- 1Robotics Domain, Italian Institute of Technology, Genoa, Italy
- 2Department of Psychology, George Mason University, Fairfax, VA, United States
Understanding and reacting to others’ nonverbal social signals, such as changes in gaze direction (i.e., gaze cue), are essential for social interactions, as it is important for processes such as joint attention and mentalizing. Although attentional orienting in response to gaze cues has a strong reflexive component, accumulating evidence shows that it can be top-down controlled by context information regarding the signals’ social relevance. For example, when a gazer is believed to be an entity “with a mind” (i.e., mind perception), people exert more top-down control on attention orienting. Although increasing an agent’s physical human-likeness can enhance mind perception, it could have negative consequences on top-down control of social attention when a gazer’s physical appearance is categorically ambiguous (i.e., difficult to categorize as human or nonhuman), as resolving this ambiguity would require using cognitive resources that otherwise could be used to top-down control attention orienting. To examine this question, we used mouse-tracking to explore if categorically ambiguous agents are associated with increased processing costs (Experiment 1), whether categorically ambiguous stimuli negatively impact top-down control of social attention (Experiment 2), and if resolving the conflict related to the agent’s categorical ambiguity (using exposure) would restore top-down control to orient attention (Experiment 3). The findings suggest that categorically ambiguous stimuli are associated with cognitive conflict, which negatively impact the ability to exert top-down control on attentional orienting in a counterpredicitive gaze-cueing paradigm; this negative impact, however, is attenuated when being pre-exposed to the stimuli prior to the gaze-cueing task. Taken together, these findings suggest that manipulating physical human-likeness is a powerful way to affect mind perception in human-robot interaction (HRI) but has a diminishing returns effect on social attention when it is categorically ambiguous due to drainage of cognitive resources and impairment of top-down control.
Introduction
In social interactions, we use information from social cues like gestures, facial expressions, and/or gaze direction to make inferences about what others think, feel, or intend (Adolphs, 1999; Emery, 2000; Gallagher and Frith, 2003). Joint attention or the ability to follow an interaction partner’s gaze in order to conjointly attend to an object of potential interest, is a fundamental social-cognitive mechanism that develops very early in life and is a precursor for higher-order social-cognitive processes, such as mentalizing or action understanding (for a review, see Frischen et al., 2007). In empirical research, joint attention can be investigated using the gaze-cueing paradigm (Friesen and Kingstone, 1998), where an abstract face stimulus is presented in the center of a screen that first looks straight at the participant and then changes its gaze direction to the left or right side of the screen (i.e., gaze cue), which is followed by a target that is presented either at the gazed-at location (i.e., valid trial) or opposite of the gazed-at location (i.e., invalid trial). This typically results in faster reaction times (RTs) to targets presented at valid than invalid locations (i.e., gaze cueing effect). Attentional orienting to gaze cues has traditionally been thought of as reflexive (i.e., a bottom-up process) as it is observable in infants as young as 3 months of age (Hood et al., 1998), is triggered by any kind of stimulus with eye-like configurations (Quadflieg et al., 2004), cannot be suppressed even when gaze direction is unlikely to predict the location of a target (i.e., counterpredictive cueing; Friesen et al., 2004; Vecera and Rizzo, 2006), and is not affected by a resource-demanding secondary task (Law et al., 2010). The few modulatory effects of gaze cueing that were originally reported were strongly dependent on participants’ age (i.e., stronger gaze cueing in children; Hori et al., 2005) and/or other individual traits (i.e., stronger gaze cueing in highly anxious individuals; Tipples, 2006; Fox et al., 2007).
More recently, however, studies have shown that attentional orienting to gaze cues can be top-down modulated when gaze cues are embedded in a richer context (the original experiments used abstract face stimuli; Friesen and Kingstone, 1998) that enhances the social relevance of the interaction for the observer (Tipples, 2006; Fox et al., 2007; Bonifacci et al., 2008; Graham et al., 2010; Kawai, 2011; Hungr and Hunt, 2012; Süßenbach and Schönbrodt, 2014; Wiese et al., 2014; Wykowska et al., 2014; Cazzato et al., 2015; Dalmaso et al., 2016, 2020; Abubshait and Wiese, 2017; Abubshait et al., 2020). Using such “social” versions of the original gaze-cueing paradigm, researchers were able to show that when social relevance is increased based on modulations of similarity-to-self (Hungr and Hunt, 2012; Porciello et al., 2014), physical humanness (Admoni et al., 2011; Martini et al., 2015), facial expression [Bonifacci et al., 2008; Graham et al., 2010; at long stimulus onset asynchrony (SOA) only], social status (Jones et al., 2010; Dalmaso et al., 2012, 2014, 2015; Ohlsen et al., 2013), social group membership (Dodd et al., 2011, 2016; Liuzza et al., 2011; Pavan et al., 2011; Ciardo et al., 2014; Cazzato et al., 2015; Dalmaso et al., 2015), and familiarity (Frischen and Tipper, 2006; Deaner et al., 2007) larger gaze cueing effects were observed (Wiese et al., 2013). Taken together, these findings suggest that engagement in joint attention may strongly depend on social context information, as well as a link between higher-level mechanisms of social cognition related to mentalizing, empathizing, or group membership and lower-level mechanisms of social cognition, such as joint attention (see Capozzi and Ristic, 2018 and Dalmaso et al., 2020, for comprehensive reviews on social factors that influence social attention).
With regard to human-robot interaction (HRI), potentially one of the most powerful contextual factors is the degree to which a robot is perceived to have a mind, with the ability to experience internal states like emotions and intentions and to execute goal-directed actions (i.e., mind perception; Gray et al., 2007). Seeing minds in others is not exclusive to humans, but “mind” can also be ascribed to agents that by definition do not have minds (e.g., robots) or whose mind status is ambiguous (e.g., animals; Gray et al., 2007). Mind perception is an automatic process that can be triggered implicitly when agents possess human-like facial features (Balas and Tonsager, 2014; Deska et al., 2016) or behaviors (Castelli et al., 2000). Decisions as to whether an agent “has a mind” are made within a few 100 ms (Wheatley et al., 2011; Looser et al., 2013), and just passively viewing stimuli that trigger mind perception is sufficient to activate social-cognitive brain networks (Wagner et al., 2011), even if their mind status is irrelevant to the task at hand (Wykowska et al., 2014; Caruana et al., 2015, 2017a). Mind status can also be explicitly ascribed to nonhuman agents when the presence of a human is needed in the current situation or when an entity has become so important to an individual that a “machine” status is no longer sufficient. For instance, agents of ambiguous physical human-likeness are more likely treated as a “human” when individuals are in an increased need of social contact due to chronic loneliness (Hackel et al., 2014) or when participants have to collaborate with them on a joint task (Hertz and Wiese, 2017). Likewise, soldiers who work with search-and-rescue robots on a regular basis are reported to be reluctant to agree to install updates on their robot “companions” because they fear this would change their “personality” (Singer et al., 2008; Carpenter et al., 2016).
Despite being an important question, studies investigating the effect of mind perception on social attention are surprisingly rare and have yielded mixed results depending on how mind perception was manipulated (Teufel et al., 2010; Wiese et al., 2012, 2014; Wykowska et al., 2014; Martini et al., 2015; Abubshait and Wiese, 2017). When mind perception was manipulated via belief (e.g., participants are instructed that changes in a robot’s gaze direction are pre-programmed vs. human-controlled), attentional orienting to gaze cues was enhanced when observed gaze behavior was believed to be caused by a human agent as opposed to a pre-programmed algorithm (Wiese et al., 2012; Wykowska et al., 2014; Caruana et al., 2015). Belief manipulations can also impact participants’ perceptions of the space around them (Müller et al., 2014; Fini et al., 2015), their performance in a joint Simon task (Müller et al., 2011), and their neural responses, as measured by activation in social regions of the brain (Kühn et al., 2014). A similar positive effect was found when mind perception was manipulated via behavior (e.g., predictive vs. random gaze cues), such that larger gaze cueing effects were observed when gaze cues predicted the target location with high likelihood as opposed to being non-predictive (80 vs. 50% predictive; Abubshait and Wiese, 2017). However, when mind perception was manipulated via physical appearance (e.g., gazers of varying degrees of human-likeness), results were more mixed: on the one hand, general differences in gaze cueing mechanisms were found between human and robot agents when using non-predictive cues (Admoni et al., 2011; Wiese et al., 2012), such that robots tended to induced smaller gaze cueing effects than humans when non-predictive gaze cues were used (i.e., 50% predictive of target location); this effect, however, was not further modulated by the robot’s physical human-likeness (Admoni et al., 2011; Martini et al., 2015; Abubshait and Wiese, 2017). On the other hand, a gazing stimulus that possesses very human-like but not perfectly human physical appearance (i.e., morphed images consisting of 70% of a human image and 30% of a robot image) disrupted top-down control of attentional orienting in counterpredictive gaze-cueing paradigms (i.e., targets appear with a higher chance at the uncued location), such that participants were less capable of shifting their attention away from the cued (i.e., not very likely target location) to the predicted (i.e., very likely target location) location when the gazer displayed ambiguous levels of human-likeness, as opposed to an unequivocally “human” or “robot” gazer (Martini et al., 2015).
The assumption that observing stimuli of ambiguous physical human-likeness negatively impacts resource-demanding cognitive processes, such as top-down control of attention, is in line with established biased-competition models of visual processing (Desimone and Duncan, 1995), showing that possible interpretations of ambiguous stimuli compete for representation in visual networks causing cognitive conflict and that cognitive resources are needed to direct selective attention to stimuli features that favor one explanation over the others (via inhibition of alternative category representations) to resolve the cognitive conflict (Meng and Tong, 2004; Sterzer et al., 2009; Ferrey et al., 2015). It is also in line with empirical examinations of the uncanny valley (UV; Mori, 1970) theory that links negative evaluations and long categorization times for ambiguously human-like face stimuli to categorical uncertainty (Cheetham et al., 2011; Hackel et al., 2014; Martini et al., 2016) and consumption of additional cognitive resources compared to categorically unambiguous stimuli (Wiese et al., 2019). Specifically, it was shown that when mind perception was manipulated via physical parameters, for instance, by morphing human images into robot images along a spectrum ranging from 0 to 100% of physical humanness, changes in mind ratings attributed to the resulting images show a categorical pattern, with significant changes in ratings at the human-nonhuman category boundary located at around 63% physical humanness, but only marginal changes in mind ratings for stimuli that unequivocally fall into either the “human” or “nonhuman” category (Cheetham et al., 2011, 2014; Hackel et al., 2014; Martini et al., 2016).
Follow-up studies showed that this qualitative change in mind ratings for stimuli located at the category boundary is associated with increased categorization times, indicating that being exposed to categorically ambiguous stimuli might be associated with increased cognitive processing costs compared to categorically unambiguous stimuli (Cheetham et al., 2011, 2014).
In support of this notion, a follow-up study used mouse tracking (Freeman and Ambady, 2010) to show that this increase in categorization time for stimuli of ambiguous human-likeness is associated with an increase in cognitive conflict, as indicated by larger mouse curvatures for stimuli of ambiguous human-likeness than unequivocally “human” or “robot” stimuli (Weis and Wiese, 2017; Wiese and Weis, 2020). Yet, another follow-up study showed that processing categorically ambiguous stimuli is also associated with an increase in cognitive costs and draining of cognitive resources over time even when the stimuli were irrelevant to the immediate task (Wiese et al., 2019). Specifically, the authors embedded face stimuli of differing levels of human-likeness (0% human, 30% human, 70% human, and 100% human) into a vigilance task, known to be sensitive to the drainage of cognitive resources (Parasuraman et al., 2009), and examined whether a categorically ambiguous stimulus of 70% physical humanness would be associated with a stronger decrease in performance over time (i.e., vigilance decrement) than a categorically unambiguous stimulus of 0, 30, or 100% physical humanness. In line with this assumption, the researchers showed that the 70% human stimuli caused a significantly larger decrement than the 0, 30, and 100% human stimuli, indicating that categorically ambiguous stimuli may drain more cognitive resources over time than categorically unambiguous stimuli, even when being irrelevant to the task (Wiese et al., 2019).
Interestingly, the negative effect on cognitive performance vanished for ambiguous stimuli when participants were perceptually pre-exposed to the stimuli before the task (i.e., both the ambiguous and unambiguous stimuli) by being asked to evaluate the stimuli regarding their capacity of having internal states (i.e., explicit mind perception; e.g., “Can the stimulus feel pain?”) or their perceptual features (i.e., implicit mind perception; e.g., “Does the stimulus have the shape of an avocado?”; Wiese et al., 2019). This suggests that cognitive conflict, when assessing the mind status of stimuli, is triggered by bottom-up mechanisms related to ambiguous perceptual stimulus features (Gao et al., 2010; Wheatley et al., 2011) and the automatic coactivation of competing categories (Ferrey et al., 2015), which can only be resolved by focusing selective attention on a subset of perceptual features that support one category over another – for instance, by pre-exposing participants to the stimuli and directing attention to their perceptual features. Regarding attentional orienting to gaze signals, this means that manipulating the degree to which a gazer is perceived to “have a mind” via physical features can have negative consequences on the effectiveness of a gaze cue when the gazer is of ambiguous physical human-likeness, which could drain cognitive resources and negatively impact top-down control of spatial attention.
Aim of Study
The goal of the current study is to investigate whether a perceptually ambiguous agent induces cognitive conflict due to an increased difficulty in categorizing a face stimulus as “human” or “nonhuman” (Experiment 1), whether the categorically ambiguous face can potentially interfere with top-down control of attentional orienting to gaze cues due to cognitive conflict (Experiment 2), and whether resolving perceptual ambiguity via pre-exposing participants to the stimuli prior to the gaze-cueing task would restore top-down control abilities (Experiment 3). To investigate these questions, we created stimuli of varying degrees of physical humanness – ranging from 0 to 100% human image contained in the morphed image in steps of 20% – and embedded them into a mouse-tracking task (Experiment 1) and a gaze-cueing task (Experiments 2 and 3). The mouse-tracking task is a force-choice categorization task that is designed to investigate the coactivation of two competing categories with larger overlap in coactivation correlating with larger cognitive conflict, as measured by mouse-movement curvatures (Freeman and Ambady, 2010). In the gaze-cueing task, we used a counterpredictive paradigm where participants responded to targets that appeared more often at the uncued location (80% of trials) than the cued location (20% of trials), to disentangle bottom-up from top-down mechanisms (Friesen et al., 2004). In order to optimize task performance, participants have to suppress bottom-up attentional orienting to the cued (but unlikely) target location and, instead, shift their attentional focus to the uncued (but likely) target location via top-down control. If attentional orienting to gaze cues follows a bottom-up pattern, reaction times will be shorter for valid than invalid trials (i.e., a standard gaze cueing effect: invalid minus valid trials reaction time difference is positive); if attentional orienting follows a top-down pattern, reaction times should be shorter for invalid than valid trials (i.e., a reversed gaze cueing effect: invalid minus valid trials reaction time difference is negative). Thus, in counterpredictive gaze-cueing paradigms, the difference in reaction times between invalid and valid trials can be used as a measure for the extent to which gaze cueing is top-down controlled: the more positive (negative) the difference in reaction times, the more pronounced the bottom-up (top-down) component is.
If mind perception caused cognitive conflict for stimuli located at the category boundary between “human” and “nonhuman” (located at around 60–70% physical humanness, as indicated by previous work; Cheetham et al., 2011, 2014; Wiese et al., 2019; Wiese and Weis, 2020), we would expect that the 60% human morph1 would induce the most cognitive conflict that is due to categorization compared to faces that are easily distinguished as “human” or “nonhuman” (Experiment 1). This cognitive conflict should also significantly disrupt top-down control of attentional orienting for the morphed face that showed the most cognitive conflict (Experiment 2). Furthermore, top-down control should be restored when participants are pre-exposed to the stimuli’s perceptual features prior to the gaze-cueing task (Experiment 3).
Experiment 1
The aim of Experiment 1 was to use mouse tracking to measure if perceptually ambiguous faces caused cognitive conflict that is due to categorizing the faces as “human” or “nonhuman” via measures of mouse curvatures and to identify which of the faces was closest to the category boundary. If, indeed, categorically ambiguous faces induced conflict due to categorization, we would expect that mouse curvatures should be largest for the 60% morphed human face as previous literature suggests that the category boundary exists around the 60% physical humanness level (Cheetham et al., 2011, 2014; Hackel et al., 2014; Martini et al., 2016).
Materials and Methods
Participants
Thirty-eight participants were recruited from the George Mason University undergraduate pool (25 females, M age = 20.68, SD = 4.07, range = 18–35). Students were given course credit for completion of the study. All participants reported normal or corrected-to-normal vision and provided written consent prior to participating. All research procedures were approved by George Mason University’s Internal Review Board. All data and analysis scripts can be found on https://osf.io/73pr6/.
Stimuli
The face stimuli were created using FantaMorph, a software that allows two images to be morphed into one another incrementally, resulting in a spectrum ranging from 0% of image A (i.e., 100% of image B) to 100% of image A (i.e., 0% of image B). On the “nonhuman” end of the spectrum, the S2 humanoid robot head developed by Meka Robotics was used. On the “human” end of the spectrum, a male face stimulus from the Karolinska Directed Emotional Faces (KDEF) database was used (Lundqvist et al., 1998). The spectrum comprised of six morph levels, resulting in stimuli of 0, 20, 40, 60, 80, and 100% physical human-likeness; see Figure 1.
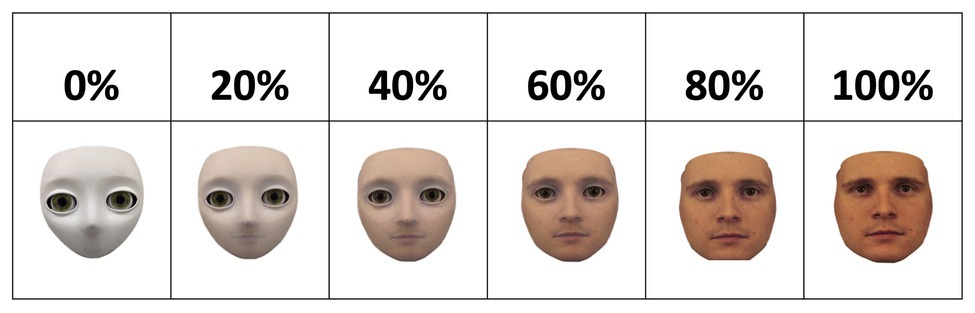
Figure 1. Spectrum of physical humanness ranging from 0 (left) to 100% (right) of physical humanness. Morphed face stimuli were created by morphing the face image of a humanoid robot into the image of a male human face from the Karolinska Directed Emotional Faces (KDEF) database (Lundqvist et al., 1998). The morphed images increase in physical humanness from the left side of the spectrum (i.e., robot) to the other (i.e., human) in increments of 20%.
Task and Procedure
Stimuli were presented (one at a time) at the bottom center of the screen and asked participants to categorize them as either “human” or “nonhuman” by moving their mouse cursor to the respective labels presented in the top left or top right corner of the screen as soon as the image appeared on the screen. The location of the labels was counterbalanced across participants. At the beginning of each trial, participants were asked to move the mouse cursor to a designated starting position, which was located centrally at the bottom of the computer screen, and to click the left mouse button to initiate the trial. Immediately afterward, one of the morphed stimuli was presented centrally at the bottom of the screen, and participants had to move the mouse cursor from the starting position to one of the two category labels located in the top two corners of the screen and click the label. For each morphed image, mouse cursor movement trajectories were measured. After each trial, participants were presented with a blank black screen for an inter-trial interval (ITI) of 1,000 ms to signify the end of the trial; see Figure 2.
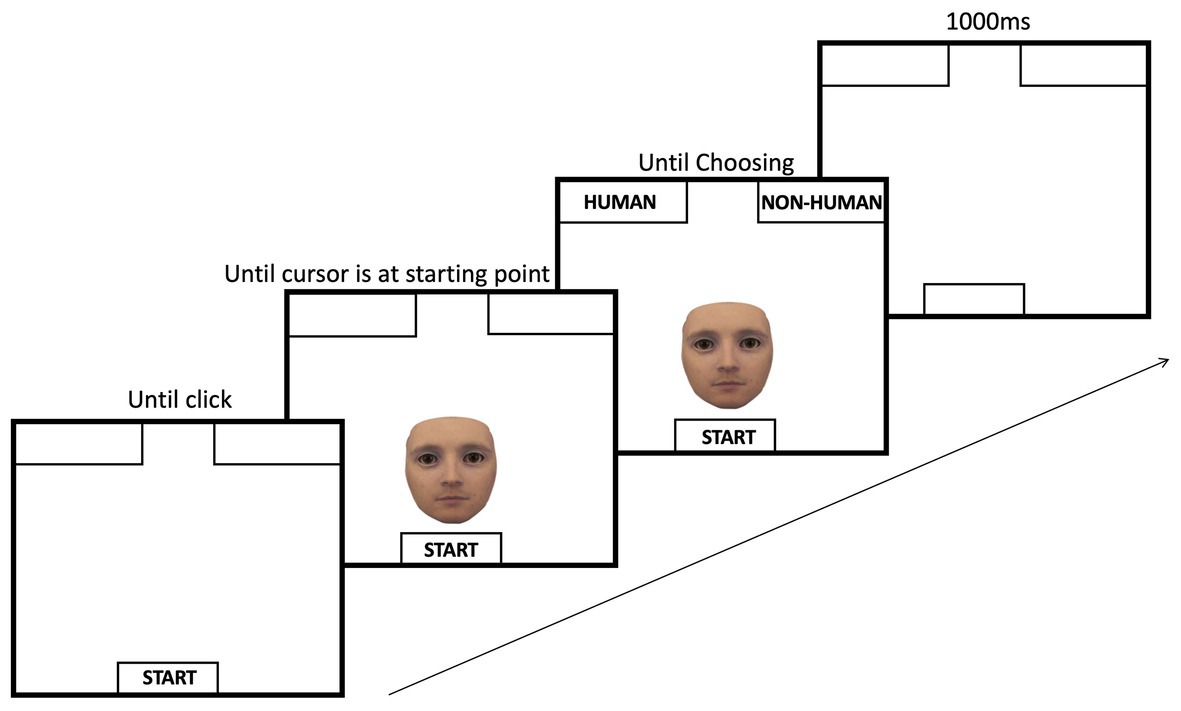
Figure 2. Sequence of events during a trial of mouse tracking. On each given trial, participants moved their mouse cursor to the start position at the bottom of the screen and clicked the start button. After the click, a face would be presented centrally at the bottom of the screen, right above the start button. Immediately after the face is presented, the category labels appeared. Next, the participant moved the mouse to pick one of the categories. The inter-trial-interval (i.e., ITI) was set to 1,000 ms.
Participants were instructed to complete the task as quickly as possible to maximize the likelihood of the mouse moving from the starting position and to limit the number of trials where participants would keep the mouse stationary and only move it once they have categorized the face. We did not want participants to notice that the faces formed a spectrum that progressed systematically in degree of human-likeness to account for any bias that could correspond to the pattern. Therefore, faces were presented to participants in a randomized fashion. Additionally, to further conceal the pattern among the faces, eight decoy human-robot spectrums (that were created with the same procedures) were included among the stimuli. Each experimental session started with a practice block in which participants completed three trials with three different morphed agents that were not included in the main task. After completing the practice block, participants moved to the experimental condition in which they saw all 54 faces in a randomized fashion. Each face was presented once for a total of 54 trials. The study took approximately 20 min to complete.
Analysis
Data were analyzed using R (version 3.6.1). The mouse-tracking software developed by Freeman and Ambady (2010) was used to collect and process mouse tracking data. The software allows researchers to record time-standardized trajectories of the mouse’s movements for a given trial. This allows users to compute the area under the curve (AUC), which is the geometric area of the mouse trajectory from the mouse’s starting point to the end point compared to a straight line trajectory from those points (Freeman and Ambady, 2010). When participants are conflicted between two choices regarding a stimulus, an overlap in activation between the two categories would cause participants to make a choice in a geometrically wide mouse movement, which would result in a large AUC; a stimulus that does not coactivate two categories should induce less conflict and result in a geometrically narrow movement and a small AUC. The general idea underlying mouse tracking is depicted in Figure 3. None of the mouse-tracking trials deviated more than 3 SD from the participant’s mean and were all kept in the analysis.
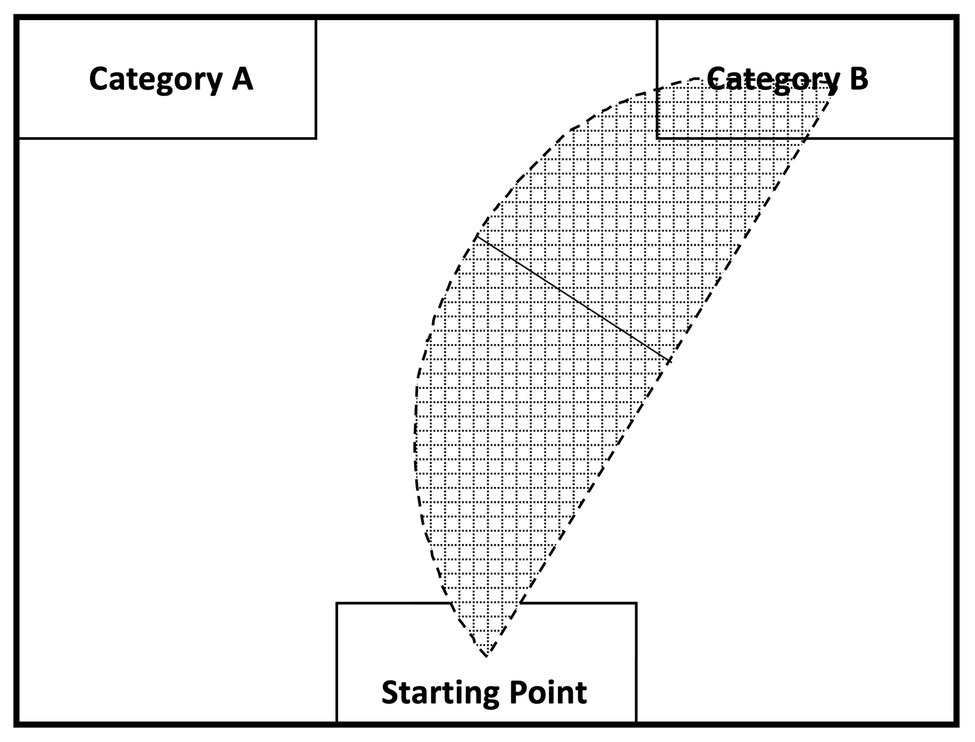
Figure 3. Mouse tracking recording and analysis (adapted from Freeman and Ambady, 2010). The shaded region visualizes how mouse curvature is used to calculate the area under the curve (AUC): the curved line represents the participant’s actual mouse trajectory while picking a category; the straight line represents the theoretical mouse trajectory if no cognitive conflict between the indicated categories occurs for a given stimulus. A comparison is, then, drawn between the maximal deviation of the actual mouse movement and the theoretical straight line to calculate the AUC (i.e., solid black line).
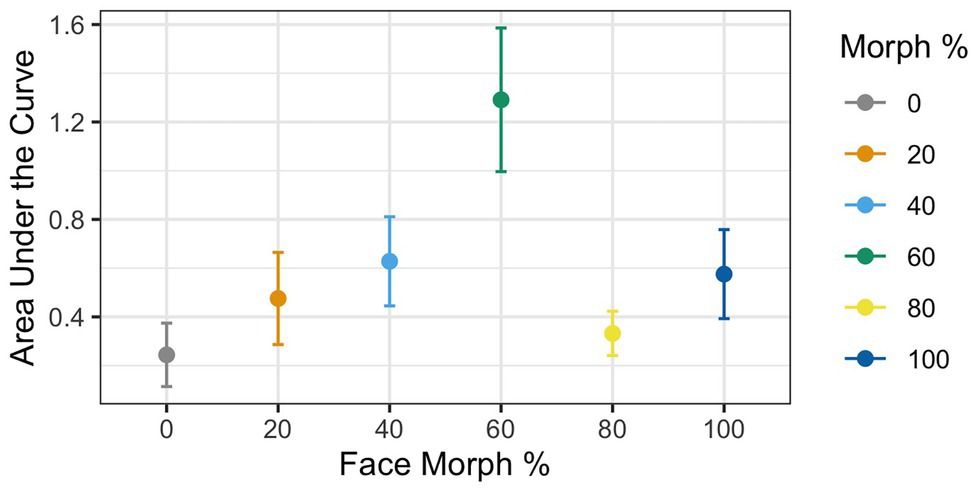
Figure 4. AUC during mouse tracking as a function of physical human-likeness. The 60% human morph is associated with a significantly stronger cognitive conflict than all the other morphs combined.
To analyze the mouse tracking data, a univariate ANOVA with AUC as a dependent variable and Agent Type as a within-participants factor (0% human vs. 20% human vs. 40% human vs. 60% human vs. 80% human vs. 100% human) was conducted. Follow-up t-tests were corrected using the false discovery rate (FDR) procedure.
Results
Greenhouse–Geisser corrections were done (ε = 0.65) due to violation of the sphericity assumption according to the Mauchly test [χ 2(6) = 0.22, p < 0.001]. Results revealed a significant main effect of Agent Type [F(1,37) = 3.83, p = 0.009, ηG 2 = 0.08], with mouse curvatures varying as a function of physical human-likeness. To examine whether mouse curvatures were more pronounced for the 60% morph than the other stimuli, we used contrast coding comparing the average mouse curvatures for the 60% morph to the grand mean of all other face stimuli. This analysis revealed a significant difference between the 60% face and the grand mean of all the other morph stimuli [t(37) = 4.03, p < 0.001, d = 0.57], such that the 60% morph had a significantly higher AUC compared to the average AUC of all the other faces (AUC: M 60% face = 1.29 vs. M Grand = 0.45; see Figure 4). This suggests that the 60% morph was perceived as categorically more ambiguous and that it potentially triggered larger cognitive conflict compared to the other face stimuli.
Discussion
Experiment 1 aimed to use an established technique for measuring cognitive conflict processing, namely mouse tracking, to examine if categorically ambiguous faces induced cognitive conflict that is due to categorizing them as a “human” or “nonhuman” and if the faces that have been previously shown to be close to the category boundary (i.e., 60% humanness) induced the most cognitive conflict. As such, we expected to find that the 60% human face would exert the most cognitive conflict. Results of Experiment 1 showed that, indeed, the level of morphing had an overall effect on cognitive conflict and that the supposedly categorically ambiguous 60% morph induced significantly more cognitive conflict than all of other morphed images together (as measured in AUC) when subjects were categorizing the faces as a human or nonhuman.
Experiment 2
Experiment 2 aimed at examining whether the category boundary face (i.e., the face that exerted the most cognitive conflict in Experiment 1) has the ability to disrupt top-down modulation of attention orienting compared to faces that are more easily distinguishable as either a human or a nonhuman. If perceptual ambiguity drained cognitive resources, less cognitive resources would remain for an observer to exert top-down modulation of attentional orienting (i.e., attending to the predicted location as opposed to the cued location). As such, we would expect to find significant differences in gaze cueing for categorically ambiguous than non-ambiguous faces. Specifically, we expect the categorically ambiguous face (i.e., the 60% human face) to elicit stronger reflexive orienting of attentional resources (i.e., standard gaze cueing effect with shorter reaction times on valid trials) than categorically unambiguous faces as cognitive resources that would facilitate top-down modulation should be more depleted for categorically ambiguous face stimuli compared to unambiguous faces.
Materials and Methods
Participants
Thirty-seven undergraduate students from George Mason University were recruited to participate in the study (20 females, M age = 20.3, SD = 3.33, range = 18–37). All participants reported normal or corrected-to-normal vision and provided written consent as required and approved by the George Mason University Office Internal Review Board. Data from Experiment 2 were previously published (Martini et al., 2015) but was retreated, reanalyzed, and re-discussed from its original framework.
Stimuli
The stimuli used for Experiment 2 were identical to Experiment 1 with the addition of averted gaze stimuli. To create the averted gaze stimuli, we used Photoshop to shift irises and pupils of the original human and robot images by 0.4° from direct gaze. The target stimuli were black capital letters (F or T) presented at 0.5° high and 0.9° wide. Targets were presented on the same horizontal axis as the eyes of the respective stimulus and were located 14.7° to either the left or right from the center of the screen. All stimuli were 7.8° wide and 8.6° high and were depicted on a white background.
Task and Procedure
At the beginning of each session, participants were seated at a distance of 70 cm away from the computer monitor and were provided instructions to the gaze-cueing paradigm by a researcher. The instructions required them to fixate their gaze on a fixation cross in the middle of the screen and to respond to the identity of a target probe (“F” or “T”) as quickly and accurately as possible (i.e., discrimination task). Half of the participants were required to press the “K” key for the target letter “T” and the “D” key for the target letter “F”; key assignments for the targets were reversed for the other half of the participants.
Afterward, participants provided written consent and completed a practice block with 20 trials. The practice block included a mechanistic-looking robot as a gazer to insure that participants were not pre-exposed to the morphed stimuli prior to the experimental block. After the practice block, participants completed six experimental blocks – one block for each of the six morphed stimuli. Experimental blocks included 60 trials of counterpredictive cueing, in which the target appeared in the uncued location 80% of the time (i.e., 20% were valid and 80% were invalid trials). The order of the experimental blocks was randomized across participants to account for potential sequence effects associated with seeing the different morphed faces at different time points throughout the experiment. At the beginning of each experimental block, participants were presented with the stimulus and were asked to rate its mind status (i.e., Do you think this agent has a mind?) on an eight-point Likert scale ranging from 1 (“definitely not”) to 8 (“definitely yes”). Afterward, participants completed 60 trials of gaze cueing for this particular stimulus and then moved on to the next stimulus. This procedure was repeated until all blocks were completed.
The sequence of events on a given trial of gaze cueing is shown in Figure 5. At the beginning of each trial, a fixation cross was presented in the center of the screen and after a jittered interval of 700–1,000 ms, and one of the stimuli appeared in the center of the screen with the fixation cross still being visible. About 700–1,000 ms later, the face shifted its gaze direction to the right or left side of the screen, which constituted the gaze cue. After a SOA of 400–600 ms, the target letter (i.e., T or F) would appear on the screen, either in the cued location (i.e., valid trial; 20% of all trials) or in the opposite direction of the gaze cue (i.e., invalid trial; 80% of all trials). The target stayed on the screen until the participant responded by key press (“K” or “D” key) or a timeout criterion was reached after 1,200 ms, whichever came first. The time between the target appearing on the screen and the participant pressing the assigned key was recorded as reaction time for the analysis. The interval between trials (i.e., ITI) was set to 680 ms.
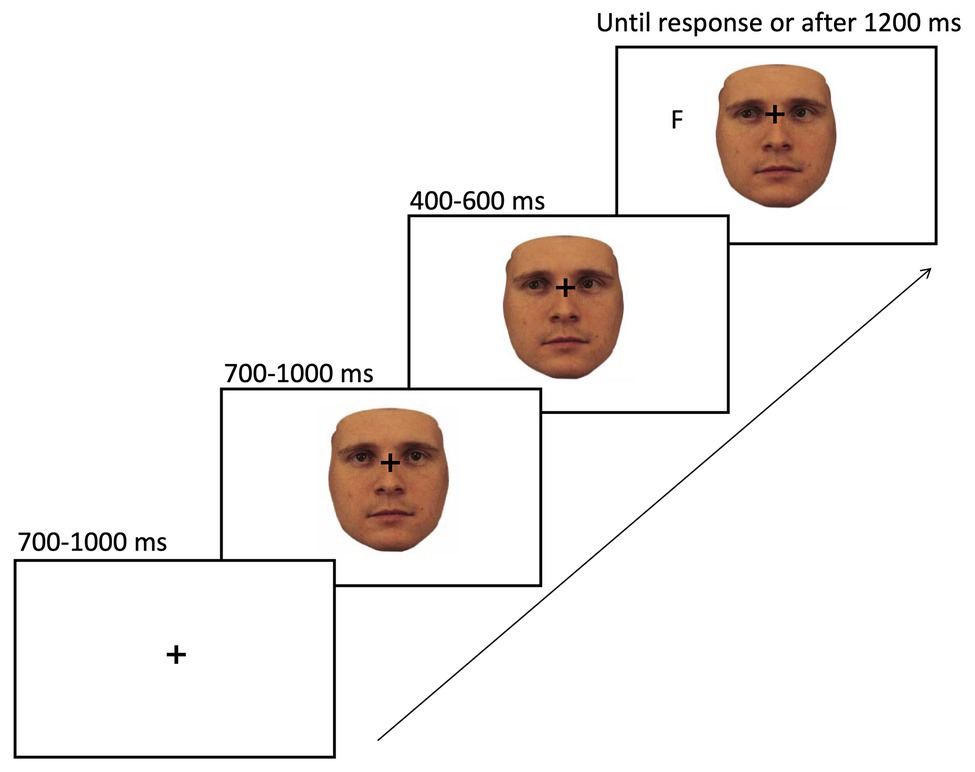
Figure 5. Sequence of events on a given trial of gaze cueing. The paradigm started with the presentation of a fixation cross in the center of the screen for 700–1,000 ms, followed by a face stimulus looking straight (here the 100% human face). About 700–1,000 ms later, the face would change its gaze direction to either the left or right side of the screen. After a stimulus onset asynchrony (SOA) of 400–600 ms, a target (F or T) would appear in either the same (i.e., valid trial) or opposite direction of the cue (i.e., invalid trial). The target letter remained on the screen until a response was given or a time out was reached, whichever came first.
Analysis
For subjective mind ratings, a six-level univariate within-subject ANOVA with Agent Type as a factor (i.e., 0% human vs. 20% human vs. 40% human vs. 60% human vs. 80% human vs. 100% human) was used to examine differences in ratings between the faces. Two post hoc t-tests were used to test if people subjectively perceived the 60% human face differently than the 80% human face and the 60% human face differently than the 40% human face.
To analyze the gaze cueing data, average RTs were computed for each valid and invalid trial per face, per participant. Only correct trials were used to compute RT averages on the single participant level. A 2 × 6 within-participant ANOVA with Validity (i.e., valid vs. invalid) and Agent Type (i.e., 0% human, 20% human, 40% human, 60% human, 80% human, and 100% human) as factors was used to examine the effect of physical human-likeness on attentional orienting to gaze cues. All post hoc t-tests were corrected using the FDR procedure.
Results
Two participants were removed from the analysis for having accuracy rates below 85%, resulting in a sample size of 35 participants. Results of the ANOVA analyzing subjective mind ratings violated Mauchly’s test for sphericity [χ 2(6) = 0.21, p < 0.001]. Greenhouse–Geisser corrections were used to account for the assumption violation (GG ε = 0.65). Corrected estimates revealed a significant effect of Agent Type [F(1,34) = 53.11, p < 0.001, ηG 2 = 0.39]. The main effect of mind ratings showed that mind ratings increased as faces increased in physical humanness; see Figure 6. Post hoc t-tests revealed that the 60% human face was significantly different from both the 40% human face [t(34) = 4.72, p < 0.001, d = 0.76] and the 80% human face [t(34) = −2.73, p < 0.01, d = −0.48].
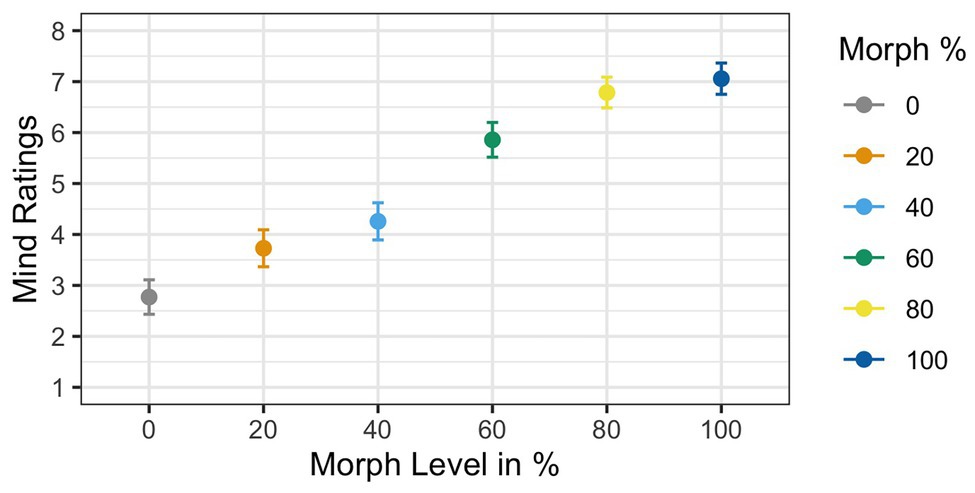
Figure 6. Mean mind ratings as a function of face morph. A main effect of agent type was found. Additionally, post hoc t-tests illustrated that the 60% human face was rated significantly different from both the 40% human and the 60% human faces.
Analysis of the RTs to targets in the gaze-cueing task showed that participants performed at a high level of accuracy as only 5% of the trials were rejected due to incorrect responses (M accuracy = 95%, SD = 0.03). Results of the 2 × 6 ANOVA revealed no significant main effect of Agent Type [F(1,34) = 0.32, p = 0.9, ηG 2 = 0.002] but a significant main effect of Validity [F(1,34) = 5.21, p = 0.02, ηG 2 = 0.001], with shorter reaction times on valid (M = 503 ms, SD = 91.1) than invalid trials (M = 510 ms, SD = 84.9), which indicated that attentional orienting followed a reflexive pattern on average; the Agent Type × Validity interaction [F(1,34) = 1.3, p = 0.26, ηG 2 = 0.002] was not significant. The gaze cueing effects as a function of physical human-likeness are shown in Figure 7.
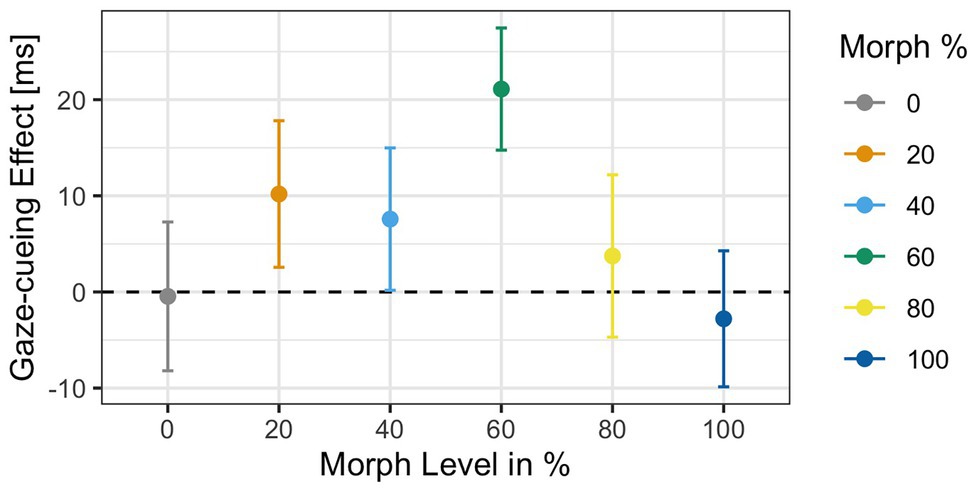
Figure 7. Reaction times as a function of physical human-likeness. Gaze cueing effects, which are calculated as the average reaction time differences between invalid and valid trials, are positive (indicative of reflexive attentional orienting to the cued location) for any of the “morphed” images but the “unmorphed” original 100% human and 100% robot faces, with the 60% morph showing the strongest “reflexive” cueing pattern. Together with the results of Experiment 1, these data indicate that there may be a direct link between the amount of cognitive conflict a face stimulus causes (and the amount of cognitive resources it drains) and the degree to which top-down control of attentional orienting to gaze cues is reduced.
Since we had the directed hypothesis that the categorically most ambiguous stimulus (i.e., 60% morph) would diminish top-down control more strongly than categorically less ambiguous stimuli, we first compared RTs on valid vs. invalid trials for each level of physical human-likeness separately and then compared the average gaze cueing effect in response to the categorically ambiguous 60% morph to those of the categorically unambiguous morphs (all other morphs). Paired t-tests for each morph level separately showed that the RT-difference between valid and invalid trials was only significant for the 60% human morph [t(34) = −2.82, p = 0.03, d = −0.22], with significantly shorter RTs on valid than invalid trials (M valid = 491 ms vs. M invalid = 512 ms), which indicated that attentional orienting to a categorically ambiguous gazer followed a reflexive pattern (i.e., standard gaze cueing effect); whereas, the other faces did not significantly induce a reflexive pattern (i.e., RT-difference between valid and invalid trials was not significant): 0% human [t(34) = 0.06, p = 0.95, d < 0.01; M valid = 509 ms vs. M invalid = 508 ms], 20% human [t(34) = −1.36, p = 0.51, d = −0.12; M valid = 505 ms vs. M invalid = 516 ms], 40% human [t(34) = −1.01, p = 0.62, d = −0.07; M valid = 500 ms vs. M invalid = 508 ms], 80% human [t(34) = −0.5, p = 0.84, d = −0.03; M valid = 509 ms vs. M invalid = 513 ms], and the 100% human [t(34) = 0.37, p = 0.84, d = 0.03; M valid = 506 ms vs. M invalid = 503 ms]. Furthermore, contrast coding showed that the gaze cueing effect for the 60% human morph differed significantly from the gaze cueing effects for all other faces combined [t(34) = 2.11, p = 0.03, d = 0.42], with larger standard/reflexive gaze cueing effects for the 60% morph than all other morphs combined [gaze-cueing effect (GCE): M 60% face = 21.1 ms vs. M Grand = 3.65 ms].
Exploratory Analysis
Although the results indicated that the 60% human morph induced a stronger cognitive conflict (Experiment 1), as well as stronger reflexive attentional orienting than any of the other morphed images (Experiment 2), the current analyses do not directly link cognitive conflict processing as measured via mouse tracking to the amount of reflexive attentional orienting to gaze cues. Since two experiments contained independent samples, a traditional correlation analysis linking gaze cueing to mouse curvatures is not possible. To address this issue, we calculated average gaze cueing effects (i.e., RTs on invalid trials − RTs on valid trials) and average AUC values for each morphed face stimulus across participants and calculated the correlations at the morph level. In doing so, the sample of interest is at the morph level (i.e., each morphed face now has one gaze-cueing effect and one AUC value) and not the participant level, which allows us to directly compare the two samples. The Spearman correlation analysis showed a significant correlation of 0.81 between AUC and GCE [r(4) = 0.81, p = 0.04], indicating that cognitive conflict measures, such as mouse tracking, are related to top-down control of social attention.
While the correlation was positive and significant, a traditional parametric correlation would not yield stable estimates if the sample size is not large enough. In other words, with a sample size of 6, parametric analyses do not yield reliable estimates due to violations of assumptions regarding normality. To alleviate this issue, we adopted a Bayesian approach as estimate interpretation is viable with a small sample when using Bayesian methods (e.g., McNeish, 2016). A Bayesian analysis would allow us to determine the strength of the evidence in support of our hypothesis that stronger cognitive conflict processing for a given stimulus would lead to stronger bottom-up processing of social attention (i.e., positive gaze cueing effects), as opposed to parametric analyses which determine, in a binary fashion, if we can or cannot reject the null hypothesis (i.e., does a relationship between cognitive conflict and gaze cueing effects exist?). If attentional orienting to gaze cues was, indeed, impacted by the amount of cognitive conflict associated with categorically ambiguous face stimuli, we would expect to find a positive relationship between gaze cueing effects and AUC (i.e., the higher the cognitive conflict triggered by a face stimulus, the higher the AUC, the more reflexive attentional orienting should be, which would be reflected in shorter RTs on valid than invalid trials).
Before estimating the Bayesian correlation, we need to use a sampling procedure that allows us to draw estimates and their probabilities from a distribution. We used 5,000 iterations of the Markov chain Monte Carlo (MCMC) sampling procedure to determine the estimate of the correlation. Additionally, we used a uniform prior distribution to draw our estimates from. This allows us to test the strength of the relationship between gaze cueing effects and AUC. Results of the Bayesian correlation showed that a positive relationship between GCE and AUC [r = 0.68, 90% CI (0.13, 0.99)] with a 93% probability that the true correlation estimate is greater than zero and positive, which indicated that the relationship is likely. This suggests that there is enough evidence to confidently conclude a positive relationship between GCE and AUC; see Figure 8.
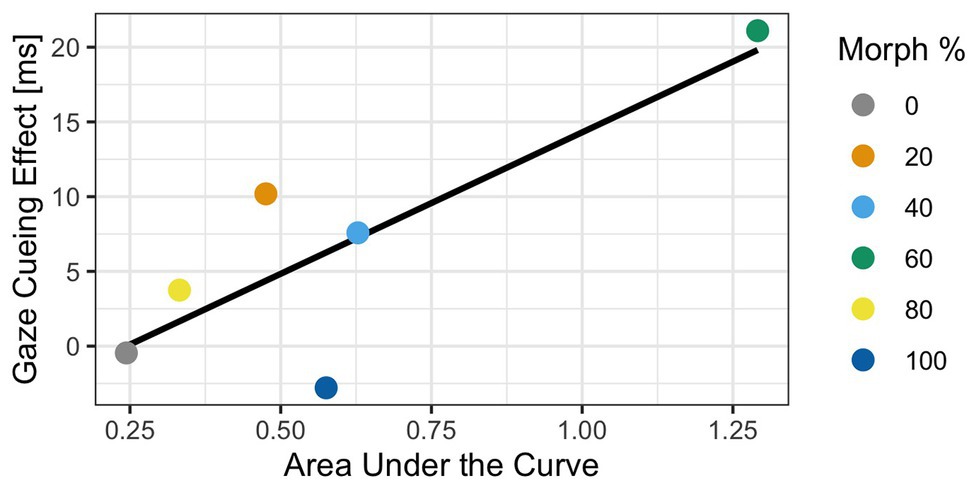
Figure 8. Results of the Bayesian analysis exploring the relationship between gaze-cueing effect (GCE) and AUC. The graph illustrates the positive relationship between GCE in Experiment 2 and AUC in Experiment 1 for each of the morphed stimuli. Specifically, as the AUC becomes larger, the GCE becomes larger too, indicating that the amount of cognitive conflict a face induces is correlated to the “reflexiveness” of gaze cueing. The blue line illustrates the line of best fit.
Discussion
After identifying which face exerted the most cognitive conflict in Experiment 1, Experiment 2 aimed to examine whether the same categorically ambiguous face (i.e., 60% human face) would negatively impact top-down control of attentional orienting to gaze signals compared to unambiguous stimuli due to drainage of cognitive resources. Using a counterpredictive gaze-cueing paradigm allowed us to determine whether attentional orienting was volitionally shifted to the uncued but likely target location (i.e., faster reaction times on invalid vs. valid trials) or whether it was reflexively shifted to the cued but unlikely target location (i.e., faster reaction times on valid vs. invalid trials). We expected to find that the 60% human face would be subjectively different than the faces that are adjacent to it (i.e., the 40% human face and the 80% human face) and if it would be associated with stronger reflexive processing as shown by faster reaction times to the cued but unlikely direction for the category boundary face compared to the non-category boundary faces.
The results of the subjective ratings show that, as expected, the 60% human face was categorically different than the 40% human face (i.e., nonhuman) and the 80% human face, indicating that participants subjectively perceived the ambiguous face differently than the other faces. Additionally, the results show that variation of gaze cueing effects (i.e., RTs on invalid trials − RTs on valid trials) as a function of physical human-likeness follows an inverted u-shaped pattern, with negative or reversed gaze cueing effects for the “unmorphed” images, namely the 100% robot and the 100% human face. All “morphed” images (20–80% human morph) showed positive or standard gaze cueing effects to some extent, indicating that they reflexively oriented attention to some degree. Inline with our main hypothesis, the categorically ambiguous 60% human morph induced gaze cueing effects that are significantly more positive/reflexive than the other, categorically unambiguous, morphs combined. These results show that although the 60% morph (i.e., the categorically most ambiguous stimulus) impacted top-down control most negatively, it is possible that the other morphed stimuli created a certain level of categorical ambiguity that affected top-down control mechanisms to varying degrees, as determined by the positive relationship between gaze cueing effects and cognitive conflict that is due to categorization.
Experiment 3
Experiments 1 and 2 illustrated that categorically ambiguous faces can induce cognitive conflict that may be due to increased cognitive costs invested in resolving perceptual conflict between two competing categories (“human” vs. “nonhuman”), and this influences observers’ ability to top-down control attentional orienting. However, based on their design, the experiments conducted so far are only able to show a correlational relationship between cognitive conflict associated with categorical ambiguity and a reduction in top-down control of attentional orienting. In order to show that cognitive conflict is causal to a modulation of top-down control, the amount of cognitive conflict a stimulus induces would have to be manipulated experimentally. To address this, we experimentally reduced the extent to which categorically ambiguous faces induced cognitive conflict by pre-exposing participants to the faces prior to the gaze-cueing task in Experiment 3. We, then, compared the size of the gaze cueing effects that they trigger from Experiment 3 to gaze cueing effects measured in Experiment 2. The assumption that pre-exposure would alleviate categorical ambiguity was based on previous reports that conflict processing on one trial leads to transient upregulation of selective attention on the next trial, which biases perception toward one particular interpretation and reduces cognitive conflict even without explicit instructions to pay attention to category-defining features (conflict adaptation; e.g., Botvinick et al., 2004; Egner and Hirsch, 2005). The effectiveness of perceptual pre-exposure to reduce categorical ambiguity and the associated drainage of cognitive resources has also been validated empirically by Wiese et al. (2019). Exposing participants to the stimuli during a perceptual task before the gaze-cueing task should speed up conflict adaptation by (a) explicitly paying attention to the perceptual features of the stimuli and (b) resolving the conflict without being forced to concurrently perform another task that requires resources. If pre-exposure resolved categorical ambiguity with the 60% morph, competing activation of the human and nonhuman categories should be reduced and free up resources for top-down control. Additionally, we were interested to see how the category boundary would be affected when people are pre-exposed to the faces.
Materials and Methods
Participants
Thirty-four undergraduate students from George Mason University (24 females, M age = 22.12, SD = 5.10, range = 18–37) were recruited for the study. All participants reported normal or corrected-to-normal vision and provided written consent prior to participating. All research procedures were approved by George Mason University’s Internal Review Board.
Stimuli, Task, and Procedure
Stimuli used in Experiment 3 were identical to Experiments 1 and 2. The task and procedure were the same as Experiment 2 with one exception: before completing six blocks of gaze cueing with the six different face stimuli, participants completed a pre-exposure task during which they were asked to rate each face stimulus on an eight-point Likert scale (1 being “Definitely Not” and 8 being “Definitely Yes”) regarding five questions related to mind perception: “Please rate how much this face has a mind?,” “Please rate how much this face looks alive?,” “Do you think this agent would feel pain if it tripped and fell on hard ground?,” “Do you think this agent like to hang out with friends?,” and “Do you think this agent is an interesting conversationalist?” (Martini et al., 2016). These questions were chosen since they have been shown to significantly reduce cognitive conflict for categorically ambiguous face stimuli during pre-exposure (Wiese et al., 2019). All faces and questions were presented in a randomized fashion during pre-exposure. After having rated all face stimuli, participants completed an identical gaze-cueing paradigm to Experiment 1. An example trial from the pre-exposure is shown in Figure 9.
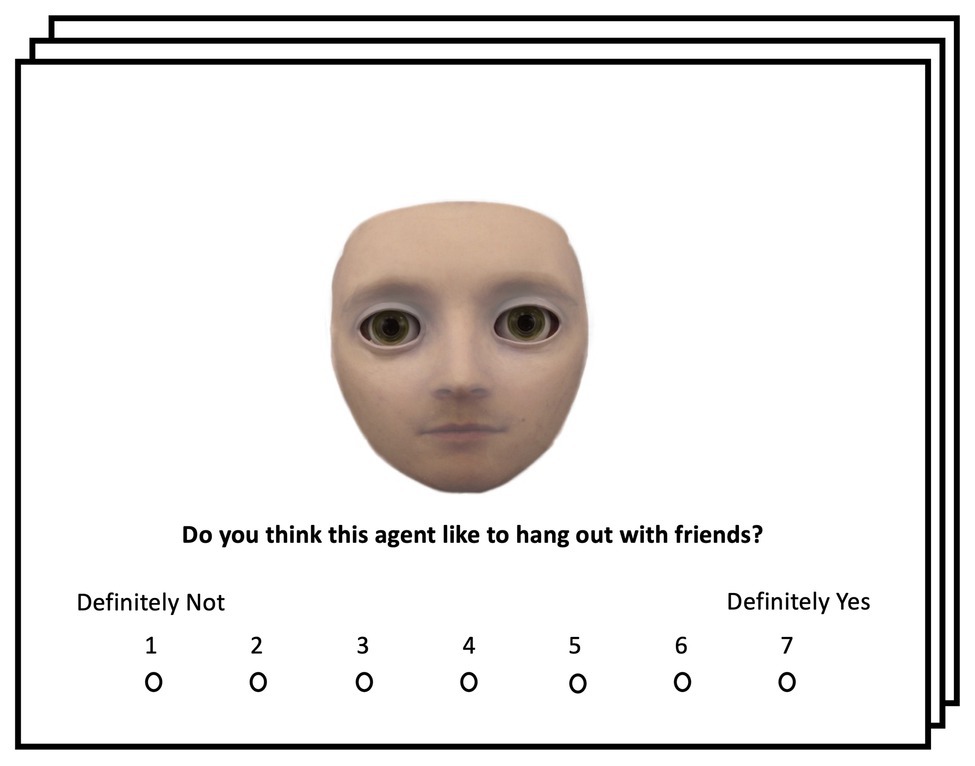
Figure 9. Example of a pre-exposure trial. Face stimuli were presented in random order and participants were instructed to rate the presented face regarding different mental capacities related to mind perception; see Wiese et al. (2019) for more details regarding the pre-exposure manipulation.
Analysis
Analyses in Experiment 3 were identical to Experiment 2, with one exception: we conducted an additional 2 × 2 mixed ANOVA for only the 60% human morph between participants in Experiment 2 and participants in Experiment 3 with Validity (valid vs. invalid) as within-factor, Exposure (yes vs. no) as a between-factor, and RTs as dependent variables to examine whether pre-exposure reduced the “reflexiveness” of attentional orienting for the 60%. We ran this additional ANOVA as we expected that top-down and bottom-up processes would cancel each other out after pre-exposure. If these two components do cancel each other out, it would lead to null results in the main 2 × 6 ANOVA, which can be challenging to interpret, therefore, the second ANOVA compared the 60% human face from Experiments 2 and 3. All post hoc t-tests were corrected using the (FDR) procedure.
Results
Two participants were excluded due to low accuracy rates (i.e., below 85%). Two additional participants had to be excluded from the mind rating analysis only, since they did not provide ratings for all six face stimuli; they were not excluded from the analysis of the gaze cueing data. Results of the ANOVA violated Mauchly’s test for sphericity [χ 2(6) = 0.29, p = 0.002]. Greenhouse–Geisser corrections were used to account for the assumption violation (GG ε = 0.73). Similar to Experiment 2, corrected estimates of the analysis of the subjective mind ratings showed a significant main effect of Agent Type [F(1,29) = 67.56, p < 0.001, ηG 2 = 0.54], such that mean ratings increased as faces increased in physical humanness; see Figure 10. Post hoc t-tests showed that the 60% human face was significantly different than the 80% human face [t(29) = −7.6, p < 0.001, d = −1.41], but not the 40% human face [t(29) = 1.41, p = 0.15, d = 0.27]. We also tested if the average ratings for the 60% face before exposure were significantly different than the 60% face after exposure using a t-test. The independent samples t-test showed a significant reduction in ratings when participants were not pre-exposed (Experiment 1; M = 5.85) compared to when they were pre-exposed [Experiment 2; M = 3.56; t(64) = 4.75, p < 0.001, d = 1.16].
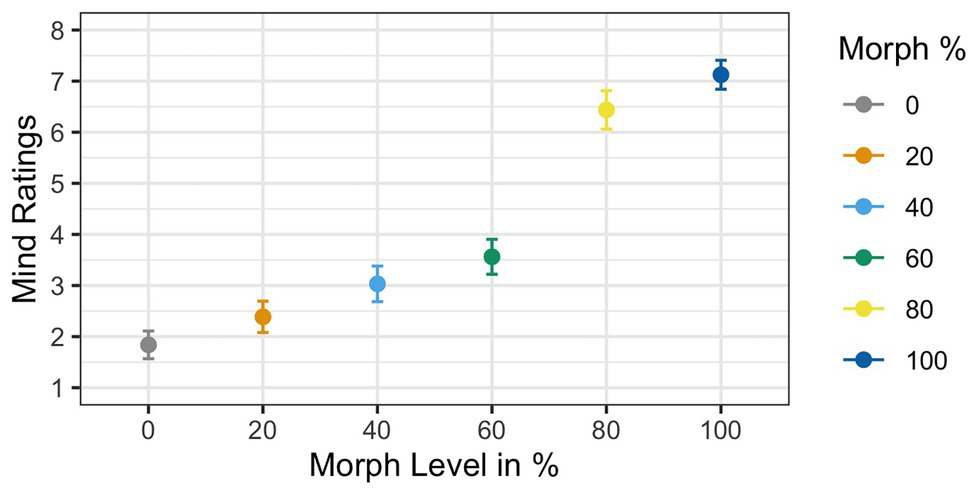
Figure 10. Mind ratings as a function of physical human-likeness. Analyses of the subjective mind ratings showed a main effect of Agent Type. Follow up tests showed that the 60% morph was rated significantly different than the 40% morph but not the 80% morph.
Similar to Experiment 2, accuracy rates in the gaze-cueing task were high, and only 5% were rejected due to incorrect responses (M accuracy = 95%, SD = 0.03). The results of the 2 × 6 ANOVA data showed no main effects of Validity [F(1,31) = 0.07, p = 0.78, ηG 2 < 0.001] or Agent Type [F(1,31) = 0.68, p = 0.63, ηG 2 = 0.002], and no Validity × Agent Type interaction [F(1,31) = 0.33, p = 0.89, ηG 2 < 0.001]. Similar to Experiment 1, we used contrast coding on gaze cueing effects to examine differences between the 60% morph and the grand average of all the faces. The post hoc test showed no significant difference between gaze cueing effects elicited by 60% morph and the grand average gaze cueing effect of all the faces [t(31) = −0.65, p = 0.51, d = −0.12; M 60% face = −3.5 ms vs. M Grand = 1.86 ms]; see Figure 11.
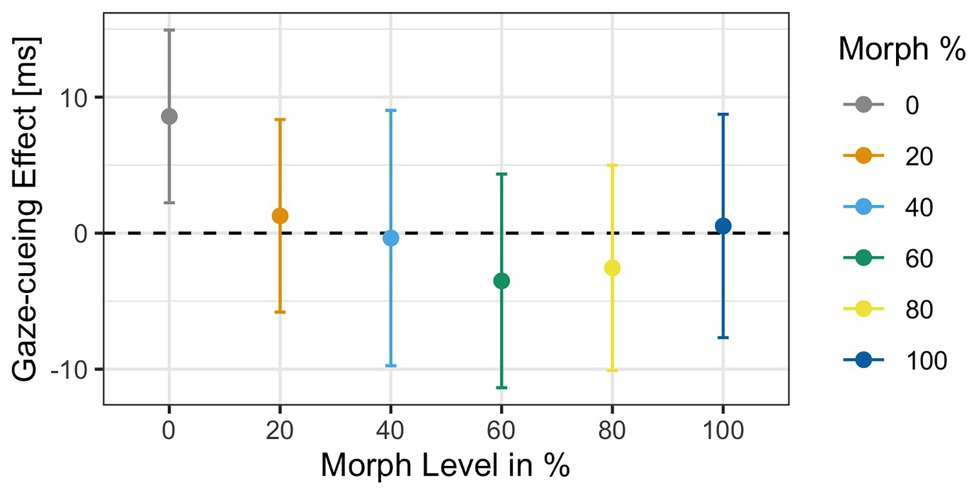
Figure 11. Gaze-cueing effect as a function of physical human-likeness. Analyses of the gaze cueing effect (difference in RT between valid and invalid trials) showed no differences in RT for any of the faces, validity, or interaction.
The results of the previous ANOVA revealed no main or interaction effects, which suggests that for all face stimuli, and especially the 60% human stimulus, top-down and bottom-up processing worked in tandem and, therefore, canceled each other out. However, since interpreting null results can be problematic, a more active method of analysis is warranted. To do this, we compared the 60% human face from the no pre-exposure experiment to the 60% human face to test if pre-exposure to ambiguous faces does indeed increase top-down processing. Results of the 2 (Validity: valid vs. invalid) × 2 (Exposure: yes vs. no) ANOVA on RTs from the gaze-cueing task showed a trending effect of Validity [F(1,65) = 3.08, p = 0.08, ηG 2 = 0.002], or Exposure [F(1,65) = 0.02, p = 0.89, ηG 2 < 0.001], but a significant Validity × Exposure interaction [F(1,65) = 6.03, p = 0.01, ηG 2 = 0.004], such that participants who were not pre-exposed to the 60% human morph showed a significant standard gaze cueing effect [i.e., significantly faster RTs on valid than invalid trials: M valid = 491 ms vs. M invalid = 512 ms; t(35) = −3.04, p < 0.01, d = −0.22], whereas participants who were pre-exposed to the faces prior to the gaze-cueing task did not show a significant standard gaze cueing effect [M valid = 500 ms vs. M invalid = 496 ms, t(31) = 0.48, p = 0.62, d = 0.03], indicating that reflexive orienting toward the cued location was significantly more under top-down control; see Figure 12.
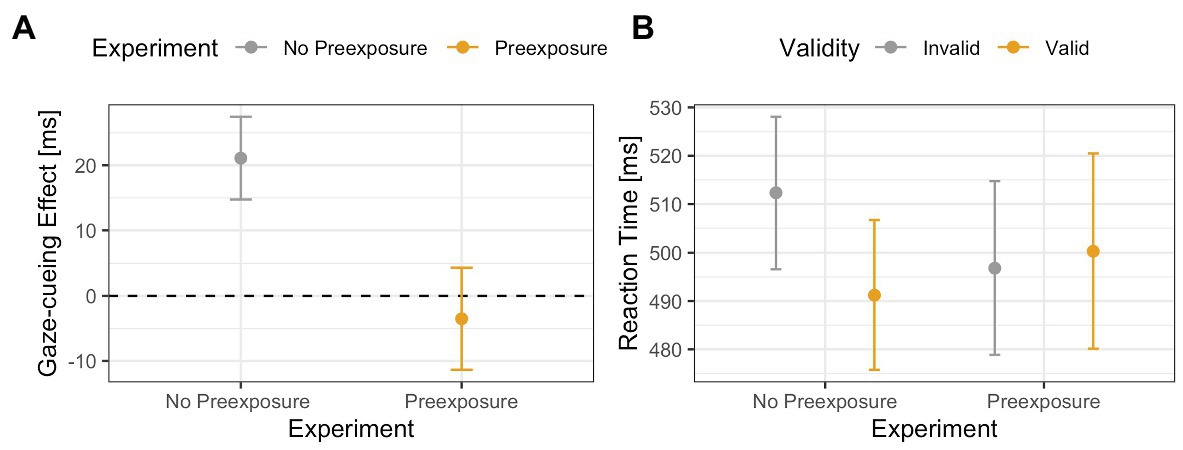
Figure 12. Comparison of the 60% human face in the Experiment 2 (no pre-exposure) and Experiment 3 (pre-exposure). The results show an interaction effect between exposure and validity, such that a validity effect (in this case faster reaction times toward targets are the cued location) was only present when participants were not pre-exposed to the faces. Panel (A) shows differences between the gaze-cueing effects of the two experiments (i.e., invalid-valid trials). Panel (B) shows the raw reaction times between valid and invalid trials.
Discussion
The third experiment aimed to investigate whether seeing categorically ambiguous gazing stimuli is causally related to participants’ ability to top-down control attentional orienting to gaze cues in a counterpredictive gaze-cueing task. To modulate the categorical ambiguity associated with morphed face stimuli, we pre-exposed participants to the stimuli prior to the gaze-cueing task and asked questions that required participants to process the perceptual features of the faces in more detail, which has been shown to reduce categorical ambiguity and drainage of cognitive resources in previous experiments (Wiese et al., 2019). If indeed categorical ambiguity was the cause for the modulation of gaze cueing effects observed in Experiment 2, pre-exposure would be expected to alleviate this modulatory effect and restore participants’ ability to top-down control attentional orienting in a counterpredictive cueing paradigm.
As expected, the results illustrated that pre-exposing participants to the morphed stimuli restored the top-down control of attention shifting as pre-exposure reduced the reflexiveness of the attention shift for the 60% morphed image compared to the 60% morphed image from Experiment 1. Specifically, while there was a significant standard gaze cueing effect for the 60% morph in Experiment 2, such that participants were faster when reacting to targets presented at the cued vs. the likely target location, there was no significant standard gaze cueing effect for the 60% morph in Experiment 3.
General Discussion
The current study investigated the effects of categorical ambiguity on top-down control of social attention. We hypothesized that categorically ambiguous human faces would induce cognitive conflict that is due to categorizing the faces as “human” or a “nonhuman” (Experiment 1), which negatively impacts top-down modulation of attention orienting, possibly due to drainage of cognitive resources (Experiment 2), and that pre-exposure to ambiguous stimuli embedded in a perceptual task should reduce categorical ambiguity and, in turn, positively impact top-down control of social attention (Experiment 3). The results show that, indeed, categorical ambiguity induces cognitive conflict (Experiment 1) and that the degree to which attentional orienting to gaze cues can be top-down controlled varies as a function of categorical ambiguity displayed by a gazing face, which is positively related to cognitive conflict processing (Experiment 2). Finally, by pre-exposing participants to the gazing stimuli, we were able to alleviate categorical ambiguity, which partially recovers top-down control abilities (Experiment 3).
After employing mouse tracking methods to find that the 60% human face induced cognitive conflict that is due to categorizing it as a “human” or a “nonhuman,” we used a counterpredictive gaze-cueing paradigm to show that the average differences between valid and invalid trials in the 60% human face were significantly larger compared to all the other faces combined. This illustrates how top-down modulation was least exerted for the face stimulus that was closest to the category boundary. An exploratory correlational analysis provided support for the hypothesis that cognitive conflict and gaze cueing effects (i.e., the RT differences between valid and invalid conditions) are positively correlated, such that as cognitive conflict increased (i.e., larger AUCs in mouse tracking), gaze cueing effects became more reflexive/positive. In Experiment 3, we used a pre-exposure intervention to reduce cognitive conflict related to categorical ambiguity. The results indicate that after pre-exposure, there were no longer any significant differences in gaze cueing effects between the different face stimuli as the reflexiveness of gaze cueing for the 60% morphed stimulus was significantly reduced.
The findings of these experiments are partially in line with theoretical assumptions about the nature of the UV. While the UV was originally only a theoretical construct, recent studies provide empirical evidence for its existence and shed light on its potential underlying mechanisms (Kätsyri et al., 2015; MacDorman and Chattopadhyay, 2016; Mathur and Reichling, 2016; Wiese and Weis, 2020). Two theories receiving strong empirical support are the categorical perception hypothesis (CPH) and the perceptual mismatch hypothesis (PMH): the former purports that the appearance of humanoid agents triggers a cognitive conflict when it is ambiguous whether they represent human or nonhuman entities and that conflict resolution requires cognitive resources which leads to negative emotional evaluations (Cheetham et al., 2011); the latter assumes that negative affinity associated with uncanny stimuli is caused by inconsistencies between the human-likeness levels of specific sensory signals contained in nonhuman faces, such as grossly enlarged eyes displayed on an otherwise perfectly human face (MacDorman et al., 2009). In line with the CPH, it has been demonstrated that ratings of human-likeness of morphed stimuli follow a non-linear pattern with significant changes in ratings around the category boundary (Looser and Wheatley, 2010; Cheetham et al., 2011, 2014; Yamada et al., 2013), together with increased categorization times (Cheetham et al., 2014) and negative stimulus evaluations in some studies (Burleigh et al., 2013; Yamada et al., 2013; Ferrey et al., 2015) but not in others (Looser and Wheatley, 2010; Cheetham et al., 2014; MacDorman and Chattopadhyay, 2016)2.
The finding that top-down control of attention orienting was negatively impacted in the first experiment for only the 60% human face can be explained in terms of the CPH. As the CPH states that categorical ambiguity reaches its peak at the category boundary, subjects would recruit more cognitive resources to categorize the face as a human or nonhuman. Therefore, less cognitive resources would be available to volitionally attend to the likely but uncued location, and we expect – and find – that the largest reduction in top-down control would be for the face at the category boundary. On the other hand, on a descriptive level, the data show that all face stimuli show some extent of reduction of top-down modulation of attentional orienting [i.e., gaze cueing effects are positive for all “morphed” stimuli (i.e., 20–80% human), but negative for the “unmorphed” stimuli (0% human and 100% human)], which would be more in line with the PMH. In other words, cognitive conflict could be induced by all the morphed images since all of them show some extent of disproportionality of physical features.
Regardless of whether cognitive conflict was caused by categorical ambiguity at a global or local feature level, the observation that cognitive conflict processing negatively impacts resource demanding processes like top-down control of attention is in line with previous studies showing that humans employ cognitive control during gaze interactions to reduce interference that is introduced by task irrelevant factors and when cognitive resources are recruited to exert control, the reflexiveness of our gaze shifts is increased in response to gaze cues. For example, when cognitive control is exerted to reduce the interference from processing emotional faces (Pecchinenda and Petrucci, 2016) or executing a cognitively demanding task (Bobak and Langton, 2015), attention shifting in response to gaze-cues is more reflexive even though top-down modulation should have reduced the reflexivity of attention shifting due to the gaze shifts being non-predictive of the target location (Bobak and Langton, 2015; Pecchinenda and Petrucci, 2016). Interestingly, this effect is also evident in other social behaviors such as head orientations and not simply gaze (Visser and Roberts, 2018). In line with the interpretation of having less cognitive resources to exert cognitive control, we show a positive correlation between gaze cueing effects (i.e., the reflexiveness of gaze) and cognitive conflict, which uses up cognitive resources (Desimone and Duncan, 1995).
Other paradigms have experienced similar effects of the negative impact of cognitive conflict from categorizing ambiguous faces on an unrelated task, which supports our interpretations. For example, in a vigilance task that measures drainage of cognitive resources, Wiese et al. (2019) found that embedding categorically ambiguous faces in the vigilance task caused cognitive resources to be drained at a faster rate compared to non-ambigious faces. More importantly, they found that pre-exposure frees up cognitive resources, showing that pre-exposure leads categorically ambiguous faces to drain less cognitive resources over time in a vigilance task (Wiese et al., 2019). The effect that categorical ambiguity influences cognitive resources is similar to the observed data that suggest that the amount of cognitive conflict that a face exerts is correlated with the reflexivity of attention orienting. This account is corroborated by both face perception literature showing that face perception is a reflexive process that does not need explicit instructions to be processed (for a review, see Palermo and Rhodes, 2007) and evidence showing that mind perception is an automatic processes that is driven by perceptual features of stimuli (Gao et al., 2010; Looser and Wheatley, 2010).
In support of our interpretation that pre-exposure allowed for cognitive conflict to be reduced, prior research has shown that merely processing stimuli in terms of their physical perceptual properties can reduce cognitive conflict (Bornstein and D’agostino, 1992; Lee, 2001) and that looking at novel stimuli for longer durations is enough to allow for more perceptual features to be processed, which results in the coactivation of multiple categories to be reduced (Li et al., 1993; Desimone and Duncan, 1995) and cognitive resources to be freed up to exert cognitive control (Desimone and Duncan, 1995; Ferrey et al., 2015). In other words, once the novelty of seeing the categorically ambiguous face is reduced due to increased exposure time, drainage of cognitive resources due to competition of categorical representations is reduced as well and more resources are available to be allocated for top-down control. This interpretation is further supported by neural data suggesting that less neurons in the inferior temporal (IT) cortex are activated as stimuli become more familiar to Rhesus monkeys when completing a delayed matching to sample (DMS) task (Li et al., 1993), which can explain why seeing an ambiguous and novel face can recruit more resources to compete for visual processing of information.
The findings of this study have several implications. First, not only did we show that cognitive conflict can reduce our ability to top-down modulate attention orienting, we also showed that resolving the conflict frees up more resources to exert cognitive control and attend to the likely location for better task performance. Although this contrasts studies that showed no effect on the modulation of gaze cueing effects when cognitive load is increased due to a secondary task (Law et al., 2010; Hayward and Ristic, 2013), it supports classical interpretations of the reflexivity of the gaze cueing effect (Friesen and Kingstone, 1998). However, it is important to note that it is possible that these gaze-cueing studies did not warrant top-down control in their design as their manipulations were considered not taxing enough to use many cognitive resources, which means that there were enough resources to be allocated for top-down modulation (Bobak and Langton, 2015). On the other hand, other studies have shown that when the gaze-cueing paradigm is in a context where a task-irrelevant influencer can affect gaze, cognitive control is warranted to inhibit the effect of the exogenous influencers, which limits the resources that allow for cognitive control to inhibit the influence of the task-irrelevant distractor (Bobak and Langton, 2015; Pecchinenda and Petrucci, 2016; Visser and Roberts, 2018). Our data are best interpreted along the latter interpretation as face processing is automatic (Palermo and Rhodes, 2007). In other words, when seeing a perceptually ambiguous face, it can serve as a distractor that limits cognitive resources from exerting top-down processing, which supports accounts that suggest continuous interactions between top-down and bottom-up processes, as opposed to classical interpretations of the gaze cueing effect (see Capozzi and Ristic, 2020, for a review on the debate between bottom-up and top-down processes of gaze-cueing
Another implication is that mind perception is an effective modulator of gaze cueing (in line with Teufel et al., 2010; Wiese et al., 2012, 2018, 2019; Morgan et al., 2018), but that the impact of mind perception in gaze cueing depends on how mind perception is manipulated. For example, studies using belief manipulations show a positive effect on attention orienting as belief can be very effective in enhancing or decreasing social relevance (Müller et al., 2011, 2014; Wykowska et al., 2014; Caruana et al., 2015, 2017a; Fini et al., 2015; Gobel et al., 2017) and that these effects persist at a short SOA of 250 ms, which suggests that the social relevance of the gazer is determined fairly quickly (Wiese et al., 2012). However, using physical appearance as a mind perception manipulation does not have a straightforward effect on performance as (1) physical appearance and mind perception have a nonlinear relationship (i.e., UV effect) and (2) people need to first process and categorize a face before the social relevance of the face can have an effect. This observation has been shown in gaze-cueing studies that illustrate the need for longer SOAs in order for top-down modulation of facial expressions to take place (Graham et al., 2010).
We also acknowledge limitations that can be addressed in future studies. While morphed stimuli can provide an advantage in experimental control, there seems to be growing criticism in using morphed stimuli in HRI (Mathur and Reichling, 2016). While previous morph studies have generalized to real-life interactions (Kompatsiari et al., 2018), it is important to acknowledge that this may not be the case with all paradigms. Therefore, future studies should examine real robot face stimuli that vary in physical human likeness (see the ABOT database for robot faces; Phillips et al., 2018). Another possible limitation is the independence of samples across participants. While the differences between the experiments can be more informative if the same samples were presented with all three studies, it would have been difficult to tease apart the influence of the pre-exposure treatment from the duration of the experiment itself. In other words, if participants went through all three experiments, they could have been pre-exposed to the faces by simply partaking in a long study. Finally, some of our analyses employ statistical procedures that require larger sample sizes than is feasible for experimental studies. While we tried to alleviate these issues, future studies should investigate these effects using large enough samples.
While these experiments link cognitive conflict and top-down processing in a gaze-cueing study behaviorally, future studies should investigate the neurophysiological origins of this effect to disentangle top-down and bottom-up processing of gaze-cueing. For example, while Wiese et al. (2018) have shown that the ventromedial prefrontal coretex (vmPFC) is correlated with more top-down processing in a gaze-cueing study, it is unclear if variations in activation of the vmPFC is related to this cognitive conflict as other studies have suggested that the anterior cingulate cortex (ACC) is related to monitoring conflict in the brain (Botvinick et al., 2004; Umemoto et al., 2018). Future studies should also investigate if cognitive conflict varies as a function of self-other representations in the task as prior work has suggested that gaze-cueing comprises of two different processes in the brain (i.e., self-representations and other-representations; Schilbach et al., 2013). These representations allow us to either respond or engage in joint attention, which the classic gaze-cueing study does not differentiate (Caruana et al., 2017b). These representations allow us to either respond to or engage in joint attention, but as of now, it is unclear if cognitive conflict that is due to seeing ambiguous faces affects both representations.
These findings provide important considerations for the field of social robotics. For example, we illustrate the importance of understanding how cognitive processing is affected in the context of robots that try to evoke social responses from humans as we show that physical human-likeness may not be the best design choice for resource-demanding tasks executed with or in the presence of robots, since categorical ambiguity seems to be processed without being task relevant and, thus, takes away resources from the main task. Additionally, we provide a possible solution to the UV phenomenon by showing that exposure can be used as an intervention to alleviate symptoms that are due to design drawbacks.
Data Availability Statement
The datasets presented in this study can be found in online repositories. The names of the repository/repositories and accession number(s) can be found here: https://osf.io/73pr6/.
Ethics Statement
The studies involving human participants were reviewed and approved by Internal Review Board of George Mason University. The patients/participants provided their written informed consent to participate in this study.
Author Contributions
EW and AM conceptualized the study. AM collected the data. AA analyzed the data. AA, EW, and AM interpreted the results and wrote the manuscript. All authors contributed to the article and approved the submitted version.
Conflict of Interest
The authors declare that the research was conducted in the absence of any commercial or financial relationships that could be construed as a potential conflict of interest.
Footnotes
1. Since we created a morphing spectrum that increased in humanness from a 0% human to a 100% human in steps of 20%, the closest morph to the category boundary is the 60% human. While the 80% human is also close to the boundary, it is also much closer to the 100% human category, which could reduce any coactivation of the human and nonhuman categories.
2. Studies examining the PMH suggest that the most negative affective evaluations are elicited by images where the mismatch between a subset of realistic (e.g., human face shape) and a subset of unrealistic image features (e.g., enlarged eyes) is maximal (Seyama and Nagayama, 2007; MacDorman et al., 2009; Mitchell et al., 2011; Mäkäräinen et al., 2014) and that maximal negative affinity does not coincide with maximal categorical uncertainty (MacDorman and Chattopadhyay, 2016).
References
Abubshait, A., Weis, P. P., and Wiese, E. (2020). Does context matter? Effects of robot appearance and reliability on social attention differs based on lifelikeness of gaze task. Int. J. Soc. Robot. [Preprint]. doi: 10.1007/s12369-020-00675-4
Abubshait, A., and Wiese, E. (2017). You look human, but act like a machine: agent appearance and behavior modulate different aspects of human-robot interaction. Front. Psychol. 8:1393. doi: 10.3389/fpsyg.2017.01393
Admoni, H., Bank, C., Tan, J., Toneva, M., and Scassellati, B. (2011). “Robot gaze does not reflexively cue human attention” in Proceedings of the 33rd Annual Conference of the Cognitive Science Society 1983–1988; July 2011.
Adolphs, R. (1999). Social cognition and the human brain. Trends Cogn. Sci. 3, 469–479. doi: 10.1016/S1364-6613(99)01399-6
Balas, B., and Tonsager, C. (2014). Face animacy is not all in the eyes: evidence from contrast chimeras. Perception 43, 355–367. doi: 10.1068/p7696
Bobak, A. K., and Langton, S. R. H. (2015). Working memory load disrupts gaze-cued orienting of attention. Front. Psychol. 6:1258. doi: 10.3389/fpsyg.2015.01258
Bonifacci, P., Ricciardelli, P., Lugli, L., and Pellicano, A. (2008). Emotional attention: effects of emotion and gaze direction on overt orienting of visual attention. Cogn. Process. 9, 127–135. doi: 10.1007/s10339-007-0198-3
Bornstein, R. F., and D’agostino, P. R. (1992). Stimulus recognition and the mere exposure effect. J. Pers. Soc. Psychol. 63, 545–552. doi: 10.1037/0022-3514.63.4.545
Botvinick, M. M., Cohen, J. D., and Carter, C. S. (2004). Conflict monitoring and anterior cingulate cortex: an update. Trends Cogn. Sci. 8, 539–546. doi: 10.1016/j.tics.2004.10.003
Burleigh, T. J., Schoenherr, J. R., and Lacroix, G. L. (2013). Does the uncanny valley exist? An empirical test of the relationship between eeriness and the human likeness of digitally created faces. Comput. Hum. Behav. 29, 759–771. doi: 10.1016/j.chb.2012.11.021
Capozzi, F., and Ristic, J. (2018). How attention gates social interactions: attention and social interactions. Ann. N. Y. Acad. Sci. 1426, 179–198. doi: 10.1111/nyas.13854
Capozzi, F., and Ristic, J. (2020). Attention AND mentalizing? Reframing a debate on social orienting of attention. Vis. Cogn. 28, 97–105. doi: 10.1080/13506285.2020.1725206
Carpenter, J. M., Green, M. C., and Vacharkulksemsuk, T. (2016). Beyond perspective-taking: mind-reading motivation. Motiv. Emot. 40, 358–374. doi: 10.1007/s11031-016-9544-z
Caruana, N., de Lissa, P., and McArthur, G. (2015). The neural time course of evaluating self-initiated joint attention bids. Brain Cogn. 98, 43–52. doi: 10.1016/j.bandc.2015.06.001
Caruana, N., de Lissa, P., and McArthur, G. (2017a). Beliefs about human agency influence the neural processing of gaze during joint attention. Soc. Neurosci. 12, 194–206. doi: 10.1080/17470919.2016.1160953
Caruana, N., McArthur, G., Woolgar, A., and Brock, J. (2017b). Simulating social interactions for the experimental investigation of joint attention. Neurosci. Biobehav. Rev. 74, 115–125. doi: 10.1016/j.neubiorev.2016.12.022
Castelli, F., Happé, F., Frith, U., and Frith, C. (2000). Movement and mind: a functional imaging study of perception and interpretation of complex intentional movement patterns. NeuroImage 12, 314–325. doi: 10.1006/nimg.2000.0612
Cazzato, V., Liuzza, M. T., Caprara, G. V., Macaluso, E., and Aglioti, S. M. (2015). The attracting power of the gaze of politicians is modulated by the personality and ideological attitude of their voters: a functional magnetic resonance imaging study. Eur. J. Neurosci. 42, 2534–2545. doi: 10.1111/ejn.13038
Cheetham, M., Suter, P., and Jäncke, L. (2011). The human likeness dimension of the “uncanny valley hypothesis”: behavioral and functional MRI findings. Front. Hum. Neurosci. 5:126. doi: 10.3389/fnhum.2011.00126
Cheetham, M., Suter, P., and Jancke, L. (2014). Perceptual discrimination difficulty and familiarity in the uncanny valley: more like a “happy valley.” Front. Psychol. 5:1219. doi: 10.3389/fpsyg.2014.01219
Ciardo, F., Marino, B. F. M., Actis-Grosso, R., Rossetti, A., and Ricciardelli, P. (2014). Face age modulates gaze following in young adults. Sci. Rep. 4:4746. doi: 10.1038/srep04746
Dalmaso, M., Castelli, L., and Galfano, G. (2020). Social modulators of gaze-mediated orienting of attention: a review. Psychon. Bull. Rev. doi: 10.3758/s13423-020-01730-x [Epub ahead of print]
Dalmaso, M., Edwards, S. G., and Bayliss, A. P. (2016). Re-encountering individuals who previously engaged in joint gaze modulates subsequent gaze cueing. J. Exp. Psychol. Learn. Mem. Cogn. 42, 271–284. doi: 10.1037/xlm0000159
Dalmaso, M., Galfano, G., and Castelli, L. (2015). The impact of same‐ and other-race gaze distractors on the control of saccadic eye movements. Perception 44, 1020–1028. doi: 10.1177/0301006615594936
Dalmaso, M., Galfano, G., Coricelli, C., and Castelli, L. (2014). Temporal dynamics underlying the modulation of social status on social attention. PLoS One 9:e93139. doi: 10.1371/journal.pone.0093139
Dalmaso, M., Pavan, G., Castelli, L., and Galfano, G. (2012). Social status gates social attention in humans. Biol. Lett. 8, 450–452. doi: 10.1098/rsbl.2011.0881
Deaner, R. O., Shepherd, S. V., and Platt, M. L. (2007). Familiarity accentuates gaze cuing in women but not men. Biol. Lett. 3, 64–67. doi: 10.1098/rsbl.2006.0564
Desimone, R., and Duncan, J. (1995). Neural mechanisms of selective visual attention. Annu. Rev. Neurosci. 18, 193–222. doi: 10.1146/annurev.ne.18.030195.001205
Deska, J. C., Lloyd, E. P., and Hugenberg, K. (2016). Advancing our understanding of the interface between perception and intergroup relations. Psychol. Inq. 27, 286–289. doi: 10.1080/1047840X.2016.1215208
Dodd, M. D., Hibbing, J. R., and Smith, K. B. (2011). The politics of attention: gaze-cuing effects are moderated by political temperament. Atten. Percept. Psychophys. 73, 24–29. doi: 10.3758/s13414-010-0001-x
Dodd, M. D., Hibbing, J. R., and Smith, K. B. (2016). “The politics of attention: differences in visual cognition between liberals and conservatives” in Psychology of learning and motivation. Vol. 65. Academic Press, 277–309.
Egner, T., and Hirsch, J. (2005). Cognitive control mechanisms resolve conflict through cortical amplification of task-relevant information. Nat. Neurosci. 8, 1784–1790. doi: 10.1038/nn1594
Emery, N. J. (2000). The eyes have it: the neuroethology, function and evolution of social gaze. Neurosci. Biobehav. Rev. 24, 581–604. doi: 10.1016/S0149-7634(00)00025-7
Ferrey, A. E., Burleigh, T. J., and Fenske, M. J. (2015). Stimulus-category competition, inhibition, and affective devaluation: a novel account of the uncanny valley. Front. Psychol. 6:249. doi: 10.3389/fpsyg.2015.00249
Fini, C., Committeri, G., Müller, B. C. N., Deschrijver, E., and Brass, M. (2015). How watching Pinocchio movies changes our subjective experience of extrapersonal space. PLoS One 10:e0120306. doi: 10.1371/journal.pone.0120306
Fox, E., Mathews, A., Calder, A. J., and Yiend, J. (2007). Anxiety and sensitivity to gaze direction in emotionally expressive faces. Emotion 7, 478–486. doi: 10.1037/1528-3542.7.3.478
Freeman, J. B., and Ambady, N. (2010). MouseTracker: software for studying real-time mental processing using a computer mouse-tracking method. Behav. Res. Methods 42, 226–241. doi: 10.3758/BRM.42.1.226
Friesen, C. K., and Kingstone, A. (1998). The eyes have it! Reflexive orienting is triggered by nonpredictive gaze. Psychon. Bull. Rev. 5, 490–495. doi: 10.3758/BF03208827
Friesen, C. K., Ristic, J., and Kingstone, A. (2004). Attentional effects of counterpredictive gaze and arrow cues. J. Exp. Psychol. Hum. Percept. Perform. 30, 319–329. doi: 10.1037/0096-1523.30.2.319
Frischen, A., Bayliss, A. P., and Tipper, S. P. (2007). Gaze cueing of attention: visual attention, social cognition, and individual differences. Psychol. Bull. 133, 694–724. doi: 10.1037/0033-2909.133.4.694
Frischen, A., and Tipper, S. P. (2006). Long-term gaze cueing effects: evidence for retrieval of prior states of attention from memory. Vis. Cogn. 14, 351–364. doi: 10.1080/13506280544000192
Gallagher, H. L., and Frith, C. D. (2003). Functional imaging of ‘theory of mind.’ Trends Cogn. Sci. 7, 77–83. doi: 10.1016/S1364-6613(02)00025-6
Gao, T., McCarthy, G., and Scholl, B. J. (2010). The wolfpack effect: perception of animacy irresistibly influences interactive behavior. Psychol. Sci. 21, 1845–1853. doi: 10.1177/0956797610388814
Gobel, M. S., Tufft, M. R. A., and Richardson, D. C. (2017). Social beliefs and visual attention: how the social relevance of a cue influences spatial orienting. Cogn. Sci. 42, 161–185. doi: 10.1111/cogs.12529
Graham, R., Kelland Friesen, C., Fichtenholtz, H. M., and LaBar, K. S. (2010). Modulation of reflexive orienting to gaze direction by facial expressions. Vis. Cogn. 18, 331–368. doi: 10.1080/13506280802689281
Gray, H. M., Gray, K., and Wegner, D. M. (2007). Dimensions of mind perception. Science 315:619. doi: 10.1126/science.1134475
Hackel, L. M., Looser, C. E., and Van Bavel, J. J. (2014). Group membership alters the threshold for mind perception: the role of social identity, collective identification, and intergroup threat. J. Exp. Soc. Psychol. 52, 15–23. doi: 10.1016/j.jesp.2013.12.001
Hayward, D. A., and Ristic, J. (2013). The uniqueness of social attention revisited: working memory load interferes with endogenous but not social orienting. Exp. Brain Res. 231, 405–414. doi: 10.1007/s00221-013-3705-z
Hertz, N., and Wiese, E. (2017). Social facilitation with nonhuman agents: possible or not? Proc. Hum. Factors Ergon. Soc. Annu. Meet. 61, 222–225. doi: 10.1177/1541931213601539
Hood, B. M., Willen, J., and Driver, J. (1998). Adult’s eyes trigger shifts of visual attention in human infants. Psychol. Sci. 9, 131–134. doi: 10.1111/1467-9280.00024
Hori, E., Tazumi, T., Umeno, K., Kamachi, M., Kobayashi, T., Ono, T., et al. (2005). Effects of facial expression on shared attention mechanisms. Physiol. Behav. 84, 397–405. doi: 10.1016/j.physbeh.2005.01.002
Hungr, C. J., and Hunt, A. R. (2012). Physical self-similarity enhances the gaze-cueing effect. Q. J. Exp. Psychol. 65, 1250–1259. doi: 10.1080/17470218.2012.690769
Jones, B. C., DeBruine, L. M., Main, J. C., Little, A. C., Welling, L. L. M., Feinberg, D. R., et al. (2010). Facial cues of dominance modulate the short-term gaze-cuing effect in human observers. Proc. Biol. Sci. 277, 617–624. doi: 10.1098/rspb.2009.1575
Kätsyri, J., Förger, K., Mäkäräinen, M., and Takala, T. (2015). A review of empirical evidence on different uncanny valley hypotheses: support for perceptual mismatch as one road to the valley of eeriness. Front. Psychol. 6:390. doi: 10.3389/fpsyg.2015.00390
Kawai, N. (2011). Attentional shift by eye gaze requires joint attention: eye gaze cues are unique to shift attention1: social attention by the gaze cues. Jpn. Psychol. Res. 53, 292–301. doi: 10.1111/j.1468-5884.2011.00470.x
Kompatsiari, K., Ciardo, F., Tikhanoff, V., Metta, G., and Wykowska, A. (2018). On the role of eye contact in gaze cueing. Sci. Rep. 8:17842. doi: 10.1038/s41598-018-36136-2
Kühn, S., Brick, T. R., Müller, B. C. N., and Gallinat, J. (2014). Is this car looking at you? How anthropomorphism predicts fusiform face area activation when seeing cars. PLoS One 9:e113885. doi: 10.1371/journal.pone.0113885
Law, A. S., Langton, S. R. H., and Logie, R. H. (2010). Assessing the impact of verbal and visuospatial working memory load on eye-gaze cueing. Vis. Cogn. 18, 1420–1438. doi: 10.1080/13506285.2010.496579
Lee, A. Y. (2001). The mere exposure effect: an uncertainty reduction explanation revisited. Pers. Soc. Psychol. Bull. 27, 1255–1266. doi: 10.1177/01461672012710002
Li, L., Miller, E. K., and Desimone, R. (1993). The representation of stimulus familiarity in anterior inferior temporal cortex. J. Neurophysiol. 69, 1918–1929. doi: 10.1152/jn.1993.69.6.1918
Liuzza, M. T., Cazzato, V., Vecchione, M., Crostella, F., Caprara, G. V., and Aglioti, S. M. (2011). Follow my eyes: the gaze of politicians reflexively captures the gaze of ingroup voters. PLoS One 6:e25117. doi: 10.1371/journal.pone.0025117
Looser, C. E., Guntupalli, J. S., and Wheatley, T. (2013). Multivoxel patterns in face-sensitive temporal regions reveal an encoding schema based on detecting life in a face. Soc. Cogn. Affect. Neurosci. 8, 799–805. doi: 10.1093/scan/nss078
Looser, C. E., and Wheatley, T. (2010). The tipping point of animacy: how, when, and where we perceive life in a face. Psychol. Sci. 21, 1854–1862. doi: 10.1177/0956797610388044
Lundqvist, D., Flykt, A., and Ohman, A. (1998). Karolinska directed emotional faces. Psychology Section, Department of Clinical Neuroscience, Karolinska Hospital, S-171, 76.
MacDorman, K. F., and Chattopadhyay, D. (2016). Reducing consistency in human realism increases the uncanny valley effect; increasing category uncertainty does not. Cognition 146, 190–205. doi: 10.1016/j.cognition.2015.09.019
MacDorman, K. F., Green, R. D., Ho, C. -C., and Koch, C. T. (2009). Too real for comfort? Uncanny responses to computer generated faces. Comput. Hum. Behav. 25, 695–710. doi: 10.1016/j.chb.2008.12.026
Mäkäräinen, M., Kätsyri, J., and Takala, T. (2014). Exaggerating facial expressions: a way to intensify emotion or a way to the uncanny valley? Cogn. Comput. 6, 708–721. doi: 10.1007/s12559-014-9273-0
Martini, M., Buzzell, G., and Wiese, E. (2015). Agent appearance modulates mind attribution and social attention in human-robot interaction. Soc. Robot. 1, 431–439. doi: 10.1007/978-3-319-25554-5
Martini, M. C., Gonzalez, C. A., and Wiese, E. (2016). Seeing minds in others—can agents with robotic appearance have human-like preferences? PLoS One 11:e0146310. doi: 10.1371/journal.pone.0146310
Mathur, M. B., and Reichling, D. B. (2016). Navigating a social world with robot partners: a quantitative cartography of the uncanny valley. Cognition 146, 22–32. doi: 10.1016/j.cognition.2015.09.008
McNeish, D. (2016). On using Bayesian methods to address small sample problems. Struct. Equ. Modeling 23, 750–773. doi: 10.1080/10705511.2016.1186549
Meng, M., and Tong, F. (2004). Can attention selectively bias bistable perception? Differences between binocular rivalry and ambiguous figures. J. Vis. 4, 539–551. doi: 10.1167/4.7.2
Mitchell, W. J., Szerszen, K. A., Lu, A. S., Schermerhorn, P. W., Scheutz, M., and MacDorman, K. F. (2011). A mismatch in the human realism of face and voice produces an uncanny valley. Iperception 2, 10–12. doi: 10.1068/i0415
Morgan, E. J., Freeth, M., and Smith, D. T. (2018). Mental state attributions mediate the gaze cueing effect. Vision 2:11. doi: 10.3390/vision2010011
Mori, M. (1970). The uncanny valley: the original essay by masahiro mori. Energy. Available at: http://spectrum.ieee.org/automaton/robotics/humanoids/the-uncanny-valley (Accessed January 2020).
Müller, B. C. N., Brass, M., Kühn, S., Tsai, C. -C., Nieuwboer, W., Dijksterhuis, A., et al. (2011). When Pinocchio acts like a human, a wooden hand becomes embodied. Action co-representation for non-biological agents. Neuropsychologia 49, 1373–1377. doi: 10.1016/j.neuropsychologia.2011.01.022
Müller, B. C. N., van Baaren, R. B., van Someren, D. H., and Dijksterhuis, A. (2014). A present for Pinocchio: on when non-biological agents become real. Soc. Cogn. 32, 381–396. doi: 10.1521/soco.2014.32.4.381
Ohlsen, G., van Zoest, W., and van Vugt, M. (2013). Gender and facial dominance in gaze cuing: emotional context matters in the eyes that we follow. PLoS One 8:e59471. doi: 10.1371/journal.pone.0059471
Palermo, R., and Rhodes, G. (2007). Are you always on my mind? A review of how face perception and attention interact. Neuropsychologia 45, 75–92. doi: 10.1016/j.neuropsychologia.2006.04.025
Parasuraman, R., de Visser, E., Clarke, E., McGarry, W. R., Hussey, E., Shaw, T., et al. (2009). Detecting threat-related intentional actions of others: effects of image quality, response mode, and target cuing on vigilance. J. Exp. Psychol. Appl. 15, 275–290. doi: 10.1037/a0017132
Pavan, G., Dalmaso, M., Galfano, G., and Castelli, L. (2011). Racial group membership is associated to gaze-mediated orienting in Italy. PLoS One 6:e25608. doi: 10.1371/journal.pone.0025608
Pecchinenda, A., and Petrucci, M. (2016). Emotion unchained: facial expression modulates gaze cueing under cognitive load. PLoS One 11:e0168111. doi: 10.1371/journal.pone.0168111
Phillips, E., Zhao, X., Ullman, D., and Malle, B. F. (2018). “What is human-like? Decomposing robots’ human-like appearance using the anthropomorphic roBOT (ABOT) database” in Proceedings of the 2018 ACM/IEEE International Conference on Human-Robot Interaction; March 2018. 105–113.
Porciello, G., Holmes, B. S., Liuzza, M. T., Crostella, F., Aglioti, S. M., and Bufalari, I. (2014). Interpersonal multisensory stimulation reduces the overwhelming distracting power of self-gaze: psychophysical evidence for ‘engazement.’ Sci. Rep. 4:6669. doi: 10.1038/srep06669
Quadflieg, S., Mason, M. F., and Macrae, C. N. (2004). The owl and the pussycat: gaze cues and visuospatial orienting. Psychon. Bull. Rev. 11, 826–831. doi: 10.3758/BF03196708
Schilbach, L., Timmermans, B., Reddy, V., Costall, A., Bente, G., Schlicht, T., et al. (2013). Toward a second-person neuroscience. Behav. Brain Sci. 36, 393–414. doi: 10.1017/S0140525X12000660
Seyama, J., and Nagayama, R. S. (2007). The uncanny valley: effect of realism on the impression of artificial human faces. Presence Teleop. Virt. 16, 337–351. doi: 10.1162/pres.16.4.337
Singer, T., Snozzi, R., Bird, G., Petrovic, P., Silani, G., Heinrichs, M., et al. (2008). Effects of oxytocin and prosocial behavior on brain responses to direct and vicariously experienced pain. Emotion 8, 781–791. doi: 10.1037/a0014195
Sterzer, P., Kleinschmidt, A., and Rees, G. (2009). The neural bases of multistable perception. Trends Cogn. Sci. 13, 310–318. doi: 10.1016/j.tics.2009.04.006
Süßenbach, F., and Schönbrodt, F. (2014). Not afraid to trust you: trustworthiness moderates gaze cueing but not in highly anxious participants. J. Cogn. Psychol. 26, 1–9. doi: 10.1080/20445911.2014.945457
Teufel, C., Alexis, D. M., Clayton, N. S., and Davis, G. (2010). Mental-state attribution drives rapid, reflexive gaze following. Atten. Percept. Psychophys. 72, 695–705. doi: 10.3758/APP.72.3.695
Tipples, J. (2006). Fear and fearfulness potentiate automatic orienting to eye gaze. Cogn. Emot. 20, 309–320. doi: 10.1080/02699930500405550
Umemoto, A., Inzlicht, M., and Holroyd, C. B. (2018). Electrophysiological indices of anterior cingulate cortex function reveal changing levels of cognitive effort and reward valuation that sustain task performance. Neuropsychologia 123, 67–76. doi: 10.1016/j.neuropsychologia.2018.06.010
Vecera, S. P., and Rizzo, M. (2006). Eye gaze does not produce reflexive shifts of attention: evidence from frontal-lobe damage. Neuropsychologia 44, 150–159. doi: 10.1016/j.neuropsychologia.2005.04.010
Visser, T. A. W., and Roberts, A. (2018). Automaticity of social cues: the influence of limiting cognitive resources on head orientation cueing. Sci. Rep. 8:10288. doi: 10.1038/s41598-018-28548-x
Wagner, D. D., Kelley, W. M., and Heatherton, T. F. (2011). Individual differences in the spontaneous recruitment of brain regions supporting mental state understanding when viewing natural social scenes. Cereb. Cortex 21, 2788–2796. doi: 10.1093/cercor/bhr074
Weis, P. P., and Wiese, E. (2017). Cognitive conflict as possible origin of the uncanny valley. Proc. Hum. Factors Ergon. Soc. Annu. Meet. 61, 1599–1603. doi: 10.1177/1541931213601763
Wheatley, T., Weinberg, A., Looser, C., Moran, T., and Hajcak, G. (2011). Mind perception: real but not artificial faces sustain neural activity beyond the N170/VPP. PLoS One 6:e17960. doi: 10.1371/journal.pone.0017960
Wiese, E., Buzzell, G. A., Abubshait, A., and Beatty, P. J. (2018). Seeing minds in others: mind perception modulates low-level social-cognitive performance and relates to ventromedial prefrontal structures. Cogn. Affect. Behav. Neurosci. 18, 837–856. doi: 10.3758/s13415-018-0608-2
Wiese, E., Mandell, A., Shaw, T., and Smith, M. (2019). Implicit mind perception alters vigilance performance because of cognitive conflict processing. J. Exp. Psychol. Appl. 25, 25–40. doi: 10.1037/xap0000186
Wiese, E., and Weis, P. P. (2020). It matters to me if you are human—examining categorical perception in human and nonhuman agents. Int. J. Hum. Comput. Stud. 133, 1–12. doi: 10.1016/j.ijhcs.2019.08.002
Wiese, E., Wykowska, A., and Müller, H. J. (2014). What we observe is biased by what other people tell us: beliefs about the reliability of gaze behavior modulate attentional orienting to gaze cues. PLoS One 9:e94529. doi: 10.1371/journal.pone.0094529
Wiese, E., Wykowska, A., Zwickel, J., and Müller, H. J. (2012). I see what you mean: how attentional selection is shaped by ascribing intentions to others. PLoS One 7:e45391. doi: 10.1371/journal.pone.0045391
Wiese, E., Zwickel, J., and Müller, H. J. (2013). The importance of context information for the spatial specificity of gaze cueing. Atten. Percept. Psychophys. 75, 967–982. doi: 10.3758/s13414-013-0444-y
Wykowska, A., Wiese, E., Prosser, A., and Müller, H. J. (2014). Beliefs about the minds of others influence how we process sensory information. PLoS One 9:e94339. doi: 10.1371/journal.pone.0094339
Keywords: human-robot interaction, social-cognition, gaze-cueing, cognitive conflict, categorical boundary, mind perception
Citation: Abubshait A, Momen A and Wiese E (2020) Pre-exposure to Ambiguous Faces Modulates Top-Down Control of Attentional Orienting to Counterpredictive Gaze Cues. Front. Psychol. 11:2234. doi: 10.3389/fpsyg.2020.02234
Edited by:
Adrian Von Mühlenen, University of Warwick, United KingdomReviewed by:
Chiara Fini, Sapienza University of Rome, ItalyMario Dalmaso, University of Padua, Italy
Copyright © 2020 Abubshait, Momen and Wiese. This is an open-access article distributed under the terms of the Creative Commons Attribution License (CC BY). The use, distribution or reproduction in other forums is permitted, provided the original author(s) and the copyright owner(s) are credited and that the original publication in this journal is cited, in accordance with accepted academic practice. No use, distribution or reproduction is permitted which does not comply with these terms.
*Correspondence: Abdulaziz Abubshait, YWJkdWxheml6LmFidWJzaGFpdEBpaXQuaXQ=