- 1Department of Social Psychology, Zhou Enlai School of Government, Nankai University, Tianjin, China
- 2Department of General Computer, College of Computer Science, Nankai University, Tianjin, China
The COVID-19 pandemic has caused tremendous loss starting from early this year. This article aims to investigate the change of anxiety severity and prevalence among non-graduating undergraduate students in the new semester of online learning during COVID-19 in China and also to evaluate a machine learning model based on the XGBoost model. A total of 1172 non-graduating undergraduate students aged between 18 and 22 from 34 provincial-level administrative units and 260 cities in China were enrolled onto this study and asked to fill in a sociodemographic questionnaire and the Self-Rating Anxiety Scale (SAS) twice, respectively, during February 15 to 17, 2020, before the new semester started, and March 15 to 17, 2020, 1 month after the new semester based on online learning had started. SPSS 22.0 was used to conduct t-test and single factor analysis. XGBoost models were implemented to predict the anxiety level of students 1 month after the start of the new semester. There were 184 (15.7%, Mean = 58.45, SD = 7.81) and 221 (18.86%, Mean = 57.68, SD = 7.58) students who met the cut-off of 50 and were screened as positive for anxiety, respectively, in the two investigations. The mean SAS scores in the second test was significantly higher than those in the first test (P < 0.05). Significant differences were also found among all males, females, and students majoring in arts and sciences between the two studies (P < 0.05). The results also showed students from Hubei province, where most cases of COVID-19 were confirmed, had a higher percentage of participants meeting the cut-off of being anxious. This article applied machine learning to establish XGBoost models to successfully predict the anxiety level and changes of anxiety levels 4 weeks later based on the SAS scores of the students in the first test. It was concluded that, during COVID-19, Chinese non-graduating undergraduate students showed higher anxiety in the new semester based on online learning than before the new semester started. More students from Hubei province had a different level of anxiety than other provinces. Families, universities, and society as a whole should pay attention to the psychological health of non-graduating undergraduate students and take measures accordingly. It also confirmed that the XGBoost model had better prediction accuracy compared to the traditional multiple stepwise regression model on the anxiety status of university students.
Introduction
COVID-19 has spread rapidly around the world and the number of people who were affected has increased dramatically since early 2020 (World Health Organization, 2020). The unprecedented swift and strict quarantine measures starting from late January in China have kept a huge number of people in isolation or socially distancing, and hence has influenced their mental health and psychological resilience (Brooks et al., 2020; Wang et al., 2020a; Xiang et al., 2020). Many studies have noted the psychological impact of COVID-19 such as post-traumatic stress symptoms, confusion, anger, helplessness, fear, depression, and anxiety, etc., in the general population during the initial phase of the COVID-19 outbreak in China (Chew et al., 2020; Li et al., 2020; Qiu et al., 2020; Wang et al., 2020a,b).
During school closures, college students were quarantined and attended their new semester remotely through online learning, and experienced different levels of psychological pressure (Cao et al., 2020; Wang and Zhao, 2020). Bruffaerts et al. (2018) found that university students were more vulnerable and easily affected by a pandemic. Previous studies have reported a higher level and prevalence of anxiety among college students during an epidemic (Jia et al., 2003; Chen et al., 2004; Li et al., 2011; Yang et al., 2015). Chang et al. (2020) investigated 3881 college students in Guangdong province in China during the epidemic of COVID-19 and found that 26.6% of students had different levels of anxiety (23.19% mild, 2.71% moderate, and 0.70% severe) and depression was detected in 21.16% of them. Cao et al. (2020) also reported that 24.9% of medical college students experienced different levels of anxiety during COVID-19. However, there was no research on how their psychological stress and anxiety changed during the outbreak.
There were many factors found to be related to anxiety, Wheaton et al. (2012) reported that health anxiety, contamination fears, and disgust sensitivity were significant predictors of swine flu-related anxiety during the H1N1 influenza pandemic of 2009–2010. Social distancing, worries about their own health and the health of their families, stay-at-home orders, and limited physical interaction with others all counted toward their anxiety and stress (Zuckerman, 1989; Martin, 2010; Cao et al., 2020). Faramarzi et al. (2014) demonstrated that moral intelligence and identity status both contributed to the mental health problems of healthcare students. They used regression analysis or a stepwise model of multiple regression analysis to assess the correlation between different variables to investigate psychological characteristics. However, the predictor variables only accounted for 34% or 22.7% of the variance (Wheaton et al., 2012; Faramarzi et al., 2014). In this article, we also aim to use machine learning to predict the nonlinear relationship between independent and dependent variables in the prediction of psychological status.
Machine learning can deal with big data in high velocity and a variety of forms, so it has been widely implemented in accurately predicting mental health problems, such as anxiety, depression, obsessive-compulsive disorder (OCD), and post-traumatic stress disorder (PTSD) (Kumar et al., 2020; Silveira et al., 2020; Tennenhouse et al., 2020; Xing et al., 2020); classification or diagnosis (Peng et al., 2013; Thabtah, 2018); predicting self-harm and imputing its presence as a missing phenotype (Kumar et al., 2020); and also in distinguishing patients with bipolar disorder from healthy individuals with neuroimaging (Mwangi et al., 2016), neurocognitive data (Wu et al., 2016, 2017a,b), and serum biomarkers (Pinto et al., 2017). This technique includes pattern recognition through the use of complex computational algorithms fed by large data and has the potential to create a paradigm shift in the prediction and stratification of clinical outcomes (Passos et al., 2016; Librenza-Garcia et al., 2017; Silveira et al., 2020). As reported by Ge et al. (2020), machine learning can be used in predicting later clinical outcomes by combining multiple pieces of information from different domains in an effective way and allowing the identification of the most predictive combination of domains. Compared to the traditional statistical prediction models, machine learning approaches may also have advantages in accounting for complex relationships between variables that may not have been previously identified and preventing potentially inaccurate model specifications (Tennenhouse et al., 2020). When there are larger and more complex variables, machine learning becomes a useful technique to disentangle variables associated with outcomes (Iniesta et al., 2016; Dwyer et al., 2018).
This article aims to investigate the prevalence and severity of anxiety among Chinese non-graduating college students and compare the difference between the anxiety status before and 1 month after the start of the new semester of online learning during COVID-19. We also test the ability of the XGBoost model to predict the anxiety level and change of anxiety level 4 weeks after the start of new semester based on the student scores we collected at the start of the new semester. This study is the first to compare the anxiety level of college students before and after the start of a new semester during the COVID-19 pandemic. Our hypothesis is that more non-graduating college students will have a higher level of anxiety in the new semester during COVID-19.
Materials and Methods
Participants
A total of 3800 non-graduating college students aged between 18 and 22 from a top multidisciplinary and research-oriented university directly under the jurisdiction of the Ministry of Education in North China were invited to attend two studies during February 15 to March 17, 2020. In total, 1172 students (female: male = 1.56:1) responded to both studies and the response rate was 30.84% (3611 students attended the first study, however, only 1172 participated in the second one). The students were from 26 colleges and schools within the university which were then categorized into arts or sciences institutions. The participants came from 34 provincial-level administrative units and 260 cities in China, which also represented the distribution of the enrolled students from different regions of China at this university. There were also 36 students from Hubei province and one from Wuhan city, where the majority of cases with COVID-19 were identified during the pandemic in China.
Measures
The questionnaire package used in these two studies consisted of three components: a sociodemographic questionnaire that required each student to provide their gender, year of study, city or province they were living in, major and colleges or schools; a measure of student anxiety (the Self-Rating Anxiety Scale); and an open question about their most recent concerns.
The Self-Rating Anxiety Scale (Zung, 1971)
The Self-Rating Anxiety Scale (SAS), developed by Zung (1971), was used to assess the subjective feeling of anxiety in the two studies. The SAS is a 20-item self-report assessment device built to measure anxiety levels. Each question is scored on a Likert-type scale of 1–4 (based on these replies: “a little of the time,” “some of the time,” “a good part of the time,” and “most of the time”). Some questions were negatively worded to avoid the problem of set response. The overall assessment was calculated by the total score. Among the 20 items, 5 were reverse scored. The total raw scores ranged from 20 to 80 and then needed to be converted to an “Anxiety Index” score which was equal to 1.25 × raw score and became the standard score which was then used to determine the clinical interpretation of the level of anxiety. The validity and reliability of the instrument has been found to be adequate among Chinese participants. According to the research on the 1158 participants, the levels of anxiety were classified as 25–49 = normal range; 50–59 = mild anxiety levels; 60–69 = moderate anxiety levels; and 70 and above as severe anxiety levels. The mean and standard deviation (SD) of the participants in the two studies were calculated and listed with the number of participants in each group in Table 1.
Procedure
This research was registered and approved by the related ethical committee at the university. The non-graduating college students were invited to participate in the two studies, respectively, during February 15 to 17, 2020, right before the new semester started and March 15 to 17, 2020, 1 month after the new semester based on online learning started. The content of the two studies were the same. Those who agreed in writing to participate were each given an online questionnaire package to complete and return to the researchers.
Statistical Analysis
Statistical analysis was performed using the SPSS 22.0 software. The participating students were divided into different groups according to their SAS scores. Measurement data were expressed as mean and SD. Counting data were expressed by the number of people (%). The descriptive statistics were conducted to illustrate the demographic and other selected characteristics of the participants. The analysis of the relationship between sex, major, grade, region, and anxiety initially used the two-sample t-test. The correlation between the SAS scores and confirmed affected cases in different regions were analyzed by Pearson’s product-moment correlation analysis, and P < 0.05 on double sides was statistically significant.
We also used XGBoost (Chen and Guestrin, 2016), a scalable machine learning system for tree boosting, to establish the prediction model of student anxiety. XGBoost is a tree ensemble model using K additive functions to predict the output. The base classifier of XGBoost are classification and regression trees (CART). The trees are learned by defining an objective function and optimizing it. The objective function is determined by the following equation: . It contains a training loss and a regularization. In our model, there were 20 items in SAS and three variables: gender, major, and grade. We also used stepwise multiple regression to establish the prediction model, and compared the prediction performance.
Results
Overall SAS Mean Scores in the Two Tests
The results demonstrated in Table 1 show that the mean SAS score in the second study was significantly higher than in the first study (P ≤ 0.001). There were 184 (15.7%, Mean = 58.45, SD = 7.81) and 221 (18.86%, Mean = 57.68, SD = 7.58) students who met the cut-off of 50 and were screened as positive for anxiety, respectively, in the two investigations. Both males (P < 0.05) and females (P ≤ 0.001) had a significantly higher level of anxiety in the second study, however, there were no differences on the level of anxiety for those who were identified as anxious in the two studies (P > 0.05). It was also found that students majoring in arts and sciences had a significantly higher level of anxiety in the second study than in the first study (P ≤ 0.001), but there were not any statistically significant differences among those who were identified as anxious (P > 0.05), though the numbers of participants who were screened as positive in the second study were more than in the first study.
Group Comparisons in the Two Tests
Table 1 also shows the comparison of the SAS mean scores in different grades in the two studies. Among freshmen, their mean SAS scores were significantly higher in the second study than in the first study (P ≤ 0.001), and it was also true in females (P = 0.001) and those majoring in sciences (P ≤ 0.001) but not in males (P = 0.15) and those majoring in arts (P = 0.32). Among sophomore students, their mean SAS scores were significantly higher in the second study than in the first study (P = 0.001), and also among those majoring in arts (P < 0.05) but not in males (P = 0.395), females (P = 0.325), and those majoring in sciences (P = 0.394). Among junior students, there were no significant differences in the mean SAS scores between the two studies (P > 0.05). For those who were identified as anxious in both studies, there were no statistically significant differences in the SAS mean scores among participants in each grade (P > 0.05).
SAS Score Ranges in the Two Tests
The SAS score ranges of the participants in the two tests were also calculated. There were 184 (15.7%) and 221 (18.86%) students who met the cut-off of 50 and had different levels of anxiety, respectively, in the two studies. Such as, there were more students identified with mild anxiety in the second study (N = 151, 12.88%) than in the first study (N = 117, 9.98%); roughly the same number of students with moderate anxiety; but more students with severe anxiety in the second study (N = 18, 1.54%) than the first one (N = 13, 1.11%). A total of 109 students (9.30%) were identified as anxious in both studies. Among male students, there were 74 (16.16%) in the first test and 96 (20.96%) in the second test that met the cut-off of 50; and among females, 110 (15.41%) and 125 (17.51%) were identified as anxious, respectively, in the two tests, which showed an increase of males and females who were anxious 1 month after the start of the new semester. Similar results were also found among students who were majoring in arts and sciences, among freshmen and sophomore, but not junior students.
As mentioned in Participants, there were 36 students from Hubei province, among whom one was from Wuhan city. The student from Wuhan city was screened as positive for anxiety in the second study (SAS = 51.25) but not in the first study (SAS = 40). Among all the 36 students from Hubei province, where the majority of affected cases of COVID-19 were confirmed in China, eight students (22.22%) had SAS scores higher than 50 (6 at the mild and 2 at the moderate level) in the first study and 12 students (33.33%) met the cut-off of 50 (10 at the mild, 1 at the moderate, and 1 at the severe level) in the second study. Seven students (19.44%) were identified as anxious in both studies and one had a moderate level of anxiety in the first study but was normal in the second study. Fourteen students (38.89%) had higher SAS scores in the second study than in the first study.
SAS Scores of Participants Who Were Identified as Anxious in the Two Tests
Table 2 demonstrates the SAS mean scores and numbers of participants who were identified as anxious. The SAS mean scores were significantly lower in the second study (Mean = 57.68, SD = 7.58) than in the first study (Mean = 58.45, SD = 7.81) (P < 0.05), though the numbers of anxious participants in the second study (N = 221) were more than in the first study (N = 184). There were more males, females, and students majoring in arts and sciences who met the cut-off of anxiety in the second study than in the first study, though their mean SAS scores, respectively, were not significantly different in the two studies (P > 0.05). As shown in Table 2, the majority of participants (N = 729, 62.2%) were freshmen. The number of sophomore and junior students who were anxious were 312 (26.62%) and 131 (11.18%) respectively, however, there were no significant differences found in the SAS mean scores among the anxious participants in each grade (P > 0.05). In both the first and second academic year, there were more students identified as anxious in the second study than in the first study, but roughly the same numbers of anxious participants among junior students.
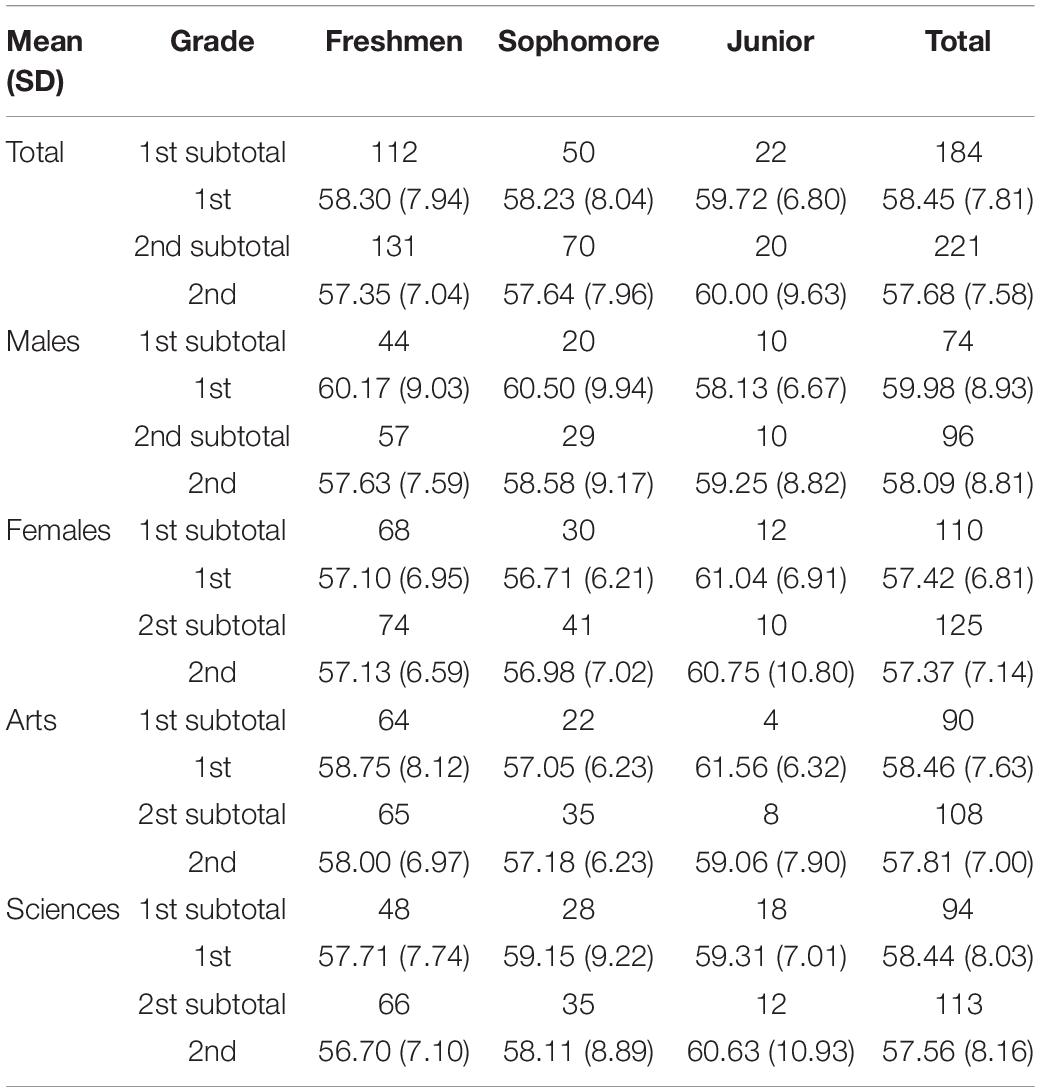
Table 2. Self-Rating Anxiety Scale (SAS) mean scores of participants who were identified as anxious in the two tests (number, mean, and SD).
XGBoost Prediction Model
Scikit-learn, also known as sklearn, is an open source library for machine learning based on Python that supports four machine learning algorithms: classification, regression, reduction, and clustering. We applied the XGBClassifier function of the XGBoost module in the sklearn library.
In this prediction model, the features of participants in the first test can forecast the anxiety levels (normal, mild, moderate, and severe) and changes of anxiety levels (increased, decreased, and unchanged) in the second test. We ranked predictive variables in the model by applying the plot_importance function in the XGBoost module. The feature importance is calculated by gain. The importance of the 20 items in the SAS in the first and second prediction models were both above 95%. So we built two XGBoost classifier prediction models. In one model, we used the 20 items of SAS in the first test together with gender, major, and grade (23 variables altogether) as the feature matrix (X) and in the other model, we only used the 20 items of SAS in the first test as the feature matrix (X). The anxiety levels in the second test and the changes of the anxiety levels were, respectively, used as the labels (y) to train the model and make the prediction. The training set and test set were divided on a scale of 7:3. We adjusted the parameters to construct the best model. We set XGBoost to do multiclass classification using the softmax objective and respectively, set num_class to 4 and 3. We specified the evaluation metrics as merror which was the multiclass classification error rate. The parameter settings are shown in Table 3, and all other parameters that are not in the table were the default values.
The XGBoost model prediction results are shown in Table 4. The accuracy rate was approximately 80%, an ideal result. Therefore, the anxiety levels of the participants can be accurately predicted and it can be possible to implement effective measures before the anxiety levels increase.
We also conducted multiple linear stepwise regression analysis. The prediction results of multiple linear stepwise regression on the anxiety levels (normal, mild, moderate, and severe) are demonstrated in Table 5 and the prediction results of multiple linear stepwise regression on the changes of anxiety levels (increased, decreased, and unchanged) are shown in Table 6. Table 6 shows that there was linear association between the items listed in the table, Nos. 11, 6, 19, 4, 14, 9, 16, 17, 10, 20, 15, and 18 in the first test and the anxiety levels in the second test (P < 0.05). Among these items, the level of No. 6 “My arms and legs shake and tremble” affected the anxiety level in the second test most, which was 12%. Besides, the explanation rate of the regression equation to the anxiety level in the second test was 27.6% (R2 = 0.28, R2adj = 0.276).
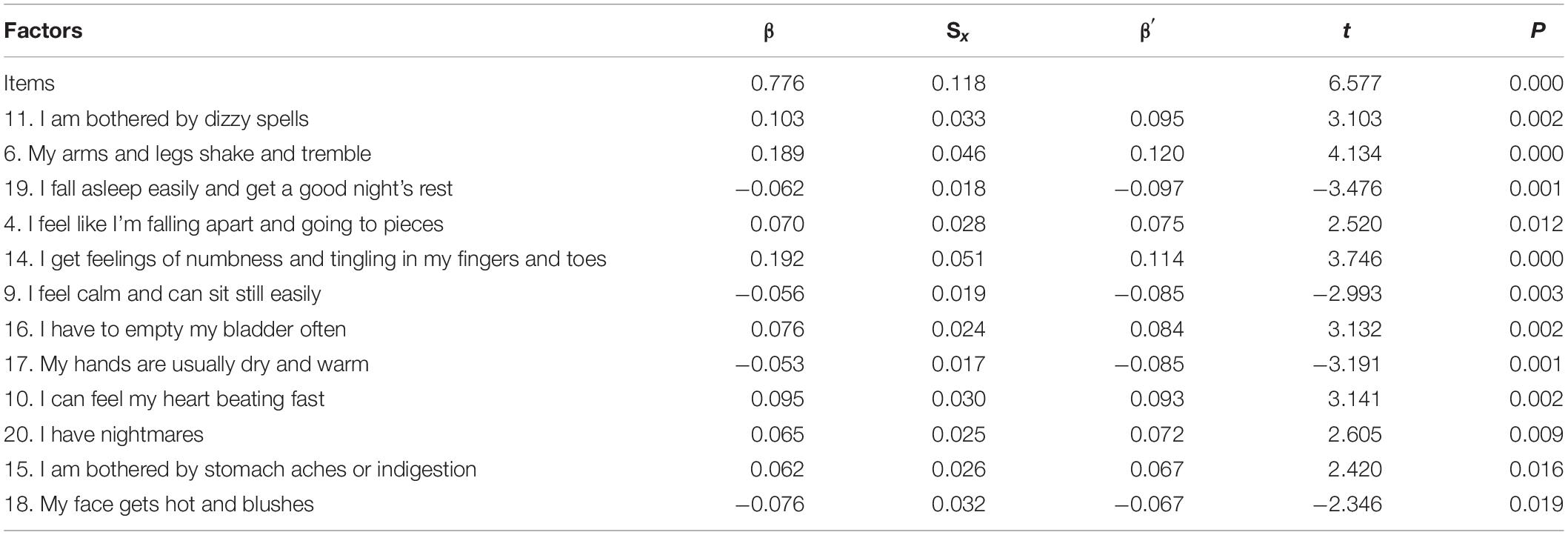
Table 5. The prediction results of multiple linear stepwise regression on the anxiety levels (normal, mild, moderate, and severe).
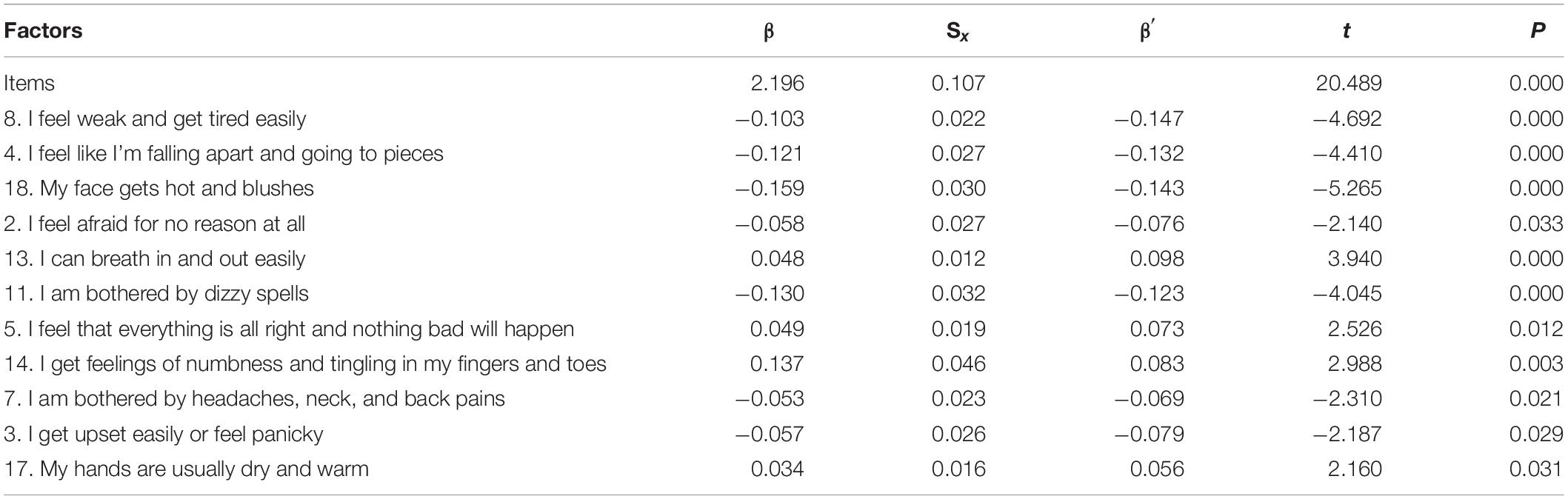
Table 6. The prediction results of multiple linear stepwise regression on the changes of anxiety levels (increased, decreased, and unchanged).
Table 6 also shows that there was linear association between the items listed in the table, Nos. 8, 4, 18, 2, 13, 11, 5, 14, 7, 3, and 17 in the first test and the anxiety levels in the second test (P < 0.05). Among these items, the level of No. 8 “I feel weak and get tired easily” affected the anxiety level in the second test most, which was −14.7%. Besides, the explanation rate of the regression equation to the anxiety level in the second test was 32.1% (R2 = 0.327, R2adj = 0.321).
Discussion
Overall Anxiety Is Higher 1 Month After the Start of the New Semester of Online Learning
Consistent with our hypothesis, the non-graduating undergraduate students had an overall higher level of anxiety and more students were identified as anxious 1 month after the new semester based on online learning started, which was also true among each group such as males, females, and students majoring in arts and sciences. College students are at the early stage of adulthood, lack analytical and decisive abilities and experiences, have unstable emotions and hence are inclined to have impulsive behaviors and be affected by public emergencies (Tan, 2003; Taylor, 2006; Li, 2007; Mei et al., 2011).
In China, the pandemic was first detected in December 2019, reached its peak in mid-February, and then from mid-March when the daily news confirmed that patients reached almost zero, the whole COVID-19 situation was under control (Chinese Center for Disease Control and Prevention, 2020). Many previous studies have focused on the initial stage or more general psychological states during the outbreak, while how anxiety levels and severity changed during this time were still unknown. In this article, we collected data on the psychological status of college students in mid-February when COVID-19 was most prevalent and 4 weeks later in mid-March when the pandemic was stable and under control. At the time of the second test, COVID-19 was assumed to have less of an impact on students. But their anxiety level became higher. This may be due to school closure, social distancing or isolation, and online learning. For college students, especially, a lack of social activities and peer interaction, prolonged holidays, and confounded academic planning, etc., would all account for higher risks of anxiety, fear, stress, and depression (Chang et al., 2020). Unlike China, the pandemic began to boost around early March in other countries and newly confirmed cases were still increasing dramatically in mid-July (World Health Organization, 2020). It would be interesting to compare the differences of psychological consequences on college students before and after the start of a new semester based on online learning in China and the rest of the world where the pandemic was still prevalent.
Group Comparisons
Consistent with previous studies, the younger college students (freshmen and sophomore) had an increased level of anxiety in the new semester but not among junior students as the older the students the more experience they have, and hence their better social adaptive abilities and psychological resilience. It was also proposed in previous research that more prevention measures should be taken to protect the mental health of young students in universities (Yi et al., 2010; Chang et al., 2020). The findings on males and females also confirmed findings from previous studies that females were more vulnerable and more easily affected psychologically. Therefore, female students were found to have a higher level of anxiety in the second test than in the first test, but not among males. Students majoring in arts and sciences both showed higher anxiety in the new semester. However, no differences in the anxiety level of students majoring in arts or sciences between the two tests were found among junior students, which confirmed the findings about the differences of students in the junior grades or more senior grades.
Correlation of SAS Scores and Confirmed Affected Cases or Regions
Different from a previous study that found no correlation between SAS scores and confirmed affected cases (Wang and Zhao, 2020), this study showed that students from Hubei province, where most cases of COVID-19 were confirmed, had a higher percentage of participants with anxiety. This could be explained by the fact that in the new semester the pandemic had a prolonged influence on college students, even though the newly confirmed cases in each city or province had been close to zero. This gave us a hint that even if their anxiety level was not significantly high during the outbreak, the impact of COVID-19 on the psychological states of college students would remain high for quite a while, therefore, measures should be taken to protect and prevent.
XGBoost Prediction Model
Comparing the two XGBoost models, model 1 performed better on the prediction of anxiety level with an accuracy rate of 79.26%, however, model 2 had higher prediction accuracy on the changes of anxiety levels (84.38%). It demonstrated that variables such as gender, grade, and major improved the prediction accuracy on anxiety level but not on the changes of anxiety levels. The results also showed that the performance of the multiple linear regression models was much lower than that of the XGBoost prediction model, as the former could only explain 27.6% of the anxiety level in the second test and 32.1% of the change of anxiety levels in the second test. Hence this article successfully tested the feasibility of the XGBoost model in predicting anxiety level and change of anxiety level in the new semester (Chen and Guestrin, 2016).
Limitations of This Study
This research has several limitations. Firstly, our sample was small and would find it hard to reflect the actual pattern of general non-graduating undergraduate students, given the limited resources available and time-sensitivity of the coronavirus outbreak. The response rate in the first study was 99.86%, however, the majority of the students were not interested in participating in the same test for a second time. In future studies, we propose that we need to make it clear when starting the first test that the research consists of two parts and that one will take place 1 month later to increase the faithfulness of students to this study. Secondly, the self-reported levels of psychological impact, such as anxiety, may not always be consistent with the assessments of professionals. Thirdly, due to the length requirement, we did not collect information on whether the participants had family members who were suspected of or had confirmed cases of COVID-19 which could affect their level of anxiety to a great degree, independent of the time of the tests.
Conclusion
It was concluded that Chinese non-graduating undergraduate students showed higher anxiety in the new semester based on online learning than before the new semester started during the COVID-19 pandemic. More students from Hubei province had different levels of anxiety than from other provinces. Families, universities, and society should pay attention to the psychological health of non-graduating undergraduate students and take measures accordingly. In addition, as this research was the first to compare the impact of COVID-19 on the anxiety of undergraduate students before and after the start of a new semester based on online learning, this study provides invaluable information on the initial psychological anxiety among university students during the early stage of the COVID-19 pandemic from participants across 260 cities in China and the data could also be used as a baseline to further explore the changes and causes of and strategies to reduce their anxiety.
Besides, this article applied XGBoost models to successfully predict the anxiety level and the changes of anxiety levels 4 weeks later based on their SAS scores in the first test. It also confirmed that the XGBoost model had better prediction accuracy compared to the traditional multiple stepwise regression model on the anxiety status of university students.
This research demonstrated the potential of traditional statistical and machine learning models for identifying predictors of anxiety disorder in college students, and has provided insight into which items are most predictive. Areas for future work include external validation of prediction model results, exploration of the predictive ability of the top items for each instrument separately, and subgroup analyses in external datasets with larger and more complex sample sizes, to further assess machine learning model performance among individuals with anxiety conditions. And this XGBoost model could also be implemented in contexts like the global pandemic and the new needs institutions have to address.
Data Availability Statement
The raw data supporting the conclusions of this article will be made available by the authors, without undue reservation.
Ethics Statement
The studies involving human participants were reviewed and approved by the Qiang Li, Chairman of Nankai University, Department of Social Psychology Ethics Committee. The patients/participants provided their written informed consent to participate in this study.
Author Contributions
CW and HZo co-designed the study. HZo conducted the study and analyzed the data. CW interpreted the data, and wrote and revised the manuscript. HZn helped with the data analysis of XGBoost. All authors contributed to the article and approved the submitted version.
Funding
This research was supported by grants from the Tianjin Social Sciences Planning Project (TJQYWT2004-05) to CW and the Teaching Reform Projects of Nankai University in 2019 and 2020 (NKJG2020225, NKJG2020150, and NKJG2019059) to HZo.
Conflict of Interest
The authors declare that the research was conducted in the absence of any commercial or financial relationships that could be construed as a potential conflict of interest.
Acknowledgments
The authors are grateful for all the students who participated in the two studies.
References
Brooks, S. K., Webster, R. K., Smith, L. E., Woodland, L., Wessely, P. S., Greenberg, P. N., et al. (2020). The psychological impact of quarantine and how to reduce it: rapid review of the evidence. Lancet 395, 912–920. doi: 10.1016/S0140-6736(20)30460-8
Bruffaerts, R., Mortier, P., Kiekens, G., Auerbach, R. P., Cuijpers, P., Demyttenaere, K., et al. (2018). Mental health problems in college freshmen: prevalence and academic functioning. J. Affect. Disord. 225, 97–103. doi: 10.1016/j.jad.2017.07.044
Cao, W., Fang, Z., Hou, G., Han, M., Xu, X., Dong, J., et al. (2020). The psychological impact of the COVID-19 epidemic on college students in China. Psychiatry Res. 287:112934. doi: 10.1016/j.psychres.2020.112934
Chang, J., Yuan, Y., and Wang, D. (2020). Mental health status and its influencing factors among college students during the epidemic of COVID-19. J. South Med. Univ. 40, 171–176.
Chen, L., Fu, C., and Li, H. (2004). Study of the relationship between coping style and anxiety of universities students during the SARS. Health Psychol. J. 12, 46–48.
Chen, T., and Guestrin, C. (2016). “XGBoost: a scalable tree boosting system,” in KDD ‘16: Proceedings of the 22nd ACM SIGKDD International Conference on Knowledge Discovery and Data Mining, (San Francisco, CA: ACM), 785–794.
Chew, Q. H., Wei, K. C., Vasoo, S., Chua, H. C., and Sim, K. (2020). Narrative synthesis of psychological and coping responses towards emerging infectious disease outbreaks in the general population: practical considerations for the COVID-19 pandemic. Singapore Med. J. 61, 350–356. doi: 10.11622/smedj.2020046
Chinese Center for Disease Control and Prevention, (2020). 2019ncov. Available online at: chinacdc.cn/2019-nCoV/. Accessed 25 July, 2020).
Dwyer, D. B., Falkai, P., and Koutsouleris, N. (2018). Machine learning approaches for clinical psychology and psychiatry. Annu. Rev. Clin. Psychol. 14, 91–118. doi: 10.1146/annurev-clinpsy-032816-045037
Faramarzi, M., Jahanian, K., Zarbakhsh, M., Salehi, S., and Pasha, H. (2014). The role of moral intelligence and identity styles in prediction of mental health problems in healthcare students. Health 6, 664–672. doi: 10.4236/health.2014.68086
Ge, F., Li, Y., Yuan, M., Zhang, J., and Zhang, W. J. (2020). Identifying predictors of probable posttraumatic stress disorder in children and adolescents with earthquake exposure: a longitudinal study using a machine learning approach. Affect. Disord. 264, 483–493. doi: 10.1016/j.jad.2019.11.079
Iniesta, R., Stahl, D., and McGuffin, P. (2016). Machine learning, statistical learning and the future of biological research in psychiatry. Psychol. Med. 46, 2455–2465. doi: 10.1017/s0033291716001367
Jia, N., Fan, N., and Lu, Z. (2003). A survey of the undergraduate anxiety in the SARS infected areas. J. Hebei Norm. Univ. 5, 57–60.
Kumar, P., Nestsiarovich, A., Nelson, S. J., Kerner, B., Perkins, D. J., and Lambert, C. G. (2020). Imputation and characterization of uncoded self-harm in major mental illness using machine learning. J. Am. Med. Inform. Assoc. 27, 136–146. doi: 10.1093/jamia/ocz173
Li, J., Zhu, L., and Zhang, W. (2011). Investigation of knowledge of prevention and control and psychological anxiety about H1N1 influenza among college students in a university, Zhengzhou. Mod. Prevent. Med. 38, 3036–3042.
Li, S., Wang, Y., Xue, J., Zhao, N., and Zhu, T. (2020). The impact of COVID-19 epidemic declaration on psychological consequences: a study on active weibo users. Int. J. Environ. Res. Public Health 17:2032. doi: 10.3390/ijerph17062032
Librenza-Garcia, D., Kotzian, J. B., Yang, J., Mwangi, B., Cao, B., Lima, L. N. P., et al. (2017). The impact of machine learning techniques in the study of bipolar disorder: a systematic review. Neurosci. Biobehav. Rev. 80, 538–554. doi: 10.1016/j.neubiorev.2017.07.004
Martin, J. M. (2010). Stigma and student mental health in higher education. High. Educ. Res. Dev. 29, 259–274. doi: 10.1080/07294360903470969
Mei, S., Yu, J., He, B., and Li, J. (2011). Investigation and psychological analysis of college students facing public health emergency in one college of Jilin. Med. Soc. 24, 84–86.
Mwangi, B., Wu, M. J., Cao, B., Passos, I. C., Lavagnino, L., Keser, Z., et al. (2016). Individualized prediction and clinical staging of bipolar disorders using neuroanatomical biomarkers. Biol. Psychiatry 1, 186–194. doi: 10.1016/j.bpsc.2016.01.001
Passos, I. C., Mwangi, B., and Kapczinski, F. (2016). Big data analytics and machine learning: 2015 and beyond. Lancet Psychiatry 3, 13–15.
Peng, X., Lin, P., Zhang, T., and Wang, J. (2013). Extreme learning machine-based classification of ADHD using brain structural MRI data. PLoS One 8:e79476. doi: 10.1371/journal.pone.0079476
Pinto, J. V., Passos, I. C., Gomes, F., Reckziegel, R., Kapczinski, F., Mwangi, B., et al. (2017). Peripheral biomarker signatures of bipolar disorder and schizophrenia: a machine learning approach. Schizo Res. 188, 182–184. doi: 10.1016/j.schres.2017.01.018
Qiu, J., Shen, B., Zhao, M., Wang, Z., Xie, B., and Xu, Y. (2020). A nationwide survey of psychological distress among Chinese people in the COVID-19 epidemic: implications and policy recommendations. Gen. Psychiatry 33:e100213. doi: 10.1136/gpsych-2020-100213
Silveira, E. ìM. Jr., Passos, I. C., Scott, J., Bristot, G., Scotton, E., Teixeira Mendes, L. S., et al. (2020). Decoding rumination: a machine learning approach to a transdiagnostic sample of outpatients with anxiety, mood and psychotic disorders. J. Psychiatr. Res. 121, 207–213. doi: 10.1016/j.jpsychires.2019.12.005
Tan, X. (2003). Prevention and Control of Public Health Emergencies. Wuhan: Hubei Province Health Press, 45.
Tennenhouse, L. G., Marrie, R. A., Bernstein, C. N., and Lix, L. M., and Cihr Team in Defining the Burden and Managing the Effects of Psychiatric Comorbidity in Chronic Immunoinflammatory Disease, (2020). Machine-learning models for depression and anxiety in individuals with immune-mediated inflammatory disease. J. Psychosom. Res. 134:110126. doi: 10.1016/j.jpsychores.2020.110126
Thabtah, F. (2018). Machine learning in autistic spectrum disorder behavioral research: a review and ways forward. Inform. Health Soc. Care 44, 278–297. doi: 10.1080/17538157.2017.1399132
Wang, C., Horby, P. W., Hayden, F. G., and Gao, G. F. (2020a). A novel coronavirus outbreak of global health concern. Lancet 395, 470–473. doi: 10.1016/s0140-6736(20)30185-9
Wang, C., Pan, R., Wan, X., Tan, Y., Xu, L., Ho, C., et al. (2020b). Immediate psychological responses and associated factors during the initial stage of the 2019 Coronavirus Disease (COVID-19) Epidemic among the General Population in China. Int. J. Environ. Res. Public Health 17:1729. doi: 10.3390/ijerph17051729
Wang, C., and Zhao, H. (2020). The impact of COVID-19 on anxiety in Chinese university students. Front. Psychol. 11:1168. doi: 10.3389/fpsyg.2020.01168
Wheaton, M. G., Abramowitz, J. S., Berman, N. C., et al. (2012). Psychological predictors of anxiety in response to the H1N1 (Swine Flu) pandemic. Cogn. Ther. Res. 36, 210–218. doi: 10.1007/s10608-011-9353-3
World Health Organization, (2020). Coronavirus Disease 2019 (COVID-19) Situation Report – 186. Geneva: World Health Organization.
Wu, M. J., Mwangi, B., Bauer, I. E., Passos, I. C., Sanches, M., Zunta-Soares, G. B., et al. (2017a). Identification and individualized prediction of clinical pheno- types in bipolar disorders using neurocognitive data, neuroimaging scans and machine learning. Neuroimage 145, 254–264. doi: 10.1016/j.neuroimage.2016.02.016
Wu, M. J., Mwangi, B., Passos, I. C., Bauer, I. E., Cao, B., Frazier, T. W., et al. (2017b). Prediction of vulnerability to bipolar disorder using multivariate neurocognitive patterns: a pilot study. Int. J. of Bip Dis 5:32.
Wu, M. J., Passos, I. C., Bauer, I. E., Lavagnino, L., Cao, B., Zunta-Soares, G. B., et al. (2016). Individualized identification of euthymic bipolar disorder using the Cambridge Neuropsychological Test Automated Battery (CANTAB) and ma- chine learning. J. Affective Disord 192, 219–225. doi: 10.1016/j.jad.2015.12.053
Xiang, Y. T., Yang, Y., Li, W., Zhang, L., Zhang, Q., Cheung, T., et al. (2020). Timely mental health care for the 2019 novel coronavirus outbreak is urgently needed. Lancet Psychiatry 7, 228–229. doi: 10.1016/S2215-0366(20)30046-8
Xing, X., Jin, L., Li, Q., Yang, Q., Han, H., Xu, C., et al. (2020). Modeling essential connections in obsessive-compulsive disorder patients using functional MRI. Brain Behav. 10:e01499. doi: 10.1002/brb3.1499
Yang, X., Jia, W., Xia, G., Wang, J., and Yu, W. (2015). Analysis of the influence of social hierarchy background on depression and anxiety among college students. Chin. J. Dis. Control. Prev. 19, 1269–1272.
Yi, L., Wang, Z., Jiang, Z., et al. (2010). The researches on the psychological intervention of public health emergencies. Chin. J. Public Health 26, 929–930.
Zuckerman, D. M. (1989). Stress, self-esteem, and mental health: how does gender make a difference? Sex Roles 7-8, 429–444. doi: 10.1007/BF00288001
Keywords: COVID-19, college students, anxiety, new semester, machine learning, XGBoost model
Citation: Wang C, Zhao H and Zhang H (2020) Chinese College Students Have Higher Anxiety in New Semester of Online Learning During COVID-19: A Machine Learning Approach. Front. Psychol. 11:587413. doi: 10.3389/fpsyg.2020.587413
Received: 26 July 2020; Accepted: 16 November 2020;
Published: 03 December 2020.
Edited by:
Julia Maria D’andréa Greve, University of São Paulo, BrazilReviewed by:
Daniele Di Lernia, Catholic University of the Sacred Heart, ItalyZhenyu Huang, Central Michigan University, United States
Copyright © 2020 Wang, Zhao and Zhang. This is an open-access article distributed under the terms of the Creative Commons Attribution License (CC BY). The use, distribution or reproduction in other forums is permitted, provided the original author(s) and the copyright owner(s) are credited and that the original publication in this journal is cited, in accordance with accepted academic practice. No use, distribution or reproduction is permitted which does not comply with these terms.
*Correspondence: Hong Zhao, emhhb2hAbmFua2FpLmVkdS5jbg==