- Autonomous Driving Department, CDV – Transport Research Centre, Brno, Czechia
This study reviews the current information concerning the measurement of the situation awareness (SA) of the teleoperated drivers of remotely controlled cars. The teleoperated drivers who drive these cars are in a remote location, and they control the cars through a communication interface. The objective methods with probes are beneficial in measuring SA on a closed circuit without real traffic. Questions specifically should address the information provided on the road by haptic sensations, such as the slope of the road and the vehicle's speed. Methods for measuring SA that involve probes and interruptions obviously are not suitable for use on public roads. A stable environment for the display and control of the communication interface is suitable for an eye tracker in measuring SA. These features also facilitate the use of subjective observer-rating methods. Both of these methods are suitable for driving on real roads because they are not intrusive. SA research in a real-road environment also should demonstrate how the SA of other drivers is affected by seeing a car without a driver. Given the remote character of driving, cultural differences in cognition may have a significant influence on the SA of the teleoperated driver.
Introduction
Technological development allows for the operation of vehicles without a driver in the vehicle. Especially, the importance of autonomous vehicles has increased significantly, and it is likely to continue to do so (Alawadhi et al., 2020). However, even though fully autonomous cars currently are being developed, there will be situations in which automation must be supplemented by a person's involvement (Hampshire et al., 2020). For example, the automated controllers and devices may suddenly malfunction, leaving the autonomous car stranded. In such cases, as the passenger may not possess the driving skills to drive the car, a human teleoperator in an office can take control and operate the car remotely using video information and other data transmitted from the car through a wireless network (Berman, 2019). Besides, such remote operation can be useful in other situations, e.g., when the driver gets drunk and unable to control the vehicle. In such a case, the remote operator must have good driving skills to get the driver home safely. Therefore, it is important to determine how to assess the quality of people's remote driving skills. Several factors are crucial to the performance of people in unmanned systems, these include situational awareness (SA), cognitive workload, complacency, and overtrust of automated systems (Stark et al., 2012).
A remotely controlled vehicle (RCV) is a vehicle that is controlled by a person, i.e., a “teleoperator,” from a remote location using a communication interface. The vehicle contains a sensor module that provides information about the environment and other information to the teleoperator. Typical sensors are cameras, lidar systems, radars, and sonar. The teleoperator drives the vehicle from a remote control station. An RCV could be a train, an agricultural machine, an underwater vehicle (Ho et al., 2011), a drone (Chen et al., 2018), or a robot for destroying mines (Riley et al., 2004).
Remotely controlled cars (RCCs) are cars without drivers operated on public roads and driven by an operator in a remote location. Therefore, all RCCs are also RCVs. Currently, there is a lack of research regarding the SA of the RCCs operators. The RCCs operators are also referred to as “teleoperated drivers.” In this study, we provide directions concerning how to measure the SA of the RCCs' teleoperated drivers to assess their driving skills.
The rest of the paper is organized as follows: We review the concept of SA and its measurement in the next section. The following section analyzes the measurement of SA in the context of RCVs. The final section suggests an optimum approach for measuring SA as it relates to RCCs.
Situation Awareness and its Measurement
Situation awareness is the ability to see and understand (Endsley, 2008), including what “that information means to you now and in the future” (Endsley and Jones, 2011:13). Gawron (2019:135) defines SA as “knowledge relevant to the task being performed.” SA usually is used in a specific context, and it is defined more specifically for that particular context (Endsley and Jones, 2011:13). SA can be defined for humans, but it also can be defined for self-directed systems that use artificial intelligence (Adams, 2007), such as autonomous vehicles (Freedman and Adams, 2007). SA for robots concerns the need to understand orders from people, to know how to act after understanding the orders, and collect information about other robots and people as part of their repertoire (Drury et al., 2006). SA is dependent on many factors and environmental characteristics. Table 1 summarizes driver, environment, and car characteristics that influence a driver's SA. Table 2 summarizes operator, environment, and operation interface characteristics that influence a teleoperator's SA.
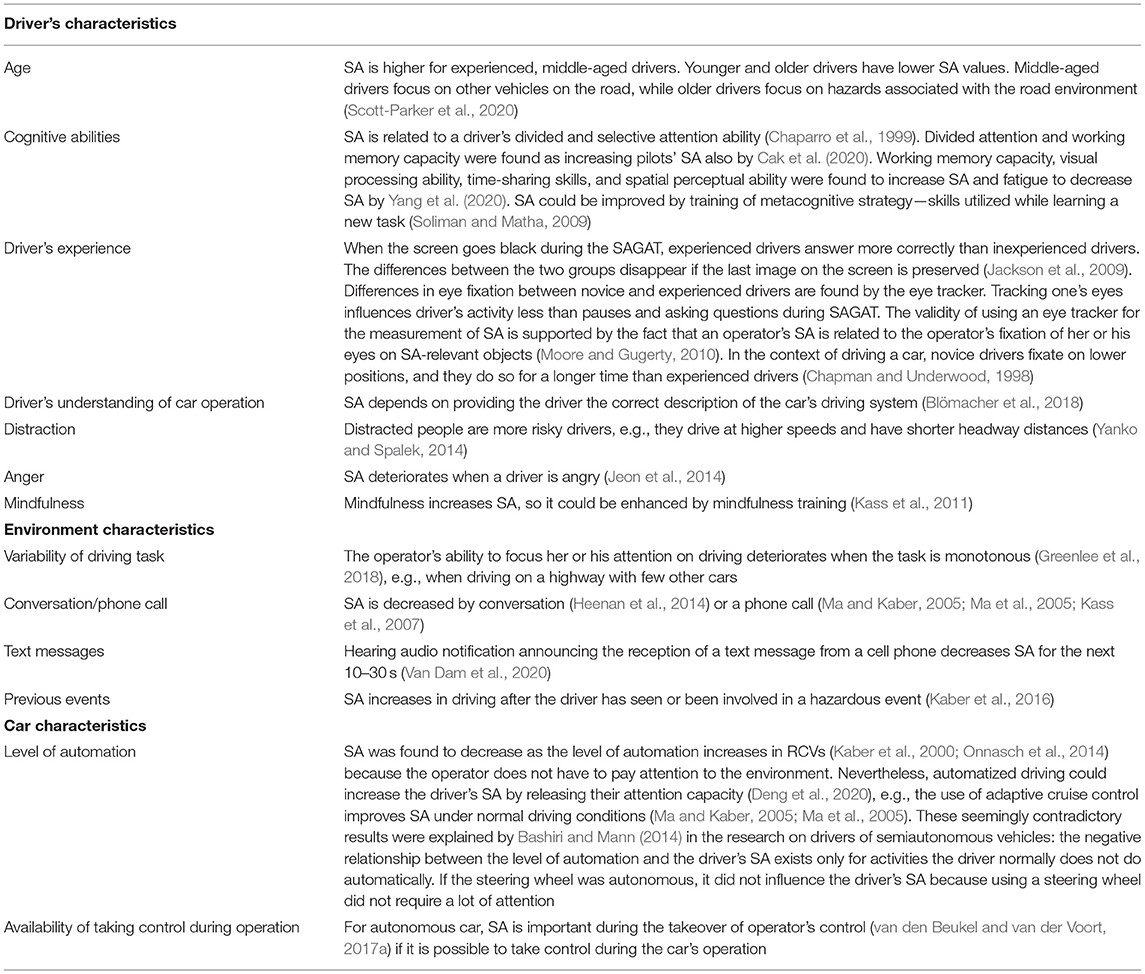
Table 1. Driver, environment, and car characteristics that influence a driver's situational awareness (SA).
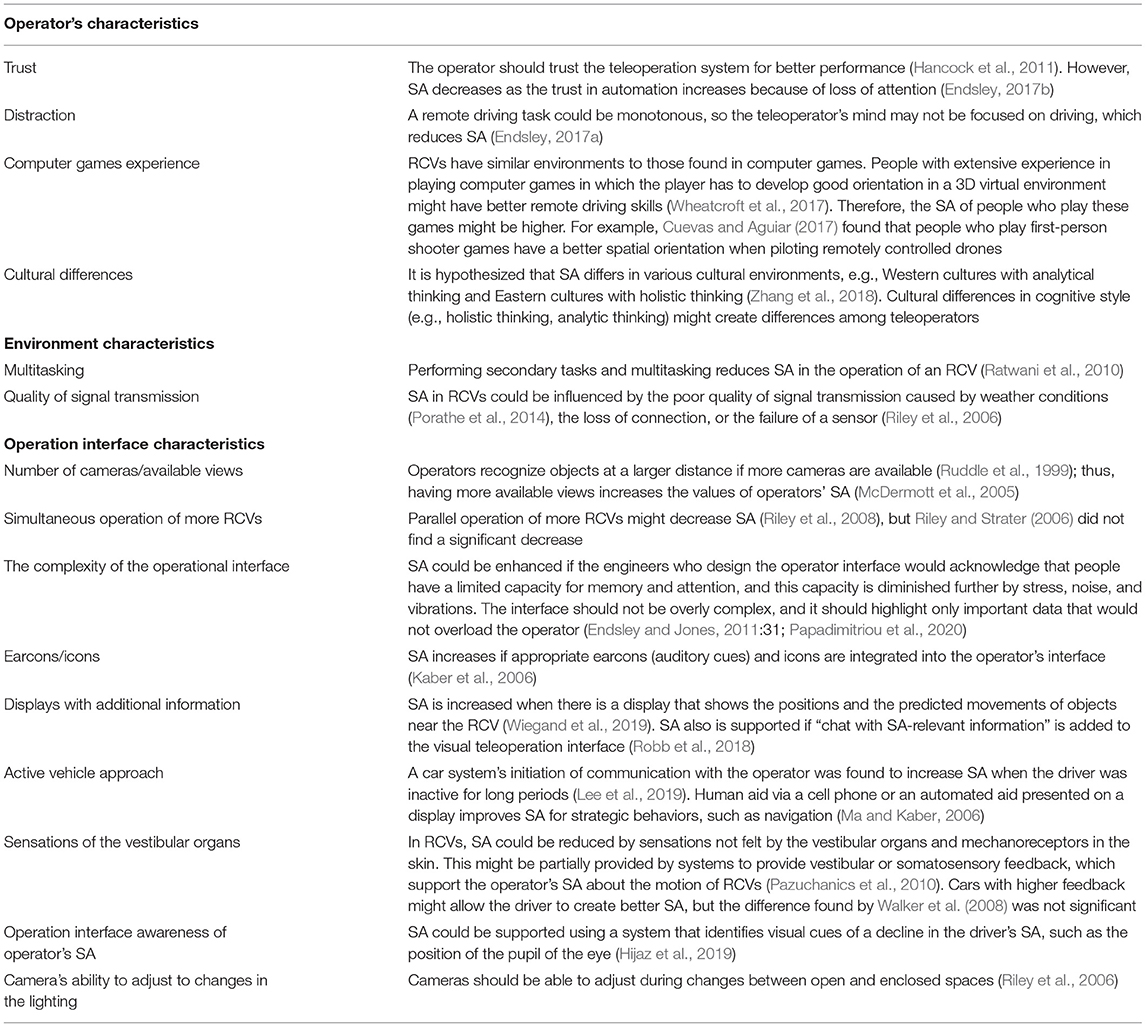
Table 2. Remotely controlled vehicle (RCV) operator, environment, and operation interface characteristics that influence a teleoperator's situational awareness (SA).
SA can be viewed from three perspectives (Salmon et al., 2012). The individual perspective is a purely cognitive phenomenon that exists in people's minds. The engineering perspective arises from the interaction between people, the displayed information, and other indicators. The system perspective is based on the interaction between people and various technologies, including displays and indicators. An example is a railway crossing, which includes the SA of the car driver, the train, and the train operator, and the railway crossing (Salmon et al., 2012). SA for emergencies is different from SA for behavior in normal situations (Wickens, 2000) because emergencies require the operator to pay more attention to the environment (Wu et al., 2019).
Many methods to measure SA range from self-rating scales to eye trackers (Zhou et al., 2019). SA measurement methods can be classified into three groups (Pew, 2008). The first group consists of direct performance methods that measure the results of the operator, and it is expected that these results are related to the operator's SA. Second, experimental methods that include queries, probes, and other techniques to measure the operator's behavior and SA knowledge during the performance. Third, SA could be measured by subjective methods based on either an observer's ratings or the operator's self-ratings. Subjective methods for the SA measurement are the Situation Awareness Rating Technique (SART; Taylor, 1990), Post-assessment of Situation Awareness (Gatsoulis et al., 2010), and the Situational Awareness Rating Scale for self-rating and the Situation Awareness Behavioral Rating Scale (SABARS; Strater et al., 2001) for rating by expert observers.
Experimental methods are the Situation Awareness Global Assessment Technique (SAGAT; Endsley, 1995), the Situation Present Assessment Method (SPAM; Durso et al., 1998), and an eye tracker. It is possible to record an operator when he or she is describing what he or she actually is doing and thinking. Then, what has been recorded can be analyzed (Heikoop et al., 2018). SAGAT was developed for simulation research (Endsley, 2015), and it involves pauses in activity. When the simulation is stopped, the operator is asked about their environmental perception. SPAM was designed for real-time research, so the operator is asked questions about the environment during the activity. SAGAT is more sensitive to experimental differences if the probes into the operator's activities occur at random times rather than saving the questions for the end. SAGAT questions for operators should fulfill several requirements. They should ask only about things that are relevant to the operator's SA; questions must be clear and not be subjective, and it should be easy to decide whether the answer is right or wrong (Endsley, 2019). Eye fixation was found to be a predictor of SAGAT scores (Argyle et al., 2020). SAGAT might face challenges in collecting data about real road traffic because of interruptions.
SA measurement methods based on performance encounter problems with validity because performance and SA might be two different things (Nguyen et al., 2019). Endsley (2020) found that self-rating methods for SA measurements do not correlate with methods such as SAGAT, which aim to objectively measure SA knowledge. The author concluded that these methods measure different things, and self-rating methods should not be considered to measure the general SA. Rather, they should be used to measure only the self-confidence about one's own SA. Combining low SA with high self-confidence might be especially dangerous (Endsley, 2020). Besides, methods based on self-rating after activity have the problem that the subject might have already forgotten details (Nguyen et al., 2019). Experimental objective methods also face some criticism. Salmon et al. (2012) criticized SAGAT as being useless for the analysis of the interaction of different SA components. SAGAT also has been criticized for being unable to capture the unconscious part of SA, for being unsuitable for the study of the SA dynamics (Winter et al., 2019), and for excessive interference with an operator's activity. However, Endsley (2019) did not find such interference when reviewing the research that had examined this problem. The studies she reviewed found no SAGAT negative effects on the operator's performances.
Situation Awareness in Remotely Controlled Vehicles
With the advancement of RCVs, it is crucial to study the teleoperators SA. Being aware of the environment is pivotal for driving (Zhou et al., 2020). The controls and displays of the RCV should provide accurate information to avoid having the teleoperator stop trusting them. Besides, alarms should warn the teleoperator only when something crucial happens, to avoid displaying false warnings that lead to distrust (Endsley and Jones, 2011). The RCV operators should develop SA in their local environment, i.e., the controls, and displays where they sit, as well as in the remote environment where the vehicle is located, e.g., traffic signs. An important trait is the skills to divide one's attention without losing sight of what is happening elsewhere (Riley et al., 2010). SA in RCVs depends on the interfaces between many vehicle and operational characteristics. Table 1 summarizes driver, environment, and car characteristics that influence a driver's SA. Table 2 summarizes operator, environment, and operation interface characteristics that influence a teleoperator's SA.
Techniques for measuring SA have been developed for many RCVs. Compared to other RCVs, it is more important to study SA for RCCs in real life rather than using simulation because many unexpected things can happen in real traffic especially in countries where traffic rules are less respected (Huang et al., 2006). Endsley and Jones (2011:226–233) summarized challenges for good SA in RCVs, some of which are relevant for RCCs, e.g., (1) the operator's bad sensory information, (2) time delays in the signals transmission, (3) lightning problems at the vehicle's location, and (4) the vehicle's interface complexity and multitasking. A careful design of the RCV's displays and controls can mitigate these problems, thereby allowing for higher SA. The requirements for the RCV's operator could be adapted from the requirements established for ground robots by Endsley and Jones (2011:224–225), i.e., the requirements are that the operator should know the car status (e.g., its speed), its characteristics (e.g., weight), the location of other objects, the status of the motor system, the communication quality, the weather conditions, details of the terrain, and the quality of the car sensors. Besides, the operator must understand how to project all previous experience to deal with present and future situations. In addition, RCC operators should understand the traffic rules in the country, what traffic signs mean, the current status of the traffic lights, car's winkers, whether they are overtaking another vehicle or being overtaken, and the current speed limit.
SA in RCVs often is measured according to the operator's performance. Yanco and Drury (2004) measured the SA of the teleoperator of an urban rescue robot as the number of the collisions with other objects and the time spent adjusting the camera. Velagapudi et al. (2012) measured SA in the context of unmanned aerial vehicles based on the difference between the real positions of colored targets in a video from the vehicle and the positions where the subject thought these targets were on a map. Performance methods also are suitable for studying SA in RCVs. Experts can rate the teleoperator's behavior using SABARS pre-defined scales created for the specific activity, such as those developed by Kaber et al. (2013).
Suggestions for the Measurement of Situation Awareness in Remotely Controlled Cars
Researchers attempting to measure the SA in RCCs might discover a scarcity of past studies on the SA of RCC-teleoperated drivers. These researchers should follow the following suggestions based on the SA measurement in other environments.
The SA measurement for RCC-teleoperated drivers differs from the measurement of classic car drivers. Teleoperated drivers cannot perceive the car's speed, the slope of the road, or the weight of the load using physical sensation. Besides, they cannot hear the sound of the car's engine telling them about a possible problem. There might be blind spots between cameras, and it might be difficult for the teleoperated drivers to react if there is a loss of connection. The researcher must pay attention to how the operator gets the SA about these things (e.g., repeated checks of the speed indicator due to the lack of physical sensation). SAGAT and SPAM questions concerning these issues might be related to the slope of the road, the quality of the transmission, speed, the position of the wheel, the positions of other objects, and whether all of the car's systems are working correctly. Eye tracker might serve in studying where the operator looks to when searching for information regarding speed, slope, and transmission quality.
Taking the SA of RCCs from the perspective of the system, in addition to the car, other vehicles, pedestrians, and parts of the infrastructure should be considered. Specifically, this means the reaction to viewing a car without a driver. Pedestrians, cyclists, and drivers might behave in different ways in this situation, and this might result in dangerous situations.
All SA measurement methods might be used for studying the SA of RCCs' operators. As with other types of RCVs, performance methods specific for cars might be useful; this includes counting the number of hit skittles or the number of jerks, i.e., episodes of braking too rapidly, measured as occurrences when the second derivative of speed is >−9.9 m/s3 (Bagdadi and Várhelyi, 2011). For the analysis of how a driver follows traffic rules, some modification of the Wiener Fahrprobe method (Chaloupka and Risser, 1995) might be useful when one trained researcher rates how the driver/operator follows the traffic rules, and the second researcher watches for unexpected events. This method is a standardized method that has been used for a long time for classic cars. Besides, it assesses drivers'/operators' actual behaviors in a real environment. However, it is not adapted for RCCs, and it requires a significant amount of resources, i.e., the driver should be watched by two researchers, and the observation should be done for a long time in order to see a sufficient number of mistakes. In addition, the method only captures behavior from the perspective of the observer and does not allow to study processes inside the driver's mind.
SAGAT could be useful when studying an operator's behavior on the test tracks. When the monitors are blinded, the control of the car could be taken over by the safety driver. It is more effective than SART (van den Beukel and van der Voort, 2017b). SAGAT disadvantage is that it might cause problems in traffic safety if used in real traffic. It is impossible to use SAGAT there because of the difficulties associated with interruptions.
SPAM might be used when studying the SA of RCC operators in real road traffic. Nevertheless, SAGAT is more successful than SPAM in finding differences between experimental conditions (Endsley, 2019). SPAM possibly could decrease traffic safety in real traffic—it was found to create problems with intrusiveness or workload in 40% of studies reviewed by Endsley (2019). This concern might be decreased by showing “Yes/No” questions on one of the system's displays, as is the case for the Daze system developed by Sirkin et al. (2017). In this case, drivers do not have to listen to SPAM questions. Rather, they can look at the display beside the steering wheel, which requires less attention. However, drivers' interactions with such a display can decrease SA (Wulf et al., 2013), so this solution might be not successful in ensuring traffic safety.
Expert-rating methods like SABARS are important for traffic safety in real road traffic, as they are not interfering with the operators' activities. Given the office-based nature of the remote operation, teleoperated drivers offer better opportunities for observers than the driver in the car. However, SABARS has not been used in the context of car driving or vehicle remote operation.
Self-rating methods are safe in real road traffic. SART was found to be better than SPAM in predicting the performance of remotely controlled robots (Schuster et al., 2012). However, SART has several limitations. It measures a different construct than SAGAT (Endsley, 2020), so it is questionable whether it measures SA, and it is unable to capture the operator's state of SA.
The benefit of eye tracking is its non-intrusiveness, so it does not interfere with traffic safety. Nevertheless, eye tracking does not allow for the assessment of the attention, audio cognition, and information evaluation of the operator's brain (Endsley, 2019). Eye trackers are complicated to use when drivers are inside road vehicles (Nguyen et al., 2019) because of noise (Heikoop et al., 2018). Given the office-based character of the RCC's interface when compared with the driving interface in the car, the eye tracker would provide better data than when used on the road.
The above information is applicable for remotely controlled buses and trucks. The main difference is that trucks and buses are longer and need more cameras. This means that teleoperated drivers should divide attention between more displays at teleoperated driver's interface, which might decrease SA.
Conclusion
The present study reviews the SA measurement for a teleoperated driver in RCCs. Previous reviews of SA measurement concerned classic car drivers or teleoperators of non-road vehicles; the current text merges both these themes into one review. The study enhances a greater understanding of researchers aiming to decrease the risk of human-factor failure in remote driving. The entire SA measurement methods are available if RCCs are tested on a circuit. Researchers aiming to measure SA on a circuit should prefer objective experimental methods, such as SAGAT. Nevertheless, when studying RCCs on roads in densely populated urban areas, researchers should consider other SA less-intrusive measurement methods and evaluate whether the safety benefits of these methods outbalance their limitations. To date, no intensive research is available on the SA measurements for operators of RCCs, but we expect that it will be studied more extensively in the future.
Author Contributions
All authors listed have made a substantial, direct and intellectual contribution to the work, and approved it for publication.
Funding
This article was produced with the financial support of the Ministry of Education, Youth and Sports within National Sustainability Programme I, a project of the Transport R&D Centre (LO1610), on a research infrastructure acquired from the Operation Programme Research and Development for Innovations (CZ.1.05/2.1.00/03.0064).
Conflict of Interest
The authors declare that the research was conducted in the absence of any commercial or financial relationships that could be construed as a potential conflict of interest.
References
Adams, J. A. (2007). “Unmanned vehicle situation awareness: a path forward,” in Proceedings of the 2007 Human Systems Integration Symposium (Annapolis, MD).
Alawadhi, M., Almazrouie, J., Kamil, M., and Khalil, K. A. (2020). Review and analysis of the importance of autonomous vehicles liability: a systematic literature review. Int. J. Syst. Assurance Eng. Manage. 11, 1227–1249. doi: 10.1007/s13198-020-00978-9
Argyle, E. M., Gourley, J. J., Kang, Z., and Shehab, R. L. (2020). Investigating the relationship between eye movements and situation awareness in weather forecasting. Appl. Ergon. 85:103071. doi: 10.1016/j.apergo.2020.103071
Bagdadi, O., and Várhelyi, A. (2011). Jerky driving – an indicator of accident proneness? Accid. Anal. Prev. 43, 1359–1363. doi: 10.1016/j.aap.2011.02.009
Bashiri, B., and Mann, D. D. (2014). Automation and the situation awareness of drivers in agricultural semi-autonomous vehicles. Biosyst. Eng. 124, 8–15. doi: 10.1016/j.biosystemseng.2014.06.002
Berman, B. (2019). Teleoperations Will Keep Humans in the AV Loop. SAE International. Available online at: https://www.sae.org/news/2019/10/human-in-the-loop-autonomous-teleoperations (accessed August 2, 2018).
Blömacher, K., Nöcker, G., and Huff, M. (2018). The role of system description for conditionally automated vehicles. Transportation Res. F 54, 159–170. doi: 10.1016/j.trf.2018.01.010
Cak, S., Say, B., and Misirlisoy, M. (2020). Effects of working memory, attention, and expertise on pilots' situation awareness. Cogn. Technol. Work 22, 85–94. doi: 10.1007/s10111-019-00551-w
Chaloupka, C., and Risser, R. (1995). Don't wait for accidents – possibilities to assess risk in traffic by applying the “Wiener Fahrprobe”. Saf. Sci. 19, 137–147. doi: 10.1016/0925-7535(94)00015-U
Chaparro, A., Groff, L., Tabor, K., Sifrit, K., and Gugerty, L. (1999). Maintaining situational awareness: the role of visual attention. Proc. Hum. Factors Ergon. Soc. Ann. Meet. 43, 1343–1347. doi: 10.1177/154193129904302317
Chapman, P. R., and Underwood, G. (1998). Visual search of driving situations: danger and experience. Perception 1998, 951–964. doi: 10.1068/p270951
Chen, J., Zhang, Q., Qiu, X., and Hou, B. (2018). An Assessment Method of Pilot Situation Awareness in Manned/Unmanned-Aerial-Vehicles Team, “ in ChinaCom 2017, LNICST 237, eds. B. Li et al., 132–140. doi: 10.1007/978-3-319-78139-6_14
Cuevas, H. M., and Aguiar, M. (2017). Assessing situation awareness in unmanned aircraft systems operations. Int. J. Aviat. Aeronaut. Aerosp. 4:3. doi: 10.15394/ijaaa.2017.1176
Deng, Y., Shirley, J., Zhang, W., Kim, N. Y., and Kaber, D. (2020). “Influence of dynamic automation function allocations on operator situation awareness and workload in unmanned aerial vehicle control,” in AHFE 2019, AISC 959, ed I. L. Nunes (Cham: Springer), 337–348. doi: 10.1007/978-3-030-20040-4_31
Drury, J. L., Riek, L., and Radcliffe, N. (2006). “A decomposition of UAV-related situation awareness,” in HRI '06: Proceedings of the 1st ACM SIGCHI/SIGART conference on Human-Robot Interaction (Salt Lake City UT), 88–94. doi: 10.1145/1121241.1121258
Durso, F. T., Hackworth, C. A., Truitt, T. R., Crutchfield, J., Nikolic, D., and Manning, C. A. (1998). Situation awareness as a predictor of performance for en route air traffic controllers. Air Traffic Control Q. 6, 1–20. doi: 10.2514/atcq.6.1.1
Endsley, M. R. (1995). Measurement of situation awareness in dynamic systems. Hum. Factors 37, 65–84. doi: 10.1518/001872095779049499
Endsley, M. R. (2008). “Theoretical underpinnings of situation awareness: a critical review,” in Situation Awareness Analysis and Measurement, eds M. R. Endsley, D. J. Garland (Boca Raton, FL: CRC Press), 3–32.
Endsley, M. R. (2015). Final reflections: situation awareness models and measures. J. Cogn. Eng. Decis. Mak. 9, 101–111. doi: 10.1177/1555343415573911
Endsley, M. R. (2017a). Autonomous driving systems: a preliminary naturalistic study of the Tesla model S. J. Cogn. Eng. Decis. Mak. 11, 225–238. doi: 10.1177/1555343417695197
Endsley, M. R. (2017b). From here to autonomy: lessons learned from human-automation research. Hum. Factors 59, 5–27. doi: 10.1177/0018720816681350
Endsley, M. R. (2019). A systematic review and meta-analysis of direct objective measures of situation awareness: a comparison of SAGAT and SPAM. Hum. Factors 63, 124–150. doi: 10.1177/0018720819875376
Endsley, M. R. (2020). The divergence of objective and subjective situation awareness: a meta-analysis. J. Cogn. Eng. Decis. Mak. 14, 34–53. doi: 10.1177/1555343419874248
Endsley, M. R., and Jones, D. G. (2011). Designing for Situation Awareness. An Approach to User-Centered Design. Boca Raton, FL: CRC Press.
Freedman, S. T., and Adams, J. A. (2007). “The inherent components of unmanned vehicle situation awareness,” in 2007 IEEE International Conference on Systems, Man and Cybernetics (Montreal, QC), 973–977. doi: 10.1109/ICSMC.2007.4414129
Gatsoulis, Y., Virk, G. S., and Dehghani-Sanij, A. A. (2010). On the measurement of situation awareness for effective human-robot interaction in teleoperated systems. J. Cogn. Eng. Decis. Mak. 4, 69–98. doi: 10.1518/155534310X495591
Gawron, V. J. (2019). Human Performance and Situation Awareness Measures. Boca Raton, FL: CRC Press.
Greenlee, E. T., DeLucia, P. R., and Netwon, D. C. (2018). Driver vigilance in automated vehicles: hazard detection failures are a matter of time. Hum. Factors 60, 465–476. doi: 10.1177/0018720818761711
Hampshire, R. C., Bao, S.h., Lasecki, W. S., Daw, A., and Pender, J. (2020). Beyond safety drivers: applying air traffic control principles to support the deployment of driverless vehicles. PLoS ONE 15:e0232837. doi: 10.1371/journal.pone.0232837
Hancock, P. A., Billings, D. R., Schaefer, K. E., Chen, J. Y. C., de Visser, E. J., and Parasuraman, R. (2011). A meta-analysis of factors affecting trust in human-robot interaction. Hum. Factors 53, 517–527. doi: 10.1177/0018720811417254
Heenan, A., Herdman, C.h.M., Brown, M. S., and Robert, N. (2014). Effects of conversation on situation awareness and working memory in simulated driving. Hum. Factors 56, 1077–1092. doi: 10.1177/0018720813519265
Heikoop, D. D., de Winter, J. C. F., van Arem, B., and Stanton, N. A. (2018). Effects of mental demands on situation awareness during platooning: a driving simulator study. Transportat. Res. F 58, 193–209. doi: 10.1016/j.trf.2018.04.015
Hijaz, A., Louie, W.-Y. G., and Mansour, I. (2019). “Towards a driver monitoring system for estimating driver situational awareness,” in 28th IEEE International Conference on Robot and Human Interactive Communication (New Delhi), 1–6. doi: 10.1109/RO-MAN46459.2019.8956378
Ho, G., Pavlovic, N., and Arrabito, R. (2011). Human factors issues with operating unmanned underwater vehicles. Proc. Hum. Factors Ergon. Soc. Ann. Meet. 55, 429–433. doi: 10.1177/1071181311551088
Huang, Y.-H., Zhang, W., Roetting, M., and Melton, D. (2006). Experiences from dual-country drivers: driving safely in China and the US. Saf. Sci. 44, 785–795. doi: 10.1016/j.ssci.2006.05.002
Jackson, L., Chapman, P., and Crundall, D. (2009). What happens next? Predicting other road users' behaviour as a function of driving experience and processing time. Ergonomics 52, 156–164. doi: 10.1080/00140130802030714
Jeon, M., Walker, B. N., and Gable, T. M. (2014). Anger effects on driver situation awareness and driving performance. Presence 23, 71–89. doi: 10.1162/PRES_a_00169
Kaber, D., Jin, S., Zahabi, M., and Pankok, J.r. C. (2016). The effect of driver cognitive abilities and distractions on situation awareness and performance under hazard conditions. Transportat. Res. F 42, 177–194. doi: 10.1016/j.trf.2016.07.014
Kaber, D. B., Onal, E., and Endsley, M. R. (2000). Design of automation for telerobots and the effect on performance, operator situation awareness, and subjective workload. Hum. Factors Ergon. Manufact. 10, 409–430. doi: 10.1002/1520-6564(200023)10:4andlt;409::AID-HFM4andgt;3.0.CO;2-V
Kaber, D. B., Riley, J. M., Endsley, M. R., Sheik-Nainar, M., Zhang, T., and Lampton, D. R. (2013). Measuring situation awareness in virtual environment-based training. Military Psychol. 25, 330–344. doi: 10.1037/h0095998
Kaber, D. B., Wright, M. C., and Sheik-Nainar, M. A. (2006). Investigation of multi-modal interface features for adaptive automation of a human-robot system. Int. J. Hum. Comput. Stud. 64, 527–540. doi: 10.1016/j.ijhcs.2005.11.003
Kass, S. J., Cole, K. S., and Stanny, C. J. (2007). Effects of distraction and experience on situation awareness and simulated driving. Transportat. Res. F 10, 321–329. doi: 10.1016/j.trf.2006.12.002
Kass, S. J., VanWormer, L. A., Mikulas, W. L., Legan, S., and Bumgarner, D. (2011). Effect of mindfulness training on simulated driving: preliminary results. Mindfulness 2, 236–241. doi: 10.1007/s12671-011-0066-1
Lee, J., Hirano, T., Hano, T., and Itoh, M. (2019). “Conversation during partially automated driving: how attention arousal is effective on maintaining situation awareness,” in 2019 IEEE International Conference on Systems, Man and Cybernetics (Bari), 3718–3724. doi: 10.1109/SMC.2019.8914632
Ma, R., and Kaber, D. B. (2005). Situation awareness and workload in driving while using adaptive cruise control and a cell phone. Int. J. Ind. Ergon. 35, 939–953. doi: 10.1016/j.ergon.2005.04.002
Ma, R., and Kaber, D. B. (2006). Situation awareness and driving performance in a simulated navigation task. Proc. Hum. Factors Ergon. Soc. Ann. Meet. 50, 270–274. doi: 10.1177/154193120605000313
Ma, R., Sheik-Nainar, M. A., and Kaber, D. B. (2005). Situation awareness while using adaptive cruise control and a cell phone. Proc. Hum. Factors Ergon. Soc. Ann. Meet. 49, 381–385. doi: 10.1177/154193120504900335
McDermott, P. L., Luck, J. A., Allender, L., and Fisher, A. (2005). Effective human to human communication of information provided by an unmanned vehicle. Proc. Hum. Factors Ergon. Soc. Ann. Meet. 49, 402–406. doi: 10.1177/154193120504900340
Moore, K., and Gugerty, L. (2010). Development of a novel measure of situation awareness: the case for eye movement analysis. Proc. Hum. Factors Ergon. Soc. Ann. Meet. 54, 1645–1649. doi: 10.1037/e578802012-062
Nguyen, T., Lim, C.h. P, Nguyen, N. D., Gordon-Brown, L., and Nahavandi, S. (2019). A review of situation awareness assessment approaches in aviation environments. IEEE Syst. J. 13, 3590–3603. doi: 10.1109/JSYST.2019.2918283
Onnasch, L., Wickens, C. D., Li, H., and Manzey, D. (2014). Human performance consequences of stages and levels of automation: an integrated meta-analysis. Hum. Factors 56, 476–488. doi: 10.1177/0018720813501549
Papadimitriou, E., Schneider, C.h., Tello, J. A., Damen, W., Vrouenraets, M. L., and ten Broeke, A. (2020). Transport safety and human factors in the era of automation: what can transport modes learn from each other? Accid. Anal. Prev. 144:105656. doi: 10.1016/j.aap.2020.105656
Pazuchanics, S. L., Chadwick, R. A., Sapp, M. V., and Gillan, D. J. (2010). “Robots in space and time: the role of object, motion and spatial perception in the control and monitoring of uninhabitated ground vehicles,” in Human-Robot Interactions in Future Military Operations, ed F. Jentsch (London: CRC Press), 83–101.
Pew, R. W. (2008). “The state of situation awareness measurement: heading toward the next century,” in Situation Awareness Analysis and Measurement,” eds M. R. Endsley, and D. J. Garland (Boca Raton, FL: CRC Press), 33–47.
Porathe, T., Prison, J., and Man, Y. (2014). “Situation awareness in remote control centres for unmanned ships,” in Proceedings of Human Factors in Ship Design and Operation (London), 93–101.
Ratwani, R. M., McCurry, J. M., and Trafton, J. G. (2010). “Single operator, multiple robots: an eye movement based theoretic model of operator situation awareness,” in Proceedings of the 5th ACM/IEEE International Conference on Human Robot Interaction (Osaka), 235–242. doi: 10.1145/1734454.1734549
Riley, J. M., Kaber, D. B., and Draper, J. V. (2004). Situation awareness and attention allocation measures for quantifying telepresence experiences in teleoperation. Hum. Factors Ergon. Manuf. 14, 51–67. doi: 10.1002/hfm.10050
Riley, J. M., Murphy, R. R., and Endsley, M. R. (2006). “Situation awareness in the control of unmanned ground vehicles,” in Human Factors of Remotely Operated Vehicles, eds N. J. Cooke, H. L. Pringle, H. K. Pedersen, and O. Connor (Bingley: Emerald Group Publishing Limited), 359–371. doi: 10.1016/S1479-3601(05)07025-6
Riley, J. M., and Strater, L. D. (2006). Effects of robot control mode on situation awareness and performance in a navigation task. Proc. Hum. Factors Ergon. Soc. Ann. Meet. 50, 540–544. doi: 10.1177/154193120605000369
Riley, J. M., Strater, L. D., Chappell, S. L., Connors, E. S., and Endsley, M. R. (2010). “Situation awareness in human-robot interaction: challenges and user interface requirements,” in Human-Robot Interactions in Future Military Operations, ed F. Jentsch (London: CRC Press), 171–192.
Riley, J. M., Strater, L. D., Sethumadhavan, A., Davis, F., Tharanathan, A., and Kokini, C. (2008). Performance and situation awareness effects in collaborative robot control with automation. Proc. Hum. Factors Ergon. Soc. Ann. Meet. 52, 242–246. doi: 10.1177/154193120805200410
Robb, D. A., Garcia, F. J. Ch, Laskov, A., Liu, X., Patron, P., et al. (2018). “Keep me in the loop: increasing operator situation awareness through a conversational multimodal interface,” in Proceedings of the 20th ACM International Conference on Multimodal Interaction (New York, NY), 384–392. doi: 10.1145/3242969.3242974
Ruddle, R. A., Savage, J. C., and Jones, D. M. (1999). Effects of camera configurations on target observation that is performer from an uninhabitated air vehicle. Proc. Hum. Factors Ergon. Soc. Ann. Meet. 43, 81–85. doi: 10.1177/154193129904300117
Salmon, P. M., Stanton, N., and Young, K. L. (2012). Situation awareness on the road: review, theoretical and methodological issues, and future directions. Theor. Issues Ergon. Sci. 13, 472–492. doi: 10.1080/1463922X.2010.539289
Schuster, D., Keebler, J. R., Jentsch, F., and Zuniga, J. (2012). Comparison of SA measurement techniques in a human-robot team task. Proc. Hum. Factors Ergon. Soc. Ann. Meet. 56, 1713–1717. doi: 10.1177/1071181312561343
Scott-Parker, B., De Regt, T., Jones, C.h., and Caldwell, J. (2020). The situation awareness of young drivers, middle-aged drivers, and older drivers: same but different? Case Stud. Transport Policy 8, 206–214. doi: 10.1016/j.cstp.2018.07.004
Sirkin, D., Martelaro, N., Johns, M., and Ju, W. (2017). “Toward measurement of situation awareness in autonomous vehicles,” in Proceedings of the 2017 CHI Conference on Human Factors in Computing Systems (New York, NY), 405–415. doi: 10.1145/3025453.3025822
Soliman, A. M., and Matha, E. K. (2009). Metacognitive strategy training improves driving situation awareness. Soc. Behav. Pers. 37, 1161–1170. doi: 10.2224/sbp.2009.37.9.1161
Stark, B., Coopmans, C., and Chen, Y. (2012). “A framework for analyzing human factors in unmanned aerial systems,” in 5th International Symposium on Resilient Control Systems (Salt Lake, UT), 13–18. doi: 10.1109/ISRCS.2012.6309286
Strater, L. D., Endsley, M. R., Pleban, R. J., and Matthews, M. D. (2001). Measures of Platoon Leader Situation Awareness in Virtual Decision Making Exercises (Research Report 1770). Alexandria, VA: U.S. Army Research Institute for the Behavioral and Social Sciences. doi: 10.1037/e500592012-001
Taylor, R. M. (1990). “Situational Awareness Rating Technique (SART): the development of a tool for aircrew systems design,” in Situational Awareness in Aerospace Operations (Neuilly Sur Seine: NATO-AGARD), 3/1–3/17.
Van Dam, J., Kass, S. J., and VanWormer, L. (2020). The effects of passive mobile phone interaction on situation awareness and driving performance. J. Transportat. Saf. Secur. 12, 1007–1024. doi: 10.1080/19439962.2018.1564947
van den Beukel, A. P., and van der Voort, M. C. (2017a). “The influence of time-criticality on situation awareness when retrieving human control after automated driving,” in Proceedings of the 16th International IEEE Annual Conference on Intelligent Transportation Systems (The hague), 2000–2005.
van den Beukel, A. P., and van der Voort, M. C. (2017b). How to assess driver's interaction with partially automated driving systems – a framework for early concept assessment. Appl. Ergon. 59, 302–312. doi: 10.1016/j.apergo.2016.09.005
Velagapudi, P., Owens, S., Scerri, P., Sycara, K., and Lewis, M. (2012). “Environmental factors affecting situation awareness in unmanned aerial vehicles,” in AIAA Infotech@Aerospace Conference (Garden Grove, CA).
Walker, G. H., Stanton, N. A., and Young, M. S. (2008). Feedback and driver situation awareness (SA): a comparison of SA measures and contexts. Transportat. Res. F 11, 282–299. doi: 10.1016/j.trf.2008.01.003
Wheatcroft, J. M., Jump, M., Breckell, A. L., and Adams-White, J. (2017). Unmanned aerial systems (UAS) operators' accuracy and confidence of decisions: professional pilots or video game players? Cogent Psychol. 4, 1–23. doi: 10.1080/23311908.2017.1327628
Wickens, M. (2000). “The trade-off of design for routine and unexpected performance: Implications of situation awareness,” in Situation Awareness Analysis and Measurement, eds M. R. Endsley, D. J. Garland (Boca Raton, FL: CRC Press), 211–225.
Wiegand, G., Schmidmaier, M., Weber, T., Liu, Y., and Hussmann, H. (2019). “I drive - you trust: explaining driving behavior of autonomous cars,” in Extended Abstracts of the 2019 CHI Conference on Human Factors in Computing Systems, paper no. LBW0163 (Glasgow). doi: 10.1145/3290607.3312817
Winter, J. C. F., de, Eisma, Y. B., Cabrall, C. D. D., Hancock, P. A., and Stanton, N. A. (2019). Situation awareness based on eye movements in relation to the task environment. Cogn. Technol. Work 21, 99–111. doi: 10.1007/s10111-018-0527-6
Wu, S., Guo, J., Zeng, S., and Lu, Z. (2019). “Direct measurement of situation awareness in abnormal situation during aircraft landing,” in Proceedings of the 2019 International Conference on Quality, Reliability, Risk, Maintenance, and Safety Engineering (Zhangjiajie), 173–179. doi: 10.1109/QR2MSE46217.2019.9021212
Wulf, F., Zeeb, K., Rimini-Döring, M., Arnon, M., and Gauterin, F. (2013). “Effects of human-machine interaction mechanisms on situation awareness in partly automated driving,” in Proceedings of the 16th International IEEE Annual Conference on Intelligent Transportation Systems (The Hague), 2012–2019. doi: 10.1109/ITSC.2013.6728525
Yanco, H. A., and Drury, J. (2004). “Where am i?” Acquiring situation awareness using a remote robot platform,” in 2004 IEEE International Conference on Systems, Man and Cybernetics, vol. 3 (IEEE Cat. No.04CH37583) (The Hague), 2835–2840. doi: 10.1109/ICSMC.2004.1400762
Yang, Y., Chen, M., Wu, C., Easa, S. M., and Zheng, X. (2020). Structural equation modeling of drivers' situation awareness considering road and driver factors. Front. Psychol. 11:1601. doi: 10.3389/fpsyg.2020.01601
Yanko, M. R., and Spalek, T. M. (2014). Driving with the wandering mind: the effect that mind-wandering has on driving performance. Hum. Factors 56, 260–269. doi: 10.1177/0018720813495280
Zhang, X., Jia, G., and Chen, Z. (2018). “The Literature Review of Human Factors Research on Unmanned Aerial Vehicle – What Chinese Researcher Need to Do Next?,” in Cross-Cultural Design. Methods, Tools, and Users. CCD 2018. Lecture Notes in Computer Science, vol 10911, eds P. L. Rau (Cham: Springer), 375–384. doi: 10.1007/978-3-319-92141-9_29
Zhou, F., Yang, X. J., and Zhang, X. (2020). Takeover transition in autonomous vehicles: a youtube study. Int. J. Hum. Comput. Interact. 36, 295–306. doi: 10.1080/10447318.2019.1634317
Keywords: remotely controlled road vehicles, measurement, traffic safety, human factor, situation awareness, remotely controlled cars, remotely operated cars
Citation: Linkov V and Vanžura M (2021) Situation Awareness Measurement in Remotely Controlled Cars. Front. Psychol. 12:592930. doi: 10.3389/fpsyg.2021.592930
Received: 08 August 2020; Accepted: 22 February 2021;
Published: 20 April 2021.
Edited by:
Oren Musicant, Ariel University, IsraelReviewed by:
Mica Endsley, SA Technologies, United StatesAnat Meir, Holon Institute of Technology, Israel
Copyright © 2021 Linkov and Vanžura. This is an open-access article distributed under the terms of the Creative Commons Attribution License (CC BY). The use, distribution or reproduction in other forums is permitted, provided the original author(s) and the copyright owner(s) are credited and that the original publication in this journal is cited, in accordance with accepted academic practice. No use, distribution or reproduction is permitted which does not comply with these terms.
*Correspondence: Václav Linkov, dmFjbGF2LmxpbmtvdkBjZHYuY3o=