to induce superior gains in performance. Second, players who accumulated higher training loads in the HIIT86 model demonstrated the largest improvements in RSA, 15-m Sprint, and vertical jump performance, while the opposite was observed for the HIIT100 model. Although these results cannot be easily explained from the data analysis employed in our study, it is possible to suggest that the training loads derived from other training strategies (technical-tactical, strength-power, friendly and official matches) may have influenced the players’ adaptive response to training. For instance, players in the HIIT100 model tended to accumulate a higher (with a 73% probability) TL derived from these other training contents (i.e., not involving HIIT sessions) than the HIIT86 model (Figure 5).
The explained variances derived from regression models (TL and HIIT type inserted as covariates) for the changes in maximal (VO2peak, PSFIET, PSTREADMILL) and submaximal (VT2 and VT1) aerobic performance measures were considered low (25–28%) and moderate (45–59%), respectively. Contrary to what was observed in the anaerobic and jump performance measures, the dose-response relationship for aerobic performance outcomes did not show a distinct pattern between HIIT models (i.e., regression slopes in contrary directions). Of note, positive and negative/null relationships were observed between accumulated TL and changes in maximal (VO2peak and PSTREADMILL) and submaximal (VT2 and VT1) aerobic indices, respectively. Several studies have demonstrated a linear dose-response relationship between RPE-based TL and changes in aerobic performance indicators in team sports athletes (Oliveira et al., 2013; De Freitas et al., 2015; Clemente et al., 2019; Ellis et al., 2020; Nakamura et al., 2020). Interestingly, a recent study conducted by Ellis et al. (2020) also found a negative weak association between TL and changes at fixed lactate thresholds of 2 and 4 mmol/L (Bayesian r = −0.17 and −0.16, respectively) after 6 weeks of pre-season in a sample of male junior soccer players. Furthermore, in agreement with our data, a small positive relationship was verified between TL and PSTREADMILL changes (Bayesian r = 0.37) in the previously cited study (Ellis et al., 2020). It should be highlighted that the present study included 2 different shuttle-run HIIT types, so we chose to analyze the dose-response data with an interaction between TL and HIIT type. Thus, our Bayesian R2 values consider both the relationship of the response to the TL and the HIIT model. Therefore, comparisons with the R2 from other studies should be performed with caution to avoid misinterpretations, since they only used the TL as a covariate.
An interesting finding from our study to be highlighted was that similar improvements in the resting HRV were noticed after both HIIT models (HIIT86 and HIIT100) outlined here using PSFIET as the reference speed to calibrate running distance. Although improved resting HRV after a period of futsal training has been previously documented in the literature (Oliveira et al., 2013; De Freitas et al., 2015; Nakamura et al., 2020), our data reinforce the effectiveness of shuttle run HIIT training models to induce positive adaptations in the cardiac autonomic function of young futsal players. This assumption was previously confirmed by Buchheit et al. (2008) who showed that HIIT models are preferable compared to repeated all-out sprint training methods, since its effects on cardiac autonomic function were significantly more pronounced in young handball players. The resting HRV, a non-invasive assessment of cardiac autonomic modulation, has also been constantly related to aerobic fitness indices and high-intensity running performance changes in team sport athletes (De Freitas et al., 2015; Esco et al., 2016; Nakamura et al., 2020). The present study showed a positive relationship between changes in resting HRV (i.e., rMSSD) and VO2peak after the training period, suggesting that players in both HIIT models with greater increases in resting rMSSD demonstrated the largest increments in VO2peak. Of interest, changes in resting HRV and HIIT types accounted for 72% of the inter-individual variance in VO2peak changes in our sample. Another two studies performed with futsal players also showed that an enhanced vagal modulation (inferred by an increase in resting rMSSD value) was largely positively correlated (r = 0.62 and 0.64) with improvements in Yo-Yo IR1 performance (De Freitas et al., 2015; Nakamura et al., 2020). These explained variances (38–40%) are, at least in part, like those observed in our study for the ΔPSFIET (39%) and ΔPSTREADMILL (47%) (Figure 7). Collectively, the current and previous results indicate that resting rMSSD may be a simple and sensitive indicator to monitor changes in physical fitness during training.
One of the strengths of this study was to show how different HIIT models associated with other training components can influence the adaptive responses of players from the same futsal team. In this scenario, where few traditional HIIT sessions are planned by the team technical staff due to the matches schedule and the importance given to technical-tactical and strength/injury prevention sessions, the selection of training stimuli in HIIT sessions is key to maximizing subsequent performance adaptations. From a practical perspective, strength and conditioning specialists should consider spending more time in less intense shuttle run HIIT sessions with more COD than in more intense and shorter sessions with fewer directional changes.
The main limitation of the present study was the small sample size (n = 11). This can be justified by the low number of players who are part of a futsal squad (12–15 players). It would be extremely difficult to monitor more than one futsal team at one time. However, Bayesian analysis is better suited for making inferences on small sample sizes, as the MCMC methods used to produce posterior distributions do not depend on asymptotic behavior in the same way that traditional frequentist methods do (Kadane, 2015). Additionally, Bayesian inferences are more intuitive by posterior probability distributions of parameters (Kruschke, 2018). Nevertheless, a challenge in Bayesian statistics is the necessity to impose a prior knowledge in the parameters (i.e., prior distributions). In this way, we used non-informative default priors of the brms package (Bürkner, 2017). Therefore, the priors had little influence on the results (Gelman et al., 2008). Another point related to the analysis is that in team sports, such as futsal, there is no ROPE directly linked to performance. Thus, we used a ROPE of 20% between subjects SD [i.e., Cohen’s d standardized mean differences transformed to percent units (Hopkins et al., 2009; Kruschke, 2018)] to infer about the substantiality of our results. Also, we acknowledge that the use of a single day HRV records at pre and post-training period is in disagreement with recent statements suggesting a minimum of 3 randomly selected HRV measurements per week (Plews et al., 2014; Nakamura et al., 2020). Another limitation of the present study is that no objective external load measure was used. A recent prior study suggested that decelerations, number of sprints, and distance covered should be considered to better discriminate the physical load of elite futsal players (Ribeiro et al., 2020). Future studies should consider using larger samples and adding more objective measures to run multiple linear regressions to explain the variations in determinant fitness variables.
This study showed that those players who underwent 8 shuttle run HIIT sessions at 86% PSFIET had superior gains in aerobic, RSA, and neuromuscular performance measures than those who trained at 100% PSFIET during a typical 10-week training period. In addition, the variance explained by the TL along with the HIIT type was clearly larger for the changes in RSA performance outcomes than that observed for aerobic and neuromuscular performance changes. Finally, monitoring resting HRV could be a suitable tool to track changes in VO2peak, since temporal alterations in HRV are strongly related to VO2peak changes.
The raw data supporting the conclusions of this article will be made available by the authors, without undue reservation.
The studies involving human participants were reviewed and approved by this study was approved by the local research ethics committee (n° 93777318.0.0000.0121). Written informed consent to participate in this study was provided by the participants’ legal guardian/next of kin.
FC participated in the design of the study, data collection, data organization, and drafted the manuscript. FB participated in the data organization, and performed the statistical analysis, interpretation, and discussion of results. LF and LB contributed with support of materials and data collection. AT participated in the design of the study and interpretation and discussion of results. RHN contributed to the design of the study and interpretation of results. LG contributed to the design of the study, interpretation and discussion of results, and coordination of project. All authors contributed to the writing and approved the final version.
This study was financed by the Conselho Nacional de Desenvolvimento Científico e Tecnológico (CNPq) and the Coordenação de Aperfeiçoamento de Pessoal de Nível Superior – Brazil (CAPES) – Finance Code 001.
The authors declare that the research was conducted in the absence of any commercial or financial relationships that could be construed as a potential conflict of interest.
We would like to express our gratitude to all colleagues who contributed and to the subjects in this study and the team staff.
Akenhead, R., French, D., Thompson, K., and Hayes, P. (2014). The physiological consequences of acceleration during shuttle running. Int. J. Sports Med. 36, 302–307. doi: 10.1055/s-0034-1389968
Álvarez, J. C. B., D’ottavio, S., Vera, J. G., and Castagna, C. (2009). Aerobic fitness in Futsal players of different competitive level. J. Strength Cond. Res. 23, 2163–2166. doi: 10.1519/JSC.0b013e3181b7f8ad
Attene, G., Nikolaidis, P. T., Bragazzi, N. L., Dello Iacono, A., Pizzolato, F., Zagatto, A. M., et al. (2016). Repeated sprint ability in young basketball players (part 2): the chronic effects of multidirection and of one change of direction are comparable in terms of physiological and performance responses. Front. Physiol. 7:262. doi: 10.3389/fphys.2016.00262
Ayarra, R., Nakamura, F. Y., Iturricastillo, A., Castillo, D., and Yanci, J. (2018). Differences in physical performance according to the competitive level in Futsal players. J. Hum. Kinet. 64, 275–285. doi: 10.1515/hukin-2017-0201
Baker, J., Ramsbottom, R., and Hazeldine, R. (1993). Maximal shuttle running over 40 m as a measure of anaerobic performance. Br. J. Sports Med. 27, 228–232. doi: 10.1136/bjsm.27.4.228
Barbero-Alvarez, J. C., Soto, V. M., Barbero-Alvarez, V., and Granda-Vera, J. (2008). Match analysis and heart rate of Futsal players during competition. J. Sports Sci. 26, 63–73. doi: 10.1080/02640410701287289
Borg, E., and Borg, G. (2002). A comparison of AME and CR100 for scaling perceived exertion. Acta Psychol. (Amst.) 109, 157–175. doi: 10.1016/S0001-6918(01)00055-5
Bosco, C., Luhtanen, P., and Komi, P. V. (1983). A simple method for measurement of mechanical power in jumping. Eur. J. Appl. Physiol. Occup. Physiol. 50, 273–282. doi: 10.1007/BF00422166
Buchheit, M., Al Haddad, H., Laursen, P. B., and Ahmaidi, S. (2009). Effect of body posture on postexercise parasympathetic reactivation in men. Exp. Physiol. 94, 795–804. doi: 10.1113/expphysiol.2009.048041
Buchheit, M., and Laursen, P. B. (2013). High-intensity interval training, solutions to the programming puzzle. Sports Med. 43, 927–954. doi: 10.1007/s40279-013-0066-5
Buchheit, M., Millet, G. P., Parisy, A., Pourchez, S., Laursen, P. B., and Ahmaidi, S. (2008). Supramaximal training and postexercise parasympathetic reactivation in adolescents. Med. Sci. Sports Exerc. 40, 362–371. doi: 10.1249/mss.0b013e31815aa2ee
Bürkner, P.-C. (2017). brms: an R package for bayesian multilevel models using stan. J. Stat. Softw. 80, 1–28. doi: 10.18637/jss.v080.i01
Caetano, F. G., de Oliveira, M. J., Marche, A. L., Nakamura, F. Y., Cunha, S. A., and Moura, F. A. (2015). Characterization of the sprint and repeated-sprint sequences performed by professional futsal players, according to playing position, during official matches. J. Appl. Biomech. 31, 423–429. doi: 10.1123/jab.2014-0159
Caiozzo, V. J., Davis, J. A., Ellis, J. F., Azus, J. L., Vandagriff, R., Prietto, C. A., et al. (1982). A comparison of gas exchange indices used to detect the anaerobic threshold. J. Appl. Physiol. Respir. Environ. Exerc. Physiol. 53, 1184–1189. doi: 10.1152/jappl.1982.53.5.1184
Castagna, C., and Barbero Álvarez, J. C. (2010). Physiological demands of an intermittent Futsal-oriented high-intensity test. J. Strength Cond. Res. 24, 2322–2329. doi: 10.1519/JSC.0b013e3181e347b9
Castagna, C., D’Ottavio, S., Vera, J. G., and Álvarez, J. C. B. (2009). Match demands of professional Futsal: a case study. J. Sci. Med. Sport 12, 490–494. doi: 10.1016/j.jsams.2008.02.001
Clemente, F. M., Nikolaidis, P. T., Rosemann, T., and Knechtle, B. (2019). Dose-response relationship between external load variables, body composition, and fitness variables in professional soccer players. Front. Physiol. 10:443. doi: 10.3389/fphys.2019.00443
Cronin, J. B., and Hansen, K. T. (2005). Strength and power predictors of sports speed. J. Strength Cond. Res. 19, 349–357. doi: 10.1519/14323.1
da Silva, J. F., Nakamura, F. Y., Carminatti, L. J., Dittrich, N., Cetolin, T., and Guglielmo, L. G. A. (2015). The effect of two generic aerobic interval training methods on laboratory and field test performance in soccer players. J. Strength Cond. Res. 29, 1666–1672. doi: 10.1519/JSC.0000000000000776
Daniels, M., Highton, J., and Twist, C. (2019). Pre-season training responses and their associations with training load in elite Rugby league players. Sci. Med. Footb. 3, 313–319. doi: 10.1080/24733938.2019.1612529
De Freitas, V. H., Pereira, L. A., De Souza, E. A., Leicht, A. S., Bertollo, M., and Nakamura, F. Y. (2015). Sensitivity of the yo-yo intermittent recovery test and cardiac autonomic responses to training in Futsal players. Int. J. Sports Physiol. Perform. 10, 553–558. doi: 10.1123/ijspp.2014-0365
De Oliveira Bueno, M. J., Caetano, F. G., Pereira, T. J. C., De Souza, N. M., Moreira, G. D., Nakamura, F. Y., et al. (2014). Analysis of the distance covered by Brazilian professional futsal players during official matches. Sports Biomech. 13, 230–240. doi: 10.1080/14763141.2014.958872
Ellis, M., Penny, R., Wright, B., Noon, M., Myers, T., and Akubat, I. (2020). The dose–response relationship between training-load measures and aerobic fitness in elite academy soccer players. Sci. Med. Footb. 1–9. (in press). doi: 10.1080/24733938.2020.1817536
Esco, M., Flatt, A., and Nakamura, F. (2016). Initial weekly HRV response is related to the prospective change in VO2MAX in female soccer players. Int. J. Sports Med. 37, 436–441. doi: 10.1055/s-0035-1569342
Fanchini, M., Ferraresi, I., Modena, R., Schena, F., Coutts, A. J., and Impellizzeri, F. M. (2016). Use of the CR100 scale for session rating of perceived exertion in soccer and its interchangeability with the CR10. Int. J. Sports Physiol. Perform. 11, 388–392. doi: 10.1123/ijspp.2015-0273
Ferrari-Bravo, D., Impellizzeri, F., Rampinini, E., Castagna, C., Bishop, D., and Wisloff, U. (2008). Sprint vs. interval training in football. Int. J. Sports Med. 29, 668–674. doi: 10.1055/s-2007-989371
Foster, C., Florhaug, J. A., Franklin, J., Gottschall, L., Hrovatin, L. A., Parker, S., et al. (2001). A new approach to monitoring exercise training. J. Strength Cond. Res. 15, 109–115. doi: 10.1519/00124278-200102000-00019
Gelman, A., Goodrich, B., Gabry, J., and Vehtari, A. (2019). R-squared for bayesian regression models. Am. Stat. 73, 307–309. doi: 10.1080/00031305.2018.1549100
Gelman, A., Jakulin, A., Pittau, M. G., and Su, Y. S. (2008). A weakly informative default prior distribution for logistic and other regression models. Ann. Appl. Stat. 2, 1360–1383. doi: 10.1214/08-AOAS191
Gelman, A., Lee, D., and Guo, J. (2015). Stan. J. Educ. Behav. Stat. 40, 530–543. doi: 10.3102/1076998615606113
Hopkins, W. G., Marshall, S. W., Batterham, A. M., and Hanin, J. (2009). Progressive statistics for studies in sports medicine and exercise science. Med. Sci. Sport. Exerc. 41, 3–13. doi: 10.1249/MSS.0b013e31818cb278
Kadane, J. B. (2015). Bayesian methods for prevention research. Prev. Sci. 16, 1017–1025. doi: 10.1007/s11121-014-0531-x
Kruschke, J. K. (2018). Rejecting or accepting parameter values in bayesian estimation. Adv. Methods Pract. Psychol. Sci. 1, 270–280. doi: 10.1177/2515245918771304
Kuipers, H., Rietjens, G., Verstappen, F., Schoenmakers, H., and Hofman, G. (2003). Effects of stage duration in incremental running tests on physiological variables. Int. J. Sports Med. 24, 486–491. doi: 10.1055/s-2003-42020
Maggioni, M. A., Bonato, M., Stahn, A., La Torre, A., Agnello, L., Vernillo, G., et al. (2019). Effects of ball drills and repeated-sprint-ability training in Basketball players. Int. J. Sports Physiol. Perform. 14, 757–764. doi: 10.1123/ijspp.2018-0433
McGinley, C., and Bishop, D. J. (2016). Influence of training intensity on adaptations in acid/base transport proteins, muscle buffer capacity, and repeated-sprint ability in active men. J. Appl. Physiol. 121, 1290–1305. doi: 10.1152/japplphysiol.00630.2016
McLellan, T. (1985). Ventilatory and plasma lactate response with different exercise protocols: a comparison of methods. Int. J. Sports Med. 6, 30–35. doi: 10.1055/s-2008-1025809
Morey, R. D., Hoekstra, R., Rouder, J. N., Lee, M. D., and Wagenmakers, E.-J. (2016). The fallacy of placing confidence in confidence intervals. Psychon. Bull. Rev. 23, 103–123. doi: 10.3758/s13423-015-0947-8
Nakamura, F. Y., Antunes, P., Nunes, C., Costa, J. A., Esco, M. R., and Travassos, B. (2020). Heart rate variability changes from traditional vs. ultra–short-term recordings in relation to preseason training load and performance in Futsal players. J. Strength Cond. Res. 34, 2974–2981. doi: 10.1519/JSC.0000000000002910
Oliveira, R. S., Leicht, A. S., Bishop, D., Barbero-Álvarez, J. C., and Nakamura, F. Y. (2013). Seasonal changes in physical performance and heart rate variability in high level futsal players. Int. J. Sports Med. 34, 424–430. doi: 10.1055/s-0032-1323720
Pedro, R. E., Milanez, V. F., Boullosa, D. A., and Nakamura, F. Y. (2013). Running speeds at ventilatory threshold and maximal oxygen consumption discriminate futsal competitive level. J. Strength Cond. Res. 27, 514–518. doi: 10.1519/JSC.0b013e3182542661
Plews, D. J., Laursen, P. B., Le Meur, Y., Hausswirth, C., Kilding, A. E., and Buchheit, M. (2014). Monitoring training with heart-rate variability: how much compliance is needed for valid assessment? Int. J. Sports Physiol. Perform. 9, 783–790. doi: 10.1123/IJSPP.2013-0455
R Core Team (2020). R: A Language and Environment for Statistical Computing. Vienna: R Foundation for Statistical Computing. Available online at: https://www.R-project.org/
Rabbani, A., Kargarfard, M., Castagna, C., Clemente, F. M., and Twist, C. (2019). Associations between selected training-stress measures and fitness changes in male soccer players. Int. J. Sports Physiol. Perform. 14, 1050–1057. doi: 10.1123/ijspp.2018-0462
Ribeiro, J. N., Gonçalves, B., Coutinho, D., Brito, J., Sampaio, J., and Travassos, B. (2020). Activity profile and physical performance of match play in elite Futsal players. Front. Psychol 11:1709. doi: 10.3389/fpsyg.2020.01709
Sanchez-Sanchez, J., Carretero, M., Ramirez-Campillo, R., Petisco, C., Diego, M., Gonzalo-Skok, O., et al. (2018). Effects of high-intensity training with one versus three changes of direction on youth female basketball players’ performance. Kinesiology 50, 117–125.
Seiler, S., Jøranson, K., Olesen, B. V., and Hetlelid, K. J. (2013). Adaptations to aerobic interval training: interactive effects of exercise intensity and total work duration. Scand. J. Med. Sci. Sports 23, 74–83. doi: 10.1111/j.1600-0838.2011.01351.x
Task Force (1996). Heart rate variability: standards of measurement, physiological interpretation and clinical use. task force of the European society of cardiology and the North American society of pacing and electrophysiology. Circulation 93, 1043–1065. doi: 10.1161/01.CIR.93.5.1043
Taylor, R. J., Sanders, D., Myers, T., Abt, G., Taylor, C. A., and Akubat, I. (2018). The dose-response relationship between training load and aerobic fitness in academy rugby union players. Int. J. Sports Physiol. Perform. 13, 163–169. doi: 10.1123/ijspp.2017-0121
Teixeira, A. S., Arins, F. B., de Lucas, R. D., Carminatti, L. J., Dittrich, N., Nakamura, F. Y., et al. (2018). Shuttle-run interval training with more directional changes induces superior gains in shuttle sprint performance in female professional Futsal players. Hum. Mov. 2018, 40–51. doi: 10.5114/hm.2018.79623
Teixeira, A. S., Arins, F. B., De Lucas, R. D., Carminatti, L. J., Dittrich, N., Nakamura, F. Y., et al. (2019). Comparative effects of two interval shuttle-run training modes on physiological and performance adaptations in female professional Futsal players. J. Strength Cond. Res. 33, 1416–1428. doi: 10.1519/JSC.0000000000002186
Keywords: sports, physical performance, high intensity interval training, shuttle-run, sprint
Citation: Campos FS, Borszcz FK, Flores LJF, Barazetti LK, Teixeira AS, Hartmann Nunes RF and Guglielmo LGA (2021) HIIT Models in Addition to Training Load and Heart Rate Variability Are Related With Physiological and Performance Adaptations After 10-Weeks of Training in Young Futsal Players. Front. Psychol. 12:636153. doi: 10.3389/fpsyg.2021.636153
Received: 01 December 2020; Accepted: 04 January 2021;
Published: 22 January 2021.
Edited by:
Bruno Travassos, University of Beira Interior, PortugalReviewed by:
Júlio Alejandro Costa, Portuguese Football Federation, PortugalCopyright © 2021 Campos, Borszcz, Flores, Barazetti, Teixeira, Hartmann Nunes and Guglielmo. This is an open-access article distributed under the terms of the Creative Commons Attribution License (CC BY). The use, distribution or reproduction in other forums is permitted, provided the original author(s) and the copyright owner(s) are credited and that the original publication in this journal is cited, in accordance with accepted academic practice. No use, distribution or reproduction is permitted which does not comply with these terms.
*Correspondence: Fernando Klitzke Borszcz, ZmVybmFuZG9ib3JzemN6QGdtYWlsLmNvbQ==; Lucinar Jupir Forner Flores, bHVjaW5hcjA1QGdtYWlsLmNvbQ==; Luiz Guilherme Antonacci Guglielmo, bHVpei5ndWlsaGVybWVAdWZzYy5icg==
Disclaimer: All claims expressed in this article are solely those of the authors and do not necessarily represent those of their affiliated organizations, or those of the publisher, the editors and the reviewers. Any product that may be evaluated in this article or claim that may be made by its manufacturer is not guaranteed or endorsed by the publisher.
Research integrity at Frontiers
Learn more about the work of our research integrity team to safeguard the quality of each article we publish.