- 1School of Education, Beijing Open University, Beijing, China
- 2School of Foreign Languages, Renmin University of China, Beijing, China
In the education context, The Motivational Strategies for Learning Questionnaire (MSLQ) is extensively used in assessing self-regulated learning strategies. However, more research is needed to address whether it is applicable for distance education. The exploratory and confirmatory factor analysis were used to test the Chinese version of the Motivated Strategies for Learning Questionnaire part-B for distance learning (MSLQ-B-DL) using two samples totalling 385 participants. This paper substantiates MSLQ-B-DL's criterion-related, convergent, and factorial validity, as well as its internal consistency, in China. Specifically, the concurrent validity of the MSLQ-B-DL was shown from three aspects: (a) the negative correlation of MSLQ-B-DL with trait procrastination; (b) the positive correlation of MSLQ-B-DL with self-control; and (c) the positive correlation of MSLQ-B-DL with instrumental help-seeking and the former's negative correlation with help-seeking avoidance and executive help-seeking. Finally, this study highlights the MSLQ-B-DL's validity and reliability in evaluating the learning strategies in adult distance education in China.
Introduction
Distance learning is the education of students who may not always be physically present at a school. Distinct from face-to-face education, distance learning requires students to adopt self-regulated learning (SRL) behaviors (Adam et al., 2017). As a result, distance learning is considered to improve learners' motivation and their academic performance (Hartnett, 2016). Over the past 30 years, research has proposed various theoretical models to explain the process of self-regulated learning. Until recently, there were six theoretical models describing self-regulated learning: models from Zimmerman (2000), Boekaerts (2011), Winne and Hadwin (2008), Pintrich (2000), Efklides (2011), and Hadwin et al. (2018).
The researcher Panadero (2017) conducted a comparative study of the six models listed above and found that the models from Pintrich (2000) and Zimmerman (2000) are the most popular. They are similar in terms of the elements of self-regulation, and the role of context. However, Pintrich's model is more comprehensive, easier to understand, and more applicable to the classroom setting (Dignath and Büttner, 2008) than Zimmerman's model. It contains a complete description of different types of sub-processes and proposes more specific sub-processes, including motivation and strategies. Pintrich's model emphasizes the difference between each stage and its sub-processes, believing that each stage has unique characteristics. Besides, it treats motivational process an independent variable which interacted with cognitive, behavioral, and contextual factors to affect self-learning (Pintrich et al., 1993a). According to this model, more specific intervention measures can be proposed. Moreover, it focuses more on metacognition and is utilized in investigating the target orientation's function in SRL (Pintrich and de Groot, 1990). For these reasons, this study is based on Pintrich's model. For these reasons, this study is based on Pintrich's model in order to facilitate research comparison and theory correction.
According to theoretical and empirical research, learning strategies are important predictors of learning performance in the online environment. Learning strategies are processes that involve generating, organizing, or converting data for academic achievement (Neroni et al., 2019). SRL strategies refer to the application of resource management and cognitive strategies to supervise learning (Panadero, 2017). Research shows that there is a positive relationship between self-regulated learning strategies in the distance learning environment and academic performance (Broadbent and Poon, 2015; Broadbent, 2017). Studies on distance learning tend to focus on domain-general strategies and have found that students use these self-regulated strategies to benefit their academic achievement (e.g., Lin et al., 2017). However, students practice distinct forms of learning strategies to fulfill various academic goals (Neroni et al., 2019). In the context of academic performance, extensive research has explored the most effective as well as the unfavorable learning strategies (e.g., Matcha et al., 2019). Nevertheless, the majority of these studies were performed on undergraduate students on campus, and few explore the issue in the context of distance education.
Determining which learning strategies deliver the most optimal benefits for academic performance is vital for students and their teachers who can provide valuable support accordingly (Donker et al., 2013). Different classifications of learning strategies have been developed over time. For example, learning strategies can be classified into resource management, metacognitive, and cognitive strategies (McKeachie et al., 1987).
The Motivational Strategies for Learning Questionnaire (MSLQ) is a widely used tool to measure learning strategies used by students because of its good psychometric properties for different samples (Jackson, 2018; Soemantri et al., 2018). There are two versions of the MSLQ that have been widely translated into different languages and used in research: one version is for college students and consists of 15 subscales and 81 items (Pintrich et al., 1993b), and the other version is for junior high students and consists of five subscales and 44 items (Pintrich and de Groot, 1990). For young students, both the full version and specific subscales are applicable. For example, Pintrich and de Groot (1990) examined the motivational factors (internal value, self-efficacy, and test anxiety) and self-regulated learning factors (strategy use and self-regulation) in a sample of young students. The MSLQ has been translated and applied in many countries, such as Australia (Martin and Marsh, 2006), China (Yang et al., 2012; Tong et al., 2019), and Egypt (Shawer, 2013).
Through the use of the MSLQ in many studies, two concerns have been raised. First, social cognitive theory proposes that students' motivation and learning strategies are context-specific. However, the MSLQ is designed for all subjects and all kinds of learning activities, failing to account for context. Second, psychometrics problems have arisen in some studies, where it has been found, for example, that the partial molecular scale of the MSLQ lacks discriminant validity and that the factor structure of latent variables is not stable (Hilpert et al., 2013). In addition, in a study using the MSLQ in a sample of 611 college students, exploratory factor analysis showed that for Chinese adult learners, the factor of internal goal orientation disappears, the factor of peer learning is not very stable, and the factor of time and environment management is actually two factors (Tong et al., 2019).
There are relatively few empirical studies on distance learning strategies. Each scale has different measurement standards, Kizilcec et al. (2016) believe that it is not practical to create a new questionnaire to measure existing concepts or to use a complete but lengthy scale in an attempt to obtain high quality answers. Moreover, each study is based on a unified understanding of the construct and structure of learning strategies, without considering the difference between traditional learners and distance learners.
Recently, researchers have used the MSLQ to measure students' self-regulated learning in an online environment. Based on these studies, one option is that distance education studies could use either the MSLQ's subscale of learning strategies or a combination of items from the MSLQ. Cho and Summers (2012) used the complete version of the MSLQ to study learning strategies in distance education, but found that the structure did not fit the sample well. Because the MSLQ was originally developed for traditional face-to-face education, it is possible that many items do not directly reflect the learning behaviors of distance learners. Recognizing this possibility, Meijs et al. (2019) revised the subscales of the MSLQ to conform to distance education. Factor analysis of the new learning strategy questionnaire for distance education (MSLQ-B-DL) revealed five factors. Further research (Neroni et al., 2019) proved the stability, reliability, and validity of its factor structure.
In line with this context, this paper aims to ensure the validity and reliability of MSLQ-B-DL's Chinese version (Meijs et al., 2019). Specifically, this study will investigate factor validity, convergence validity, internal consistency, and criterion-related validity of the MSLQ-B-DL. For the reasons stated below, convergence validity will be investigated by calculating the correlation between distance learning strategies and procrastination, self-control, and help-seeking behaviors; criterion-related validity will be examined by calculating the correlation between distance learning strategies and academic performance.
Construct Validity
As for construct validity, this study examines three constructs that are related to distance learning strategies: procrastination, self-control, and help-seeking behavior.
Procrastination
Achievement goal theory suggests that one result of adopting goal orientation is the differential use of learning strategies (Pintrich et al., 2000). Therefore, learning strategies are related to self-regulated learning and procrastination. Since the use of learning strategies consumes energy and time, students with little motivation for distant tasks may not apply learning strategies frequently. Schouwenburg (2004) proposed that procrastination is negatively correlated with the systematic and disciplined approach to word as well as the planning and management of time, which were all manifestations of poor organization. As a part of conscientiousness in an individual's personality, organization is negatively correlated with procrastination (Steel, 2007). Procrastination is linked to the reduced application of metacognitive and cognitive strategies (Limone et al., 2020). Therefore, this study hypothesizes that distance learning strategies are negatively correlated with procrastination.
Self-Control
Self-control is related to a broad spectrum of behaviors. Empirical studies have demonstrated that people with high self-control are more skilled in emotion regulation as well as thought and impulse control than their low self-control counterparts (Robson et al., 2020).
An essential mediating variable between perceived ability and learning engagement could be the perceived academic control, which describes a person's perception of his or her capacity to impact and forecast academic performance (Respondek et al., 2020). In the former perspective, ability can be improved by learning and effort, which promotes a higher sense of academic control. Therefore, this study hypothesizes that distance learning strategies are positively correlated with self-control.
Help-Seeking Behavior
Help-seeking behavior, as opposed to dependence, is regarded as an important self-regulated learning strategy (Karabenick and Gonida, 2018). This recognition began by distinguishing instrumental help-seeking behavior (Sideridis and Stamovlasis, 2016), which involved help-seekers' requests for others to explain problem-solving method. By contrast, learners with executive help-seeking requested for others to solve problem for them. And learners with avoidance of help-seeking did not seek help. Thus, in the context of distance learning strategies, this study theorizes their (a) negative correlation with help-seeking avoidance and executive help-seeking, and (b) positive correlation with instrumental help-seeking.
Criterion-Related Validity
For criterion-related validity, this study adopted academic performance in the course to examine the predictive criterion-related validity of the scale. A study that used the MSLQ with Chinese adult learners suggested that future studies use academic performance to verify the predictive validity of the MSLQ (Tong et al., 2019). In distance learning, time management learning strategies, metacognitive strategies, and effort regulation strategies can improve academic performance (Broadbent and Poon, 2015). These approaches assist learners in processing and storing information systematically (Dignath and Büttner, 2008). The usage of the learning strategies aims at improving academic performance. Therefore, this study hypothesizes that distance learning strategies are positively related to academic performance.
Methods
Translation
Two graduate students majored in English translated the original MSLQ-B-DL (Meijs et al., 2019) into Chinese independently. The “translate and back translate” procedure was used to get a readable Chinese expression of the same meaning.
Participants and Procedures
There were 150 participants (113 women) in sample A, who were recruited from an adult distance learning course entitled “Psychology.” It's a self-paced course of the undergraduate degree program from an open university in China. All participants provided informed consent and were financially compensated for their participation. All participants took the course in the autumn of 2018, filled out e-questionnaires at the beginning of the semester. One hundred and fifty eight people participated in the survey, 150 of whom were valid, with a 95% response rate. The academic ethics committee of the first author's institution reviewed and approved this study. The data from sample A were used in the exploratory factor analysis (EFA).
There were 236 participants (143 women) in sample B. All participants studied the course in the spring semester of 2019, filled in questionnaires at the beginning of the semester, and took part in the course examination at the end. Two hundred and forty one people filled in the questionnaire, 5 of whom were absent from the exam, with a response rate of 98%. The data from sample B were used in the confirmatory factor analysis (CFA) and validity test. The participants' characteristics for both samples are presented in Table 1.
Concerning the sample size, the N:p ratio is used in EFA, i.e., of participants (N) to variables (p) set traditionally to 5:1 (Kyriazos, 2018). A CFA rule of thumb is the ratio of cases to free parameters, or N:q is commonly used for minimum recommendations and 10:1–20:1 is a commonly suggested ratio (Schumacker and Lomax, 2015).
Measures
MSLQ-B-DL
The MSLQ-B-DL (Meijs et al., 2019), which was adapted from the MSLQ, measures the learning strategies of distance learners through 25 items. A 6-point Likert scale was used in both samples (1 = strongly disagree, 6 = strongly agree), with higher scores representing better use of learning strategies. The MSLQ-B-DL had acceptable reliability in this sample (see the “Result” section). Both English and Chinese versions of the scale are presented in Appendix 1.
General Procrastination Scale (GPS)
The General Procrastination Scale (Sirois et al., 2019) measures trait procrastination and contains 9 items. A 6-point Likert scale was used in both samples (1 = strongly disagree, 6 = strongly agree), with higher scores representing higher levels of procrastination tendency. The GPS had excellent reliability with Cronbach's α as 0.83 in sample B.
Self-Control
The self-control scale (Tan and Guo, 2008) measures the self-control ability of university students and consists of 19 items. A 6-point Likert scale was used in both samples (1 = strongly disagree, 6 = strongly agree), with higher scores representing higher levels of self-control ability. This scale was reliable with Cronbach's α as 90 in sample B.
Help-Seeking Behavior
The help-seeking behavior scale (Tang, 2004) contains 14 items, and includes three dimensions: instrumental help-seeking, executive help-seeking, and help-seeking avoidance. A 6-point Likert scale was used in both samples (1 = strongly disagree, 6 = strongly agree). Higher scores for instrumental help-seeking represent more positive aspects of help-seeking behavior. Higher scores for executive help-seeking and help-seeking avoidance represent more negative aspects of help-seeking behavior. The math learning terms from the original scale were replaced with “online learning.” For example, “for me, online learning will be easier with the help of others.” The Cronbach's α with the sample B was 0.82.
Academic Performance
Fifty questions were selected from the course database to form the knowledge test, ensuring that questions were drawn from all of the course units. All questions were single choice questions. If the answer was correct, the participant earned 2 points. The scores ranged from 36 to 98, with an average score of 76.91 (SD = 11.64).
Demographic Variables
Demographic variables included gender, age, and online learning experience.
Data Analysis
Explorative factor analysis, descriptive analysis and correlations were used with SPSS 21.0. Confirmative factor analysis was used with AMOS 24.0. McDonald ω and Cronbach's α was calculated with Jamovi 1.6.9.
Results
Exploratory Factor Analysis (EFA)
First, factor validity was analyzed using sample A (N = 150), and the critical ratio (CR) and discrimination index (D) were used to analyse 25 items. The t-tests of the 25 items were all significant, and the correlations of the 25 items ranged from 0.67 to 0.89.
Construct validity was evaluated using the EFA, also with sample A. This paper adopted Bartlett's test of sphericity and the Kaiser-Meyer-Olkin (KMO) index to assess the sampling adequacy. The sample was found adequate based on the KMO value at 0.87, which was > 0.5 (Kaiser, 1974), and the statistically significant Bartlett's test of sphericity value [χ2(190) = 2106.35, p < 0.001]. Through a screen plot and the Promax rotation, we extracted the EFA's latent factors by maximum likelihood. As shown in Table 2, there were five factors with eigenvalues above 1 (range from 1.03 to 8.31), and these five factors explained 73.37% of the total variance of the 25 items. The McDonald's ω is 0.85. The composite Cronbach's α is 0.93. The average variance extracted (AVE) range from 0.45 to 0.66. The composite reliability (CR) ranges from 0.68 to 0.94. Only the Time management factor's AVE value is 0.45 which is < 0.50. And its composite reliability (CR) value is 0.71. If AVE is <0.5, but composite reliability is higher than 0.6, the convergent validity of the construct is still adequate (Fornell and Larcker, 1981).
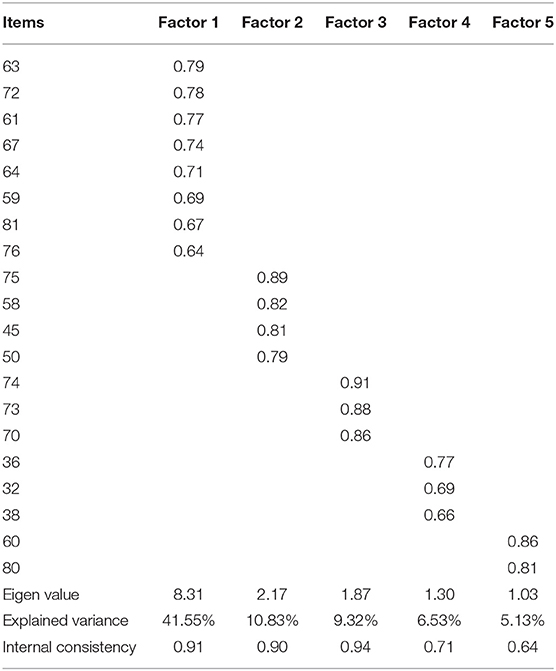
Table 2. Standardized factor loading of exploratory factor analysis and internal consistency with Sample A.
Five items (items 71, 43, 46, 47, and 51) were deleted from the eventual final questionnaire because the loadings were lower than 0.4, or cross-loading on more than one factor and the loadings were above 0.3. The loadings of the remaining 20 items were all above 0.50, among which the loadings of 17 items were above 0.70.
Confirmatory Factor Analysis (CFA)
The CFA was conducted on sample B, using AMOS 24.0. As shown in Table 3, model fit was measured using the Satorra-Bentler scaled chi-square (χ2 = 370.315), comparative fit index (CFI = 0.93), Tucker-Lewis index (TLI = 0.92), and root mean square error of approximation (RMSEA = 0.07). The goodness-of-fit indices of the CFA model were acceptable (Baloglu, 2016).
Based on the items in the five factors, the factors were named as time management, effort regulation, cognitive strategy, critical thinking, and help seeking (see Figure 1).
The original time and environment management factor is divided into two factors: time management and effort regulation. The time management factor has three items. Item 74 (Despite the dull and unexciting course materials, I can continue working until I complete the tasks) belongs to effort regulation in the original questionnaire. However, in this study, participants paid attention to the deadline in the item. As a result, this item was considered to be related to time management factor. Distance learners may invest different resources and energies on time management and effort regulation than traditional students in a classroom setting. For example, adult online learners arrange their learning time in a highly autonomy. Besides, two of the reverse scoring items constitute the effort regulation factor.
The cognitive strategy factor constitutes of complex cognitive strategy and single cognitive strategy in the original MSLQ-B-DL (Davis et al., 2018). The eight items in subscale of the self-learning are also covered by the all the items of the complex and simple cognitive strategies in this study.
Critical thinking factor includes the original items, and another item (32: As I revise the course readings, I organize my thoughts by outlining the material) comes from the single cognitive strategy.
The fifth factor is help seeking. Four items in this factor were consistent with the original questionnaire. Help seeking is different from other learning strategies in that it is also social interaction. It can be defined as the process of seeking assistance from other individuals or other sources that facilitate accomplishing desired goals, which in academic context may consist of completing assignments or satisfactory test performance (Karabenick and Gonida, 2018).
Internal Consistency and Convergent Validity
The Cronbach's alpha was calculated to evaluate the internal consistency of scores on the five factors. Cronbach's alpha estimates ranged from 0.64 to 0.94 for sample A, and from 0.71 to 0.94 for sample B.
Bivariate correlations between sample B's scores on the MSLQ-B-DL factors and scores on procrastination, self-control, and help-seeking behavior are displayed in Table 4. As hypothesized, correlations between the five factors of MSLQ-B-DL and procrastination were significant and negative. The correlations ranged from −0.18 to −0.49. Self-control was significantly correlated with cognitive strategy, help seeking, critical thinking, effort regulation, and the total score of MSLQ-B-DL. The relationships between instrumental help-seeking behavior and the five factors in MSLQ-B-DL were significant and positive. The analysis found (a) the significant and negative correlation of executive help-seeking behavior with effort regulation, and (b) the significant and negative correlation of help-seeking avoidance with effort regulation and the total MSLQ-B-DL score. These results provide strong convergent validity support for the MSLQ-B-DL.
Criterion-Related Validity
Pearson correlations among gender, age, online learning experience, MSLQ-B-DL, and academic performance are shown in Table 5. Gender was negatively correlated with academic performance, indicating that male participants had better performance than female participants. Online learning experience was positively correlated with academic performance, indicating that academic performance increased with online learning experience. The total score of MSLQ-B-DL was positively related to academic performance, indicating that better use of online learning strategies improved performance.
The results from regressing gender, age, online learning experience, and MSLQ-B-DL on academic performance are shown in Table 6. When gender, age, and online learning experience entered the model first, gender (β = −4.097, p < 0.01) and experience (β = 2.27, p < 0.05) were related to academic performance. When the total score of MSLQ-B-DL entered the model in the second step, gender remained significantly related to academic performance (β = −2.071, p < 0.01), and MSLQ-B-DL was significantly related to academic performance (β = 15.47, p < 0.001). In sum, the correlation and regression analyses provide support for the criterion validity of the MSLQ-B-DL.
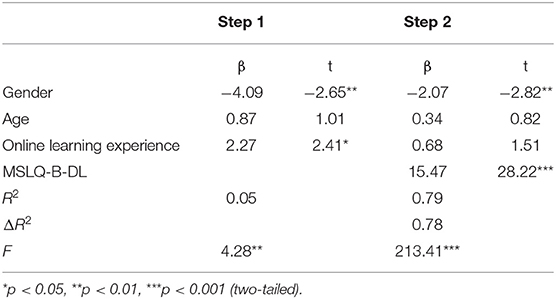
Table 6. Hierarchical regression analyses with gender, age, and online learning experience entered at step 1, and total score of MSLQ-B-DL Entered at Step 2.
Discussion
This study involves a total of 385 participants (129 men, and 256 women) in two different samples. This research demonstrates that the MSLQ-B-DL is a useful tool to measure online learning strategies of adult distance learners in China. The factorial validity of the MSLQ-B-DL was good. Moreover, the MSLQ-B-DL's factor structure, similar to its original version, was presented in the EFA results (Meijs et al., 2019). The results of the CFA demonstrate that the data fit well with the factor structure and the items' loadings are acceptable. The internal consistency indices of each factor in the two samples reach the appropriate standards, which are similar to the original MSLQ-B-DL. The MSLQ-B-DL has good convergence validity. The convergence validity criteria are procrastination, self-control, and help-seeking behavior. The total score of MSLQ-B-DL is negatively correlated with procrastination, executive help-seeking behavior, and help-seeking avoidance. Additionally, it is positively correlated with self-control and executive help-seeking behavior. This study also examined the correlation between adult distance learning strategy and academic performance. The Chinese version of the MSLQ-B-DL can distinguish between high and low performance, indicating that the questionnaire has acceptable predictive validity. The ability to measure differences is useful for research and practice, and for developing appropriate interventions for self-regulation.
To summarize, it is very necessary to emphasize students' self-regulated learning in distance education. It is of great significance to guide students to formulate personalized learning goals according to their own actual conditions. The previous MSLQ survey targets at traditional college students, which is not suitable for adult distance learners, who can determine their own time, place and learning pace. The newly developed questionnaire is based on a based on a mature questionnaire, and adapted to adult distance learners' online learning environment and learners' characteristics. The development of measurement tools in Chinese version not only contributes to the comparability of research results across cultures, but also has a guiding role for students who study independently on the Internet.
The Chinese version of the scale is different from the original scale in terms of two factors and items. Firstly, time management and effort management become two factors. The result is consistent with the Spain version (Ramírez-Dorantes et al., 2013) and Chinese version (Tong et al., 2019) of the MSLQ. Reverse scoring items constitutes the effort regulation factor, which is consistent with a previous study. Chinese subjects tend to be more careful when answering reverse scoring questions (Law et al., 2008). The self-regulated learning theory asserts that learners will invest their efforts flexibly to learn better (Panadero, 2017). The effort regulation strategy is one of the most important self-regulated learning strategies for online learners (Kizilcec et al., 2016). In addition, an earlier study on the online learning environment found that effort regulation strategy was positively correlated with academic performance (Broadbent and Poon, 2015). In an online learning environment, one of the reasons for learners' success is their persistence in the face of distractions or obstacles when watching videos or performing boring tasks (Lee et al., 2019). Secondly, the simple and complex cognitive strategies combine to cognitive strategy factor. As suggested by the slow progress of research on interventions for the complex cognitive strategy, it is complex. Additionally, it is difficult to distinguish the complex strategy from other cognitive strategies, particularly in large-scale samples. Consistent with prior research (Kocdar et al., 2018) on self-regulated learning in open and distributed distance education, self-regulated learning strategies include self-learning, goal setting, help-seeking, environment management, and effort regulation strategies.
The COVID-19 pandemic has resulted in schools shut all across the world. Education has changed dramatically, with the distinctive rise of online learning, whereby teaching is undertaken remotely and on digital platforms. The integration of information technology in education will be further accelerated and that online education will eventually become an integral component of school education. The lack of structured in-class learning settings may have required more self-regulation and self-motivation to learn with less support. Given these challenges for educators, students, and parents, this study investigated the reliability and validity of self-regulated learning strategy measurement. It contributed to monitor students' use of learning strategies during online learning. Parents and teachers can provide timely guidance to ensure the effectiveness of learning.
There are some limitations in this study. First, the reliability and validity of the questionnaire needs to be further tested in different language and cultures. Second, the cross-sectional research design would not draw causality conclusions. Third, all the variables are self-reporting. The participants may overestimate theirs usage of strategies and introduce bias.
Conclusion
With the popularization and application of online learning, the use of a localized perspective to understand the phenomenon of online learning is of great significance to online education research. This study examined the reliability and validity of Chinese version of the MSLQ-B-DL. For Chinese adult distance learners, this revised 20-item Chinese version of the questionnaire can effectively assess the quality of their learning strategies and lay the foundation for predicting their learning performance. Future research can be carried out in social science courses and STEM courses, respectively, to further verify the reliability and validity of the questionnaire.
Data Availability Statement
The raw data supporting the conclusions of this article will be made available by the authors, without undue reservation.
Ethics Statement
The studies involving human participants were reviewed and approved by Ethics Committee of Beijing Open University. The patients/participants provided their written informed consent to participate in this study.
Author Contributions
Both authors listed have made a substantial, direct and intellectual contribution to the work, and approved it for publication.
Funding
This research was supported by the National Social Science Foundation of China (19BYY103).
Conflict of Interest
The authors declare that the research was conducted in the absence of any commercial or financial relationships that could be construed as a potential conflict of interest.
Supplementary Material
The Supplementary Material for this article can be found online at: https://www.frontiersin.org/articles/10.3389/fpsyg.2021.620564/full#supplementary-material
References
Adam, N. L., Alzahri, F. B., Cik Soh, S., Abu Bakar, N., and Mohamad Kamal, N. A. (2017). “Self-regulated learning and online learning: a systematic review,” in Advances in Visual Informatics. IVIC 2017. Lecture Notes in Computer Science, ed H. Badioze Zaman (Cham: Springer), 143–154. doi: 10.1007/978-3-319-70010-6_14
Baloglu, M. (2016). The impact of cultural collectivism on knowledge sharing among information technology majoring undergraduates. Comput. Hum. Behav. 56, 65–71. doi: 10.1016/j.chb.2015.11.031
Boekaerts, M. (2011). “Emotions, emotion regulation, and self-regulation of learning,” in Educational Psychology Handbook Series. Handbook of Self-Regulation of Learning and Performance, eds B. J. Zimmerman and D. H. Schunk (New York, NY: Routledge), 408–425.
Broadbent, J. (2017). Comparing online and blended learner's self-regulated learning strategies and academic performance. Int. High. Educ. 33, 24–32. doi: 10.1016/j.iheduc.2017.01.004
Broadbent, J., and Poon, W. (2015). Self-regulated learning strategies & academic achievement in online higher education learning environments: a systematic review. Int. High. Educ. 27, 1–13. doi: 10.1016/j.iheduc.2015.04.007
Cho, M. H., and Summers, J. (2012). Factor validity of the motivated strategies for learning questionnaire (MSLQ) in asynchronous online learning environments (AOLE). J. Interact. Learn. Res. 23, 5–28.
Davis, D., Chen, G., Hauff, C., and Houben, G. J. (2018). Activating learning at scale: a review of innovations in online learning strategies. Comput. Educ. 125, 327–344. doi: 10.1016/j.compedu.2018.05.019
Dignath, C., and Büttner, G. (2008). Components of fostering self-regulated learning among students. A meta-analysis on intervention studies at primary and secondary school level. Metacogn. Learn. 3, 231–264. doi: 10.1007/s11409-008-9029-x
Donker, A. S., de Boer, H., Kostons, D., Dignath van Ewijk, C. C., and van der Werf, M. P. C. (2013). Effectiveness of learning strategy instruction on academic performance: a meta-analysis. Educ. Res. Rev. 11, 1–26. doi: 10.1016/j.edurev.2013.11.002
Efklides, A. (2011). Interactions of metacognition with motivation and affect in self-regulated learning: the MASRL model. Educ. Psychol. 46, 6–25. doi: 10.1080/00461520.2011.538645
Fornell, C., and Larcker, D. F. (1981). Structural equation models with unobservable variables and measurement error: algebra and statistics. J. Market. Res. 18, 382–388. doi: 10.1177/002224378101800313
Hadwin, A., Järvelä, S., and Miller, M. (2018). “Self-regulation, co-regulation and shared regulation in collaborative learning environments,” in Educational Psychology Handbook Series. Handbook of Self-Regulation of Learning and Performance, eds D. H. Schunk and J. A. Greene (New York, NY: Routledge), 83–106. doi: 10.4324/9781315697048-6
Hartnett, M. (ed.). (2016). “The importance of motivation in online learning,” in Motivation in Online Education (Singapore: Springer), 5–32. doi: 10.1007/978-981-10-0700-2
Hilpert, J., Stempien, J., van der Hoeven Kraft, K., and Husman, J. (2013). Evidence for the latent factor structure of the MSLQ: a new conceptualization of an established questionnaire. SAGE Open. 3:21582440135. doi: 10.1177/2158244013510305
Jackson, C. R. (2018). Validating and adapting the motivated strategies for learning questionnaire (MSLQ) for STEM courses at an HBCU. AERA Open. 4, 1–16. doi: 10.1177/2332858418809346
Kaiser, H. F. (1974). An index of factorial simplicity. Psychometrika 39, 31–36. doi: 10.1007/BF02291575
Karabenick, S. A., and Gonida, E. N. (2018). “Academic help seeking as a self-regulated learning strategy: Current issues, future directions,” in Educational Psychology Handbook Series. Handbook of Self-Regulation of Learning and Performance, eds D. H. Schunk and J. A. Greene (New York, NY: Routledge), 421–433. doi: 10.4324/9781315697048-27
Kizilcec, R., Pérez-Sanagustín, M., and Maldonado, J. (2016). Self-regulated learning strategies predict learner behavior and goal attainment in massive open online courses. Comput. Educ. 104, 18–33. doi: 10.1016/j.compedu.2016.10.001
Kocdar, S., Karadeniz, A., Bozkurt, A., and Buyuk, K. (2018). Measuring self-regulation in self-paced open and distance learning environments. Int. Rev. Res. Open Distrib. Learn. 19, 24–43. doi: 10.19173/irrodl.v19i1.3255
Kyriazos, T. A. (2018). Applied psychometrics: sample size and sample power considerations in fac-tor analysis (EFA, CFA) and SEM in gen-eral. Psychology 9, 2207–2230. doi: 10.4236/psych.2018.98126
Law, Y., Chan, C. K. K., and Sachs, J. (2008). Beliefs about learning, self-regulated strategies and text comprehension among Chinese children. Br. J. Educ. Psychol. 78, 51–73. doi: 10.1348/000709907X179812
Lee, D., Watson, S. L., and Watson, W. R. (2019). Systematic literature review on self-regulated learning in massive open online courses. Aust. J. Educ. Technol. 35, 28–41. doi: 10.14742/ajet.3749
Limone, P., Sinatra, M., Ceglie, F., and Monacis, L., (2020). Examining Procrastination Among University Students Through The Lens Of The Self-Regulated Learning Model. Behav. Sci. 10:184. doi: 10.3390/bs10120184
Lin, C., Zhang, Y., and Zheng, B. (2017). The roles of learning strategies and motivation in online language learning: a structural equation modeling analysis. Comput. Educ. 113, 75–85. doi: 10.1016/j.compedu.2017.05.014
Martin, A., and Marsh, H. (2006). Academic resilience and its psychological and educational correlates: a construct validity approach. Psychol. Schools 43, 267–281. doi: 10.1002/pits.20149
Matcha, W., Gašević, D., Uzir, N. A. A., Jovanović, J., and Pardo, A. (2019). “Analytics of learning strategies: associations with academic performance and feedback,” in Proceedings of the 9th International Conference on Learning Analytics and Knowledge (LAK'19): Learning Analytics to Promote Inclusion and Success, eds C. Brooks, R. Ferguson, and U. Hoppe (Tempe, AZ: ACM International Conference Proceeding Series; Association for Computing Machinery (ACM)), 461–470. doi: 10.1145/3303772.3303787
McKeachie, W., Pintrich, P. R., Lin, Y. G., and Smith, D. A. F. (1987). Teaching and Learning in the College Classroom: A Review of the Research Literature. Ann Arbor, MI: University of Michigan Press.
Meijs, C., Neroni, J., Gijselaers, H. J. M., Leontjevas, R., Kirschner, P. A., and de Groot, R. H. M. (2019). Motivated strategies for learning questionnaire part B revisited: new subscales for an adult distance education setting. Int. High. Educ. 40, 1–11. doi: 10.1016/j.iheduc.2018.09.003
Neroni, J., Meijs, C., Gijselaers, H. J. M., Kirschner, P., and de Groot, R. H. M. (2019). Learning strategies and academic performance in distance education. Learn. Individ. Diff. 73, 1–7. doi: 10.1016/j.lindif.2019.04.007
Panadero, E. (2017). A review of self-regulated learning: six models and four directions for research. Front. Psychol. 8:422. doi: 10.3389/fpsyg.2017.00422
Pintrich, P. R. (2000). “The role of goal orientation in self-regulated learning,” in Handbook of Self-Regulation, eds M. Boekaerts, P.R. Pintrich, and M. Zeidner (San Diego, CA: Academic Press), 451–502. doi: 10.1016/B978-012109890-2/50043-3
Pintrich, P. R., and de Groot, E. V. (1990). Motivated and self-regulated learning components of classroom academic performance. J. Educ. Psychol. 82, 33–40. doi: 10.1037/0022-0663.82.1.33
Pintrich, P. R., Marx, R. W., and Boyle, R. A. (1993a). Beyond cold conceptual change: the role of motivational beliefs and classroom contextual factors in the process of conceptual change. Rev. Educ. Res. 63, 167–199. doi: 10.3102/00346543063002167
Pintrich, P. R., Smith, D. A. F., Duncan, T., and McKeachie, W. (1993b). Reliability and predictive validity of the motivated strategies for learning questionnaire (MSLQ). Educ. Psychol. Meas. 53, 801–813. doi: 10.1177/0013164493053003024
Pintrich, P. R., Wolters, C. A., and Baxter, G. (2000). Assessing metacognition and self-regulated learning. Issues Meas. Cogn. 3, 43–98. Available online at: https://digitalcommons.unl.edu/burosmetacognition/3
Ramírez-Dorantes, M. C., Canto y Rodríguez, J. E., Bueno-Alvarez, J.-A., and Echazarreta-Moreno, A. (2013). Psychometric validation of the “Motivated Strategies for Learning Questionnaire” with Mexican university students. Electron. J. Res. Educ. Psychol. 11, 193–214. doi: 10.25115/ejrep.v11i29.1563
Respondek, L., Seufert, T., Hamm, J. M., and Nett, U. E. (2020). Linking changes in perceived academic control to university dropout and university grades: a longitudinal approach. J. Educ. Psychol. 112, 987–1002. doi: 10.1037/edu0000388
Robson, D. A., Allen, M. S., and Howard, S. J. (2020). Self-regulation in childhood as a predictor of future outcomes: a meta-analytic review. Psychol. Bull. 146, 324–354. doi: 10.1037/bul0000227
Schouwenburg, H. C. (2004). “Procrastination in academic settings: general introduction,” in Counseling the Procrastinator in Academic Settings, eds H. C. Schouwenburg, C. H. Lay, T. A. Pychyl, and J. R. Ferrari (Washington, DC: American Psychological Association), 3–17. doi: 10.1037/10808-000
Schumacker, R. E., and Lomax, R. G. (2015). A Beginner's Guide to Structural Equation Modeling, 4th Edn. New York, NY: Routledge.
Shawer, S. (2013). Initial teacher education: does self-efficacy influence candidate teacher academic achievement and future career performance? J. Furth. High. Educ. 37, 201–223. doi: 10.1080/0309877X.2011.645448
Sideridis, G. D., and Stamovlasis, D. (2016). Instrumental help-seeking as a function of normative performance goal orientations: a “catastrophe”. Motiv. Emot. 40, 82–100. doi: 10.1007/s11031-015-9515-9
Sirois, F. M., Yang, S., and van Eerde, W. (2019). Development and validation of the general procrastination scale (GPS-9): a short and reliable measure of trait procrastination. Pers. Indiv. Diff. 146, 26–33. doi: 10.1016/j.paid.2019.03.039
Soemantri, D., Mccoll, G., and Dodds, A., (2018). Measuring medical students' reflection on their learning: modification and validation of the motivated strategies for learning questionnaire (MSLQ). BMC Med. Educ. 18:274. doi: 10.1186/s12909-018-1384-y
Steel, P. (2007). The nature of procrastination: a meta-analytic and theoretical review of quintessential self-regulatory failure. Psychol. Bull. 133, 65–94. doi: 10.1037/0033-2909.133.1.65
Tan, S., and Guo, Y. (2008). Revision of self-control scale for Chinese college students. Chin. J. Clin. Psychol. 16, 468–470.
Tang, G. (2004). Eighth graders' academic help-seeking and its relation to achievement goals, self-efficacy and culture factors. Chin. J. Clin. Psychol. 12, 250–259.
Tong, F., Guo, H., Wang, Z., Min, Y., Guo, W., and Yoon, M. (2019). Examining cross-cultural transferability of self-regulated learning model: an adaptation of the motivated strategies for learning questionnaire for Chinese adult learners. Educ. Stud. 4, 422–439. doi: 10.1080/03055698.2019.1590183
Winne, P. H., and Hadwin, A. F. (2008). “The weave of motivation and self-regulated learning,” in Motivation and Self-Regulated Learning: Theory, Research, and Applications, eds D. H. Schunk and B. J. Zimmerman (Mahwah, NJ: Lawrence Erlbaum Associates Publishers), 297–314.
Yang, C., Lai, A., Chen, M., Hwang, M., and Li, C. (2012). “An investigation on procrastination in the e-learning environment,” in 2012 8th International Conference on Information Science and Digital Content Technology (ICIDT2012) (Jeju: IEEE), 616–620.
Keywords: learning strategies, questionnaire, validation, adult distance learning, psychometrics
Citation: Zhou Y and Wang J (2021) Psychometric Properties of the MSLQ-B for Adult Distance Education in China. Front. Psychol. 12:620564. doi: 10.3389/fpsyg.2021.620564
Received: 23 October 2020; Accepted: 01 February 2021;
Published: 23 February 2021.
Edited by:
Cristina M. Pulido, Autonomous University of Barcelona, SpainReviewed by:
Antonio Baena Extremera, University of Granada, SpainPedro F. S. Rodrigues, University of Minho, Portugal
Copyright © 2021 Zhou and Wang. This is an open-access article distributed under the terms of the Creative Commons Attribution License (CC BY). The use, distribution or reproduction in other forums is permitted, provided the original author(s) and the copyright owner(s) are credited and that the original publication in this journal is cited, in accordance with accepted academic practice. No use, distribution or reproduction is permitted which does not comply with these terms.
*Correspondence: Jianhua Wang, wjhsfl@ruc.edu.cn
†These authors have contributed equally to this work