- 1Human Physiology and Sports Physiotherapy Research Group, Faculty of Physical Education and Physiotherapy, Vrije Universiteit Brussel, Brussels, Belgium
- 2Experimental Anatomy Research Group, Faculty of Physical Education and Physiotherapy, Vrije Universiteit Brussel, Brussels, Belgium
- 3Department of Physiotherapy, International University of Applied Sciences THIM, Landquart, Switzerland
- 4Faculty of Applied Engineering, Universiteit Antwerpen, Antwerp, Belgium
- 5Center for Human Movement Sciences, University Medical Center Groningen, University of Groningen, Groningen, Netherlands
- 6School of Sport Studies, Hanze University Groningen, Groningen, Netherlands
- 7Exercise Science and Neuroscience Unit, Department Exercise and Health, Faculty of Science, University of Paderborn, Paderborn, Germany
- 8Amsterdam Collaboration on Health and Safety in Sports, Amsterdam Universitair Medische Centra, Department of Public and Occupational Health, Amsterdam Movement Sciences, Vrije Universiteit Amsterdam, Amsterdam, Netherlands
- 9Epidemiology and Public Health, Sciensano, Brussels, Belgium
- 10Rehabilitation Research Laboratory 2rLab, Department of Business Economics, Health and Social Care, University of Applied Sciences and Arts of Southern Switzerland, Landquart/Manno, Switzerland
Background: Differential learning (DL) is a motor learning method characterized by high amounts of variability during practice and is claimed to provide the learner with a higher learning rate than other methods. However, some controversy surrounds DL theory, and to date, no overview exists that compares the effects of DL to other motor learning methods.
Objective: To evaluate the effectiveness of DL in comparison to other motor learning methods in the acquisition and retention phase.
Design: Systematic review and exploratory meta-analysis.
Methods: PubMed (MEDLINE), Web of Science, and Google Scholar were searched until February 3, 2020. To be included, (1) studies had to be experiments where the DL group was compared to a control group engaged in a different motor learning method (lack of practice was not eligible), (2) studies had to describe the effects on one or more measures of performance in a skill or movement task, and (3) the study report had to be published as a full paper in a journal or as a book chapter.
Results: Twenty-seven studies encompassing 31 experiments were included. Overall heterogeneity for the acquisition phase (post-pre; I2 = 77%) as well as for the retention phase (retention-pre; I2 = 79%) was large, and risk of bias was high. The meta-analysis showed an overall small effect size of 0.26 [0.10, 0.42] in the acquisition phase for participants in the DL group compared to other motor learning methods. In the retention phase, an overall medium effect size of 0.61 [0.30, 0.91] was observed for participants in the DL group compared to other motor learning methods.
Discussion/Conclusion: Given the large amount of heterogeneity, limited number of studies, low sample sizes, low statistical power, possible publication bias, and high risk of bias in general, inferences about the effectiveness of DL would be premature. Even though DL shows potential to result in greater average improvements between pre- and post/retention test compared to non-variability-based motor learning methods, more high-quality research is needed before issuing such a statement. For robust comparisons on the relative effectiveness of DL to different variability-based motor learning methods, scarce and inconclusive evidence was found.
Introduction
Motor learning is a set of processes associated with practice or experience leading to relatively permanent gains in the capability for skilled performance (Schmidt and Lee, 2013). From an applied point of view, the focus of motor learning is on how different practice variables impact performance to lead to relatively permanent changes in capability. Differential learning (DL) is a motor learning method that was proposed in 1999 (Schöllhorn, 1999) and considers learning of a movement or action as being dependent on the amount of noise (practice variability) that accompanies the acquisition process (etiology: learning from differences).
Traditional (= non-variability based) motor learning (TL) methods include, for instance, repetitive practice (REP) (Gentile, 1972) or methodological series of exercises (MSE) (Djatschkow, 1973) wherein practice variability is minimized to natural movement variability and a fixed progression of exercises. In contrast, methods such as variable practice (VP) (Schmidt, 1975), contextual interference (CtIt) (Shea and Morgan, 1979), DL (Schöllhorn et al., 2010a), structural learning (SL) (Braun et al., 2010; Hossner et al., 2016b), or the constraint-led approach (CLA) (Renshaw et al., 2010) utilize practice variability in an attempt to further enhance motor learning outcomes. Schöllhorn et al. (2009a) depicted these various motor learning methods in a continuum of increasing variability and noise, with optimal variability levels being dependent on subject and situational constraints (Schöllhorn and Horst, 2020). In practice, however, these different theoretical concepts are often merged when trainers or clinicians aim to improve the motor performance of athletes or patients.
DL distinguishes itself from the other methods in the sense that its rationale is based on the rebuttal of two implicit assumptions in other methods, namely, (1) the to-be-learned movement is considered independent of the individual and time, and (2) the movement performance can be improved by repetitions of (invariant parts of) the movement (Schöllhorn et al., 2010a). In brief, this implies that practicing a movement needs to be done in many varieties and thus no exact repetition, and without corrective feedback on the movement pattern (Hackfort et al., 2019). An example of Peter Valentiner utilizing the DL approach in shot put training can be found online1 and implies that the athlete continuously varies the technique used in an attempt to explore movement patterns to discover what works best.
The inspiration for DL's crucial role of practice variability in learning comes from principles of self-organization and dynamical systems theory (Schöllhorn, 2000; Frank et al., 2008) and the concept of stochastic resonance. Although not a central component in the DL theory (Schöllhorn, 2016), the following explanations can be found on the concept of stochastic resonance: “With an increasing number of offered exercises the probability increases of having one exercise for every group member where s/he will respond to in an adequate way” (Schöllhorn, 2000). “By confronting athletes with a high number of practice activities, the probability increases that any of the training exercises can get in resonance with the athlete's needs” (Schöllhorn et al., 2006). Here, the rationale is for DL exercises to cover a maximal range (or plausible range) of motion patterns in order to maximize the chance that they get in resonance with the individual and time-dependent optimum. In other words, the learner discovers useful components during the exploration of various movement executions that are beneficial for the learner's specific constraints at that time point.
However, the theory and mechanism behind the DL method is not undebated (Schoner, 1995; Scholz and Schöner, 1999; Latash et al., 2007; Beek, 2011; Künzell and Hossner, 2012, 2013; Schmidt and Hennig, 2012; Willimczik, 2013; Schöllhorn et al., 2015; Hossner et al., 2016a; Schöllhorn, 2016). Experimental designs and theoretical rationales of DL have been put forward and discussed but require further examination (Schöllhorn et al., 2009a, 2010a; Schöllhorn and Horst, 2020). The most recent review (Schöllhorn and Horst, 2020) explains DL's enhanced learning rate by an overloading mechanism of the pre-frontal cortex with too many decisions regarding movement execution, which would subsequently enlarge the working memory of the motor control system. There is evidence based on EEG data that suggests DL to cause different brain processes immediately after a training session (Henz and Schöllhorn, 2016; Henz et al., 2018), but in isolation, these data cannot confirm the underlying neural mechanisms of DL and reveal the need for further research.
Regardless of the underlying neural mechanism at play, DL has been experimentally tested in various settings with a large range in the rates of success. The initial experiments were mainly oriented toward performance in a single movement in a sport context (Schöllhorn et al., 2004; Beckmann and Schöllhorn, 2006) or laboratory tasks (James, 2014; James and Conatser, 2014), but recently, it has been adopted within more complex tactical sport contexts (Mateus et al., 2015; Coutinho et al., 2018; Santos et al., 2018), clinical settings (Repšaite et al., 2015; Kurz et al., 2016; Benjaminse et al., 2017; Pabel et al., 2017, 2018; Gokeler et al., 2019), and industrial production processes (Weisner et al., 2019). Collectively, these findings hold valuable information which could support trainers in developing tailored athletic training programs and working toward maximal performance, and could aid clinicians working in injury prevention and rehabilitation.
Despite DL being proposed over 20 years ago, no comprehensive overview with additional analyses currently exists comparing the learning rate of DL with the learning rate of various other motor learning methods. Providing such an overview with analyses could help trainers and clinicians to make better-informed decisions concerning the choice of one or more particular motor learning method(s) in daily practice. However, to date, no systematic review and meta-analysis exists that examines the effectiveness of DL compared to traditional or other variability-based motor learning methods on the performance enhancement of skill (sport context: e.g., dribbling, shooting) or movement tasks (laboratory setting: e.g., unilateral arm rotations) in both the acquisition and retention phase. Therefore, the objective of this meta-analytical review is to examine the evidence from (cluster-)randomized experiments (S) that compared the learning rate of DL (I) to other motor learning methods (C: REP, MSE, VP, CtIt, CLA, and SL) in the performance of movement tasks or skills (O) in humans (P) (PICOS: Population, Intervention, Control, Outcome, Studies). Based on the dynamical systems model of DL by Frank et al. (2008) and the review of Lage et al. (2015), we hypothesized that the learning effectivity of DL would be larger in the retention phase than in the acquisition phase. Besides a systematic summary of the evidence, this meta-analytic review can also be used to explore whether the current empirical evidence supports the claim of DL being an enhanced learning method, to identify gaps in the current state of the art, and to stress various research methodological aspects that require improvement in future research.
Methodology
The Preferred Reporting Items for Systematic Reviews and Meta-Analyses (PRISMA) statement was followed for the development of the abovementioned research question and review protocol (Moher et al., 2015; Shamseer et al., 2015). The scope of the PICOS question was very broad and consequently stresses the fact that the meta-analysis is rather exploratory in nature. Patterns in the dispersion of results of different studies are as much of interest as the overall mean effects (Borenstein et al., 2009).
Information Sources
PubMed (MEDLINE), Web of Science, and Google Scholar were searched for relevant articles.
Eligibility Criteria
The a priori set inclusion criteria were as follows: (1) studies had to be (cluster-)randomized controlled experiments comparing DL to a different motor learning method; (2) the use of co-interventions (e.g., physical literacy and strength training) in both groups was allowed since they represent general practice in non-laboratory contexts and are in line with representative learning design directives to ensure functionality and action fidelity in training and learning environments (Pinder et al., 2011); (3) studies had to describe the effects on one or more measures of performance in a movement task; (4) the study report had to be published as a full paper in a journal or as a book chapter to be able to make a reliable risk-of-bias assessment. Exclusion criteria encompassed the following: (1) lack of practice for the control group; (2) the use of non-performance outcomes (e.g., movement patterns), as it is unclear what changes constitute improvement or deterioration, and would be in contradiction with the DL assumptions. In addition, no specific criteria were specified for the population. No restrictions were applied to language or year of publication. DL was defined according to the definition in the Dictionary of Sport Psychology (2019) (Hackfort et al., 2019).
Search Process
The search strategy was developed by two authors (BS and BT). The following search string was used in PubMed: [((differential-learning) OR differential-training) OR differencial-learning] OR differencial-training[all]. The last search was carried out on February 3, 2020. To ensure a sensitive search strategy, additional searches were done based on the reference lists of included articles and reviews, and on the ResearchGate profiles of authors of included articles.
Screening Procedure
All retrieved titles, abstracts, full texts, and citations were integrated in the Rayyan web application (https://rayyan.qcri.org) (Ouzzani et al., 2016). After removal of duplicates, titles and abstracts were screened, followed by an inspection of the full text. All full texts were independently screened by two authors (BS and BT). In case of disagreement on the eligibility of a study, a third researcher (JV) checked the variable in the original study and agreement was sought by consensus. The following information was extracted: first author, year of publication, study design, description of participants (number, age, gender, and other characteristics), description of the movement task and the performance variable, and description of the training intervention of the DL and other groups (context of the intervention, duration, frequency, number of exercises, number of repetitions, and description of the exercises).
Risk of Bias Assessment
The included studies were assessed using the Cochrane Risk of Bias Tool, analyzing eight sources of bias: selection, performance, detection, attrition, reporting, and other reasons of bias (Moher et al., 2010). This was done independently by two authors (BS and BT) and discrepancies were resolved through discussion. In case of disagreement, a third researcher (JV) was consulted and agreement was sought by consensus.
Calculation of Effect Sizes for Quantitative Synthesis
The effect size of choice was a standardized mean difference (Morris, 2008):
, where c represents a correction factor for small sample sizes (close to 1 for large samples), M are means, SDpre is the pooled standard deviation at the pre-test, and C is the control group (other motor learning method). This effect size represents a standardized difference in learning rate between the DL and control group. Learning rate was presented as the order parameter most relevant for DL (Frank et al., 2008). The same effect size was used for the retention test (retention – pre). When a study reported more than one retention test, the latest test was used in our analysis. Results on transfer tests to other than the target movement were not included because there were too few studies on transfer effects. In studies that provided no means and SEs or SDs, but the individual change scores (δ) were given, the effect size was calculated as . To estimate the standard error of d, we needed the pre–post correlation, but this was not included in any report. For the primary analysis, we took r = 0.50 as a reasonable mean estimate. Sensitivity analyses were performed with r = 0.15 and 0.85 to examine the influence of this parameter on the overall results of the meta-analysis. In case of a discrete outcome measure (e.g., fail or pass on an exam), the log odds ratio was calculated for the data presented in this study and then converted to a standardized mean difference with the formulas presented in Borenstein et al. (2009) (chapters 5 and 7). Similar procedures were applied for studies reporting log odds ratios. For studies that reported multiple outcome variables, we calculated the weighted average effect size. When a study did not report all outcomes, authors were contacted by email. When authors did not respond, but the article contained figures with enough information to calculate the effect size, a software program (GetData-Graph-Digitizer.com) was used to extract the raw study data. However, when authors did not respond and data could not be extracted via other means, the article was excluded from the final quantitative analysis. The interpretation of the effect sizes was done in accordance with Cohen's (1988) guidelines: “negligible,” d < 0.2; “small,” 0.2 < d < 0.5; “medium,” 0.2 < d < 0.8; “large,” d > 0.8 (Cohen, 1988).
Meta-Analyses
Separate meta-analyses for the effects of acquisition (pre-test vs. post-test) and learning (pre-test vs. retention test) were carried out. Subgroup analyses were performed based on the type of task (e.g., sport performance, technical skill) and type of contrasted learning method (e.g., DL vs. TL and DL vs. CtIt). Subgroups based on the type of task were defined by the following separation criteria: (1) “sport performance” encompassed outcomes focusing on the speed or strength component of the skill performed by the participant. For example, how far a participant could throw, how fast a participant sprinted in a straight line or around the track, how high a participant jumped, how hard a participant could kick a ball, etc. (i.e., shot put, high jump, hurdle racing, ice skating race, and countermovement jump); (2) “sport technical skills” focused more on the precision aspect of skills (e.g., shooting/passing/kicking/serving accuracy as measured by the error with respect to a target, reception of a pass as measured by the distance from the reception point, completion of a technical/agility circuit against time); (3) “sport tactical behavior (skills)” included outcomes assessed during match play (e.g., triple threat position/give-and-go/explore 1-on-1 game/field goals characterized as whether the behavior was successful or not; these variables were then normalized); (4) “fine motor skills”: healthy participants had to carry subtle or refined movement tasks or skills outside the sport context (i.e., toothbrushing, dental surgery, handle rotation, and standing as still as possible); (5) “rehabilitation”: injured or post-operative participants (this category was left out of the meta-analysis, since the two studies could not be included in the quantitative analyses). All meta-analyses were carried out in Review Manager 5.3 (Cochrane Collaboration). Studies that used different subgroups (e.g., based on age) were entered separately in the meta-analysis. Random effects models were used throughout as between-study variation was expected based on the heterogeneity of movement tasks, subject characteristics, study designs, and performance variables (Borenstein et al., 2009). The inverse of the variance was used to weigh each study result on the overall mean and 95% CI. For the interpretation of heterogeneity, Higgins' I2 values were calculated (Higgins et al., 2003). Publication bias was visually inspected with a funnel plot. Supplementary material may be found online at https://osf.io/m4sje/.
Results
Qualitative Synthesis
The flowchart in Figure 1 shows the results of the search and screening process, as well as the numbers of articles included. For the qualitative synthesis, there are 27 original studies included that contain 31 original experiments. For the quantitative synthesis (acquisition phase), there are 23 original studies included that contain 27 original experiments. For the quantitative synthesis (learning phase), there are 12 original studies included that contain 12 original experiments. The features of the included articles are described in Table 1.
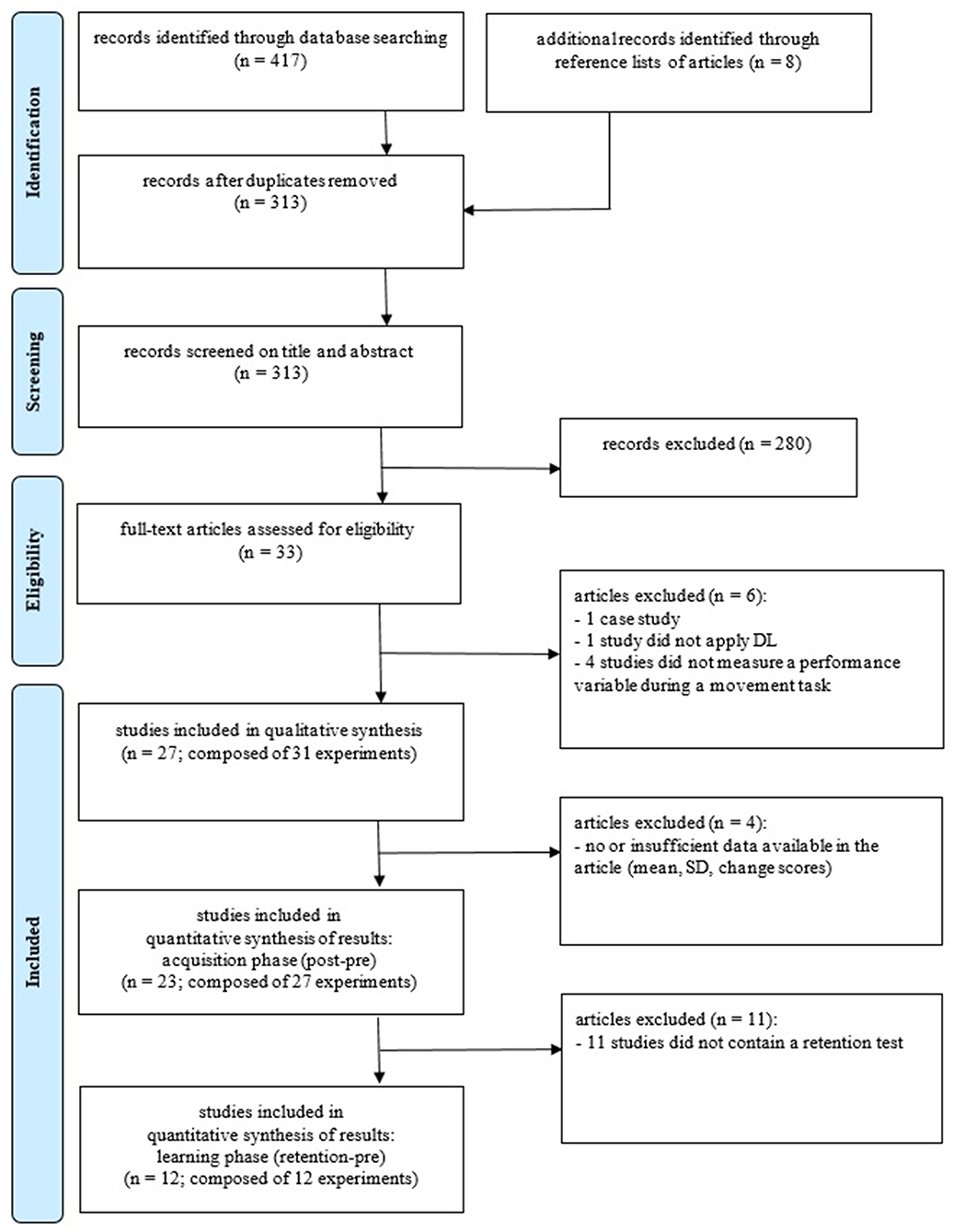
Figure 1. Flowchart of the search and screening process (based on the PRISMA statement template). DL, differential learning; TL, traditional learning; CtIt, contextual interference; SL, structural learning (Moher et al., 2015).
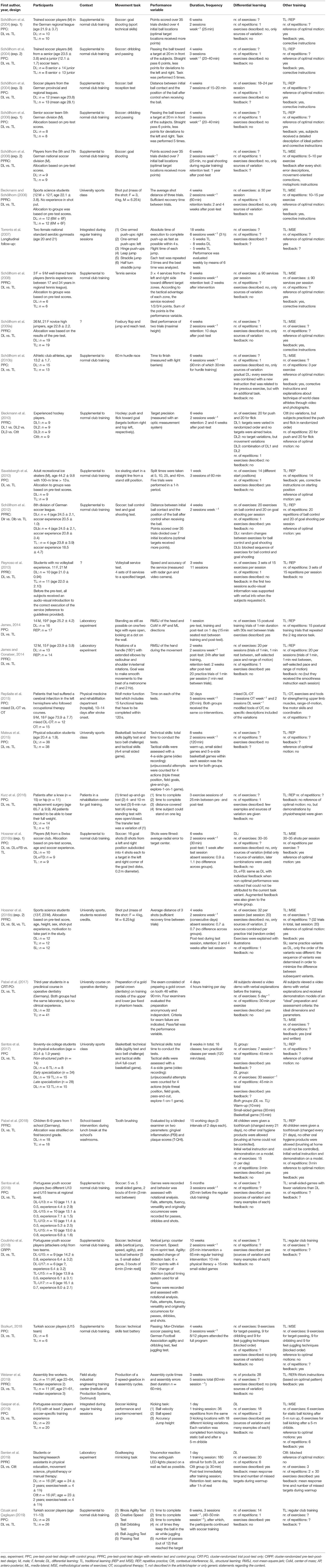
Table 1. Design, participants, movement tasks, performance variables, and training interventions of studies included in the qualitative synthesis.
Twenty-seven articles met the inclusion criteria, resulting in 31 experiments providing data on 897 participants (DL group: n = 453; control group: n = 446). DL has been used in a variety of contexts: (1) sport performance outcomes (i.e., shot put, high jump, hurdle racing, ice skating race, and countermovement jump); (2) technical skills in a single sports movement (i.e., service in volleyball/tennis; soccer: passing, shooting accuracy, and ball control; hockey: goal shooting precision); (3) tactical skills in a sport context (i.e., during match play in basketball or soccer); (4) fine motor skills (toothbrushing, dental surgery, handle rotation, and balance); and (5) rehabilitation (Repšaite et al., 2015; Kurz et al., 2016). Mateus et al. (2015), Santos et al. (2017), and Coutinho et al. (2018) assessed the effects of DL on both technical and tactical skills. The majority of studies examined the effects of DL directly after the intervention (acquisition effect), while only 12/27 experiments included a retention test (learning effect). When available in the manuscript, Table 1 summarizes the timing of post- and retention tests and delays between them. Most post-tests were organized on the same day or within 24 h of the last training session whereas some post-tests were organized a week after the last training session. The time between post-test and retention test varied between 1 h and 1 year (most studies between 1 and 2 weeks).
Risk of Bias Analysis
Table 2 gives an overview of the risk of bias of each study (experiment). Concerning randomization, 15/31 experiments had a low risk of bias and the other were unclear, whereas two studies used cluster randomization (high risk). Allocation concealment was unclear in all but four experiments with high risk of bias and two with low risk of bias. Given the nature of the experiments, blinding of participants and personnel was not possible. Outcome assessment was blinded in 7/31 experiments and unclear otherwise (blinded researcher or computerized registrations). Incomplete outcome data were high risk or unclear in 8/31 experiments, the rest had low risk. Selective outcome reporting was high risk of bias in 9/31 experiments (reported no means, standard deviations, and/or statistics and did not respond to emails for further inquiry). Other reasons of bias were an incomplete description of the training/control intervention and outcome variables that are susceptible to subjective interpretation. With exception for the studies from the groups of Savelsbergh, James, Hossner, Pabel, and Serrien, risk of bias was overall high for all studies (fewer than 4/7 items with low risk of bias).
Quantitative Synthesis of Results
To compare the effects of DL vs. other motor learning methods, effect sizes were extracted from the original research papers and grouped according to relevant context and outcomes. All data on individual effect sizes, 95% CI, overall estimated effect sizes, and heterogeneity are presented in Figure 2 (acquisition phase) and Figure 3 (learning phase). Given the relatively low number of experiments and heterogeneity between them, no further selection on quality was done and all experiments that provided data were used in the meta-analysis.
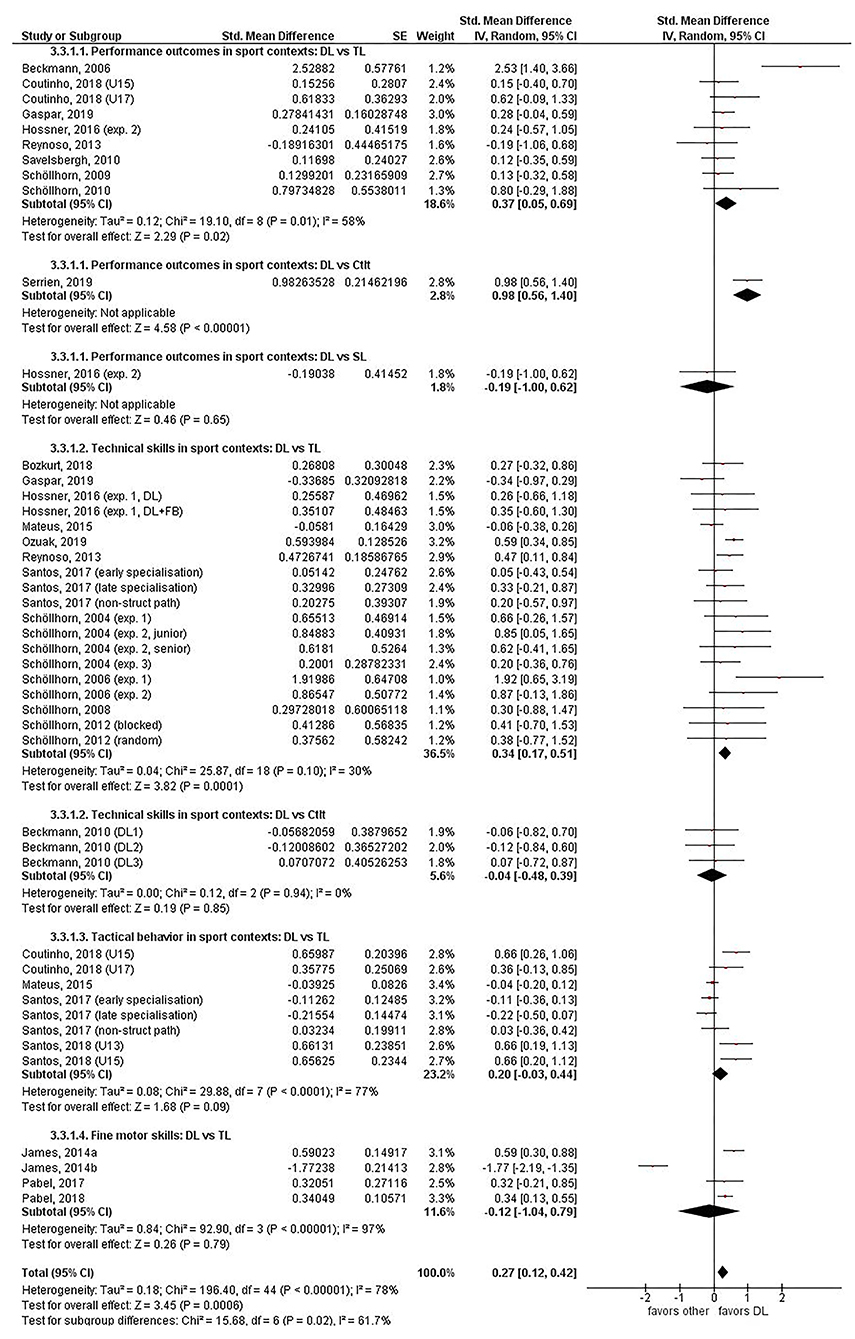
Figure 2. Acquisition phase (post – pre). Forest plot for the effects of differential learning vs. other methods grouped by category of movement task. DL, differential learning; TL, traditional learning; CtIt, contextual interference; SL, structural learning.
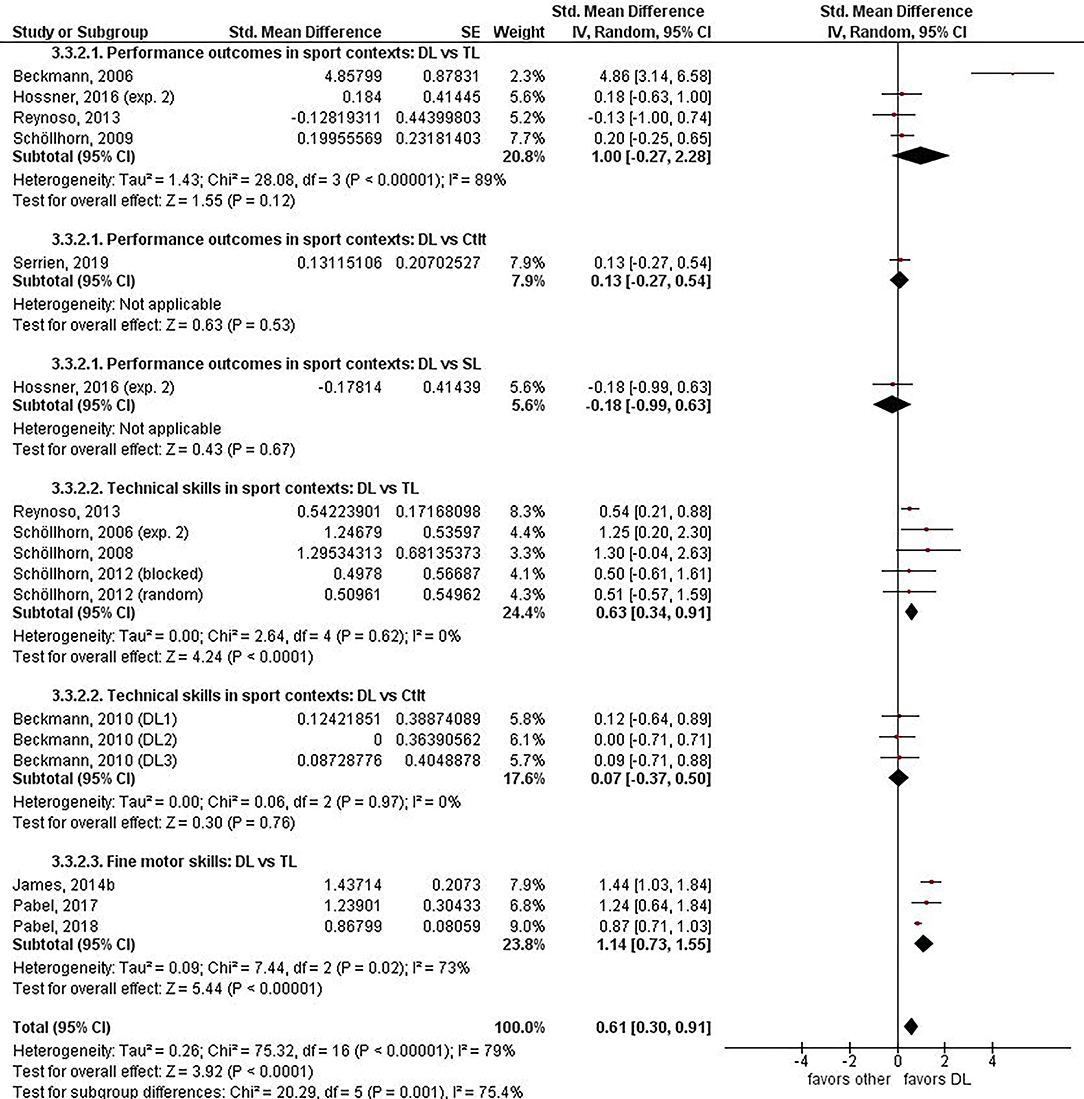
Figure 3. Learning phase (retention – pre). Forest plot for the effects of differential learning vs. other methods grouped by category of movement task. DL, differential learning; TL, traditional learning; CtIt, contextual interference; SL, structural learning.
Acquisition Phase (Post – Pre, in Accordance With q30)
The forest plot of the acquisition phase can be found in Figure 2. Twenty-seven experiments reported the effects of DL in the acquisition phase compared to other motor learning methods (Schöllhorn et al., 2004, 2006, 2008, 2009b, 2010b; Beckmann and Schöllhorn, 2006; Beckmann et al., 2010; Savelsbergh et al., 2010; Reynoso et al., 2013; James, 2014; James and Conatser, 2014; Mateus et al., 2015; Hossner et al., 2016b; Pabel et al., 2017, 2018; Santos et al., 2017, 2018; Bozkurt, 2018; Coutinho et al., 2018; Gaspar et al., 2019; Ozuak and Çaglayan, 2019; Serrien et al., 2019). The overall effect was small and in favor of DL (d = 0.27, 95% CI = [0.12–0.42], p = 0.0006), and the test for overall subgroup differences was statistically significant (χ2 = 15.7, p = 0.02, I2 = 61.7%), indicating different effects of DL among the several subgroup analyses.
Performance Outcomes in Sport Contexts
Nine experiments were included in this subgroup analysis (Beckmann and Schöllhorn, 2006; Schöllhorn et al., 2009b, 2010b; Savelsbergh et al., 2010; Reynoso et al., 2013; Hossner et al., 2016b; Coutinho et al., 2018; Gaspar et al., 2019; Serrien et al., 2019). Participants in the DL group showed greater improvements from pre- to post-test than those in the TL group in seven of the eight experiments with a relatively small overall effect size (d = 0.37, 95% CI = [0.05–0.69], I2 = 58%). The study of Beckmann and Schöllhorn (2006) was considered an outlier across the entire meta-analysis. Only one study compared performance outcomes after SL to DL, with participants in the DL group showing less improvement than participants in the SL group (d = −0.19, 95% CI = [−1.00, 0.62]) (Hossner et al., 2016b). Also, one single study compared performance outcomes after CtIt to DL, with participants exposed to DL showing greater improvement than the CtIt group (d = 0.98, 95% CI = [0.56–1.40]) (Serrien et al., 2019).
Technical Skills in Sport Contexts
Fourteen experiments documented the effects of DL compared to TL (Schöllhorn et al., 2004, 2006, 2008, 2012; Reynoso et al., 2013; Mateus et al., 2015; Hossner et al., 2016b; Santos et al., 2017; Bozkurt, 2018; Coutinho et al., 2018; Gaspar et al., 2019; Ozuak and Çaglayan, 2019). Participants in the DL group showed on average greater improvements from pre- to post-test than participants exposed to TL in 12 out of the 14 experiments. The overall effect size and 95% CI was positive but small (d = 0.34, 95% CI = [0.17–0.51], I2 = 30%). Subgroup analysis on one study evaluating the effects of DL compared to CtIt revealed a negligible negative effect size (d = −0.04, 95% CI = [−0.48, 0.39]) (Beckmann et al., 2010).
Tactical Behavior in Sport Contexts
Four experiments were included in this subgroup analysis, showing a small positive overall effect size (d = 0.20, 95% CI = [−0.03, 0.44], I2 = 77%) with the DL group showing on average greater improvements from pre- to post-test in two of the four experiments (Mateus et al., 2015; Santos et al., 2017, 2018; Coutinho et al., 2018).
Fine Motor Skills
This subgroup analysis encompassed four experiments evaluating the effects of DL compared to TL (James, 2014; James and Conatser, 2014; Pabel et al., 2017, 2018). On average, participants in the DL group showed greater improvements from pre- to post-test than those in the TL group in three of the four experiments, but the overall effect size was negative but negligible (d = −0.12, 95% CI = [−1.04, 0.79]; I2 = 97%).
Learning Phase (Retention – Pre, in Accordance With q30)
The forest plot of the acquisition phase can be found in Figure 3. Twelve experiments reported the effects of DL in the retention phase compared to other motor learning methods (Beckmann and Schöllhorn, 2006; Schöllhorn et al., 2006, 2008, 2009b, 2012; Beckmann et al., 2010; Reynoso et al., 2013; James and Conatser, 2014; Hossner et al., 2016b; Pabel et al., 2017, 2018; Serrien et al., 2019). Not one experiment or outcome encompassed tactical behavior. The overall effect size was moderate in strength and in favor of DL (d = 0.61, 95% CI = [0.30–0.91], p < 0.0001) and the test for overall subgroup differences was statistically significant at the 5% level (χ2 = 20.29, p = 0.001, I2 = 75%) indicating different effects of DL among the several subgroup analyses.
Performance Outcomes in Sport Contexts
Six experiments were included in total, with four of them looking into DL-TL comparisons, only one experiment examining DL-CtIt, and one other researching DL-SL (Beckmann and Schöllhorn, 2006; Schöllhorn et al., 2009b; Reynoso et al., 2013; Hossner et al., 2016b; Serrien et al., 2019). Participants in the DL group demonstrated on average greater improvements from pre- to retention test than participants in the TL group in three of the four experiments with an overall large positive effect size (d = 1.00, 95% CI = [−0.27, 2.28], I2 = 89%) (Beckmann and Schöllhorn, 2006; Schöllhorn et al., 2009b; Reynoso et al., 2013; Hossner et al., 2016b). Only one study compared performance outcomes of DL to SL, with participants in the DL group showing on average less improvement with a negligible negative effect size (d = −0.18, 95% CI = [−0.99, 0.63]) (Hossner et al., 2016b). Also, one study compared performance outcomes after CtIt to DL, with the DL group showing negligible more improvement from pre- to retention test compared to CtIt (d = 0.13, 95% CI = [−0.27, 0.54]) (Serrien et al., 2019).
Technical Skills in Sport Contexts
Subgroup analysis on four experiments evaluating the effects of DL compared to TL showed on average stronger improvements from pre- to retention tests for the DL group (d = 0.63, 95% CI = [0.34–0.91]) (Schöllhorn et al., 2006, 2008, 2012; Reynoso et al., 2013). When comparing DL to CtIt for technical skills, only one study could be included, and a negligible effect of DL compared to CtIt was observed (d = 0.07, 95% CI = [−0.37, 0.50], I2 = 0%) (Beckmann et al., 2010).
Fine Motor Skills
Three experiments were included in this subgroup analysis and all studies showed superior improvements from pre- to retention test for DL compared to TL with large effect sizes (overall effect: d = 1.14, 95% CI = [0.73–1.55]) (James and Conatser, 2014; Pabel et al., 2017, 2018).
Sensitivity Analyses
Table 3 presents the results of the sensitivity analyses on the calculation of the effect size variances, using various levels of the pre–post correlation. The results are fairly robust under a wide range of plausible correlation coefficients.
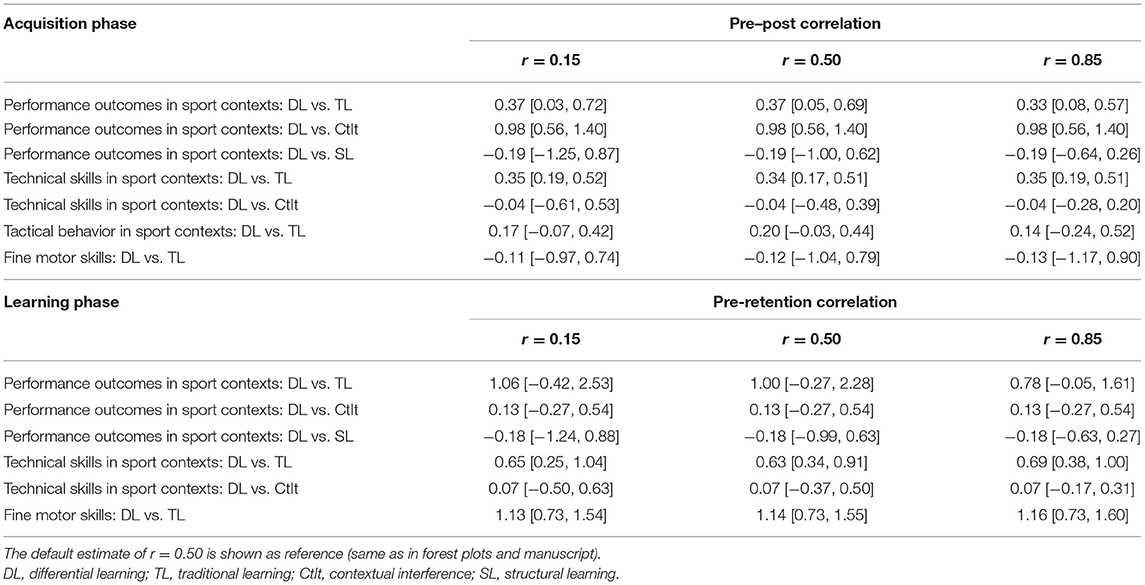
Table 3. Sensitivity analysis of the effect sizes [95% CI] based on various levels of the pre–post correlation coefficient.
Publication Bias
Figure 4 presents the funnel plot of all included studies. Visually, a moderate asymmetry toward the right is present in both funnel plots, but this is primarily due to the presence of strong outliers in both directions (Beckmann and Schöllhorn, 2006; Schöllhorn et al., 2006; James and Conatser, 2014). However, not every study could be included in the meta-analysis, which biases the interpretation of the funnel plots. In addition, the presence of many unpublished abstracts (e.g., https://sport.uni-mainz.de/publikationsliste/) indicates that publication bias is present and affected the results of the meta-analysis.
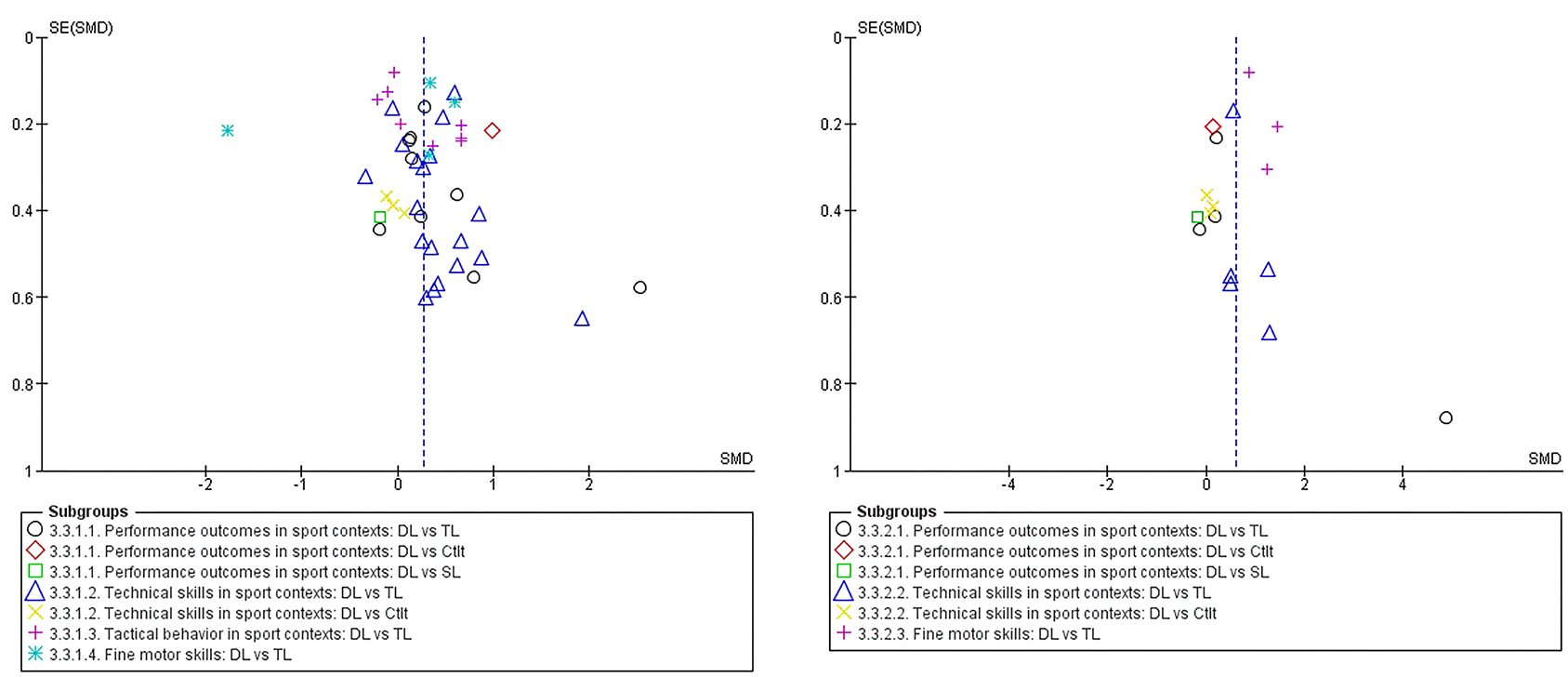
Figure 4. Funnel plots of the effect sizes of the acquisition phase (left) and learning phase (right). Vertical dashed line shows the overall effect size. DL, differential learning; TL, traditional learning; CtIt, contextual interference; SL, structural learning.
Discussion
The objective of this meta-analytical review was to examine the evidence of studies that compared the effectiveness of DL to other motor learning methods in the performance of skills and movement tasks. We included 27 articles reporting outcomes of 31 experiments, with only 12 experiments documenting outcome measures in the retention phase. In the acquisition phase, DL is more effective compared to other motor learning methods with an overall small effect size of 0.27 [0.12, 0.42]. In the retention phase, however, DL appears on average to be more effective than other motor learning methods with an overall effect size of 0.61 [0.30, 0.91]. At first sight, one might be tempted to conclude that variability-based motor learning, DL in this case, culminates in higher improvements following practice than other motor learning methods (Frank et al., 2008; Lage et al., 2015; Schöllhorn and Horst, 2020). Nevertheless, it is important to emphasize that overall heterogeneity for the acquisition phase as well as for the retention phase was large, I2 = 78% and I2 = 79%, respectively. Also, the included papers in general had low sample sizes and showed high risk of bias and possible publication bias. The funnel plot (Figure 4) indicates that overall effect sizes should be carefully interpreted and warrants more high-quality research.
Critical Interpretation on the Effects of DL in the Acquisition Phase
Bearing in mind that overall large heterogeneity (p < 0.00001, I2 = 78%) was found across the included studies, interpreting the results regarding improvements following practice of DL compared to other motor learning methods in the acquisition phase should be made with considerable care. At the subgroup level, concerning performance outcomes in sport contexts, DL showed higher improvements following practice than TL with a relatively small overall effect size. However, it is more than likely that the true effect size is lower, since the study of Beckmann and Schöllhorn (2006) had a strong influence on this subgroup's effect size. Heterogeneity between effects was large (I2 = 58%), indicating the presence of unexplained factors, such as the type of performance outcome (e.g., ice skating speed vs. throwing distance). Furthermore, the included studies did not show unanimous positive results, while the CIs for all studies, except the study of Beckmann and Schöllhorn (2006), crossed the line of null effect. Remarkably, the study of Hossner et al. (2016b, exp. 2) used a similar sample (size), similar context, duration, frequency, amount of exercises, and task as the study of Beckmann and Schöllhorn (2006) but the effect size was 10.5 times larger in the latter study than the former. Differences in the application of feedback and demonstrations probably contributed to these vastly different outcomes, although this alone might not sufficiently explain the big difference in effect sizes between these two studies. Moving on to another subgroup, DL might enable slightly higher improvements following practice in tactical behavior in sports. Nevertheless, also in this case large heterogeneity was present across the included experiments of this subgroup (I2 = 77%). This can be partially explained by differences in population (e.g., experience level, age) and used outcome measures (e.g., basketball vs. soccer). Another possible factor contributing to this high level of heterogeneity could have been the subjective nature and interpretation of some tactical variables (e.g., creative components). Although these studies were the first to research tactical outcome measures and play an important role in the development of motor learning research by providing insights in this previously unexplored area, more objective tactical outcome measures should be included in future research. Regarding fine motor skills, DL performed on average better than TL. Yet, the overall effect size was negative and the CI covered zero (d = −0.12, [−1.04, 0.79]) largely due to a strong negative outlier causing large heterogeneity (I2 = 97%). The “technical sport skills (DL vs. TL)” was the only subgroup with a relative low amount of heterogeneity (I2 = 30%). Here, a small positive effect was found for DL compared to TL. These results should nonetheless be interpreted with caution, as not all included studies demonstrated effects favoring the DL method; the CIs of the majority of studies crossed the line of null effect, and most of the experiments were carried out by the same research group. The results of three subgroups [DL vs. CtIt (sport performance outcomes), DL vs. CtIt (sport technical skill), and DL vs. SL (sport performance outcomes)] should not be interpreted separately, since an insufficient number of experiments (1) and participants were included in each subgroup.
In summary, the test for overall effect shows a statistically significant difference favoring DL over other motor learning methods in the acquisition phase (p = 0.0006). Nevertheless, as already stated above, to interpret this total summary, statistical results would be premature in light of the considerable amount of heterogeneity. Given that this information is less meaningful, it is recommended to devote more attention to the subgroup analyses. Three out of seven subgroups had very large variances due to low sample sizes, while three other subgroups only encompassed one study, which limits generalizability of the results. Therefore, the validity of the improvements following practice estimate for each subgroup is uncertain, as individual trial results are inconsistent. Despite the circumstantial and low-quality evidence, it seems that the acquisition could be slightly enhanced when applying DL in comparison to TL. When comparing DL to other variability-based motor learning methods (i.e., SL and CtIt), not one motor learning method currently appears to be superior for acquisition. Although it might be too early to assert these general statements, the discrepancy in results and the large heterogeneity proclaim the need for further high-quality research on this topic by independent research groups and clear demarcation of both the DL method and other motor learning methods.
Critical Interpretation on the Effects of DL in the Retention Phase
Given that the overall heterogeneity was large across the included studies in the retention phase (p < 0.00001, I2 = 79%) and the amount of included experiments was low (n = 12), interpreting the results regarding improvements following practice of DL compared to other motor learning methods in the retention phase should be made with great caution if they are to be made at all. Comparable to the acquisition phase, similar disconcerting patterns emerge regarding heterogeneity, low sample sizes, low power, etc. Even though fewer studies could be included during the retention phase, averaged across all subgroup comparisons, the effect of DL was two to three times larger in the retention phase (d = 0.61, [0.30, 0.91]) compared to the acquisition phase (d = 0.26 [0.10, 0.42]). Nevertheless, readers should critically interpret and reflect on these effect sizes. Similar to the acquisition effect for shot put training, both studies of Beckmann and Schöllhorn (2006) and Hossner et al. (2016b) found a better learning effect for DL compared to TL, but a very large discrepancy was observed for the effect sizes. Despite their similar designs, the study of Beckmann and Schöllhorn (2006) demonstrated a 27 times larger effect size than the study of Hossner et al. (2016b). Mainly fine motor skills and sports technical skills seem to be better retained after DL intervention in comparison to TL. Although sensible interpretations should be made on these two topics. The sport technical skills subgroup mainly encompassed studies from one research group with the CIs of some studies exceeding the line of null effect, while the fine motor skills subgroup encompassed a large amount of heterogeneity (I2 = 73%). Furthermore, three out of seven subgroups (all DL vs. other variability-based motor learning methods) could only include one study, which implicates very low generalizability and minimal attributable value to potential inferences based on these results. Nevertheless, the result of the overall effect shows a statistically significant difference favoring DL over other motor learning methods (p < 0.0001). However, general interpretations about the effectiveness of DL compared to other motor learning methods in the retention phase should be made with great caution. This is due to the large amount of heterogeneity, the limited number of studies, low sample sizes, and considerable risk of bias across all studies.
Does the Current Empirical Evidence on DL Support Its Theoretical Rationale and the Variability-Based Continuum?
The findings of the meta-analysis are partly in line with the theoretical rationale of DL that strives to achieve an individual optimal level of variability in practice, allowing the athlete to discover different aspects of his/her dynamic movement landscape and withhold the most efficient and effective movement solution as part of the motor learning process. Recently, the DL method received a high degree of attention in research and practice, partly due to its hypothesis of potentially being an enhanced motor learning method (= provides the learner with a higher learning rate than other methods), partly due to researchers' critical attitude toward the DL method (Pabel et al., 2017, 2018; Bozkurt, 2018; Coutinho et al., 2018; Santos et al., 2018; Gokeler et al., 2019; Serrien et al., 2019; Weisner et al., 2019).
The differences of DL with other methods that employ practice variability are the amount and/or structure of the exercise variations. Schöllhorn et al. (2009a) depicted various motor learning methods in a continuum of increasing variability and noise (REP, MSE, VP, CtIt, CLA, SL, and DL) with DL being hypothesized to exemplify the highest learning rates (Schöllhorn et al., 2009a; Schöllhorn and Horst, 2020). However, the results of the current meta-analysis question the validity of this continuum. For a robust comparison of DL to other motor learning methods inspired by variability (VP, CtIt, CLA, SL), scarce and inconclusive evidence exists to examine and infer whether DL is superior or inferior in terms of learning rate. Additionally, we want to draw attention to the difficulty in distinguishing between DL and SL (Hossner et al., 2016b; Schöllhorn, 2016). Both methods use a large overall practice variability, but SL tries to minimize trial-to-trial variability (subsequent exercises are different in only a small detail). This led to the terminology of “gradual DL” as synonym for SL and “chaotic DL” for the classical interpretation that uses random trial-to-trial variability (Henz et al., 2018; Schöllhorn and Horst, 2020).
Based on the meta-analyses and in light of the low methodological quality of the included studies, DL shows potential to be considered as an enhanced motor learning method in comparison to TL methods when aiming to improve motor learning during the acquisition and retention phase. For both the acquisition and retention effect, the study with the lowest risk of bias (Pabel et al., 2018) was in line with the subgroup and omnibus effect size estimate.
Furthermore, the theory and mechanism behind the DL method is not undebated (Schoner, 1995; Scholz and Schöner, 1999; Latash et al., 2007; Beek, 2011; Künzell and Hossner, 2012, 2013; Schmidt and Hennig, 2012; Willimczik, 2013; Schöllhorn et al., 2015; Hossner et al., 2016a; Schöllhorn, 2016). Nevertheless, a detailed discussion on the theoretical background, key features, underlying (supposed) mechanisms, predictions, and limitations of DL in comparison to other motor learning methods is beyond the exploratory and practical focus of this systematic review and meta-analysis. Readers should thus also be aware of the following key points when interpreting the results of this study: (1) some fundamental limitations exist with the theoretical framework of DL, (2) DL studies are mostly focused on learning effectiveness rather than learning rate and that the effectiveness is assessed imperfectly when a pre- to post-test design is used rather than a design that also includes a retention/transfer test, (3) there are alternative methods available that predict benefits of VP but for different reasons than DL (e.g., schema theory, uncontrolled manifold hypothesis), and (4) CtIt and SL can be used to schedule VP.
How Can These Results Impact Motor Learning in Sport or Rehabilitation Contexts?
Trainers and clinicians often merge different theoretical motor learning concepts with the aim to improve athletes' or patients' motor or movement skill performance. The results of this meta-analysis do not allow for strong recommendations in favor of a specific motor learning method toward trainers or clinicians. However, a well-considered use of (increasing) variability appears to be beneficial over more traditional or repetitive motor learning methods. Farrow and Robertson (2017) discuss the role of variability-based learning within a skill acquisition periodization framework. They stress the role of variability in countering tedium, but refrain from giving general guidelines on where in the periodization of micro-, meso-, and macrocycles this is most optimal as the literature is not able to substantiate evidence-based criteria. In line with the model of Schöllhorn and Horst (2020), Farrow and Robertson (2017) propose a practical continuum of variability that can be offered to athletes, trainers, clinicians, and researchers.
Important in real-world training situations, whether it be performance or clinically oriented, is to shift focus toward individuality and specificity. Other important variables such as instruction, feedback, focus of attention, motivation, etc should also be considered besides the amount and structure of provided variability since these variables have also been shown to play an important role in motor learning in sport and rehabilitation contexts (Wulf and Lewthwaite, 2016; Gokeler et al., 2019). In a sport context, the integration of variability in motor learning possibly promotes motivation by increasing the challenge of training (Guadagnoli and Lee, 2004) as well as promoting fun and enhanced expectancies during practice (Wulf and Lewthwaite, 2016). In a clinical context, focusing on the current capacity, the individual needs and goals of the patient are essential in order to select the most fitting motor learning method. Implementing insights from DL (together with other variability-based motor learning methods) and a well-considered use of variability can improve task performance on the short term allowing for enhanced motor learning during the acquisition phase, while fine motor skills likely benefit the most from the retention effect of DL (Pabel et al., 2017, 2018). Restoring gross and fine motor skills are an important aspect of neurological and musculoskeletal rehabilitation given the known persistence of sensorimotor impairments (Repšaite et al., 2015; Gokeler et al., 2019). Increasing variability in rehabilitation should always be performed in a safe context, allowing for successful but challenging exercises to allow the patient to explore efficient and effective movement strategies that transfer to real-world scenarios (Guadagnoli and Lee, 2004). Nevertheless, data on the application of DL during a rehabilitation process after injury or in a sport injury risk mitigation plan is scarce to non-existent.
In training/rehabilitation contexts, the learning of a single movement is rarely the goal. Regarding transfer effects, many experiments that were included assessed the effects of DL on more than one movement (Schöllhorn et al., 2012) or included several different outcome variables of the same movement (Reynoso et al., 2013) or outcome variables from different movements (Mateus et al., 2015; Santos et al., 2017, 2018). Studies that explicitly used a transfer test (e.g., Beckmann et al., 2010) were scarce and not included in any meta-analysis. DL uses variability with the aim to prepare subjects to be able to cope with a large range of unforeseen situations (Schöllhorn et al., 2010a); therefore, we recommend future studies to address transfer effects to unforeseen situations or to related movements.
Limitations
Publication bias and missing data for the meta-analysis may have influenced the results. The meta-analysis was based on a very heterogeneous sample of studies with widely varying populations, motor tasks, and control conditions. These high levels of heterogeneity stress the importance to interpret these results with caution and call for high-quality future research. For the acquisition phase, the subgroups based on type of task and type of control condition were a significant factor in explaining the heterogeneity. However, only one study compared DL to SL (Hossner et al., 2016b), while one study compared it to CtIt, and all others compared it to TL. Future analyses may consider further subgroups for REP and MSE comparisons. Regarding heterogeneity in sample characteristics, future analyses must consider additional subgroup analyses based on age and/or level of expertise as we grouped results from complete novices and experts in the same analysis. Also, dividing the meta-analysis into different subgroups based on the type of task (e.g., performance, technical skill) might not be ideal for a holistic interpretation on this topic, though an overall effect size was calculated for both the overall acquisition and retention phase. From a theoretical perspective, the most important covariate to be considered in future meta-analyses is likely the noise level of the training intervention. A difficulty here will be to find a proper common metric that quantifies this outcome.
Besides co-interventions representing general practice in non-laboratory contexts and being in line with representative learning design directives to ensure functionality and action fidelity in training and learning environments (Pinder et al., 2011), the inclusion of experiments with co-interventions (Mateus et al., 2015; Repšaite et al., 2015; Santos et al., 2017) might also be a potential confounder of the results. However, as noted earlier, in practical contexts, several methods are often combined, so these experiments can provide important information. Furthermore, studies without assessment of performance variables (Menayo et al., 2014; Henz and Schöllhorn, 2016; Henz et al., 2018) were not included in this meta-analysis although they provide valuable information on specific aspects of DL. These studies are especially important for inquiry about the individuality and situation specificity of the stochastic resonance.
A final limitation is the unknown pre–post and pre-retention correlations in the study reports. The sensitivity analysis showed that this parameter had only a small influence on the overall effect sizes and their 95% CI, but this assumed a fixed correlation coefficient across all studies and may potentially have a larger influence. The overview of effect sizes and their 95% CI may be used in the design of future interventions.
Implications for Future Research
In general, further high-quality research is necessary with low risk of bias RCTs and publications in peer-reviewed journals (Beek, 2011). Given the nature of motor learning experiments, it is challenging and, in many cases, impossible to blind participants, researchers, trainers, and therapists to which condition they are assigned to. Therefore, future studies should make a bigger effort in addressing the other risk of bias items in their study design and report them accordingly. Also a major recommendation for future research is to better define, design, and report the used control conditions in the study of DL. When motor learning refers to the study of cognitive, perceptual, motor, and physiological responses that explain motor skill acquisition, more attention should be devoted to the retention effects of motor learning interventions both in the short term and in the long term. Future research should also aim to encompass more robust designs, increase sample sizes, and clearly define the motor learning method that is experimentally tested as well as the motor learning method used to compare with, and to be published in international peer-reviewed journals. In particular, studies researching the differences between variability-based methods (DL, SL, CtIt, VP, and CLA) at the theoretical and the practical level are much needed. Potential interesting variables to address in future research could be the amount and structure of applied variability. Besides variability, other variables like instruction, feedback, focus of attention, motivation, level of expertise, etc should also be considered. Given the focus on individuality in DL, it will be important to study the relationships between dose (variability) and response (learning rate), and to identify factors that predict optimal amounts in specific populations and situations (Caballero et al., 2017). Also, the problem on the role of variability in motor learning periodization requires further investigations (Farrow and Robertson, 2017). Single-subject analyses may prove valuable for these fundamental questions.
Conclusion
Given the large amount of heterogeneity, low availability of studies, low sample sizes, and considerable risk of bias across all studies, inferences about the effectiveness of DL should be made with prudence. Considering these methodological flaws, DL shows potential to be considered as an enhanced motor learning method in comparison to TL methods when aiming to improve motor learning in the acquisition and retention phase. A robust comparison and conclusion on the relative effectiveness of DL to other motor learning methods inspired by variability (i.e., SL and CtIt) would be premature, since scarce and inconclusive evidence was found. Future research should aim to perform more high-quality research. Once more high-quality research becomes available, the results of this meta-analysis should be updated in combination with stricter inclusion criteria concerning study design, risk of bias, and publication policy.
Data Availability Statement
Publicly available datasets were analyzed in this study. This data can be found here: https://osf.io/m4sje/.
Author Contributions
The conception and drafting of the work and the acquisition and the analysis of data were carried out by BT and BS. All authors made substantial contributions to the design of and interpretation of data for the work. All authors revised the manuscript critically for important intellectual content and provided approval for publication of the content. All authors agreed to be accountable for all aspects of the work in ensuring that questions related to the accuracy or integrity of any part of the work are appropriately investigated and resolved.
Conflict of Interest
The authors declare that the research was conducted in the absence of any commercial or financial relationships that could be construed as a potential conflict of interest.
Acknowledgments
We would like to thank all the authors that shared their original data with us for performing the meta-analysis. We would also like to thank the reviewers for helping to improve the quality of the manuscript.
Footnote
References
Beckmann, H., and Schöllhorn, W. I. (2006). Differenzielles Lernen im Kugelstoßen. Leistungssport 36, 44–50.
Beckmann, H., Winkel, C., and Schöllhorn, W. I. (2010). Optimal range of variation in hockey technique training. Int. J. Sport Psychol. 41, 5–10.
Beek, P. J. (2011). Nieuwe, praktisch relevante inzichten in techniektraining Motorisch leren: het belang van random variaties in de uitvoering (deel 5). Sportgericht 65, 30–35.
Benjaminse, A., Otten, B., Gokeler, A., Diercks, R. L., and Lemmink, K. (2017). Motor learning strategies in basketball players and its implications for ACL injury prevention: a randomized controlled trial. Knee Surg. Sports Traumatol. Arthrosc. 25, 2365–2376. doi: 10.1007/s00167-015-3727-0
Borenstein, M., Hedges, L. V., Higgins, J. P. T., and Rothstein, H. R. (2009). Introduction to Meta-Analysis. West Sussex: Wiley. doi: 10.1002/9780470743386
Bozkurt, S. (2018). The effects of differential learning and traditional learning trainings on technical development of football players. J. Educ. Train. Stud. 6, 25–29. doi: 10.11114/jets.v6i4a.3229
Braun, D. A., Mehring, C., and Wolpert, D. M. (2010). Structure learning in action. Behav. Brain Res. 206, 157–165. doi: 10.1016/j.bbr.2009.08.031
Caballero, C., Moreno, F. J., Reina, R., Roldan, A., Coves, A., and Barbado, D. (2017). The role of motor variability in motor control and learning depends on the nature of the task and the individual's capabilities. Eur. J. Human Movement 38, 12–26.
Coutinho, D., Santos, S., Goncalves, B., Travassos, B., Wong, D., Schoellhorn, W., et al. (2018). The effects of an enrichment training program for youth football attackers. PLoS ONE 13:e0199008. doi: 10.1371/journal.pone.0199008
Djatschkow, V. (1973). Die Vervollkommnung der Technik der Sportler (Perfection of athletes technique). Berlin: Sportverlag.
Farrow, D., and Robertson, S. (2017). Development of a Skill acquisition periodisation framework for high-performance sport. Sports Med. 47, 1043–1054. doi: 10.1007/s40279-016-0646-2
Frank, T. D., Michelbrink, M., Beckmann, H., and Schöllhorn, W. I. (2008). A quantitative dynamical systems approach to differential learning: self-organization principle and order parameter equations. Biol. Cybern 98, 19–31. doi: 10.1007/s00422-007-0193-x
Gaspar, A., Santos, S., Coutinho, D., Gonçalves, B., Sampaio, J., and Leite, N. (2019). Acute effects of differential learning on football kicking performance and in countermovement jump. PLoS ONE 14:e0224280. doi: 10.1371/journal.pone.0224280
Gentile, A. M. (1972). A working model of skill acquisition with application to teaching. Quest 17, 3–23. doi: 10.1080/00336297.1972.10519717
Gokeler, A., Neuhaus, D., Benjaminse, A., Grooms, D. R., and Baumeister, J. (2019). Principles of motor learning to support neuroplasticity after acl injury: implications for optimizing performance and reducing risk of second ACL Injury. Sports Med. 49, 853–865. doi: 10.1007/s40279-019-01058-0
Guadagnoli, M. A., and Lee, T. D. (2004). Challenge point: a framework for conceptualizing the effects of various practice conditions in motor learning. J. Mot. Behav. 36, 212–224. doi: 10.3200/JMBR.36.2.212-224
Hackfort, D., Schinke, R. J., and Strauss, B. (2019). “D,” in Dictionary of Sport Psychology, eds D. Hackfort, R. J. Schinke, and B. Strauss (Cambridge, MA: Academic Press), 69–85.
Henz, D., John, A., Merz, C., and Schöllhorn, W. I. (2018). Post-task effects on eeg brain activity differ for various differential learning and contextual interference protocols. Front. Hum. Neurosci 12:19. doi: 10.3389/fnhum.2018.00019
Henz, D., and Schöllhorn, W. I. (2016). Differential training facilitates early consolidation in motor learning. Front. Behav. Neurosci. 10:199. doi: 10.3389/fnbeh.2016.00199
Higgins, J. P. T., Thompson, S. G., Deeks, J. J., and Altman, D. G. (2003). Measuring inconsistency in meta-analyses. BMJ 327, 557–560. doi: 10.1136/bmj.327.7414.557
Hossner, E. J., Kach, B., and Enz, J. (2016a). On experimental designs, differencial learning, theoretical issues, dynamical systems, and the capability to adapt: response to Schollhorn. Hum. Mov. Sci. 47, 246–249. doi: 10.1016/j.humov.2015.11.019
Hossner, E. J., Kach, B., and Enz, J. (2016b). On the optimal degree of fluctuations in practice for motor learning. Hum. Mov. Sci. 47, 231–239. doi: 10.1016/j.humov.2015.06.007
James, E. G. (2014). Short-term differential training decreases postural sway. Gait Posture 39, 172–176. doi: 10.1016/j.gaitpost.2013.06.020
James, E. G., and Conatser, P. (2014). Effects of practice variability on unimanual arm rotation. J. Mot. Behav. 46, 203–210. doi: 10.1080/00222895.2014.881314
Künzell, S., and Hossner, E. J. (2012). Differenzielles Lehren und Lernen: eine Kritik. Sportwissenschaft 42, 83–95. doi: 10.1007/s12662-012-0251-y
Künzell, S., and Hossner, E. J. (2013). Differenzielles Lehren und Lernen: Eine Erwiderung. Sportwissenschaft 43, 61–62. doi: 10.1007/s12662-013-0287-7
Kurz, J., Gosenheimer, A., Schumann-Schmid, B., Steinmetz, F., and Schöllhorn, W. I. (2016). Differenzielles Gangtraining in der stationären Rehabilitation bei Knie- oder Hüft-TEP. B G 32, 221–225. doi: 10.1055/s-0042-119082
Lage, G. M., Ugrinowitsch, H., Apolinario-Souza, T., Vieira, M. M., Albuquerque, M. R., and Benda, R. N. (2015). Repetition and variation in motor practice: a review of neural correlates. Neurosci. Biobehav. Rev. 57, 132–141. doi: 10.1016/j.neubiorev.2015.08.012
Latash, M. L., Scholz, J. P., and Schöner, G. (2007). Toward a new theory of motor synergies. Motor Control 11, 276–308. doi: 10.1123/mcj.11.3.276
Mateus, N., Santos, S., Vaz, L., Gomes, I., and Leite, N. (2015). The effect of a physical literacy and differential learning program in motor, technical and tactical basketball skills. Revista de Psicologia Del Deporte 24, 73–76.
Menayo, R., Encarnación, A., Gea, G. M., and Marcos, P. J. (2014). Sample entropy-based analysis of differential and traditional training effects on dynamic balance in healthy people. J. Mot. Behav. 46, 73–82. doi: 10.1080/00222895.2013.866932
Moher, D., Liberati, A., Tetzlaff, J., and Altman, D. G. (2010). Preferred reporting items for systematic reviews and meta-analyses: the PRISMA statement. Int. J. Surg. 8, 336–341. doi: 10.1016/j.ijsu.2010.02.007
Moher, D., Shamseer, L., Clarke, M., Ghersi, D., Liberati, A., Petticrew, M., et al. (2015). Preferred Reporting Items for Systematic Review and Meta-Analysis Protocols (PRISMA-P) 2015 statement. Syst Rev. 4:1. doi: 10.1186/2046-4053-4-1
Morris, S. B. (2008). Estimating effect sizes from pretest-posttest-control group designs. Organ. Res. Methods 11, 364–386. doi: 10.1177/1094428106291059
Ouzzani, M., Hammady, H., Fedorowicz, Z., and Elmagarmid, A. (2016). Rayyan-a web and mobile app for systematic reviews. Syst. Rev. 5:210. doi: 10.1186/s13643-016-0384-4
Ozuak, A., and Çaglayan, A. (2019). Differential learning as an important factor in training of football technical skills. J. Educ. Training Stud. 7, 68–76. doi: 10.11114/jets.v7i6.4135
Pabel, S. O., Freitag, F., Hrasky, V., Zapf, A., and Wiegand, A. (2018). Randomised controlled trial on differential learning of toothbrushing in 6- to 9-year-old children. Clin. Oral Investig. 22, 2219–2228. doi: 10.1007/s00784-017-2313-x
Pabel, S. O., Pabel, A. K., Schmickler, J., Schulz, X., and Wiegand, A. (2017). Impact of a differential learning approach on practical exam performance: a controlled study in a preclinical dental course. J. Dent. Educ. 81, 1108–1113. doi: 10.21815/JDE.017.066
Pinder, R. A., Davids, K., Renshaw, I., and Araújo, D. (2011). Representative learning design and functionality of research and practice in sport. J. Sport Exercise Psychol. 33, 146–155. doi: 10.1123/jsep.33.1.146
Renshaw, I., Chow, J. Y., Davids, K., and Hammond, J. (2010). A constraints-led perspective to understanding skill acquisition and game play: a basis for integration of motor learning theory and physical education praxis? Phys. Educ. Sport Pedag. 15, 117–137. doi: 10.1080/17408980902791586
Repšaite, V., Vainoras, A., Berskiene, K., Baltaduoniene, D., Daunoraviciene, A., and Sendzikaite, E. (2015). The effect of differential training-based occupational therapy on hand and arm function in patients after stroke: results of the pilot study. Neurol. Neurochir. Pol. 49, 150–155. doi: 10.1016/j.pjnns.2015.04.001
Reynoso, S. R., Sabido Solana, R., Reina Vaíllo, R., and Moreno Hernández, F. J. (2013). Aprendizaje diferencial aplicado al saque de voleibol en deportistas noveles. Apunts Educación Física y Deportes 114, 45–52. doi: 10.5672/apunts.2014-0983.es.(2013/4).114.04
Santos, S., Coutinho, D., Goncalves, B., Schollhorn, W., Sampaio, J., and Leite, N. (2018). Differential learning as a key training approach to improve creative and tactical behavior in soccer. Res. Q. Exerc. Sport 89, 11–24. doi: 10.1080/02701367.2017.1412063
Santos, S., Mateus, N., Sampaio, J., and Leite, N. (2017). Do previous sports experiences influence the effect of an enrichment programme in basketball skills? J. Sports Sci. 35, 1759–1767. doi: 10.1080/02640414.2016.1236206
Savelsbergh, G. J. P., Kamper, W. J., Rabius, J., De Koning, J. J., and Schöllhorn, W. (2010). A new method to learn to start in speed skating: a differencial learning approach. Int. J. Sport Psychol. 41, 415–427.
Schmidt, M., and Hennig, M. (2012). Differenzielles Lernen. Sportwissenschaft 42, 286–287. doi: 10.1007/s12662-012-0273-5
Schmidt, R. (1975). A schema theory of discrete motor skill learning. Psychol. Rev. 82, 225–260. doi: 10.1037/h0076770
Schmidt, R. A., and Lee, T. D. (2013). Motor Learning and Performance: From Principles to Application, 5th Edn. Champaign, IL: Human Kinetics.
Schöllhorn, W., and Horst, F. (2020). Effects of complex movements on the brain as a result of increased decision-making J. Complexity Health Sci. 2, 40–45. doi: 10.21595/chs.2019.21190
Schöllhorn, W. I. (2000). Applications of systems dynamic principles to technique and strength training. Acta Acad. Olymp. Estoniae 8, 67– 85.
Schöllhorn, W. I. (2016). Invited commentary: differential learning is different from contextual interference learning. Hum. Mov. Sci. 47, 240–245. doi: 10.1016/j.humov.2015.11.018
Schöllhorn, W. I., Beckmann, H., and Davids, K. (2010a). Exploiting system fluctuations. Differential training in physical prevention and rehabilitation programs for health and exercise. Medicina-Lithuania 46, 365–373. doi: 10.3390/medicina46060052
Schöllhorn, W. I., Beckmann, H., Janssen, D., and Drepper, J. (2010b). “Stochastic perturbations in athletics field events enhances skill acquisition,” in Motor Learning in Practice : A Constraints-Led Approach, eds I. Renshaw, K. Davids, and G. J. P. Savelsbergh (London: Routledge), 69–82.
Schöllhorn, W. I., Beckmann, H., Michelbrink, M., Sechelmann, M., Trockel, M., and Davids, K. (2006). Does noise provide a basis for the unification of motor learning theories? Int. J. Sport Psychol. 37, 186–206.
Schöllhorn, W. I., Eekhoff, A., and Hegen, P. (2015). Systemdynamik und differenzielles Lernen. Sportwissenschaft 45, 127–137. doi: 10.1007/s12662-015-0366-z
Schöllhorn, W. I., Hegen, P., and Davids, K. (2012). The nonlinear nature of learning - a differential learning approach. Open Sports Sci. J. 5, 100–112. doi: 10.2174/1875399X01205010100
Schöllhorn, W. I., Humpert, V., Oelenberg, M., Michelbrink, M., and Beckmann, H. (2008). Differenzielles und Mentales Training im Tennis. Leistungssport 6, 10–14.
Schöllhorn, W. I., Mayer-Kress, G., Newell, K. M., and Michelbrink, M. (2009a). Time scales of adaptive behavior and motor learning in the presence of stochastic perturbations. Hum. Mov. Sci. 28, 319–333. doi: 10.1016/j.humov.2008.10.005
Schöllhorn, W. I., Michelbrink, M., Welminsiki, D., and Davids, K. (2009b). “Increasing stochastic perturbations enhances acquisition and learning of complex sport movements,” in Perspectives on Cognition and Action in Sport, eds D. Araujo, H. Ripoll, and M. Raab (Berlin: Nova Science Publishers, Inc.), 59–73.
Schöllhorn, W. I., Sechelmann, M., Trockel, M., and Westers, R. (2004). Nie das Richtige trainieren, um richtig zu spielen. Leistungssport 5, 13–17.
Scholz, J. P., and Schöner, G. (1999). The uncontrolled manifold concept: identifying control variables for a functional task. Exp. Brain Res. 126, 289–306. doi: 10.1007/s002210050738
Schoner, G. (1995). Recent developments and problems in human movement science and their conceptual implications. Ecol. Psychol. 7, 291–314. doi: 10.1207/s15326969eco0704_5
Serrien, B., Tassignon, B., Verschueren, J., Meeusen, R., and Baeyens, J.-P. (2019). Short-term effects of differential learning and contextual interference in a goalkeeper-like task: Visuomotor response time and motor control. Eur. J. Sport Sci. 20, 1061–1071. doi: 10.1080/17461391.2019.1696894
Shamseer, L., Moher, D., Clarke, M., Ghersi, D., Liberati, A., Petticrew, M., et al. (2015). Preferred reporting items for systematic review and meta-analysis protocols (PRISMA-P) 2015: elaboration and explanation. BMJ 350:g7647. doi: 10.1136/bmj.g7647
Shea, J., and Morgan, R. (1979). Contextual interference effects of the acquisition, retention and transfer of a motor skill. J. Exp. Psychol. 5, 179–187. doi: 10.1037/0278-7393.5.2.179
Torrents, C., Balagué, N., Perl, J., and Schöllhorn, W. (2007). Linear and nonlinear analysis of traditional and differential strength training. Sportas Biomedicinos Mokslai 3, 39–47. doi: 10.33607/bjshs.v3i66.548
Weisner, K., Knittel, M., Jaitner, T., and Deuse, J. (2019). “Increasing flexibility of employees in production processes using the differential learning approach – adaptation and validation of motor learning theories,” in Advances in Human Factors in Training, Education, and Learning Sciences, eds S. Nazir, A.-M. Teperi, and A. Polak-Sopińska (Cham: Springer International Publishing), 216–225. doi: 10.1007/978-3-319-93882-0_22
Willimczik, K. (2013). Der Wissenschaftler, der von Wahrheit spricht, ist ein Lügner. Sportwissenschaft 43, 58–60. doi: 10.1007/s12662-013-0288-6
Keywords: meta-analysis, contextual interference, sports, variability, motor learning, differential learning
Citation: Tassignon B, Verschueren J, Baeyens J-P, Benjaminse A, Gokeler A, Serrien B and Clijsen R (2021) An Exploratory Meta-Analytic Review on the Empirical Evidence of Differential Learning as an Enhanced Motor Learning Method. Front. Psychol. 12:533033. doi: 10.3389/fpsyg.2021.533033
Received: 06 February 2020; Accepted: 17 March 2021;
Published: 07 May 2021.
Edited by:
Ana Filipa Silva, Polytechnic Institute of Maia, PortugalReviewed by:
John Komar, Nanyang Technological University, SingaporeThorsten Stein, Karlsruhe Institute of Technology (KIT), Germany
Maria João Lagoa, Research Centre in Sports Sciences, Health Sciences and Human Development (CIDESD), Portugal
David Ian Anderson, San Francisco State University, United States
Copyright © 2021 Tassignon, Verschueren, Baeyens, Benjaminse, Gokeler, Serrien and Clijsen. This is an open-access article distributed under the terms of the Creative Commons Attribution License (CC BY). The use, distribution or reproduction in other forums is permitted, provided the original author(s) and the copyright owner(s) are credited and that the original publication in this journal is cited, in accordance with accepted academic practice. No use, distribution or reproduction is permitted which does not comply with these terms.
*Correspondence: Bruno Tassignon, YnJ1bm8udGFzc2lnbm9uQHZ1Yi5iZQ==