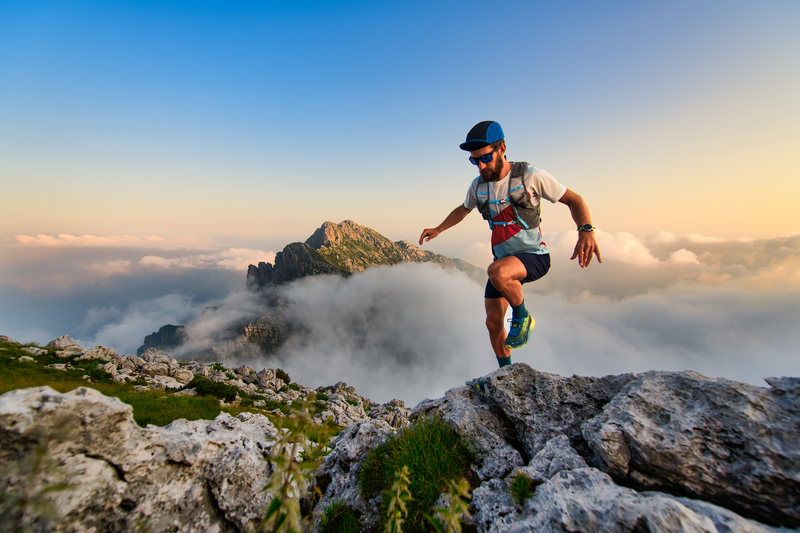
94% of researchers rate our articles as excellent or good
Learn more about the work of our research integrity team to safeguard the quality of each article we publish.
Find out more
ORIGINAL RESEARCH article
Front. Psychol. , 12 February 2021
Sec. Organizational Psychology
Volume 11 - 2020 | https://doi.org/10.3389/fpsyg.2020.632192
This article is part of the Research Topic Coronavirus Disease (COVID- 19): The Impact on the Psychology of Sustainability, Sustainable Development, and Global Economy View all 25 articles
This article aims at answering the following questions: (1) What is the influence of age structure on the spread of coronavirus disease 2019 (COVID-19)? (2) What can be the impact of stringency policy (policy responses to the coronavirus pandemic) on the spread of COVID-19? (3) What might be the quantitative effect of development levelincome and number of hospital beds on the number of deaths due to the COVID-19 epidemic? By employing the methodologies of generalized linear model, generalized moments method, and quantile regression models, this article reveals that the shares of median age, age 65, and age 70 and older population have significant positive impacts on the spread of COVID-19 and that the share of age 70 and older people in the population has a relatively greater influence on the spread of the pandemic. The second output of this research is the significant impact of stringency policy on diminishing COVID-19 total cases. The third finding of this paper reveals that the number of hospital beds appears to be vital in reducing the total number of COVID-19 deaths, while GDP per capita does not affect much the level of deaths of the COVID-19 pandemic. Finally, this article suggests some governmental health policies to control and decrease the spread of COVID-19.
Throughout human history, societies have witnessed numerous epidemic diseases (such as the Black Death, Spanish influenza, SARS, MERS, Ebola, and Zika) that have led to political, social, economic, and technological changes (Garrett, 2007; UN, 2014; Baldwin and Mauro, 2020; Ojo, 2020). The last experienced pandemic is coronavirus disease 2019 (COVID-19), which continues to have a profound global impact on the world. COVID-19, which has been declared a pandemic by the World Health Organization (WHO) on March 11, 2020, is a virus epidemic that has affected the whole world severely since its occurrence in Wuhan, China, in December 2019 (Sohrabi et al., 2020).
As expressed by many eminent scientists and especially by the WHO, the contagiousness of the virus causing COVID-19 disease was high. Over seven million COVID-19 cases and more than 400,000 deaths were reported to WHO by June 2020 (WHO, 2020a). The distribution of total COVID-19 cases around the world was as follows: America (0.478), Europe (0.327), Eastern Mediterranean (0.094), South-East Asia (0.054), Western Pacific (0.027), and Africa (0.020) (WHO, 2020a). In addition, the proportion of deaths was 0.457 (Europe), 0.455 (America), 0.037 (Eastern Mediterranean), 0.026 (South-East Asia), 0.018 (Western Pacific), and 0.008 (Africa) (WHO, 2020a). This virus is novel and no treatment methods such as vaccines or drugs still exist. The virus has inevitably caused severe uncertainty and anxiety in the whole world.
During the epidemic, various economic, and non-economic measures have been taken by governments all over the world (Bruin et al., 2020; Delivorias and Scholz, 2020; Nicola et al., 2020). By following these precautions, the economic and social costs of the pandemic are still being tried to be minimized by eliminating the negative effects of the epidemic. The costs of these pandemic outbreaks in countries can be classified into two groups: (i) direct costs due to emergent need for intervention to outbreaks like medical testing, diagnosis, treatment, and equipment costs; or (ii) indirect costs related to economical production and productivity losses due to the epidemic (Demir, 2020). These costs are expected to have several effects on social and economic life in both the short- and long-term (Ali and Alharbi, 2020; Nicola et al., 2020). These social influences resulted in poverty, income inequality, less access to essential public services, distorted social psychology, and limited social communication. At the same time, the economic effects caused undesirable changes in macroeconomic indicators. According to the estimates of ILO (2020), it is expected that the increase in global unemployment will be 5.3 million for the best scenario and 24.7 million for the worst scenario.
Considering the main effects of the COVID-19 pandemic, demographic characteristics (like age, gender, and others) come to the fore in the distribution of these effects in societies. In studies examining the effects of the COVID-19 pandemic, they have been focused on various topics such as mortality rate (Bonanad et al., 2020; Bulut and Kato, 2020; De Paz et al., 2020; Jin et al., 2020; Liu et al., 2020a; Meo et al., 2020; Roberton et al., 2020), psychological effects (Ahmed et al., 2020; Cao et al., 2020; Gorrochategi et al., 2020; Shrira et al., 2020), and the spread of the disease (Banerjee et al., 2020; Chen Z. et al., 2020; DeBiasi et al., 2020; Dolores De Luca et al., 2020; Huang et al., 2020; Li et al., 2020; Liu et al., 2020b,c; Meo et al., 2020; Mustafa and Selim, 2020; Palmieri et al., 2020). Two main findings have been reached: (a) mortality rates associated with COVID-19 are higher in individuals over 65 years of age than in younger individuals, and (b) by age groups, the impacts of the intensity of COVID-19 disease on community psychology vary. As age increases, negative psychological effects such as stress, anxiety, and depression have occurred more among individuals over 65 years old. Briefly, the specific features of the virus such as high contagion rate and risk of death have different effects primarily on populations with different age structures. For instance, the contagion rate of COVID-19 is higher in children and young age groups, while the mortality rates are higher in older people, especially those over 65 years.
Although there exist several studies examining the effects of COVID-19 from different perspectives in the relevant literature, econometrical analyses estimating the relationship between age structure and the pandemic are limited. Additionally, one may claim that majority of the existing literature follows some comparison analyses and/or some econometric analyses in which heterogeneous structure and quantile structure of the data are not considered.
In this regard, in this research, the outputs from 209 countriesare expected to reveal unbiased, efficient, and consistent results to explore the basic determinants of COVID-19 cases and deaths. The purpose of this article is to determine the impact of age structure and government policy in response to the COVID-19 outbreak (stringency policy) in 209 countries in the world by applying the generalized linear model (GLM), generalized moments method (GMM), and quantile regression model (quantile).
The article is organized as follows: after the section “Introduction,” the second section contains the “Data and Methodology.” The third section introduces the “Study Findings.” The fourth and fifth sections will reveal the “Discussion and Conclusion,” respectively.
The data, data source, and the list of cross-sections (countries) are given in Appendix Tables A1, A2. The variables are total cases per million; total deaths per million; the shares of the median age population, age 65 population, and age 70 and older population; stringency index; GDP per capita; and the number of hospital beds, respectively. The stringency index (or stringency policy index) indicates the governments’ policies in response to the COVID-19 outbreak and contains information on containment and closure policies, such as school closures and restrictions in movement; economic policies, such as income support to citizens or provision of foreign aid; and health system policies such as the COVID-19 testing regime or emergency investments into healthcare.
This article employs the methodologies of GLM, GMM, and quantile regression to yield the relevant model estimations by following the functions given in Eqs 1–6.
Where e, ϕ, η, s, ŗ, and ς denote the residuals of the estimated models. The age variables represent the shares of median age, age 65, and age 70 and older people in the population. #Hospital beds indicate the number of hospital beds.
Generalized moments method, which can be considered as a nested estimation method (maximum likelihood, least squares, instrumental variables, and two-stage least squares), is one of the most preferred methods of econometric analysis in recent years. The GMM allows us to estimate the moments of a population distribution relative to the moments predicted from a particular sample (Burguete et al., 1982; Hansen, 1982; Asteriou and Hall, 2015).
The Arellano and Bond (1991)-type one-step estimator uses the identity matrix as a weighting matrix (Cameron and Trivedi, 2005). The one-step GMM uses the weighting matrix and the estimator as defined in Eqs 7 and 8. It can be shown that the estimator is the optimal panel GMM estimator as ui|Zi. is iid [0,σ2It].
Normal distribution plays an important role in linear and nonlinear regression models. GLMs are those of the nonlinear models without normal distribution assumption in the dependent variable (Collins, 2008).
Generalized linear model consists of three components: distribution of the dependent variable, systematic part with linear estimators, and link function (De Jong and Heller, 2008). The probability density function of the response variable from the exponential distribution family can be written as shown in Eq. 9.
Where k(.),n(.), and m(.) are certain functions. The term φ is the natural location parameter. The term ω is the scale parameter. In Eq. 9, the term φ is the canonical parameter, the term ω is the propagation parameter, and m(x,ω) is a known function. The choice of the functions n(φ) and m(x,ω) determines the actual probability function such as the binomial, normal, or gamma.
Notably, in the case of heterogeneity structure in the variance, the OLS analyses might fail to estimate the coefficients efficiently and consistently. Under heterogeneity structure in the variance, we need alternative regression models such as quantile regression models in which heterogeneity structure and quantile structure of the data are considered (John and Nduka, 2009; Katchova, 2013). Quantile regression aims to estimate the conditional median or other quantiles, such as the 10th quantile, 25th quantile, 75th quantile, and 90th quantile of the response variable (Ong et al., 2015).
Quantile regression determines the model for the selected percentiles in the conditional distribution of the dependent variable (Palma et al., 2020). Hence, traditionally, the linear regression model is expressed as follows:
Parameter p in Eq. 10 shows the number of parameters in the equation. The termi is equal to the number of data points. As a similar formation to the linear regression model, the quantile regression model equation for the τ-th quantile can be written as follows:
Thus, alpha coefficients have become functions that change depending on the quantile (Dye, 2020).
The cases and deaths denote the cases and deaths from the COVID-19 pandemic. The cross-sections have been chosen based on the availability of data for 209 countries as of (a) April 2, 2020; (b) June 2, 2020; and (c) August 2, 2020, and depicted in Figures 1–3, respectively.
Table 1 shows the impacts of different age structures (shares of median age, age 65, and age 70 and older people in the population) on COVID-19 total cases per million as of April 2, 2020, in 209 countries. The second and third columns exhibit the GLM and GMM estimations. Besides GLM and GMM, due to the heteroscedasticity structure of the data, we have decided to follow quantile regression analyses to observe the parameter estimations at different quantiles of the data as the routine recommended in Katchova (2013).
In Table 1, GMM, GLM, and quantile (at 50 and 75 quantiles) regression analyses exhibit significant positive contributions of shares of median age, age 65, and age 70 and older people in the population to the total COVID-19 cases. The quantile estimations did not find a significant impact of the median age population on total cases at the 25th quantile. The quantile 50th (median) estimation reveals that the unit increase in the share of median age people caused the total number of COVID-19 cases to increase by 1.61 units. Moreover, the COVID-19 cases will be 5.88 units (cases) more due to a unit increase in the share of median age people at the 75th quantile. Table 1 reveals that upon a 1-unit increase in the share of median age in the population, the number of total COVID-19 per million increased significantly by 7.91 units (by GLM estimation) and by 6.80 units (by GMM estimation).
Table 1 reaches the evidence that an increase in the percentage of people aged 65 and older increased the total number of COVID-19 cases by 2.42 units at the 25th quantile. The quantile 50th (median) estimation exhibits that a1-unit increase in the percentage of peopleaged 65 and older increased the total number of COVID-19 cases by 8.97 units. The COVID-19 cases will be 24.51 units (cases) more due to a unit increase in the percentage of people aged65 and older at the 75th quantile.
Table 1 indicates the impacts of people aged 70 and over on the total COVID-19 cases. All three estimation models state that the higher the share of people aged70 and over in the population, the more the COVID-19 cases will be. The quantile regression results give more details on the influence of age structure on the number of cases. The quantile estimation results in Table 1 show that if any country has an additional one more unit of the share of the population aged 70 and over, that country will have 3.73 more units of the total cases (at the 25th quantile), 15.42 more units of the total cases (at the 50th quantile), and 42.66 more cases (at the 75th quantile of the total case data) as of April 2, 2020, in 200 countries.
Table 2 yields the influences of the share of the median age population on the COVID-19 pandemic as of June 2, 2020, in 209 countries. GLM, GMM, and quantile estimations reached significant positive coefficients of the share of median age on COVID-19 cases. GLM and GMM estimations are 39.13 and 37.81, respectively. The predictions of quantiles (25), (50), and (75) are 3.94, 12.07, and 46.62, respectively.
The quantile regressions show that the impacts of the share of people aged 65 (share of peopleaged 70) on the expansion of COVID-19 are 18.49 (30.41) at the 25th quantile and 143.04 (214.88) at the 75th quantile on June 2, 2020 (Table 2).
Table 3 yields the magnitudes of the responses of total COVID-19 cases per million to the unit increase in shares of median age, age 65, and age 70 in the population as of August 2, 2020, in 208 countries. In Tables 1–3, the estimation results have similar remarks depicting that the impact of age structure on dispersing of the COVID-19 epidemic (i) tends to increase significantly from median age to age 65 and from age 65 to age 70+ in the population; (ii) tends to increase from April 2, 2020, to August 2, 2020; and (iii) tends to increase considerably from lower quantile to higher quantile of the data for 209 cross-sections.
The impacts of different age structures on COVID-19 total cases in the continents of Europe, America, Asia, and Africa are given in Appendix Table A3. Two main outcomes appear. Firstly, the adverse effects of age structures on total cases in all continents are subject to a prominent increase from median age to age 70 and older people. This output confirms the earlier results given in tables from 1 to 9. Secondly, the negative effects of median age, age 65, and age 70 populations in Europe on COVID-19 cases are greater than the negative effects of the same age groups on COVID-19 cases in the other three continents. The negative effects of these age groups on total cases in the American continent are higher than the negative effects of the same age groups in the other two continents (Asia and Africa). The impact of the COVID-19 pandemic on people with chronic diseases such as hypertension and cardiac insufficiency (Chen Y. et al., 2020; Ip et al., 2020; Polverino et al., 2020; Roncon et al., 2020), diabetes (Bornstein et al., 2020; Guo et al., 2020; Liu et al., 2020c; Zhang et al., 2020c,d), and cancer (Fratino et al., 2020; Zhang et al., 2020a) is more serious. Chronic diseases are especially higher in older adults (Atella et al., 2018; Zhao et al., 2018; NCOA National Council on Aging [NCOA], 2020) because changes in gene expression during aging are seen as one of the most important biological markers of aging and chronic diseases (Mueller et al., 2020).
The average lifetime and population rates in terms of diabetes and smoking might also have a considerable role in the level of the COVID-19 pandemic. In Figures 4A, A.1, A.2 show that the percentages of female smokers in the female populations are relatively higher in Europe and America than those in Asia and Africa and that the percentages of male smokers in the male populations are greater in Asia and Europe than those in Africa and America. The statistical output of high rate of smoking in Europe might be the cause of the higher rate of COID-19 cases in Europe. According to theWHO (2020b), there is evidence that a relationship exists between smoking and increased severity of disease and death in hospitalized COVID-19 patients.
Figure 4A.3 evaluating the prevalence of diabetes, which is one of the chronic diseases, exhibits that the continents with the higher rates in all age groups are America and Asia, respectively. Figure 4A.4 shows that the average life expectancy is relatively higher in the continents of Europe and America than that in Asia and Africa. The longer average lifetime might increase the possibility of having chronic diseases in population density. This interpretation of course needs to be confirmed also by econometric models which can depict the impacts of a lifetime on COVID-19 cases in each continent separately.
In addition to the pie charts (Figure 4A; from Figures 4A.1–A.4), the bar chart (Figure 4B) has been also added to the paper in order for the potential reader to be able to follow the manuscript clearly and fluently.
As a possible determinant of COVID-19 total cases, we also considered the stringency index (government measures/policies in response to the COVID-19 outbreak) for each cross-section in the panel data. The stringency index (stringy policy) exhibits the composite measure based on COVID-19 response indicators including school closures, workplace closures, and travel bans as explained in Appendix Table A1. Table 4 reveals the role of the countries’ stringency policies in determining the spread of total COVID-19 cases in panel data as of April 2020 and June 2020, respectively.
Since the stringency index is not available during August 2020, we could not observe its impact on total cases during August. The GLM and GMM outputs yielded a negative sign but insignificant effects of stringency policy on total cases (to save space, we do not place these outputs here). Quantile regressions, on the other hand, revealed significant negative estimated impacts of stringency policy on COVID-19 total cases. The tables underline that (a) the increase in median age, age 65, and age 70 and older population will increase the COVID-19 cases during both April and June 2020; (b) the stringency policy is found significant to lower COVID-19 cases at the 25th, 50th, and 75th quantiles during April 2020; and (c) the stringency policy is important to diminish COVID-19 cases at the 75th quantile in June 2020.
Table 4 results yield that the governmental policies in response to the COVID-19 pandemic through governments’ restrictions on traditional (face-to-face) education, traditional daily social life, commerce, travel, and public and private sector services became successful to reduce the spread of COVID-19 disease.
The restrictions brought about distance education, social distancing, delays in travel, and closures of public and private working places, entertainment places, and wedding venues; church and mosque activities and other types of social gatherings were also restricted. For instance, according to Beck and Hensher (2020), the bans/restrictions due to the COVID-19 outbreak in Australia were successful through the government’s request to “stay at home.” The restriction policies, besides other privileges, targeted to impose some implementations on high-risk populations (e.g., older aged people and people having several infectious) to prevent the healthcare system from being overwhelmed by reducing especially the demand for intensive care units.
The article additionally analyzes the total deaths due to COVID-19 (Appendix Table A4). Appendix Table A4 has four emergent outcomes: (i) The number of COVID-19 cases is significant in determining the number of COVID-19 deaths; (ii) no matter what the age structure (the share of the median age, age 65, and age 70 in the population) is, it has positive significant impacts on COVID-19 deaths; (iii) the number of hospital beds has a negative significant effect on COVID-19 deaths; and (iv) GDP per capita has a negative significant effect on the number of deaths in the model in which median age is employed. In the models in which the age 65 and age 70 populations are employed, the income (per capita GDP) seems to be insignificant.
As GDP per capita increases, the COVID-19 death rate of the median age population gets lower. As the share of the median age population increases, the number of total deaths per million decreases. If hospital beds per thousand increased by 1 unit, total deaths per million will decrease by 1.84 units. Another reason for the increase in COVID-19 deaths is total cases per million. On the other hand, the findings show that GDP does not help people aged 65 and 70 and older to prevent death due to COVID-19. Considering especially the G20 countries, it might be observed that there is no common association between the values of per capita GDP and mortality. In some countries, per capita GDP and mortality rates from COVID-19 follow a positive association, while in some countries, these two variables move in the opposite direction.
For instance, taking into account the G20 countries with the highest income, while the mortality rates from COVID-19 are found relatively higher in the United States, the United Kingdom, and France, these rates are found relatively lower in Australia, Canada, Germany, and Japan (WHO, 2020b; World Bank, 2020).
On the other hand, the relationship can be evaluated in terms of diagnostic capabilities which refer to the equipment required at the diagnosis stage, such as equipment, testing, and laboratories (Aggarwal et al., 2015). For COVID-19, diagnostic tests (PCR and CRISPR) have stood out among these capabilities (Vandenberg et al., 2020). It might be stated that there is a heterogeneous structure between these tests representing diagnostic capabilities and GDP levels of countries. The total number of tests is subject to change from one high-income country to another high-income country. For instance, the tests realized in the United States, the United Kingdom, France, and Canada are 222 million, 47 million, 29 million, and 13 million, respectively. The number of tests implemented in countries with relatively low income might also vary significantly. For instance, the test numbers of China, India, Turkey, and Pakistan are 160 million, 155 million, 21 million, and 6 million, respectively (Statista, 2020).
Although different ages (median, 65, and 70+) have significant positive contributions to the number of total COVID-19 cases, the older population has a relatively more significant contribution to the number of cases due to some chronic illnesses that older people may have, such as heart disease, cancer, and diabetes.
The question is whether health administrators can follow more efficient policies that can lower the increasing rate of chronic illnesses. Health administrations, with greater allocated administrative budgets, can follow more efficient public health policies to reduce tobacco use and exposure to secondhand smoke and poor nutrition, including diets low in fruits and vegetables and high in sodium and saturated fats. Besides, relevant policies should be implemented to increase physical activity and to reduce excessive alcohol use in societies (CDC, 2020). It is also necessary to keep people away from the use of nonprescription drugs (Seyed-Hosseini et al., 2010).
The bans and/or restrictions due to the COVID-19 epidemic were found successful through the administrators’ policy to “stay at home.” However, one might ask if authorities considered also the adverse effects of the stringency policy. The government policies in response to the COVID-19 pandemic should consider also some potential adverse results such as depression, anxiety, loneliness, stigma, basic commodity insecurity, violence, and other adverse consequences in social life (Zhang et al., 2020b). There exist several articles in the COVID-19 literature regarding mental healthcare from a medical perspective (Teng et al., 2020). To reduce the adverse effect of restriction due to COVID-19, scientists, gerontologists, virologists, therapists, epidemiologists, and policymakers should consider simultaneously to reach a balance in public health (Golubev, 2020).
During the COVID-19 pandemic, the highest priority should be given to functional medical care and vital societal services. To prevent the public from additional physical and mental distress, government authorities, public health management services, and the public itself should consider maintaining physical activity (Jakobsson et al., 2020).
During the COVID-19 pandemic, the countries’ healthcare infrastructure becomes more important to reduce the fatalities caused by the outbreak. In this stage, societies and their representative authorities ought to give more priority to health and environmental issues than other domestic and foreign affairs/politics. Domestic and international actions should keep the current dialog on the role of domestic and global markets in sustainable development considering the quality environment and health (Crawford, 2006; Mihalache-O’Keef, 2018).
Continental differences in the number of high-risk groups, particularly the presence of older people in Europe and the Americas, may raise the question of whether these governments’ public health policies are ineffective. The recent experience postulates the existence of ineffective measures for suppressing and controlling transmission in Europe and the United States. These measures must be supported by financial and social programs that encourage community responses and address the inequities that have been amplified by the pandemic (Alwan et al., 2020). Thus, permanent restrictions will be required in the short-term to lessen transmission and fix ineffective pandemic response systems in order to prevent future lockdowns. By contrast, some countries like Japan, Vietnam, and New Zealand have shown that robust public health responses contribute positively to reduce transmission, allowing life to return to nearnormal. Besides, it is necessary to adopt measures that must change rapidly and fundamentally if these tensions are to be successfully managed, aimed to correct collective action, information asymmetries, irrationality, negative externalities, and the related free-riding phenomenon persistently distorting the Member States’ combined efforts, resulting in deficient attempts to contain the spread of COVID-19 (Kovac et al., 2020). Hence, the lack of institutional mechanisms, long-term planning, and willingness to invest in healthcare have all come to light with the COVID-19 outbreak (Peeri et al., 2020). In consequence, both regions, Europe and the United States, need to assume a well-coordinated policy response to limit economic, social, and health damage and re-establish financial stability (Regling, 2020).
We conducted multicollinearity tests for estimated models through VIF analyses. The findings indicate that all estimated models, except the model in which the independent variables are median age, age 65, and age 70 populations, do not suffer from multicollinearity. Multicollinearity needs to be considered seriously. On the other hand, the multicollinearity problem may not be a serious problem when R2 is high and individual t statistics are significant (Johnston, 1984). Blanchard (1987) also states that multicollinearity is essentially a data deficiency problem and sometimes researchers might have no choice over the available data for parameter estimations (Kennedy, 1998). However, considering multicollinearity a problem in estimations, further study might launch ridge regression and/or a combination of cross-sectional data and time series (pooled data) or drop some variables from the model to eliminate the multicollinearity issue.
Although our research conducted efficient panel model estimations, future researches might follow different methodologies such as panel spatial models to discover the direct and indirect effects of explanatory variables on COVID-19 cases. Our research employed 209 cross-sectional observations of April, June, and August 2020. Due to the lack of data for the stringency index in August, this article could not analyze the impact of government policies in response to the COVID-19 outbreak during August. Future works might explore the stringency index of August 2020 and analyze its effect on the spread of the COVID-19 pandemic. Future works can also consider analyzing the adverse effects of government policies in response to the COVID-19 epidemic (depression, anxiety, loneliness, and stigma) quantitatively.
This article considers evaluating the potential determinants of the spread of COVID-19 pandemic disease in 209 countries around the world. This work has observed (a) the impacts of the shares of median age and older age population (age 65 and age 70 or older) on COVID-19 total cases, (b) the effect of stringency policy on COVID-19 cases, and (c) the influences of total COVID-19 cases, age structure, number of hospital beds, and income level on the number of total deaths due to COVID-19. The highlights and results of this research are as follows:
(i) GLM, GMM, and quantile regressions indicate that age structure is vital in determining the total number of cases in April, June, and August of 2020. The impact of age structure on COVID-19 shows a prominent increase from median age to age 70 and older people. According to quantile (75) regression analyses in August 2020, the effect of a unit increase in the age 65 population on total cases is three times greater than the effect of a unit increase in the median age population on total cases. The impact of the share of age 70 in the population on total cases is 1.6 times greater than that of the share of the age 65 population.
(ii) Stringency policies cover government policies inresponse to the COVID-19 outbreak such as school closures, workplace closures, travel bans, and others, which are found significant to lower COVID-19 cases during April and June 2020. The bans/restriction regulations should impose some additional practices on high-risk people (older people with chronic disease) to reduce the demand for intensive care units of healthcare systems.
(iii) The estimations also yield that the availability of more hospital beds can reduce the number of deaths stemming from the COVID-19 epidemic. This output indicates the necessity of more employment of physical/technical equipment and associated medical personnel, especially in intensive care units.
(iv) Except GLM estimations, GMM and quantile regression estimations assert that GDP per capita does not matter in both less developed and developed countries, which have been affected by the corona pandemic. This outcome might exhibit evidence that low-income countries as well as high-income countries couldnot prevent their societies from deaths due to COVID-19 disease.
(v) Continental-based findings indicate that higher-risk groups (with the presence of older people) in Europe and America are higher than those in Asia and Africa.
The outputs of this work might suggest that policymakers of both developed and less developed countries follow strict health policies to diminish the adverse effects of COVID-19 disease. The relevant health policies might be (a) the policy of staying at home; (b) if necessary, considering meetings only in hygienic places by observing social distancing efficiently and by wearing masks; (c) the policy of recommending/supporting the essential medicine and health products/technologies; (d) following healthy diabetes/eating/drinking modules/programs; (e) considering regular sport and exercises; (f) following distance education programs; (g) subsidizing e-commerce and/or shopping through media; and (g) implementing the policy for people aged 65 or over to follow strictly items (a), (b), (c), (d), (e), and (f). These suggestions have the potential to lower the level of undesired adverse effects of COVID-19 disease in the world.
Overall, this research paper underlines the fact that age 65 or 70 and older populations might affect prominently the undesired results of the pandemic. This article, hence, suggests that administrators follow the outcomes of this research to diminish the potential current and future influences of COVID-19 on the welfare of the societies through some efficient health, education, commerce, and social programs.
The original contributions presented in the study are included in the article/Supplementary Material, further inquiries can be directed to the corresponding author/s.
FB: conceptualization, methodology, data curation and analysis, visualization, project administration—supervision and coordination, writing, original draft preparation, reviewing, editing, and validation. MD: project administration—supervision and coordination, writing, original draft preparation, reviewing, editing, and validation. SK: software, conceptualization, analysis, project administration—coordination, writing, original draft preparation, reviewing, and editing. DL: investigation, conceptualization, analysis, project administration—coordination, writing, original draft preparation, reviewing, and editing. FÜ: data curation, original draft preparation, reviewing, editing, and validation. PG: writing, original draft preparation, and reviewing. EM: original draft preparation, reviewing, and editing. All authors contributed to the article and approved the submitted version.
The authors declare that the research was conducted in the absence of any commercial or financial relationships that could be construed as a potential conflict of interest.
The Supplementary Material for this article can be found online at: https://www.frontiersin.org/articles/10.3389/fpsyg.2020.632192/full#supplementary-material
Aggarwal, R., Ringold, S., Khanna, D., Neogi, T., Johnson, S. R., Miller, A., et al. (2015). Distinctions between diagnostic and classification criteria? Arthritis Care Res. (Hoboken) 67, 891–897. doi: 10.1002/acr.22583
Ahmed, A., Ahmed, O., Aibao, Z., Hanbin, S., Siyu, L., and Ahmad, A. (2020). Epidemic of COVID-19 in China and associated psychological problems. Asian J. Psychiatry 51:102092. doi: 10.1016/j.ajp.2020.102092
Ali, I., and Alharbi, O. M. L. (2020). COVID-19: disease, management, treatment, and social impact. Sci. Total Environ. 728:138861. doi: 10.1016/j.scitotenv.2020.138861
Alwan, N. A., Burgess, R. A., Ashworth, S., Beale, R., Bhadelia, N., Bogaert, D., et al. (2020). Scientific consensus on the COVID-19 pandemic: we need to act now. Lancet 396, e71–e72.
Arellano, M., and Bond, S. (1991). Some test of specification for panel data: Monte Carlo evidence and an application to employment equations. Rev. Econ. Stud. 58, 277–297. doi: 10.2307/2297968
Atella, V., Mortari, A. P., Kopinska, J., Belotti, F., Lapi, F., Cricelli, C., et al. (2018). Trends in age−related disease burden and healthcare utilization. Aging Cell 18:e12861. doi: 10.1111/acel.12861
Banerjee, A., Pasea, L., Harris, S., Gonzalez-Izquierdo, A., Torralbo, A., Shallcross, L., et al. (2020). Estimating excess 1-year mortality associated with the COVID-19 pandemic according to underlying conditions and age: a population-based cohort study. Lancet 395, 1715–1725. doi: 10.1016/S0140-6736(20)30854-0
Beck, M. J., and Hensher, D. A. (2020). Insights into the impact of COVID -19 on household travel and activities in Australia–The early days under restrictions. Transp. Policy 96, 76–93. doi: 10.1016/j.tranpol.2020.07.001
Blanchard, O. J. (1987). [Vector Autoregressions and Reality]: Comment. J. Bus. Econ. Stat. 5, 449–451.
Bonanad, C., García-Blas, S., Tarazona-Santabalbina, F., Sanchis, J., Bertomeu-González, V., Fácila, L., et al. (2020). The effect of age on mortality in patients with Covid-19: a metanalysis with 611,583 subjects. J. Am. Med. Dir. Assoc. 21, 915–918. doi: 10.1016/j.jamda.2020.05.045
Bornstein, R. S., Rubino, F., and Khunti, K. (2020). Practical recommendations for the management of diabetes in patients with COVID-19. Lancet Diabetes Endocrinol. 8, 546–550. doi: 10.1016/s2213-8587(20)30152-2
Bruin, Y. B., Lequarrea, A. S., McCourt, J., Clevestig, P., Pigazzani, F., and Zare, Jeddi M, et al. (2020). Initial impacts of global risk mitigation measures taken during the combatting of the COVID-19 pandemic. Saf. Sci. 128:104773. doi: 10.1016/j.ssci.2020.104773
Bulut, C., and Kato, Y. (2020). Epidemiology of COVID-19. Turk. J. Med. Sci. 50, 563–570. doi: 10.3906/sag-2004-172
Burguete, J., Gallant, R., and Souza, G. (1982). On unification of the asymptotic theory of nonlinear econometric models. Econom. Rev. 1, 151–190. doi: 10.1080/07311768208800012
Cameron, A. C., and Trivedi, P. K. (2005). Microeconometrics Methods and Applications. Cambridge: Cambridge University Press.
Cao, W., Fang, Z., Hou, G., Han, M., Xu, X., Dong, J., et al. (2020). The psychological impact of the COVID-19 epidemic on college students in China. Psychiatry Res. 287:112934. doi: 10.1016/j.psychres.2020.112934
CDC (2020). About Chronic Diseases, National Center for Chronic Disease Prevention and Health Promotion (NCCDPHP). Available online at: https://www.cdc.gov/chronicdisease/about/index.htm (accessed January 12, 2021).
Chen, Y., Gong, X., and Wang, L. (2020). Effects of hypertension, diabetes and coronary heart disease on COVID-19 diseases severity: a systematic review and meta-analysis. medRxiv [Preprint] doi: 10.1101/2020.03.25.20043133
Chen, Z., Fan, H., Cai, J., Li, Y., Wu, B., Hou, Y., et al. (2020). High-resolution computed tomography manifestations of COVID-19 infections in patients of different ages. Eur. J. Radiol. 126:108972. doi: 10.1016/j.ejrad.2020.108972
Collins, D. (2008). The Performance of Estimation Methods for Generalized Linear Mixed Models. Ph.D. thesis, University of Wollongong, School of Mathematics and Applied Statistics- Faculty of Informatics, 223, Wollongong NSW.
Crawford, M. (2006). No watertight compartments: trade agreements, International Health Care Reform, and the legal politics of public sector exemptions. Policy Soc. 25, 1–21. doi: 10.1016/S1449-4035(06)70080-7
De Jong, P., and Heller, D. G. Z. (2008). Generalized Linear Models for Insurance Data. London: Cambridge University Press.
De Paz, C., Muller, M., Munoz Boudet, A. M., and Gaddis, I. (2020). Gender Dimensions of the COVID-19 Pandemic (No. 33622). The World Bank Group Policy Notes. Available online at: http://documents.worldbank.org/curated/en/618731587147227244/Gender-Dimensio ns-of-the-COVID-19-Pandemic (accessed April 16, 2020).
DeBiasi, R. L., Song, X., Delaney, M., Bell, M., Smith, K., Pershad, J., et al. (2020). Severe COVID-19 in children and young adults in the Washington, DC metropolitan region. J. Pediatrics 223, 199–203.e1. doi: 10.1016/j.jpeds.2020.05.007
Delivorias, A., and Scholz, N. (2020). Economic Impact of Epidemics and Pandemics. Rue Wiertz: European Parliamentary Research Service Briefing.
Demir, İ (2020). Kovid-19 Salgının Seyri ve Türkiye Ekonomisi. Ankara: Ankara Yıldırım Beyazıt Üniversitesi Uluslararası İlişkiler ve Stratejik Araştırmalar (ULİSA) Enstitüsü Politika Notu, 7–16.
Dolores De Luca, C. D., Esposito, E., Cristiani, L., Mancino, E., Nenna, R., Cortis, E., et al. (2020). Covid-19 in children: a brief overview after three months experience. Paediatric Respir. Rev. 35, 9–14. doi: 10.1016/j.prrv.2020.05.006
Dye, S. (2020). Quantile Regression. Available online at: https://towardsdatascience.com/quantile-regression-ff2343c4a03 (accessed July 07,2020).
Fratino, L., Procopio, G., Maio, M. D., Cinieri, S., Leo, S., and Beretta, G. (2020). Coronavirus: older persons with cancer in Italy in the COVID- 19 pandemic. Front. Oncol. 10:648. doi: 10.3389/fonc.2020.00648
Garrett, T. A. (2007). Economic Effects of the 1918 Influenza Pandemic. St. Louis, MO: Federal Reserve Bank.
Golubev, A. G. (2020). COVID-19: a challenge to physiology of aging. Front. Physiol. 11:584248. doi: 10.3389/fphys.2020.584248
Gorrochategi, M. P., Munitis, A. E., Santamaria, M. D., and Ozamiz Etxebarria, N. (2020). Stress, anxiety, and depression in people aged over 60 in the COVID-19 outbreak in a sample collected in Northern Spain. Am. J. Geriatric Psychiatry 28, 993–998. doi: 10.1016/j.jagp.2020.05.022
Guo, W., Li, M., Dong, Y., Zhou, H., Zhang, Z., and Tian, C. (2020). Diabetes is a risk factor for the progression and prognosis of COVID-19. Diabetes Metab. Res. Rev. 36:e3319. doi: 10.1002/dmrr.3319
Hansen, L. P. (1982). Large sample properties of generalized method of moment estimators. Econometrica 50, 1029–1054. doi: 10.2307/1912775
Huang, L., Zhang, X., Zhang, X., Wei, Z., Zhang, L., Xu, J., et al. (2020). Rapid asymptomatic transmission of COVID-19 during the incubation period demonstrating strong infectivity in a cluster of youngsters aged 16-23 years outside Wuhan and characteristics of young patients with COVID-19: a prospective contact-tracing study. J. Infect. 80, e1–e13. doi: 10.1016/j.jinf.2020.03.006
ILO (2020). COVID-19 ve Çalışma Yaşamı: Etkiler ve Yanıtlar. Uluslararası Çalışma Örgütü Bilgi Notu. Available online at: https://www.ilo.org/ (accessed April, 25 2020).
Ip, A., Parikh, K., and Parrillo, J. E. (2020). Hypertension and renin–angiotensin–aldosterone system inhibitors in patients with Covid-19. medRxiv [Preprint] doi: 10.1101/2020.04.24.20077388
Jakobsson, J., Malm, C., Furberg, M., Ekelund, U., and Svensson, M. (2020). Physical activity during the coronavirus (COVID-19) pandemic: prevention of a decline in metabolic and immunological functions. Front. Sports Act. Living 2:57. doi: 10.3389/fspor.2020.00057
Jin, J., Bai, P., He, W., Wu, F., Liu, X. F., Han, D. M., et al. (2020). Gender differences in patients with COVID-19: focus on severity and mortality. Front. Public Health 8:152. doi: 10.3389/fpubh.2020.00152
John, O. O., and Nduka, E. C. (2009). Quantile regression analysis as a robust alternative to ordinary least squares. Sci. Afr. 8, 61–65. doi: 10.14419/ijasp.v3i2.4686
Katchova, A. (2013). Quantile Regression Example. Available online at: https://sites.google.com/site/econometricsacademy/econometrics-models/quantile-regression (accessed December 10, 2020).
Kovac, M., Elkanawati, A., and Gjikolli, V. (2020). The COVID-19 pandemic: collective action and European public policy under stress. Law & Economics of COVID-19 Working Paper Series 3:20201.
Li, H., Liu, S., Yu, X., Tang, S. L., and Tang, C. K. (2020). Coronavirus disease 2019 (COVID-19): current status and future perspectives. Int. J. Antimicrob. Agents 55:105951. doi: 10.1016/j.ijantimicag.2020.105951
Liu, K., Chen, Y., Lin, R., and Han, K. (2020a). Clinical features of COVID-19 in elderly patients: a comparison with young and middle-aged patients. J. Infect. 80, e14–e18. doi: 10.1016/j.jinf.2020.03.005
Liu, Y., Mao, B., Liang, S., Yang, J. W., Lu, H. W., and Chai, Y. H. (2020b). Association between age and clinical characteristics and outcomes of COVID-19. Eur. Respir. J. 55:2001112. doi: 10.1183/13993003.01112-2020
Liu, Z., Li, J., Huang, J., Guo, L., Gao, R., Luo, K., et al. (2020c). Association between diabetes and COVID-19: a retrospective observational study with a large sample of 1,880 cases in Leishenshan hospital, Wuhan. Front. Endocrinol. 11:478. doi: 10.3389/fendo.2020.00478
Meo, S. A., Al-Khlaiwi, T., Usmani, A. M., Meo, A. S., Klonoff, D. C., Hoang, T. D., et al. (2020). Biological and epidemiological trends in the prevalence and mortality due to outbreaks of novel coronavirus COVID-19. J. King Saud Univ. 32, 2495–2499. doi: 10.1016/j.jksus.2020.04.004
Mihalache-O’Keef, A. S. (2018). Whose greed, whose grievance, and whose opportunity? Effects of foreign direct investments (FDI) on internal conflict. World Dev. 106, 187–206. doi: 10.1016/j.worlddev.2018.01.012
Mueller, A. L., McNamara, M. S., and Sinclair, D. A. (2020). Why does COVID-19 disproportionately affect older people? Aging 12, 9959–9981. doi: 10.18632/aging.103344
Mustafa, N. M., and Selim, L. A. (2020). Characterisation of COVID-19 pandemic in paediatric age group: a systematic review and meta-analysis. J. Clin. Virol. 128:104395. doi: 10.1016/j.jcv.2020.104395
NCOA National Council on Aging [NCOA] (2020). Healthy Aging Fact. Available online at: https://www.ncoa.org/news/resources-for-reporters/get-the-facts/healthy-aging-facts/ (accessed December 17, 2020).
Nicola, M., Alsafi, Z., Sohrabi, C., Kerwan, A., Al-Jabir, A., Iosifidis, C., et al. (2020). The socio-economic implications of the coronavirus and COVID-19 pandemic: a review. Int. J. Surg. 78, 185–193. doi: 10.1016/j.ijsu.2020.04.018
Ojo, O. B. (2020). Socio-economic impacts of 1918–19 Influenza epidemic in Punjab. J. Asian Afr. Stud. 55:2020. doi: 10.1177/0021909619900906
Ong, B., Lee, T. M., Li, G., and Kuo Chuen, D. L. (2015). Evaluating the potential of alternative cryptocurrencies. Handbook of Digital Currency Bitcoin, Innovation, Financial Instruments, and Big Data, ed. D. L. K. Chuen 81–135. doi: 10.1016/C2014-0-01905-3
Palma, M., Tavakoli, S., Brettschneider, J., Nichols, T. E., and Alzheimer’s Disease Neuroimaging Initiative (2020). Quantifying uncertainty in brain-predicted age using scalar-on-image quantile regression. Neuroimage 219:116938. doi: 10.1016/j.neuroimage.2020.116938
Palmieri, L., Vanacore, N., Donfrancesco, C., Lo, Noce C, Canevelli, M., Punzo, O., et al. (2020). Clinical characteristics of hospitalized individuals dying with COVID-19 by age group in Italy. Gerontol. Soc. Am. 75, 1796–1800.
Peeri, N. C., Shrestha, N., Rahman, M. S., Zaki, R., Tan, Z., Bibi, S., et al. (2020). The SARS, MERS and novel coronavirus (COVID-19) epidemics, the newest and biggest global health threats: what lessons have we learned? Int. J. Epidemiol. 49, 717–726. doi: 10.1093/ije/dyaa033
Polverino, F., Stern, D. A., Ruocco, G., Balestro, E., Bassetti, M., Candelli, M., et al. (2020). Comorbidities, cardiovascular therapies, and COVID-19 mortality: a nationwide, Italian observational study (ItaliCO). Front. Cardiovasc. Med. 7:585866. doi: 10.3389/fcvm.2020.585866
Regling, K. (2020). The Time for EU Solidarity is Now. Available online at: https://www.ekathimerini.com (accessed April 02, 2020).
Roberton, T., Carter, E. D., and Chou, V. B. (2020). Early estimates of the indirect effects of the COVID-19 pandemic on maternal and child mortality in low-income and middle-income countries: a modelling study. Lancet Global Health 8, e901–e908. doi: 10.1016/S2214-109X(20)30229-1
Roncon, L., Zuin, M., Zuliani, G., and Rigatelli, G. (2020). Patients with arterial hypertension and COVID-19 are at higher risk of ICU admission. Br. J. Anaesth. 125, e255–e257. doi: 10.1016/j.bja.2020.04.073
Seyed-Hosseini, M., Taylor, J., and Quest, D. (2010). Discussing side effects of over-the-counter medicines: impact of adding percentage data. Int. J. Pharm. Pract. 18, 275–281. doi: 10.1111/j.2042-7174.2010.00057.x
Shrira, A., Hoffman, Y., and Bodner, E. (2020). COVID-19 related loneliness and psychiatric symptoms among older adults: the buffering role of subjective age. Am. J. Geriatr. Psychiatry 28, 1200–1204. doi: 10.1016/j.jagp.2020.05.018
Sohrabi, C., Alsafi, Z., and O’Neill, N. (2020). World Health Organization declares global emergency: a review of the 2019 novel coronavirus (COVID-19). Int. J. Surg. 76, 71–76. doi: 10.1016/j.ijsu.2020.02.034
Statista (2020). Number of Coronavirus (COVID-19) Tests Performed in the Most Impacted Countries Worldwide as of December 16, 2020. Available online at: https://www.statista.com/statistics/1028731/covid19-tests-select-countries-worldwide/ (accessed December 16, 2020).
Teng, Y.-M., Wu, K. S., and Lin, K. L. (2020). Life or livelihood? Mental health concerns for quarantine hotel workers during the COVID-19 pandemic. Front. Psychol. 11:2168. doi: 10.3389/fpsyg.2020.02168
UN (2014). With Spread of Ebola Outpacing Response, Security Council Adopts Resolution 2177 (2014) Urging Immediate Action, End to Isolation of Affected States. Available online at: https://www.un.org/press/en/2014/sc11566.doc.htm (accessed December 10 2020).
Vandenberg, O., Martiny, D., Rochas, O., van Belkum, A., and Kozlakidis, Z. (2020). Considerations for diagnostic COVID-19 tests. Nat. Rev. Microbiol. 1–13.
WHO (2020a). Coronavirus Disease (COVID-19) Pandemic. Available online at: https://www.who.int/emergencies/diseases/novel-coronavirus-2019 (accessed December 10, 2020).
WHO (2020b). Smoking and COVID-19. Available online at: https://www.who.int/publications/i/item/WHO-2019-nCoV-Sci_Brief-Smoking-2020.2 (accessed October 17, 2020).
World Bank (2020). Global Economic Prospects June 2020. The World Bank Group Flagship Report. Available online at: https://openknowledge.worldbank.org/handle/10986/33748 (accessed June 09, 2020).
Zhang, L., Zhu, F., and Xie, L. (2020a). Clinical characteristics of COVID-19-infected cancer patients: a retrospective case study in three hospitals within Wuhan, China. Ann. Oncol. 31, 894–901. doi: 10.1016/j.annonc.2020.03.296
Zhang, N., Cheng, P., and Jia, W. (2020b). Impact of intervention methods on COVID-19 transmission in Shenzhen. Build. Environ. 180:107106. doi: 10.1016/j.buildenv.2020.107106
Zhang, N., Wang, C., Zhu, F., Mao, H., Bai, P., Chen, L. L., et al. (2020c). Risk factors for poor outcomes of diabetes patients with COVID-19: a single-center, retrospective study in early outbreak in China. Front. Endocrinol. 11:571037. doi: 10.3389/fendo.2020.571037
Zhang, Y., Cui, Y., and Shen, M. (2020d). Comorbid diabetes mellitus was associated with poorer prognosis in patients with COVID-19: a retrospective cohort study. medRxiv [Preprint] doi: 10.1101/2020.03.24.20042358
Zhao, C., Wong, L., Zhu, Q., and Yang, H. (2018). Prevalence and correlates of chronic diseases in an elderly population: a community-based survey in Haikou. PLoS One 13:e0199006. doi: 10.1371/journal.pone.0199006
TABLE A3. The impact of age structure on spread of COVID-19 total cases in continents generalized linear model (Newton-Raphson/Marquardt steps).
Keywords: COVID-19 cases, income, stringency policy, COVID-19 deaths, 70-aged people, median aged people, 65-aged people, government responses
Citation: Bilgili F, Dundar M, Kuşkaya S, Lorente DB, Ünlü F, Gençoğlu P and Muğaloğlu E (2021) The Age Structure, Stringency Policy, Income, and Spread of Coronavirus Disease 2019: Evidence From 209 Countries. Front. Psychol. 11:632192. doi: 10.3389/fpsyg.2020.632192
Received: 22 November 2020; Accepted: 23 December 2020;
Published: 12 February 2021.
Edited by:
Ilhan Ozturk, Çağ University, TurkeyReviewed by:
Michele Maffia, University of Salento, ItalyCopyright © 2021 Bilgili, Dundar, Kuşkaya, Lorente, Ünlü, Gençoğlu and Muğaloğlu. This is an open-access article distributed under the terms of the Creative Commons Attribution License (CC BY). The use, distribution or reproduction in other forums is permitted, provided the original author(s) and the copyright owner(s) are credited and that the original publication in this journal is cited, in accordance with accepted academic practice. No use, distribution or reproduction is permitted which does not comply with these terms.
*Correspondence: Faik Bilgili, ZmJpbGdpbGlAZXJjaXllcy5lZHUudHI=; ZmFpay1iaWxnaWxpQGhvdG1haWwuY29t
†ORCID: Faik Bilgili, orcid.org/0000-0003-4138-6897; Munis Dundar, orcid.org/0000-0003-0969-4611; Sevda Kuşkaya, orcid.org/0000-0003-4527-5713; Daniel Balsalobre Lorente, orcid.org/0000-0002-6099-7899; Fatma Ünlü, orcid.org/0000-0003-1822-9965; Pelin Gençoğlu, orcid.org/0000-0003-2985-2875; Erhan Muğaloğlu, orcid.org/0000-0001-5362-6259
Disclaimer: All claims expressed in this article are solely those of the authors and do not necessarily represent those of their affiliated organizations, or those of the publisher, the editors and the reviewers. Any product that may be evaluated in this article or claim that may be made by its manufacturer is not guaranteed or endorsed by the publisher.
Research integrity at Frontiers
Learn more about the work of our research integrity team to safeguard the quality of each article we publish.