- 1Wageningen Food & Biobased Research, Wageningen University & Research, Wageningen, Netherlands
- 2Wageningen Economic Research, Wageningen University & Research, Wageningen, Netherlands
The primary goal of this study is to be able to discern specific types of consumers in terms of their psychosocial characteristics who may need different ways of receiving dietary advice. Knowing these types will enable a better fit of advice to consumers’ psychosocial characteristics, hereby stimulating healthy eating as the probability of compliance to the advice can potentially increase. The study draws upon several psychological theories to distinguish unique underlying factors that can subsequently be used to personalize nutrition information for consumers. A number of general psychological scales (self-regulation, action and coping self-efficacy, social comparison, intrinsic motivation, health info processing, need for cognition and for affect, and regulatory focus) are filled out by 988 respondents, including their preferences for receiving personalized forms of nutrition advice. The set of joint items from various psychological constructs is analyzed using a Principal Component Analysis to find underlying psychological characteristics. The PCA produces four components (explaining 51% of variation), that could be interpreted as ‘intrinsic interest and capabilities for healthy eating,’ ‘perceived difficulty to eat healthily,’ ‘self-worth insecurity,’ and ‘seeking positive challenges,’ respectively. By means of a Logistic Regression these components are able to predict preferences for different forms of receiving nutrition advice. This first component shows that a mind set for maintaining a healthy diet goes together with an interest in receiving an advice on what do to and on how that will affect one’s health. The second component predicts a preference for a fixed moment to receive information/advice. This may be a strategy of those that perceive difficulties to eat healthily, to help them control their healthy food intake. The insecurity that the third component models seems to lead to a wish for receiving specific advice about their health situation at fixed moments in time. The fourth component is a small component, therefore its prediction of a wish for an advice focussing on prevention of negative consequences is probably not a strong result. The study does point out that there appear different psychosocial types of consumers, that may benefit by being addressed according to their preference for receiving nutrition advice on specific moments, of a specific level of detail or pointing at the type of consequences the advice has. A better fit of the advice to the psychosocial characteristics of the recipient, captured in the identified components in the current study, may lead to an increase in compliance, although that will have to be further investigated in subsequent work.
Introduction
Many people in the Western world currently have an unhealthy lifestyle, which in part is the result of consumers’ diets which overall are relatively unhealthy. For instance because they consume too much meat, have a high (saturated) fat intake, and consume too little fruits and vegetables (Bray and Popkin, 1998; Pomerleau et al., 2005). Such unhealthy diets increase the odds of consumers getting various (chronic) diseases, such as diabetes or cardiovascular diseases (Wing et al., 2001). One type of approach to persuade consumers to eat more healthily is to provide consumers with nutrition information (e.g., Block and Peracchio, 2006; Glanz et al., 2012; Wendel et al., 2013). This nutrition information can be provided to consumers in a generic manner, where all consumers receive the same information (in terms of content and form), or in a more personalized manner. The concept of personalized nutrition in this study entails that nutrition information is provided to consumers taking into account some of their psychological characteristics. Consequently, content and/or form of the information can be personalized based on these characteristics. The aim is that this will make the provided nutrition information more personally relevant (e.g., Brug et al., 1999), in turn leading to a higher compliance. Perceiving more benefits than risks drives this higher compliance and ambivalent feelings and eating context can be barriers (Berezowska et al., 2015; Reinders et al., 2020). Overall, personalizing nutrition information has been shown to be more effective in affecting consumers’ food choices than providing consumers with generic nutrition information (e.g., Brug et al., 2003; Elder et al., 2009; Livingstone et al., 2016; Celis-Morales et al., 2017), which also demonstrates the potential of personalized nutrition advice as a strategy to ultimately reduce the incidence of diseases and consequently health costs (Stewart-Knox et al., 2016).
Nutrition information can be personalized in different ways. A distinction can be made between a biological/medical basis on the one hand and a behavioral/psychological basis on the other hand (Ordovas et al., 2018). The majority of studies that have addressed effects of personalized nutrition on consumers’ food choices have used a biological/medical basis to personalize nutrition information. Examples are a personalized nutrition approach based on blood parameters, anthropometrics and dietary habits (Zeevi et al., 2015), on epigenetics (vel Szic et al., 2015) or on nutrigenomics (Ronteltap et al., 2013; Berezowska et al., 2015).
Behavioral or psychological approaches to personalize nutrition information have been more scarce. Many behavior change techniques used in this field originate from psychological theories (Peters et al., 2015). Psychological characteristics of consumers can strongly affect consumers’ behavior, such as the food choices they make (e.g., Mela, 2001; Gibson, 2006; Köster, 2009), although to our knowledge these psychological insights have not yet been applied to personalize nutrition information. Macready et al. (2018) developed a study protocol for a personalized nutrition approach based on multiple behavior change techniques, which included the Theory of Planned Behavior (Ajzen and Manstead, 2007), social cognitive theory (Bandura, 1989), and the Information-Motivation-Behavioral Skills Model (Fisher et al., 2002). However, identifying which behavior change techniques, based on psychological theories, are most relevant to include in a personalized nutrition intervention is challenging, as the practice of including these is currently still mostly exploratory (Macready et al., 2018).
While each theory will have its own merits, there is overlap between the psychological theories in the sense that they draw on either individual cognitions, capabilities and motivation, or on contextual and/or social variables (Davis et al., 2015). This overlap makes it difficult to pinpoint which factors can be distinguished, as also noted by Macready et al. (2018). The primary goal of this study is to draw upon several psychological theories in an attempt to distinguish unique underlying factors that can subsequently be used to personalize nutrition information for consumers, to ultimately stimulate healthy eating. A secondary goal is to explore the relation between the identified factors and consumers’ preferences for receiving certain forms of dietary advice/information. In this way, we check to what extent the identified factors can provide a clearer and hopefully leaner psychological basis for personalizing nutrition information.
A meta-analysis of Noar et al. (2007) points at several behavior change techniques and psychological characteristics that can be effective for personalized approaches to promote healthy behavior in general (not specifically in relation to nutrition). Their overview, combined with the behavior change techniques and underlying psychological characteristics as used by Macready et al. (2018), provide our starting point to make a selection of psychological theories. Below we provide a short overview of the theories we draw upon, including a short elaboration on why they could be useful for personalizing nutrition information.
We stress that our approach is of an exploratory nature, and not hypothesis-driven. We include a set of scales based on them potentially capturing relevant psychological traits, not based on theoretical considerations about what traits may cause a specific type of consumer to prefer specific types of food and health related information.
Psychological Theories as a Basis for Personalizing Nutrition Information
Personalized nutrition advice is a tool to help individuals regulate their dietary behaviors. In the current study, we take into account various psychological theories focused on individual differences, in terms of capabilities and dispositions that potentially affect preferences for dietary advice and whether the advice will be processed and in turn can affect compliance rates and dietary behaviors. Following an advice mainly involves conscious processes, like goal setting and self-monitoring (Rankin et al., 2017). Social Cognitive Theory (SCT; Bandura, 1977) gives insight into how individuals regulate their behavior to achieve goals that can be maintained over time. Bandura (1991) elaborates that an interaction between personal factors (self-reflective capabilities that give individuals some control over their thoughts, feelings, motivation and actions) and environmental matters (e.g., social influences, role models, behavioral standards) influences self-regulation skills and behavior. Therefore, we included self-regulation, self-efficacy (the extent to which one feels capable of performing a certain behavior) and social comparison in our study. Within the Health Action Process Approach model (HAPA; Schwarzer, 1992, 1999) self-efficacy is further subdivided into action self-efficacy and coping self-efficacy. Action self-efficacy refers to the capability to imagine success scenarios, anticipate potential outcomes of strategies and initiate new behaviors. Coping self-efficacy refers to the capability to deal with barriers that arise. Both action self-efficacy and coping self-efficacy are included in our study. Based on Self-Determination Theory, a motivational theory that gives insight into the reason behind self-regulation (SDT; Ryan and Deci, 2000), we also included intrinsic motivation. Intrinsic motivation is referred to when one is enjoying an activity in itself, without other reasons than performing the activity. Intrinsic motivation has been shown an important predictor of long term behavior change (Ryan and Deci, 2000; Orji et al., 2013).
Personalized advice can increase the effect of a message by achieving a fit between a person and a message. According to the Elaboration Likelihood Model (ELM; Petty and Cacioppo, 1986), information can be processed in two different ways, namely via the content of a message, i.e., based on its meaning (the central processing route) or via the appearance of a message (the peripheral processing route). The central route is used when individuals are motivated, capable and able to attentively take in information. When this is not the case, the peripheral route is used. When it comes to healthy diets, there are differences between individuals, in motivation, capability and ability (e.g., Dibsdall et al., 2003), which according to ELM leads to different information processing routes (Cacioppo et al., 1984; Trumbo and McComas, 2003). Therefore, we included information processing from ELM (central & peripheral information processing) in the current study.
Haddock et al. (2008) found that people differ in their general need to have information about matters (need for cognition) and in their need for affect, through praise and reinforcement. They found that a cognitive message is more persuasive among cognition-oriented individuals (i.e., individuals who have a high need for cognition), whereas an affective message is more persuasive among affect-oriented individuals (i.e., individuals who have a high need for affect). Similar effects were demonstrated by Mayer and Tormala (2010), who suggest that personalizing nutrition information based on peoples’ need for cognition and need for affect can be effective in stimulating people to make more healthy food choices. Therefore, we included the constructs need for affect and need for cognition in our study.
Regulatory Focus Theory posits differences between people in motivational orientation, namely a promotion or prevention focus (Higgins, 1997). People with a promotion focus, focus on achieving positive outcomes and primarily think “How would I like to be?”. People with a prevention focus, focus on preventing negative outcomes and primarily think “How should I be?”. Research shows that framing information in terms of gains is more effective for people with a promotion focus, and using loss-frames is more effective for people with a prevention focus (Lee and Aaker, 2004). The majority of studies in the review by Ludolph and Schulz (2015) confirmed that regulatory fit enhances the effectiveness of health messages. Thus, we also included regulatory focus in our study.
In the current study the items from the above mentioned psychological constructs have been combined in one online survey (see Table 1; more details on the survey can be found in the “Materials and Methods” section).
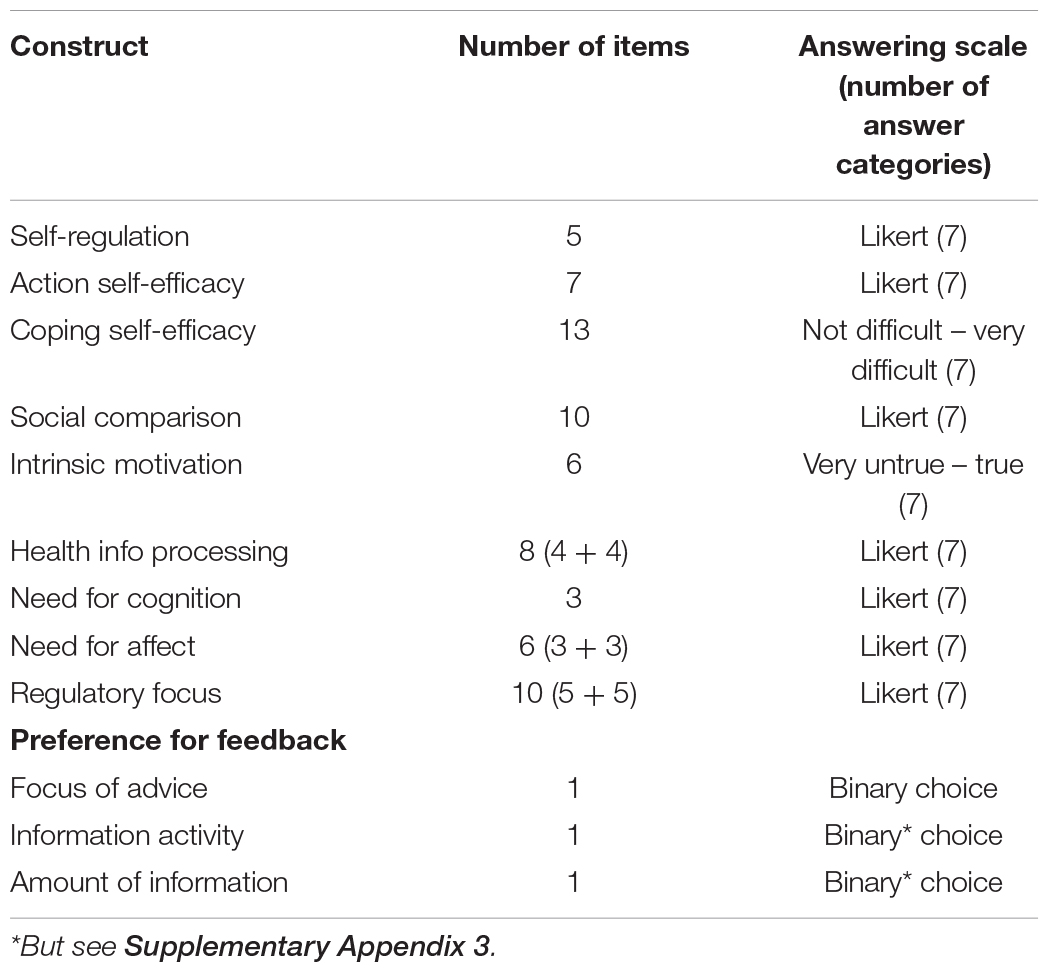
Table 1. Used constructs in the survey, their number of items and the answering scale (and number of answer categories) used (top part); binary questions about the preference for feedback (lower part).
Materials and Methods
Sample and Procedure
An online survey was conducted in August 2018 under 1,013 respondents in the Netherlands. The survey was administered by a professional market research company (MSI-ACI Europe BV). The respondents were approached by email. As the data was gathered anonymously and only average scores were used in the analyses, no formal ethical approval needed to be officially obtained. At the start of the study participants were informed about the global goal of the study namely, to map individuals’ choices and opinions about personalized nutrition advice. The participants were explained that they could refrain from filling out the survey at any moment and could quit without providing any reason for their withdrawal. To ensure a nationally representative sample, respondents were quota-sampled based on gender, age, highest level of completed education and income. Due to a lack of variation in their responses, 25 respondents were excluded1, thus the final sample consisted of 988 respondents with a completely filled out survey. The final sample contained 486 males and 500 females (2 respondents did not fill out their gender) and a mean age of 46.2 years ranging from 18 to 75 years. These, and some more, demographics can be found in Supplementary Appendix 1.
The questionnaire started with a measurement of the psychological characteristics. Respondents then continued with several multiple choice questions which measured their stated preferences for receiving certain forms of personalized nutrition and health advice or information. Finally, the demographics were assessed.
Measures
Validated scales were used to measure the various constructs. When necessary, the items were translated to Dutch using back-translation and reversed items were framed positively, because reversed items have shown to be able to lead to measurement problems like unduly complex factor structures (cf. Weijters and Baumgartner, 2012).
Self-regulation with regard to healthy eating was measured using a 5-item scale developed and validated by Kliemann et al. (2016). The items were answered on seven-point Likert scales ranging from 1 (strongly disagree) to 7 (strongly agree). Action self-efficacy with regard to healthy eating was measured with 7 items (based on Wilson-Barlow et al., 2014). The items were also answered using seven-point Likert scales ranging from 1 (strongly disagree) to 7 (strongly agree). Coping self-efficacy with regard to healthy eating was measured with 13 items from the Eating Self-Efficacy Scale (ESES), based on Glynn and Ruderman (1986). These items were answered on seven-point scales ranging from 1 (not difficult at all) to 7 (very difficult). Social comparison was measured with a 10-item questionnaire from Gibbons and Buunk (1999). The items were answered on seven-point Likert scales ranging from 1 (strongly disagree) to 7 (strongly agree). Intrinsic motivation to eat healthily was measured with 6 items that assessed self-reports of interest and enjoyment of healthy eating (Ryan and Deci, 2000), items were answered on seven-point scales ranging from 1 (very untrue) to 7 (very true). Healthy information processing was measured with 8 items −4 items measured central processing and 4 items measured peripheral processing– based on Trumbo and McComas (2003). Need for cognition was measured with 3 items based on Cacioppo et al. (1984). Need for affect was measured with 3 items that assessed approaching emotions and 3 items that assessed avoiding emotions based on Appel et al. (2012). Regulatory focus was measured with 5 items assessing prevention focus and 5 items assessing promotion focus based on Haws et al. (2010). Items for information processing, need for cognition, need for affect and regulatory focus were all measured with seven-point Likert scales ranging from 1 (strongly disagree) to 7 (strongly agree). An overview of the items in the survey is presented in Table 1.
All constructs and their items, means, standard deviations and Cronbach’s alphas are shown in Supplementary Appendix 2. The Cronbach’s alpha’s are all rather high, ranging from 0.71 to 0.95, indicating that our sample answered like expected with respect to the psychological scales, which were designed to show only one underlying concept.
Three questions were asked concerning the preferred way that respondents would like to receive health advice or feedback about their health status (bottom part of Table 1). The following concepts were probed by these questions about receiving personalized feedback on respondents’ health status or receiving personalized health advice (for the verbatim items, see Supplementary Appendix 3):
• Focus of advice: preference for information on either how to obtain positive results, or on how to prevent negative consequences.
• Information activity: preference for looking for advice when the respondent itself wishes it, or to always receive advice on the same fixed moment.
• Amount of information: preference for short, to-the-point information or for detailed information that includes explanations about why the advice is good for the respondent.
For all three items answer categories with low frequencies (below 10%) were deleted from the analyses (see also Supplementary Appendix 3). The 2nd and 3rd answer category from the ‘amount of information’ item were merged. The reason is the fact that the detailed information mentioned in the 2nd category may for many respondents have included what is specifically named in the 3rd. These questions were measured with a single-item, also to keep the survey relatively concise; in many cases, single-item measures perform equally well as multiple-item measures of the same construct (Bergkvist and Rossiter, 2007).
Analysis of Data
Principal Component Analysis
A Principal Component Analysis (PCA) was conducted on the 68 items in the survey (using SPSS v.23), which resulted in a four dimensional solution on which a Varimax rotation was performed. The scores for this four dimensional model are used to predict reported preferences for three different forms in which dietary health information can be provided to people. These predictions have been carried out separately by means of three logistic regression analyses (using SPSS v.23). The reason to carry out three separate logistic regressions, rather than combining these into one model in order to include interactions between dependent variables is rather practical. One of the aims of this study was to explore the possibility to use the psychological profiles obtained to provide tailored dietary advice in a specialized computer program (ultimately in a smartphone-app). The three dependent variables are linked to matters that could be separately implemented in such an app. Furthermore, in hindsight, the correlations between the three variables turned out to be very low (Supplementary Material).
Results
Principal Component Analysis
The amount of variance in the four component (correlation) PCA model is 51% and the choice for this four component model is not based on a formal criterion like the eigenvalue > 1 criterion (in a full dimensional PCA, without post hoc rotation, this would have yielded a 12 component model with the component 5 through 12 together containing 12% variance). Visual inspection of the scree graph (see Supplementary Material) suggested a four component model, although the fourth component contains a mere 8% of variation. All four components showed an interpretable set of loading items (see Table 2), although the fourth component should be interpreted with caution due to its low amount of explained variance. Loadings with an absolute value < 0.3 are not used to interpret the components nor are they shown in Table 2.
The first component (18% VAF) shows items that concern an intrinsic interest in healthy eating, combined with the will (and capability) to maintain a healthy diet. The first component (18% VAF), coined ‘intrinsic interest and capabilities for healthy eating,’ combines six items from the scale ‘intrinsic motivation for healthy eating,’ seven items from ‘action self-efficacy concerning healthy eating,’ eight items from ‘information processing concerning healthy eating,’ and five items from ‘self-regulation concerning healthy eating.’ These items have loadings over 0.44 on this component.
The second component (13% VAF) contains all items regarding ‘coping self-efficacy’ (Schwarzer and Renner, 2000). These items all concern a difficulty in maintaining a healthy diet, under a broad range of circumstances, which includes a variety of eating occasions and emotional occurrences. These items can therefore be viewed as (a general lack of) coping self-efficacy to eat healthily. We label this second component “perceived difficulty to eat healthily.”
The third component (12% VAF) shows a general attitude which we interpret as a form of ‘self-worth insecurity.’ It contains the ten items from the ‘social comparison’ scale, three ‘emotion avoidance’ items from the ‘need for affect’ scale and one ‘prevention focus’-item from the ‘regulatory focus’ scale. Most items loading on this component (loadings over 0.47) point to a social comparison orientation and an avoidance to experience emotions.
The fourth component contains a mere 8% of variance and contains the three items from the ‘need for cognition’ scale, the three ‘approach emotions’-items from the ‘need for affect’ scale, five ‘promotion focus’-items and one ‘prevention focus’-item from the ‘regulatory focus’ scale. These items have loadings over 0.43 on this component. Thus, the items in this component appear related to seek cognitive challenges, seek positive results and seek the experience of emotions. We interpret and label this component as “seeking positive challenges.” Three other ‘prevention focus’-items also loaded on this component, however, we decided not to take this into consideration because they either had low loadings (below 0.38) or a similar (low) loading on more than one component.
In summary, we have thus reified the four components as follows:
(1) Intrinsic interest and capabilities for healthy eating,
(2) Perceived difficulty to eat healthily,
(3) Self-worth insecurity,
(4) Seeking positive challenges.
Relation Between the Components and Personalized Health Feedback Preferences
The three separate logistic regressions show that some of the four principal components predict some of the three dependent variables. The results of the three logistic regressions are given in Table 3. Significant (p < 0.05) results are indicated by italicizing the corresponding lines in the table.
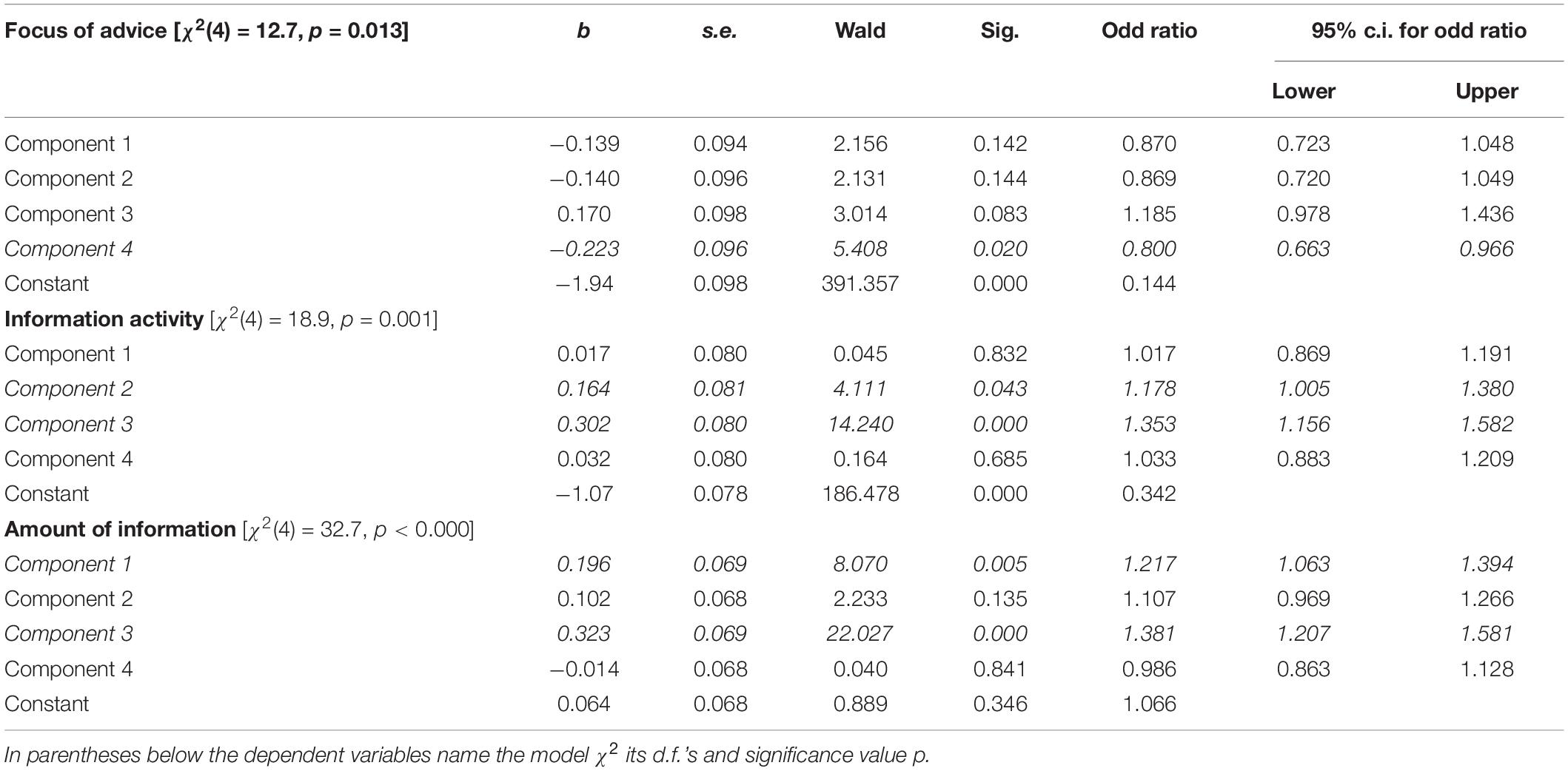
Table 3. Results of the three logistic regressions, Lines holding significant predictions (p < 0.05) italicized [in the table: regression weight b, their standard errors (s.e.), Wald statistics, significance values, odd ratios and the lower and upper borders of the 95% confidence interval for the odd ratios, respectively].
Figure 1 presents an overview of the findings, the extent to which the four components can predict the stated preferences for receiving advice/information.
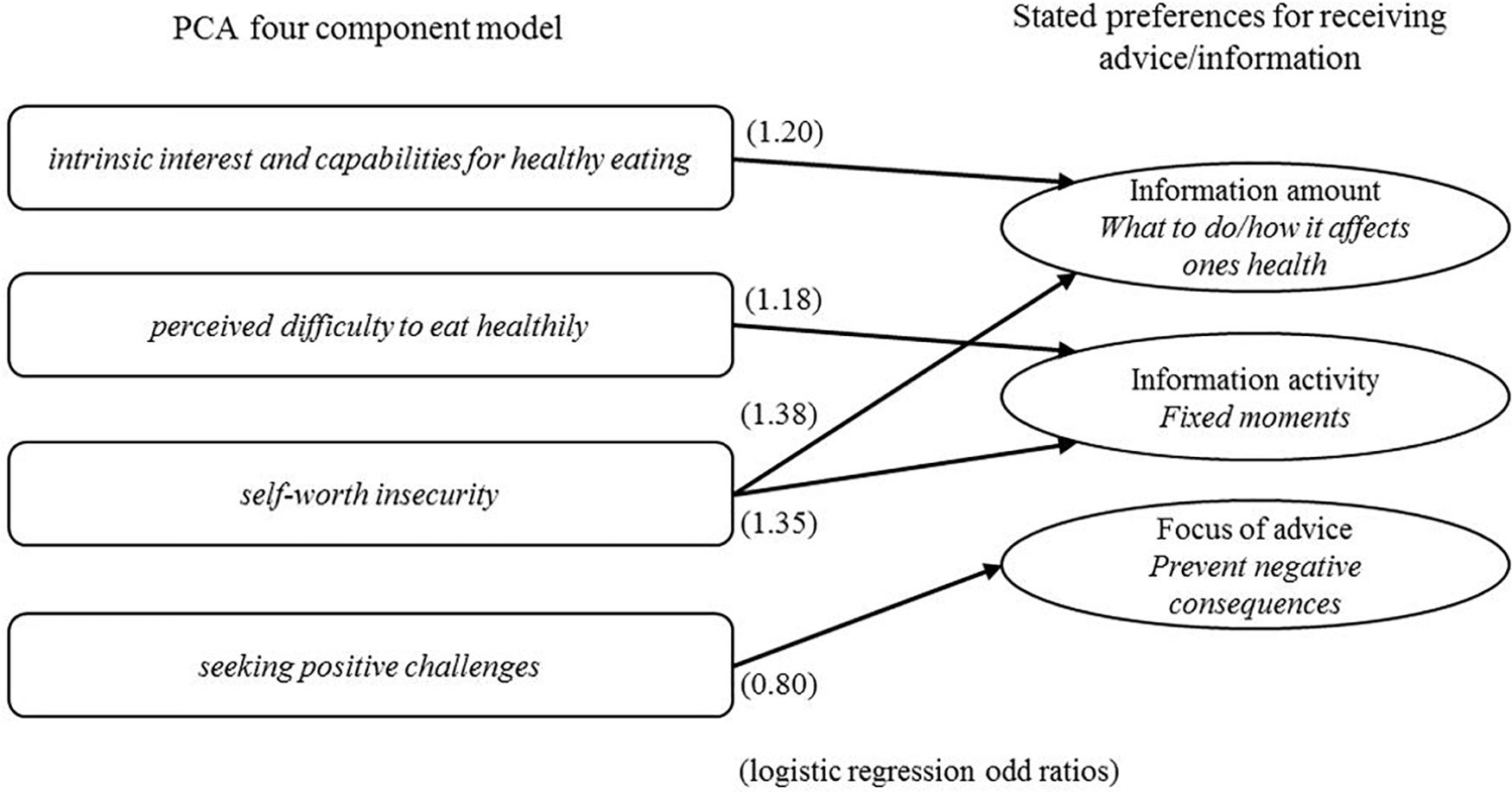
Figure 1. Overview of the significant components (p > 0.05), in three separate logistic regressions, (with odd ratios) predicting preferences for receiving health advice/information.
The logistic regression predicting Focus of advice (a preference to receiving advice pointing at ways to prevent negative consequences) shows a significant effect (p = 0.02) only for the 4th component (‘seeking positive challenges’), with an odd ratio of 0.80. An increase of the score on the 4th component results in a lowering of the probability of preferring to receive advice when it shows how to prevent negative consequences.
Information activity (a preference to receiving information on fixed moments) shows to be predicted by both the 2nd component (perceived difficulty to eat healthily, p = 0.04) and the 3rd component (self-worth insecurity, p = 0.00). A higher score on the 2nd component results in a heightened (odd ratio 1.18) probability for a preference for receiving information on a fixed moment, analogously does a higher score on component 3 with an odd ratio of 1.35.
The third logistic regression shows that amount of information (a preference to receiving detailed information pointing out what to do and how this affects one’s health) can be predicted by the 1st (p = 0.01) and 3rd (p = 0.00) component (‘intrinsic interest and capabilities for healthy eating’ and ‘self-worth insecurity,’ resp.). A higher score on component 1 results in a 1.22 times higher probability of preferring detailed information, a higher score on component 3 in a 1.38 times higher probability of preferring detailed information.
Discussion
Personalizing nutrition information can be an effective manner to increase compliance and lead to consumers making more healthy food choices (e.g., Brug et al., 2003; Elder et al., 2009). However, previous studies have mostly used a biological/medical basis to personalize nutrition information. In the current study, we extend previous work that shows a relation between psychological characteristics and both dietary choices (e.g., Mayer and Tormala, 2010; Ludolph and Schulz, 2015; Macready et al., 2018) and compliance with personalized advice (Berezowska et al., 2015; Livingstone et al., 2016; Celis-Morales et al., 2017; Rankin et al., 2017; Reinders et al., 2020) and aim to develop a psychological approach to personalize nutrition information based on an elaborate questionnaire (Macready et al., 2018). Furthermore, the current study also explores the relation between the (psychological) components and respondent preferences for certain forms in which dietary information can be provided. The latter provides an indication of the degree to which this psychological approach to personalize nutrition information can be a basis to predict respondent preferences for how respondents would like to receive nutrition information (e.g., in detailed form or not). Ultimately the findings can be used for the development of smartphone apps by which consumers can choose to receive dietary advice in a manner befitting their psychological profile.
Principal Components
In the current study, we conducted a PCA which delivered four components: intrinsic interest and capabilities for healthy eating, perceived difficulty to eat healthily, self-worth insecurity and seeking positive challenges.
The first component (‘intrinsic interest and capabilities for healthy eating’) combines all the items from the action self-efficacy, self-regulation, intrinsic motivation and information processing scales. That the four scales jointly load on the first component in this study, hence correlate, can mean that they measure the same underlying construct in the context of food and healthy eating. Scores on this component increase as respondents have a higher intrinsic motivation and a higher involvement in eating healthily (this is the central route in the ELM of Cacioppo et al., 1984), a higher action self-efficacy and a higher self-regulation. We interpret this as the higher people score on this component, the more they show a combination of an intrinsic interest and having the capabilities to eat healthily. Intrinsic motivation and a high involvement in a certain issue pertain to an intrinsic interest, in this case for healthy eating. Action self-efficacy and self-regulation pertain to having the capability to eat healthily, according to respondents’ own perception. Self-efficacy has also been seen as a motivational aspect of self-regulation (Dinsmore et al., 2008), which theoretically connects action self-efficacy to both self-regulation and intrinsic motivation.
Motivation and capability are often treated as separate concepts in behavioral theories (e.g., Social Cognitive Theory, Bandura, 1977; Health Action Process Approach model, Schwarzer, 1992, 1999; Theory of Planned Behaviour Ajzen and Manstead, 2007). However, the two concepts are important in the sense that behavioral models such as the Motivation, Opportunity, Ability (MOA) model (Ölander and Thøgersen, 1995) state, that behavior change is more likely when an individual is both motivated and capable to change one’s behavior.
Based on this first PCA-component, it can be suggested that the motivational aspect of self-regulation, is indeed tied to respondents’ level of self-efficacy to change their diet. Self-regulation also has a more cognitive part, which in this component is reflected in the form of information processing. Previous work has linked information processing with self-regulation, specifically with regard to self-regulated learning (e.g., Winne, 2001). This can be interpreted for the current study, as respondents aiming to learn how to eat more healthily in a self-regulated manner, and processing the necessary information in order to do so.
Items referring to difficulties with following a healthy diet load together on the second component (‘perceived difficulty to eat healthily’). These items all stem from the same self-efficacy scale (Glynn and Ruderman, 1986). That they score uniquely on this component, and apparently do not clearly correlate with any other items in this survey shows that they act as a single force in the whole of the survey in this study. There thus seems to be a general trend which is strong enough to surface in our sample of 988 respondents. This is not in line with the original scale (Glynn and Ruderman, 1986), which comprises of two components, namely difficulty eating healthily when experiencing negative affect (NA) and difficulty eating healthily during socially acceptable circumstances (SAC). In the specific food and health context of this survey these two components may not have been distinguished by the respondents, in the sense that difficulty to eat healthily in terms of NA and SAC appears to be connected to each other. This may indicate that the component structure can depend on the specific context of this survey.
The fact that the first and second component are built from domain-specific items explicitly concerning food and/or health is noteworthy. This may point at a methodological artifact where items that are comparably stated, or are on similar matters, are scored alike. The reason that the first and second component are separate components, can be explained by the fact that the components have a different focus (Bandura, 1977). Items in the second component focus on the difficulty to perform healthy dietary behaviors, whereas the items in the first component focus on positive motivations and capabilities with regard to eating healthily. And as mentioned before, reversed items often result in a separate component (Weijters and Baumgartner, 2012).
Most items loading on the third component (‘self-worth insecurity’) point to psychosocial characteristics of a type of individual that compares his/her behavior to others in order to judge his/her own behavior, and at an avoidance to experience emotions. One reason why people might structurally compare themselves to others is that they are insecure about themselves (Maslow et al., 1945; White et al., 2006) and have a lower self-worth (Crocker and Wolfe, 2001). Similarly, people attempt to avoid emotions when emotional lows are expected that affect one’s self-worth (Crocker and Knight, 2005), thus avoiding emotions can be tied to maintaining one’s self-worth.
The fourth component (‘seeking positive challenges’) combines items from the ‘need for cognition’ scale, the ‘approach emotions’-items from the ‘need for affect’ scale, and ‘promotion focus’-items and ‘prevention focus’-item from the ‘regulatory focus’ scale. The latter two sets of items are not easy to reconcile as this component seems to combine a promotion focus with a prevention focus, which are traits believed to be not in line with each other. We must again stress that the fourth component represents a rather low amount of variance in the data, so it is does not represent a very strong force to explain this result. Nevertheless it appeared interpretable as ‘seeking positive challenges’, and we have included it in the prediction models.
We observe that the first two components are food related components. Component 3 and 4 are related to general psychosocial characteristics, which are not specifically related to food. This suggests that, in these results, answers to the food related items are unrelated to answers to general items, as the four components are uncorrelated (the varimax rotation maintains orthogonality of the PCA components).
Prediction of Feedback Preferences
This first component (‘intrinsic interest and capabilities for healthy eating’) shows up as predictor of the ‘information amount’ in the logistic regression. A mind set for maintaining a healthy diet seems to go together with an interest in receiving an advice/information on what do to, together with information on how the advice will affect one’s health, compared to only receiving an advice. So individuals who have intrinsic interest and capabilities to eat healthily prefer extra information on why an advice is good for their health in addition to receiving dietary advice.
The second component (‘perceived difficulty to eat healthily’), tallies with it predicting a preference for a fixed moment to receive information/advice. This may be a strategy of those that perceive a difficulty in themselves, to help them control their healthy food intake. They may see themselves unable to cope with a freedom to find information on their own account, hence making it more likely that a form of advice is chosen that is received on a fixed moment.
Component 3 (‘self-worth insecurity’), together with the first component, predicts a preference to receiving information/advice together with instructions about what to do and how it relates to one’s health. Component 3, together with the second component, also predicts a preference for a fixed moment to receive information/advice. The insecurity of the individuals scoring high on this component seems to lead to a wish for specific information/advice about their health situation at fixed moments in time. The social comparison part of the third component, seems to be in line with wanting to receive specific information and instruction with the advice, as the advice comes from others, which enables a social comparison.
The fourth component negatively predicts the preference for advice with a focus on preventing negative consequences. As this component models ‘seeking positive challenges’, this seems to make sense, with the proviso that the merger of both a prevention and a promotion focus appears strange. Note that this component contains a mere 8% variance in the data, so this component’s size is not very large, which may lead to the possibility that it combines small effects, despite their being theoretically not compatible.
That four scales, adapted to focus on food and health, together make up the first component suggests that these four underlying constructs merge when seen in the context of food and health. Intrinsic motivation, self-efficacy, information processing and self-regulation appear to correlate highly when seen in the context of food and health. This points at the possibility to reduce the set of items in future surveys designed to probe relevant psychological parameters of a sample of respondents, in a food and health context.
Study Limitations
One potential limitation of the study is that for the ‘preference for feedback’ constructs single-item measures were used. However, in many cases single-item measures perform just as well as multiple-item measures (Bergkvist and Rossiter, 2007). Since the psychological constructs are relatively complex, we decided to use validated, multiple-item scales for those constructs, but to use single-item measures for the more simple choice constructs. Also keeping in mind the need to keep the survey relatively concise. Future research could test whether the findings of the current study are similar when also using multiple-item measures for the choice constructs.
Furthermore, though we aimed to keep the survey concise, potentially part of the respondents could have experienced a sense of respondent fatigue. Typically, an average maximum length of 20 min is recommended for a survey before respondent fatigue becomes an issue (Cape and Phillips, 2015; Revilla and Ochoa, 2017). This was taken into account when designing the survey, also by limiting the number of items. It was also agreed with the market research company before the survey was sent out to double-check the survey would take no longer than 20 min to complete.
For the current study, an online survey was administered relying on self-reports from participants. This can have certain disadvantages as is discussed by McDonald (2008), such as for instance participants responding in manners through which they can view themselves as favorable. To account for these potential drawbacks of self-reports, follow-up research can include actual behavioral measures with actual consequences, such as performing a study where the outcome variable is not a self-reported choice, but actually choosing a certain format of nutrition advice that participants subsequently receive.
Conclusion
This study shows that it is possible to use psychological characteristics to predict the way consumers would like to receive advice/information about their health status (and diet). It also points out that there are different personalities of consumers, that may benefit from being addressed according to their preference for receiving advice/information on specific moments, of a specific level of detail and pointing at the type of consequences the advice has. When developing a computer program (typically a smartphone app) it is advisable to tailor the way health advice is provided to the type of consumer. The latter can be assessed by, e.g., asking the user of the app some questions upon installation of the app. The long survey in this study is not suited for this, but the PCA points at a way to reduce the length to a low number of questions.
Compliance to an advice was not addressed in this study, obviously that is the ultimate ‘proof of the pudding’ that needs to be further investigated, possibly with the survey presented here as an instrument for tailoring advice. Based on the four component PCA model a reduction of items can be achieved, to in a more concise manner personalize nutrition advice. Of course this basically means the construction of a new psychological scale, specifically focused at ‘food and health’-related psychological characteristics. Such a scale will need to be studied and analyzed in detail before it can validly be applied in subsequent studies in this field.
Data Availability Statement
The raw data supporting the conclusions of this article will be made available by the authors, without undue reservation.
Ethics Statement
Ethical review and approval was not required for the study on human participants in accordance with the local legislation and institutional requirements. Written informed consent for participation was not required for this study in accordance with the national legislation and the institutional requirements.
Author Contributions
All authors listed have made a substantial, direct and intellectual contribution to the work, and approved it for publication.
Funding
This research was conducted within the collaboration project ‘PPP Personalised Nutrition and Health,’ co-funded through TKI AgriFood and Health-Holland, Top Sector Life Sciences & Health (TKI-AF-15262).
Conflict of Interest
The authors declare that the research was conducted in the absence of any commercial or financial relationships that could be construed as a potential conflict of interest.
Acknowledgments
The authors thank Machiel Reinders and Esmée Doets for comments on earlier versions of the manuscript.
Supplementary Material
The Supplementary Material for this article can be found online at: https://www.frontiersin.org/articles/10.3389/fpsyg.2021.575465/full#supplementary-material
Footnotes
- ^ These 25 respondents had 0 standard deviation in their responses to items measuring regulatory focus (prevention focus vs. promotion focus) and/or need for affect (approach vs. avoidance of affect), in combination with scoring only ‘1,’ ‘2,’ ‘6,’ or ‘7’ in all these responses. Given that these two constructs contain opposite items (e.g., A ‘7’ in one item indicates a high prevention focus, while a ‘7’ in a next item indicates a high promotion focus), these 25 respondents were excluded.
References
Ajzen, I., and Manstead, A. S. (2007). “Changing health-related behaviours: An approach based on the theory of planned behaviour,” in The Scope of Social Psychology, eds M. Hewstone, H. A. W. Schut, J. B. F. De Wit, K. Van Den Bos, and M. S. Stroebe (New York, NY: Psychology Press), 55–76.
Appel, M., Gnambs, T., and Maio, G. R. (2012). A short measure of the need for affect. J. Pers. Assess. 94, 418–426. doi: 10.1080/00223891.2012.666921
Bandura, A. (1977). Self-efficacy: toward a unifying theory of behavioral change. Psychol. Rev. 84, 191–215. doi: 10.1037/0033-295x.84.2.191
Bandura, A. (1989). Human agency in social cognitive theory. Am. Psychol. 44:1175. doi: 10.1037/0003-066x.44.9.1175
Bandura, A. (1991). Social cognitive theory of self-regulation. Organ. Behav. Hum. Decis. Process. 50, 248–287. doi: 10.1016/0749-5978(91)90022-l
Berezowska, A., Fischer, A. R., Ronteltap, A., Lans, I. A., and Trijp, H. C. (2015). Consumer adoption of personalised nutrition services from the perspective of a risk–benefit trade-off. Genes Nutr. 10:42.
Bergkvist, L., and Rossiter, J. R. (2007). The predictive validity of multiple-item versus single-item measures of the same constructs. J. Mark. Res. 44, 175–184. doi: 10.1509/jmkr.44.2.175
Block, L. G., and Peracchio, L. A. (2006). The calcium quandary: how consumers use nutrition labels. J. Publ. Policy Mark. 25, 188–196. doi: 10.1509/jppm.25.2.188
Bray, G. A., and Popkin, B. M. (1998). Dietary fat intake does affect obesity! Am. J. Clin. Nutr. 68, 1157–1173. doi: 10.1093/ajcn/68.6.1157
Brug, J., Campbell, M., and van Assema, P. (1999). The application and impact of computer-generated personalized nutrition education: a review of the literature. Patient Educ. Couns. 36, 145–156. doi: 10.1016/s0738-3991(98)00131-1
Brug, J., Oenema, A., and Campbell, M. (2003). Past, present, and future of computer-tailored nutrition education. Am. J. Clin. Nutr. 77, 1028S–1034S.
Cacioppo, J. T., Petty, R. E., and Feng Kao, C. (1984). The efficient assessment of need for cognition. J. Pers. Assess. 48, 306–307. doi: 10.1207/s15327752jpa4803_13
Cape, P., and Phillips, K. (2015). Questionnaire Length and Fatigue Effects: The Latest Thinking and Practical Solutions. White paper. Available online at: www.surveysampling.com/site/assets/files/1586/questionnaire-length-and-fatiigue-effects-the-latest-thinking-and-practicalsolutions.pdf (accessed October 29, 2020).
Celis-Morales, C., Livingstone, K. M., Marsaux, C. F., Macready, A. L., Fallaize, R., O’Donovan, C. B., et al. (2017). Effect of personalized nutrition on health-related behaviour change: evidence from the food4me european randomized controlled trial. Int. J. Epidemiol. 46, 578–588.
Crocker, J., and Knight, K. M. (2005). Contingencies of self-worth. Curr. Dir. Psychol. Sci. 14, 200–203.
Davis, R., Campbell, R., Hildon, Z., Hobbs, L., and Michie, S. (2015). Theories of behaviour and behaviour change across the social and behavioural sciences: a scoping review. Health Psychol. Rev. 9, 323–344. doi: 10.1080/17437199.2014.941722
Dibsdall, L. A., Lambert, N., Bobbin, R. F., and Frewer, L. J. (2003). Low-income consumers’ attitudes and behaviour towards access, availability and motivation to eat fruit and vegetables. Public Health Nutr. 6, 159–168. doi: 10.1079/phn2002412
Dinsmore, D. L., Alexander, P. A., and Loughlin, S. M. (2008). Focusing the conceptual lens on metacognition, self-regulation, and self-regulated learning. Educ. Psychol. Rev. 20, 391–409. doi: 10.1007/s10648-008-9083-6
Elder, J. P., Ayala, G. X., Slymen, D. J., Arredondo, E. M., and Campbell, N. R. (2009). Evaluating psychosocial and behavioral mechanisms of change in a tailored communication intervention. Health Educ. Behav. 36, 366–380. doi: 10.1177/1090198107308373
Fisher, J. D., Fisher, W. A., Bryan, A. D., and Misovich, S. J. (2002). Information-motivation-behavioral skills model-based HIV risk behavior change intervention for inner-city high school youth. Health Psychol. 21:177. doi: 10.1037/0278-6133.21.2.177
Gibbons, F. X., and Buunk, B. P. (1999). Individual differences in social comparison: development of a scale of social comparison orientation. J. Pers. Soc. Psychol. 76, 129. doi: 10.1037/0022-3514.76.1.129
Gibson, E. L. (2006). Emotional influences on food choice: sensory, physiological and psychological pathways. Physiol. Behav. 89, 53–61. doi: 10.1016/j.physbeh.2006.01.024
Glanz, K., Hersey, J., Cates, S., Muth, M., Creel, D., Nicholls, J., et al. (2012). Effect of a nutrient rich foods consumer education program: results from the nutrition advice study. J. Acad. Nutr. Diet. 112, 56–63. doi: 10.1016/j.jada.2011.08.031
Glynn, S. M., and Ruderman, A. J. (1986). The development and validation of an eating self-efficacy scale. Cogn. Ther. Res. 10, 403–420. doi: 10.1007/bf01173294
Haddock, G., Maio, G. R., Arnold, K., and Huskinson, T. (2008). Should persuasion be affective or cognitive? The moderating effects of need for affect and need for cognition. Pers. Soc. Psychol. Bull. 34, 769–778. doi: 10.1177/0146167208314871
Haws, K. L., Dholakia, U. M., and Bearden, W. O. (2010). An assessment of chronic regulatory focus measures. J. Mark. Res. 47, 967–982. doi: 10.1509/jmkr.47.5.967
Higgins, E. T. (1997). Beyond pleasure and pain. Am. Psychol. 52, 1280–1300. doi: 10.1037/0003-066x.52.12.1280
Kliemann, N., Beeken, R. J., Wardle, J., and Johnson, F. (2016). Development and validation of the self-regulation of eating behaviour questionnaire for adults. Int. J. Behav. Nutr. Phys. Act. 13:87.
Köster, E. P. (2009). Diversity in the determinants of food choice: a psychological perspective. Food Q. Prefer. 20, 70–82. doi: 10.1016/j.foodqual.2007.11.002
Lee, A. Y., and Aaker, J. L. (2004). Bringing the frame into focus: the influence of regulatory fit on processing fluency and persuasion. J. Pers. Soc. Psychol. 86, 205–218. doi: 10.1037/0022-3514.86.2.205
Livingstone, K. M., Celis-Morales, C., Navas-Carretero, S., San-Cristobal, R., O’Donovan, C. B., Forster, H., et al. (2016). Profile of European adults interested in internet-based personalised nutrition: the Food4Me study. Eur. J. Nutr. 55, 759–769. doi: 10.1007/s00394-015-0897-y
Ludolph, R., and Schulz, P. J. (2015). Does regulatory fit lead to more effective health communication? A systematic review. Soc. Sci. Med. 128, 142–150. doi: 10.1016/j.socscimed.2015.01.021
Macready, A. L., Fallaize, R., Butler, L. T., Ellis, J. A., Kuznesof, S., Frewer, L. J., et al. (2018). Application of behavior change techniques in a personalized nutrition electronic health intervention study: protocol for the web-based Food4Me randomized controlled trial. JMIR Res. Protoc. 7:e87. doi: 10.2196/resprot.8703
Maslow, A. H., Hirsh, E., Stein, M., and Honigmann, I. (1945). A clinically derived test for measuring psychological security-insecurity. J. Gen. Psychol. 33, 21–41. doi: 10.1080/00221309.1945.10544493
Mayer, N. D., and Tormala, Z. L. (2010). “Think” versus “feel” framing effects in persuasion. Pers. Soc. Psychol. Bull. 36, 443–454. doi: 10.1177/0146167210362981
McDonald, J. D. (2008). Measuring personality constructs: the advantages and disadvantages of self-reports, informant reports and behavioural assessments. Enquire 1, 1–19.
Mela, D. J. (2001). Determinants of food choice: relationships with obesity and weight control. Obes. Res. 9, 249S–255S.
Noar, S. M., Benac, C. N., and Harris, M. S. (2007). Does tailoring matter? meta-analytic review of tailored print health behavior change interventions. Psychol. Bull. 133, 673–693. doi: 10.1037/0033-2909.133.4.673
Ölander, F., and Thøgersen, J. (1995). Understanding of consumer behaviour as a prerequisite for environmental protection. J. Consum. Policy 18, 345–385. doi: 10.1007/BF01024160
Ordovas, J. M., Ferguson, L. R., Tai, E. S., and Mathers, J. C. (2018). Personalised nutrition and health. BMJ 361:bmj–k2173.
Orji, R., Vassileva, J., and Mandryk, R. L. (2013). LunchTime: a slow-casual game for long-term dietary behavior change. Pers. Ubiquit. Comput. 17, 1211–1221. doi: 10.1007/s00779-012-0590-6
Peters, G. J. Y., De Bruin, M., and Crutzen, R. (2015). Everything should be as simple as possible, but no simpler: towards a protocol for accumulating evidence regarding the active content of health behaviour change interventions. Health Psychol. Rev. 9, 1–14. doi: 10.1080/17437199.2013.848409
Petty, R. E., and Cacioppo, J. T. (1986). “The elaboration likelihood model of persuasion”, in Communication and Persuasion (New York, NY: Springer), 1–24.
Pomerleau, J., Lock, K., Knai, C., and McKee, M. (2005). Interventions designed to increase adult fruit and vegetable intake can be effective: a systematic review of the literature. J. Nutr. 135, 2486–2495. doi: 10.1093/jn/135.10.2486
Rankin, A., Kuznesof, S., Frewer, L. J., Orr, K., Davison, J., de Almeida, M. D., et al. (2017). Public perceptions of personalised nutrition through the lens of Social Cognitive Theory. J. Health. Psychol. 22, 1233–1242. doi: 10.1177/1359105315624750
Reinders, M. J., Bouwman, E. P., Van Den Puttelaar, J., and Verain, M. C. (2020). Consumer acceptance of personalised nutrition: the role of ambivalent feelings and eating context. PLoS One 15:e0231342. doi: 10.1371/journal.pone.0231342
Revilla, M., and Ochoa, C. (2017). Ideal and maximum length for a web survey. Int. J. Mark. Res. 59, 557–565.
Ronteltap, A., Trijp, H., Berezowska, A., and Goossens, J. (2013). Nutrigenomics-based personalised nutritional advice: in search of a business model? Genes Nutr. 8:153. doi: 10.1007/s12263-012-0308-4
Ryan, R. M., and Deci, E. L. (2000). Intrinsic and extrinsic motivations: classic definitions and new directions. Contemp. Educ. Psychol. 25, 54–67. doi: 10.1006/ceps.1999.1020
Schwarzer, R. (1992). “Self-efficacy in the adoption and maintenance of health behaviors: theoretical approaches and a new model,” in Self-Efficacy: Thought Control of Action, ed. R. Schwarzer (Washington, DC: Hemisphere), 217–242.
Schwarzer, R. (1999). Self-regulatory processes in the adoption and maintenance of health behaviors. J. Health Psychol. 4, 115–127. doi: 10.1177/135910539900400208
Schwarzer, R., and Renner, B. (2000). Social-cognitive predictors of health behavior: action self-efficacy and coping self-efficacy. Health Psychol. 19:487. doi: 10.1037/0278-6133.19.5.487
Stewart-Knox, B. J., Markovina, J., Rankin, A., Bunting, B. P., Kuznesof, S., Fischer, A. R. H., et al. (2016). Making personalised nutrition the easy choice: creating policies to break down the barriers and reap the benefits. Food Policy 63, 134–144. doi: 10.1016/j.foodpol.2016.08.001
Trumbo, C. W., and McComas, K. A. (2003). The function of credibility in information processing for risk perception. Risk Anal. Int. J. 23, 343–353. doi: 10.1111/1539-6924.00313
vel Szic, K. S., Declerck, K., Vidakoviæ, M., and Berghe, W. V. (2015). From inflammaging to healthy aging by dietary lifestyle choices: is epigenetics the key to personalized nutrition? Clin. Epigenet. 7:33.
Weijters, B., and Baumgartner, H. (2012). Misresponse to reversed and negated items in surveys: a review. J. Mark. Res. 49, 737–747. doi: 10.1509/jmr.11.0368
Wendel, S., Dellaert, B. G., Ronteltap, A., and van Trijp, H. C. (2013). Consumers’ intention to use health recommendation systems to receive personalized nutrition advice. BMC Health Serv. Res. 13:126. doi: 10.1186/1472-6963-13-126
White, J. B., Langer, E. J., Yariv, L., and Welch, J. C. (2006). Frequent social comparisons and destructive emotions and behaviors: the dark side of social comparisons. J. Adult Dev. 13, 36–44. doi: 10.1007/s10804-006-9005-0
Wilson-Barlow, L., Hollins, T. R., and Clopton, J. R. (2014). Construction and validation of the healthy eating and weight self-efficacy (HEWSE) scale. Eating Behav. 15, 490–492. doi: 10.1016/j.eatbeh.2014.06.004
Wing, R. R., Goldstein, M. G., Acton, K. J., Birch, L. L., Jakicic, J. M., Sallis, J. F., et al. (2001). Behavioral science research in diabetes: lifestyle changes related to obesity, eating behavior, and physical activity. Diabetes Care 24, 117–123. doi: 10.2337/diacare.24.1.117
Winne, P. H. (2001). “Self-regulated learning viewed from models of information processing”, in Self-Regulated Learning and Academic Achievement: Theoretical Perspectives, 2, 153–189.
Keywords: health advice, nutrition advice, psychological scales, personalized nutrition and health advice, psychosocial characteristics
Citation: Dijksterhuis GB, Bouwman EP and Taufik D (2021) Personalized Nutrition Advice: Preferred Ways of Receiving Information Related to Psychological Characteristics. Front. Psychol. 12:575465. doi: 10.3389/fpsyg.2021.575465
Received: 23 June 2020; Accepted: 30 April 2021;
Published: 22 June 2021.
Edited by:
Mark Conner, University of Leeds, United KingdomReviewed by:
Valentina Carfora, Catholic University of the Sacred Heart, ItalyDaniela Caso, University of Naples Federico II, Italy
Copyright © 2021 Dijksterhuis, Bouwman and Taufik. This is an open-access article distributed under the terms of the Creative Commons Attribution License (CC BY). The use, distribution or reproduction in other forums is permitted, provided the original author(s) and the copyright owner(s) are credited and that the original publication in this journal is cited, in accordance with accepted academic practice. No use, distribution or reproduction is permitted which does not comply with these terms.
*Correspondence: Garmt B. Dijksterhuis, ZTpnYXJtdC5kaWprc3Rlcmh1aXNAd3VyLm5s