- 1School of Business Sciences, Universidad del Pacífico, Lima, Peru
- 2School of Accounting, Economic and Business Sciences, Universidad de Manizales, Manizales, Colombia
- 3School of Administration, Universidad del Tolima, Ibagué, Colombia
- 4School of Economic and Business Sciences, Universidad de Sevilla, Seville, Spain
- 5Colegio de Estudios Superiores de Administración, Bogotá, Colombia
Literature suggests that human resources of non-profit hospitals (NPHs) present features that could potentially reach any expected organizational performance even when the attention to human resource management (HRM) are often low in non-profit organizations. Nowadays ambitious organizations strive to obtain a profitable performance that is also innovate and do it through building an organizational culture (OC), while for NPHs a positive culture is given by their human resources traits. However, there is not enough literature to understand how these three variables behave together. This study aims to explain the influence of HRM on IP mediated by OC. The research model was assessed through Partial Least Squares Structural Equation Modeling (PLS-SEM). The results support all the stated hypotheses. Both, HRM and OC are moderately strong predictors of IP, and OC mediates partially and in a complementary way the relationship between HRM on IP. An importance-performance map analysis (IPMA) was performed to expand the PLS-SEM results. The OC indicators show greater importance to explain IP, consequently, they are the most relevant indicators to initiate management actions by NPHs. The influence of HRM on IP represent an opportunity for NPH as it implies an affordable investment in comparison to the cost of technological solutions for enterprises.
Introduction
Non-profit hospital (NPH) is a governmental or private organization that orient their services to low-income population. For this reason, NPH’s patients are not expected to have the money to pay for advanced medical care, however, medical advancements have a great deal with providing effective treatment and covering a wider scope of illnesses than regular medicine does. For this reason, the use of innovation in NPHs marks the quality of the health services they provide.
Current organizations follow a vision that involves innovation as it guarantees their success and survival in an evolutionary environment and in outcome terms it is translated as an innovative performance (IP). Innovation is needed to redefine business models in order to make them more affordable for low- and middle-income people. In developing countries, the organizational IP has redesigned cost structure that make products and services more affordable and accessible (George et al., 2015). For this reason, social innovation represents an opportunity to democratize access to basic medical services (Michelini, 2012; Christensen et al., 2015) through the implementation of innovative solution in non-profit hospitals. Actually, non-profit organizations orient their IP to social innovation that leads to aims more aligned to their users’ expectation (Westley et al., 2014; Phillips et al., 2015).
Particularly, NPH’s hold a responsibility to be innovative when delivering medical care. An IP for this organizations consists on answering the diverse needs of patients by new projects, processes or services (Sanzo-Perez et al., 2015; Jaskyte, 2018, 2020; Brimhall, 2019). In other words, innovation comes from the introduction of new ideas or practices that leads to the improvement of the treatment, the diagnose, prevention, research or education (Omachonu and Einspruch, 2010). Moreover, social innovation involves collaborations to implement solutions to social problems, particularly at local level (Brandsen et al., 2016). Solutions are assumed to have positive societal effects, either through increasing aggregate utilitarian value, or by empowering citizens in innovation processes (Ayob et al., 2016).
In this scenery, NPHs limit their performance to a tight budget and therefore have less chances to innovate. There are less resources and yet a constant need to adjust to a competitive environment (Svensson et al., 2019). In particular, aside the financial resources, human resources are other highly relevant resources to pursue an IP. Indeed, the impact of human resource management (HRM) reaches individual achievements regarding skills and motivation amongst other personal traits that are pre-requisites for innovation (Diaz-Fernandez et al., 2017). That is to say, IP, understood as the capacity of a business to obtain new products and other outputs, is strongly related to HRM (Adnan et al., 2016; Métailler, 2016; Diaz-Fernandez et al., 2017). Through the activities undertaken by HRM, namely building a culture that values new ideas or enabling employees to keep growing professionally, it is noticeable how they influence on the employees and consequently impact on IP as well (Bal et al., 2014; Liao and Huang, 2016; Diaz-Fernandez et al., 2017; Gile et al., 2018; Jaskyte, 2018; Meyer and Leitner, 2018).
The implementation of HRM’s strategies shapes the organizational culture (OC). In particular, an innovative culture might lead to an IP. Mesch (2010) pointed out that HRM is the main element to reach an effective organizational performance in non-profit organizations as in other organization types. For this reason, HRM are likely to influence OC within the particular context of NPH (Jaskyte, 2011, 2015, 2018; Brown et al., 2016; Aktar and Pangil, 2017; Baluch, 2017). Furthermore, OC is recognized by its role to dynamize the IP (Peters and Waterman, 1982; Fondas and Denison, 1991; Ahmed, 1998; Jaskyte and de Riobó, 2004; Jaskyte, 2015; Meyer and Leitner, 2018). This relationship has been also studied in contexts such as NPH finding significant results (McDonald, 2007; Brimhall, 2019; Narapareddy and Berte, 2019). Hence, OC is likely to influence IP directly and mediate the influence of HRM on IP too.
NPHs face difficulties to reach the expected health service, they do not often count with the technology needed, the specialties, or the medicine, to mention some of their many limitations. Nevertheless, they are the best affordable option for most of the population. Due to that fact, it is necessary they have alternative strategies to rise their service quality through innovation. Bearing that in mind, this study aims to examine the relationship between HRM and IP, while considering the mediating effect of OC. In order to do so, the research question is stated as follows: What is the direct effect of HRM on IP, mediated by OC, in NPH?
This study is divided into five sections. The first section has introduced the topic and elaborated on the research problem this study aims to address. Section “Literature Review” presents the theoretical framework regarding this study’s main constructs. The procedure followed to obtain and analyses the data is presented “Materials and Methods,” while the results are explained in section “Results and Discussion.” Finally, section “Conclusion and Recommendations for Future Studies” discusses the findings of this study and give directions for future research.
Literature Review
This section elaborates on the constructs involved in this study’s theoretical model. It addresses the theory reviewed about the management of the human resources, the updated literature on innovation regarding the organization performance, and finally, the organizational culture’s literature is pinpointed as a mediator among the other two constructs.
Human Resources Management
Human resources management holds a strategic role to reassure organizational effectiveness through the human resources of a company. In fact, according to the resources based theory, HRM is responsible of managing part of the strategic resources of the organization to enable firms’ growth and competitive advantages for a superior performance (Wernerfelt, 1984; Barney, 1991; Conner, 1991; Grant, 1991; Collis and Montgomery, 1995; Teece et al., 1997). The Resource-Based theory analyzes and interprets the strategic internal resources of organizations such as: resources, capacities, organizational processes, information, knowledge, among others that enable to develop and maintain competitive advantages (Barney, 1991).
However, the globalized market requires companies a strategic envision of their knowledge resource. Kang et al. (2007) elaborates on this theory and came up with the knowledge-based theory that give human resources a relevant meaning in the process of value creation. Doing so, this theory links organizational learning, social relations and HRM into the same knowledge flow. That is to say, HRM is crucial at fostering innovation processes in companies (Li et al., 2006) by influencing creativity (Jiang et al., 2012) and knowledge management system (Jiménez-Jiménez and Sanz-Valle, 2011). Moreover, knowledge-based perspective regarding organizational capacities outreached by HRM are related to OC and have an impact on innovation success (Long and Fahey, 2000; Leal-Rodríguez et al., 2014).
In the particular contexts of non-profit organizations, prior research stated that even when the investment on HRM is less than the standard, it still earns positive outcomes (Nickson et al., 2008; Baines, 2010; Atkinson and Lucas, 2013; Ariza-Montes and Lucia-Casademunt, 2016; Baluch, 2017). Yet strategies on HRM have demonstrated to bring good results in employees that later on are beneficial to the organization’s performance as earlier stated. A key factor to success of non-profit organizations is the ability to identify and develop capacities through their available resources. For this reason, resources that has been proven to be useful in past experiences such as culture, HRM, among others, are considered strategic (Colbert, 2004; Akingbola, 2013; Oliveira and Toda, 2013; Brown et al., 2016; Gile et al., 2018).
Innovative Performance
Nowadays, innovative organizations have more chances to survive in the competitive global market. Innovation is a complex term and most of the time is related to technology, however, is not only limited by the scientific and technological dimensions (Echevarría, 2008). A general approach states that “innovation is the implementation of a new or significantly improved product (good or service), or process, a new marketing method, or a new organizational method in business practices, workplace organization or external relations” (OECD/Eurostat, 2005). To complement this approach, Gurrutxaga (2011) disaggregates innovation in the ability to create and innovate as options to respond to social necessities, defining structural constraints and rescuing the relevance of innovation.
Regarding the healthcare sector, innovation is the introduction of a new concept, idea, service, process or product to improve treatment, diagnosis, education, prevention and research, aiming a long-term objective to improve quality, safety, results, efficiency and costs (Omachonu and Einspruch, 2010). This means that novel practices on the benefit of effective healthcare favors to reduce rates of mortality and morbidity. Based on the patients’ perspective, the benefit of healthcare sector innovation is evident through improved health or reduced suffering due to illness (Faulkner and Kent, 2001). In other words, innovation has a wide scope to be implemented in this sort of services.
It is necessary to notice that mostly all definitions regarding innovation come from a for-profit organization approach regardless the nature of other types of organizations such as non-profit ones. However, non-profit organizations outreach successful levels of innovation that are aligned to social innovation initiatives. Svensson et al. (2019) consider that innovation in non-profit organizations, social innovation, happens with “better ways of achieving meaningful impact in addressing a given social issue and promoting positive social change.” This put non-profit organizations in a double pressure situation to keep financially stable and maintain social performance toward meeting their mission and satisfying numerous stakeholders (Landrum, 2007). Overall, NPHs aiming to perform innovatively address healthcare in a way that makes a social change which means a social innovative performance capable to reach not just a change, but a shareable social value creation (Hwang and Christensen, 2008; Michelini, 2012) that will end up in a more inclusive healthcare service and social nosiness models (Vecchio and Rappini, 2011; Michelini, 2012; Angeli and Jaiswal, 2016).
According to the above, IP is the consequence of a set of variables, however, HRM and OC are relevant when it comes about particular organizations such as NPHs. For this reason, this study seeks to explain the influence of HRM on IP and OC on IP. These objectives determine the following hypotheses:
H1: HRM influences positively the IP.
H3: OC influence positively the IP.
Organizational Culture
The organizational culture is defined as the set of intergroup interrelationships that manifests mainly when changing opportunities and threats take place in the organization’s environment. In the healthcare sector, adaptation to new situations could determine life or death which remains mandatory for them to build an organizational culture that could handle this environment. Nevertheless, NPHs face a challenge on this matter as their resources and vision limit their possibilities to act upon an organizational culture strategy.
Many studies has determine the influence of HRM on OC and how highly related are both variables (Hartog et al., 2004; Kusluvan et al., 2010; Aktar and Pangil, 2017). It means the implementation of strategies to build and environment where human resources are capable to impact on the innovative outcomes by the right knowledge acquisition, distribution and storage by employees. Ballesteros-Rodríguez et al. (2012) point that culture define how things are done and influence leaders to stablish objectives and practices for HRM. For this reason, HRM and OC seems to have a relationship that works in a bidirectional way.
OC plays a leading role in achieving to reach the expected IP (Jaskyte and Dressler, 2005; Jaskyte, 2018). Furthermore, the literature recognizes the role of principles and climate of the organization as elements of the OC that enable an IP (Peters and Waterman, 1982; Fondas and Denison, 1991; Ahmed, 1998; Jaskyte, 2004, 2015; Meyer and Leitner, 2018). This approach has also been developed regarding the health sector (McDonald, 2007; Brimhall, 2019; Narapareddy and Berte, 2019) but not the non-profit hospitals in particular. For this reason, OC influence IP while it is affected by HRM, then HRM influence on IP might be influenced by the mediator role of OC.
The proposed model is theoretically based on mediation because a mediating variable (OC) intervenes between two constructs that are related (HRM and IP). This implies that a change in HRM will lead to a change in OC, which in turn will generate a change in the endogenous variable IP. What this mediation model seeks is to analyze the intensity of OC relationships with the other constructs, justifying the mechanisms underlying the cause-effect relationship between HRM and IP. Unlike what a moderation model seeks, where the intensity or sense of the relationship between variables depends on a third variable that does not directly interact with the exogenous or endogenous variable (Nitzl et al., 2016).
In line with the above, this study analyses how HRM influence OC. Besides it is assessed the mediator role of OC in the relationship between HRM and IP. These objectives are stated in the following hypotheses:
H2: HRM influences positively the OC.
H4: OC mediates the relationship between HRM and IP.
Figure 1 represents graphically the theoretical model for this study and the hypotheses associated to it.
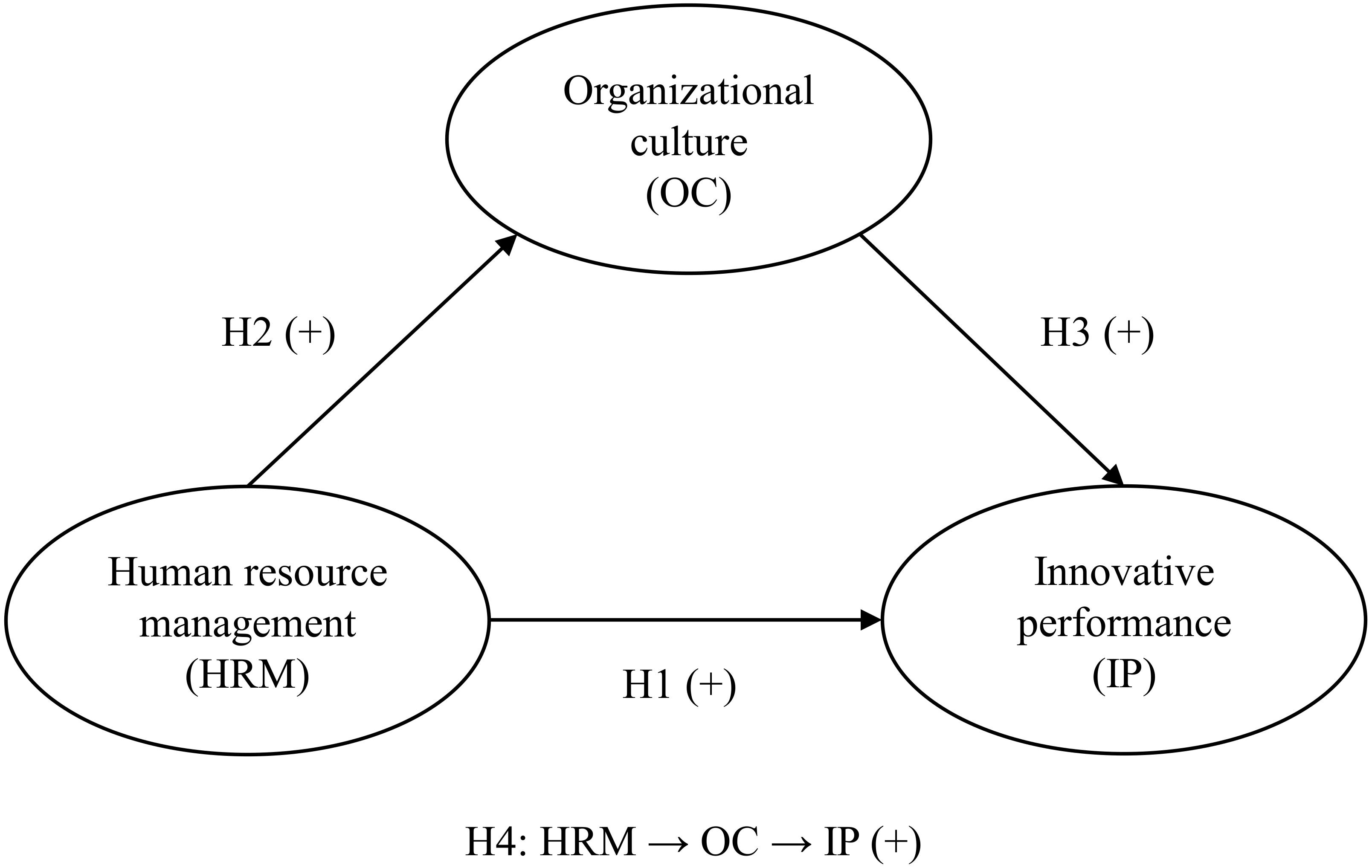
Figure 1. Theoretical model and research hypotheses; HRM, human resource management; OC, organizational culture; IP, innovative performance.
Materials and Methods
Design
According to the research designs classification system in Psychology by Ato et al. (2013) this study belongs to an empirical study. The strategy was associative as it explores the functional relationship among the three variables under analysis. The type of study is explanatory, where a mediation model was tested. Finally, latent variables design (LVD) or structural equation modeling (SEM) was used, consisting of two parts that make up the model: one structural or inner model (the relationship among the constructs) and the other measurement or outer model (the relationship between the indicators and the constructs they measure). The statistical procedure to estimate the parameters of the SEM model was based on the variances, also known as Partial Least Squares (PLS-SEM).
Participants
The study sample was made up of health sector personnel working in Colombian non-profit hospitals (NPHs). These are public, private or mixed organizations that provide medical care in three levels: (1) care by general, technical and auxiliary personnel, with low complexity technology, (2) care by specialized personnel, with medium complexity technology, and (3) care by specialized personnel and subspecialized, with the technology of the highest complexity (Prada-Ríos et al., 2017). Only third level NPHs participated and were made up of regional, university and specialized hospitals.
Participants were selected through non-probability, intentional sampling (Kerlinger and Lee, 2000). A priori statistical power analysis was computed to obtain the minimum sample size. This minimum sample size ensures that the results of the statistical method are robust and that the model is generalizable. The analysis was performed using the G∗Power 3.1.9.7 software (Faul et al., 2009). This statistical procedure requires to previously set input parameters, namely a significance level of 0.01 (two tails); an expected statistical power of 0.95, higher than the recommended by Cohen (1992) expected effect size (f2) of 0.15 or moderate effect; and two predictors. After the analysis, the minimum recommended sample size was determined to be 123 (Figure 2).
The sample size was made up of 162 Colombian NPHs workers, however, only 150 were finally included in the study. 12 measurement scales were rejected as they presented more than 15% of incomplete answers and three items or more unanswered (Sarstedt and Mooi, 2019). This resulted in a 92.59% valid response rate. Based on the 150 participants, the time they have been working in the NPHs ranged from 1 to 30 years (M = 6.046, SD = 5.72), 65.3% were women, 31.3% were doctors, almost all (99.3%) belonged to public NPHs, and 34.7% had undergraduate studies. Table 1 presents the complete sociodemographic characteristics of the participants.
Instrument
All the study variables were measured through a measurement scale with satisfactory psychometric properties developed by López et al. (2018a). It measures a set of variables related to innovation in the health sector. For this study, three of these variables were considered: human resources management (measured through 10 items, from HRM–1 to HRM–10), organizational culture (measured through two items, OC–1 and OC–2) and innovative performance (measured through three items, from IP–1 to IP–3). The items were five points Likert scales, ranging from 1 (no activity has been carried out to improve the characteristic of interest) to 5 (positive results have been obtained against the aspect investigated).
The instrument was developed in a sample of 107 Colombian hospitals workers between 2016 and 2017, and the variables associated with innovation in health organizations were selected from a systematic review. In the original scale, the three variables had high levels of internal consistency measured through the alpha coefficient (López et al., 2018b). Besides, it has validity evidence based on the internal structure, through exploratory and confirmatory factor analysis (López et al., 2018b). This sequence of analyses indicate that the instrument meets the psychometric properties required for a measurement scale in the social and health sciences (Acosta-Prado et al., 2020).
Procedure
To collect the data, a form in Google Forms was sent via email to all members of the NPHs. The instrument included information regarding the research objectives. Before sending the email, all workers gave their consent to participate in the study. The responses were stored into a spreadsheet on Google Drive, while taking care of the anonymity of the participant responses. The research was carried out following the Declaration of Helsinki.
The missing data, that is to say not-answered items, were eliminated when they reached more than 15% of unanswered items per case. The remaining missing data was replaced with the mean of the valid values of the correspondent indicator. This method was used because its ease to use and the missing values were found less than 5% of the values per indicator (Hair et al., 2017).
Data Analysis
For the statistical analysis, the Partial Least Squares (PLS), an approximation of the variance-based structural equation modeling (SEM) was used. This technique was selected due to the properties of the constructs that are part of the research model (Ali et al., 2018). PLS-SEM estimates coefficients that maximize the explained variance of endogenous constructs, giving the predictive characteristic to this statistical technique (Mateos-Aparicio, 2011). SmartPLS 3.2.9 software (Ringle et al., 2015) was used for this study.
Among the advantages of the PLS-SEM (Hair et al., 2011) are (a) the absence of identification problems with reduced sample sizes, usually reaching high levels of statistical power with small sample sizes; (b) the data distribution does not involve assumptions as it is a nonparametric method; (c) its robustness in the presence of missing values, as long as they are below a reasonable limit; (d) the amount of unexplained variance is reduced; and (e) the reliability and validity of the measurement models are assessed using various criteria.
In PLS-SEM, the structural model describes the relationships among the latent variables (constructs) and the measurement model represent the relationships between the constructs and their corresponding indicators. Regarding the latter, two specification categories must be considered: reflective and formative measurement models (Rigdon, 2012). In reflective models, the indicators are the effects or manifestations of an underlying construct; on the contrary, in formative models, the indicators form the construct using linear combinations (Jarvis et al., 2003). In this study, a reflective measurement model was used, where reflective indicators support the idea that the construct causes the measurement or covariation of the indicators. Likewise, in the structural model, HRM is an exogenous latent variable, whereas OC and IP are endogenous latent variables.
Before the PLS-SEM analysis, it was verified that the data do not deviate excessively from a normal distribution since data that are far from normal are problematic at evaluating the significance of the parameters. Specifically, these data overestimate the standard errors obtained by bootstrapping which reduces the possibility to consider some path coefficients as statistically significant (Henseler et al., 2009). Therefore, the kurtosis and skewness of the data distributions (indicators) were examined, and values between −1 and +1 were considered appropriate (Hair et al., 2019a). Besides, the mean was estimated as a measure of central tendency and the standard deviation as a measure of variability, both descriptive statistics give an idea of how the participants responded to the items on the measurement scale.
The PLS-SEM results are analyzed following a systematic process. The evaluation of the quality of the measurement and structural models focuses on statistics that indicate the predictive capacity of the model (Hair et al., 2013). Regarding the reflective measurement model, the reliability was assessed by the internal consistency method, using the alpha, rho_A, and composite reliability (CR) coefficients, as well as the reliability of the individual indicator and the average variance extracted (AVE) to evaluate convergent validity. The assessment of the reflective measurement model also included discriminant validity. The Fornell and Larcker criteria and the heterotrait-monotrait ratio (HTMT) were used to examine the discriminant validity. In the structural model, the measures considered were R2 (explained variance), f2 (effect size), Q2predict (predictive performance), and the magnitude and statistical significance of the path coefficients.
To test the statistical significance of the coefficients, the non-parametric bootstrap procedure was used with 10,000 bootstrap samples without sign change (Streukens and Leroi-Werelds, 2016). The bootstrap process provides the standard error for any estimated coefficient and this error serves as the basis to determine the empirical value of t and its associated p-value. Likewise, the bias-corrected and accelerated (BCa) bootstrap-based method was used to construct the confidence intervals at a 95% confidence level and two tails (Henseler et al., 2009).
Finally, an importance-performance map analysis (IPMA) was performed to expand the PLS-SEM results, adding a dimension to the analysis that considers the scores’ mean values of the latent and observable variables (Höck et al., 2010). The objective of this analysis was to identify the antecedent constructs and indicators that have relatively high importance in the objective construct (innovative performance) but at the same time relatively low performance. The latter is important to detect potential areas that should receive more attention (Nigel, 1994).
Results and Discussion
Descriptive Analysis of the Items
Both skewness and kurtosis are close to zero for all the items (Table 2), indicating that the response pattern corresponds to a normal distribution. Specifically, the kurtosis values varied between −0.778 (HRM–4) and 0.223 (OC–1), while the skewness coefficients fluctuated between −0.723 (OC–1) and 0.057 (HRM–8), that are below the limits indicated by Hair et al. (2019b). Likewise, the mean and standard deviation had similar values throughout all the items. The mean was close to 3 and the standard deviation was around 1 (Table 3).
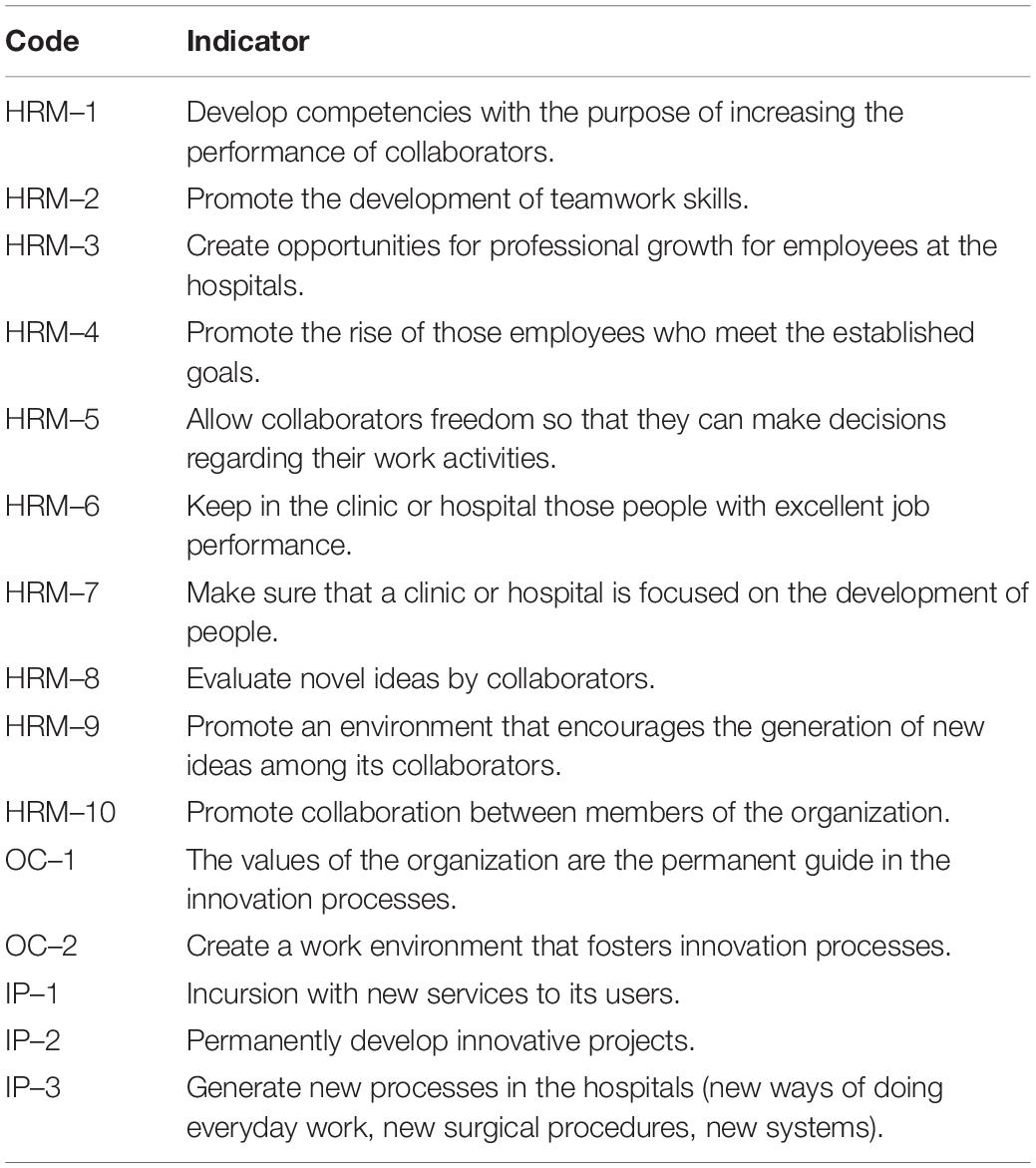
Table 2. Measurement scale to human resource management, organizational culture, and innovative performance.
Measurement Model Evaluation
Internal consistency reliability was the first criterion to evaluate. This indicates the degree of consistency among indicators to measure the constructs. The alpha coefficient, rho_A, and CR were examined and above the critical threshold of 0.700 (Nunnally and Bernstein, 1994). The HRM variable was the one that obtained the highest values in the three coefficients. Due to these results, it can be concluded that the scores obtained by the participants of the study sample in the three constructs presented adequate levels of reliability.
The convergent validity refers to the degree of positive correlation between one measure and other alternative measures of the same construct. For this purpose, the size of the outer loadings, commonly called indicator reliability, was analyzed. All the indicators of the reflective constructs presented loadings equal to or greater than 0.763 (HRM–5), above the criterion of 0.708 (Fornell and Larcker, 1981) see Table 3. Also, the AVE was calculated and it represents the mean value of the commonality of the indicators of certain construct. The three constructs presented values greater than 0.700 (Table 4), showing a very good level of convergent validity (Moral, 2019).
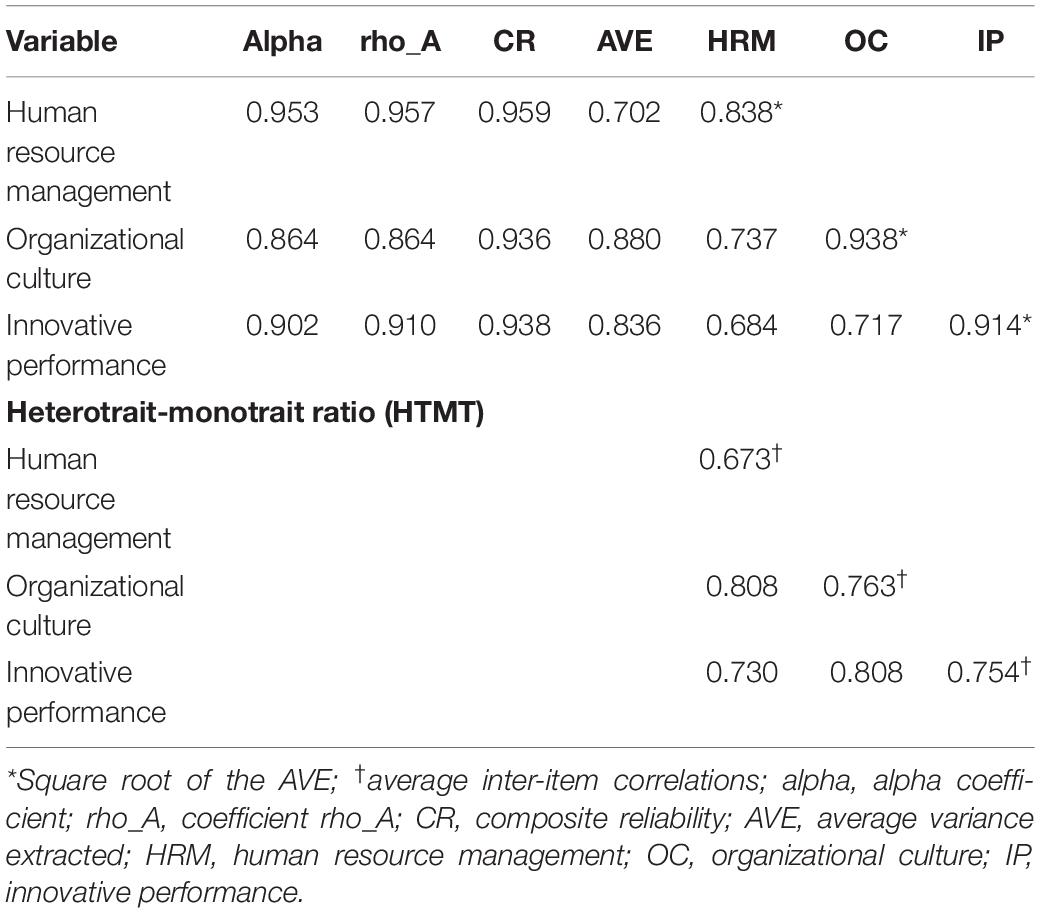
Table 4. Correlation matrix, reliability, convergent and discriminant validity, and heterotrait-monotrait ratio (HTMT).
Concerning discriminant validity, it informs to what extent a construct is different from other constructs. The first method used was the Fornell and Larcker (1981) criterion, which compares the square root of the AVE values with the correlations of the latent variables, where the first must be greater. As a second method, the HTMT ratio of the correlations was used because it is the best criterion in PLS-SEM to assess discriminant validity, and it requires, as appropriate values, numbers under 0.85 (Henseler et al., 2015). The results of the study indicate that both the Fornell and Lacker criteria and the HTMT ratio obtained satisfactory levels (Table 4). Furthermore, the HTMT for HRM and OC was 0.808 [0.702; 0.895], for HRM and IP it was 0.730 [0.612; 0.823], and for OC and IP it was 0.808 [0.694; 0.889]. The HTMT confidence interval did not include 1 (Franke and Sarstedt, 2019).
Structural Model Evaluation
The results of the structural model (Table 5) show that OC has a direct effect on IP (0.467), followed by HRM (0.340). Likewise, the two constructs explain 56.7% of the variance of the endogenous construct IP (R2 = 0.567), as observed in Figure 3. HRM also explains 54.3% of the variance of OC. On the other hand, based on the magnitudes of the path coefficients, all the relationships were statistically significant (Table 5). Regarding effect sizes, Cohen (1988) assessment was performed with values of f2 > 0.02, f2 > 0.15, and f2 > 0.35 that represent small, medium, and large effect sizes, respectively. Results indicate a small effect on H1, a medium effect on H3, and a large effect on H2. In summary, both HRM and OC are moderately strong predictors of IP (Table 5).
In addition, the statistical significance (t = 5.048, p < 0.001) of the indirect effect of HRM on IP through OC (0.344) was observed. From these results, a complementary mediation is given, where both the indirect effect and the direct effects are statistically significant and point in the same direction (Nitzl et al., 2016). That is to say, OC mediates partially and in a complementary way the relationship between HRM on IP.
Finally, the Q2predict indicator was examined to assess the predictive performance of the structural model. If the Q2predict value is positive, the prediction error of the PLS-SEM results is less than the prediction error of simply using the mean values. Q2predict values were interpreted with Hair et al. (2019a) rule of thumb and values of 0.01, 0.25, and 0.50, respectively, show small, medium and high-relevance situations of a model. Besides, a medium relevance for H1 and great relevance for H2 were found (Table 5).
Importance-Performance Map Analysis
In this analysis, the total effects represent the importance of the background indicators and constructs to explain the objective variable (innovative performance), while the mean scores of the variables represent their respective achieved performances (Höck et al., 2010). Results show that HRM has a performance of 55 and OC of 62 (Table 6). The constructs show relatively high performance. HRM expresses greater importance at predicting innovative performance (Table 6).
In Figure 4, the IPMA is presented at the level of indicators seeking to identify relevant and specific areas to improve. In this way, the weights are interpreted as the relative importance of one indicator compared to the other indicators in the measurement model. HRM indicators present a high performance but low importance for IP, so they have a lower priority when looking for improvements in their performance. On the contrary, the OC indicators show greater importance to explain innovation performance, consequently, they are the most relevant indicators to initiate management actions by NPHs.
Conclusion and Recommendations for Future Studies
Based on the analysis of the data, all the hypotheses are supported. Specifically, the influence of HRM over IP is statistically significant. Meanwhile, OC partially mediates this relationship in hospitals working in a non-profit basis. According to this evidence, IP is explained by the strategy to manage the human resources and by the underpinning culture in this type of hospitals.
These findings are evidence to support the knowledge-based theory which means that the theory is also applicable to NPH’s context. As stated by Kang et al. (2007) HRM plays an important role in the value creation process that, in the case of NPH, bears new practices such as process, service or product to improve efficacy of results. Besides, the significance of these relationships is aligned with prior studies that also pointed out the impact of HRM on IP (Adnan et al., 2016; Métailler, 2016; Diaz-Fernandez et al., 2017). Similarly, the relationship among both variables was previously studied and found to be at the other direction which may indicate that both are linked in a cyclic pattern where HRM influence IP and vice versa. Furthermore, this result entitles a chance for NPH’s to pursue innovation and at the same time reach a competitive advantage in the market (Wernerfelt, 1984; Conner, 1991; Grant, 1991; Collis and Montgomery, 1995; Teece et al., 1997).
IP has shown to be significantly influenced by OC according to this study’s results which is also aligned with prior research (Jaskyte, 2018, 2015). Innovation success has been previously associated with OC and organizational innovation capabilities (Long and Fahey, 2000; Leal-Rodríguez et al., 2014). There is evidence that organizational principles and climate are components of organizational culture and have more implications on the innovative processes (Peters and Waterman, 1982; Fondas and Denison, 1991; Ahmed, 1998; Jaskyte, 2004, 2015; Meyer and Leitner, 2018). However, this study did not explore these further dimensions remaining the study of OC as a composite construct for future research.
This study assessed the role of OC as a mediator variable between HRM and IP. This relationship still considered a direct link between these variables, so OC played a partial mediation. The data was actually consistent with these theoretical relationships stated in the first place. That is to say, even the nature of organizations for prior findings regarding this topic, namely private companies, OC behaves similarly in non-profit hospitals. This also supports the positive influence of OC over IP found in non-profit organizations (Jaskyte, 2004, 2011, 2018; Bal et al., 2014; Langer and LeRoux, 2017; Shier et al., 2019). Thus, NPHs outreach a social innovation performance that promotes a more inclusive healthcare and depict good example for other kind of social business models (Vecchio and Rappini, 2011; Michelini, 2012; Angeli and Jaiswal, 2016).
This study found support for the stated hypotheses, however, it also faced some limitations. Regarding the sample group, of the 150 participants, only one is part of a private non-profit hospital which limit the possibility to extent these results to them. Regarding the OC variable, there was not chance to include organizational principles and climate as dimensions to assess whether they influence the most to IP as found for profit organizations. Regarding the methodological limitations of the study, all the variables were measured through a self-report instrument, whose responses could be influenced by personal biases of the workers, especially in variables such as innovative performance, where it is mainly based on the perception of same. Therefore, future studies are needed to clarify these findings.
A favorable methodological aspect of the study was the use of PLS-SEM. Among the advantages of using PLS-SEM is that it allows researchers to estimate complex models with various constructs and indicators, without requiring distributional assumptions of the data (Hair et al., 2017). These methodological advantages are supported by the PLS-SEM own conceptualization, which has a causal-predictive approach (Hair et al., 2019b). PLS-SEM should be considered not only as a less demanding measure than CB-SEM but as a complementary approach in the SEM context. Thus, PLS-SEM can be used in a wide variety of research environments, obtaining high efficiency in parameter estimation, reflected in a greater statistical power of the analysis (Hair et al., 2013).
However, this technique also has some limitations, mainly when seeking to test or confirm theoretical models, because PLS-SEM does not have fully established measures of goodness of fit, although there is a line of research in this regard, with positive results in recent years (Henseler et al., 2014). Therefore, when there is little knowledge about the relationship of the structural model or the characteristics of the measurement model, or when the study focuses more on exploration than confirmation, PLS-SEM is a better alternative than CB-SEM (Hair et al., 2017).
Future studies are advised to be addressed regarding the following points. The relationship between HRM and IP need more clarification as prior studies contradict this study’s findings leaving unsolved the direction of possible influences between these variables. The role and nature of OC because prior literature and the mediator role it has between HRM and IP raise questions about how it behaves as a composite construct and what exactly are its elements, particularly, in the context of NPHs where the mainstream differs from for-profit organizations and such. Finally, further investigations need to explore the empirical indicators of innovative performance, such as the number of patents registered, the number of new services implemented, or others in order to have a better understanding of this endogenous variable and how it is influenced.
Regarding practical implications, this study reflects an opportunity for NPHs to implement an innovative approach regarding their organizational performance. Technology is often related to innovation that demands organizations the investment of money that sometimes is difficult to count on. This is the case of most non-profit organizations such as non-profit hospitals. For this reason, the resources-based theory (Barney, 1991) pushes companies to take advantage of all their resources to earn the expected performance and develop competitive advantages. This view leads to value the HRM as an element to foster the IP, which could be more affordable in a general basis. Particularly, as human resources influence positively the performance of non-profit organizations without much effort to manage them, a reasonable investment on the staff management might be even more beneficial for an innovative performance.
The findings support the hypotheses remaining some aspects to deepen. HRM is a wide element in the organizational performance that is supposed to manage the OC as well as staff training, compensation, promotion and so forth. Similarly, the organizational climate and principles are related to OC and they are worth being included in the model. Broadening the model considering their dimensions might lead to better understand and predict an innovative performance. Furthermore, assessing the model to other non-profit organizations into the health sector that handle tight budgets as well, for instance nursing homes, might be conclusive about the role of HRM to positive influence IP to enhance quality of life overall.
Data Availability Statement
The datasets presented in this article are not readily available because data is the property of a third party. Requests to access the datasets should be directed to OL-M, ohlopezm@ut.edu.co.
Ethics Statement
Ethical review and approval was not required for the study on human participants in accordance with the local legislation and institutional requirements. Written informed consent to participate in this study was provided by the participants.
Author Contributions
JA-P, OL-M, CS-P, and RZ-T contributed to the conception and design of the study, organized the database, performed the statistical analysis, wrote the first draft of the manuscript, and wrote sections of the manuscript. All authors contributed to the manuscript revision, read and approved the submitted version.
Conflict of Interest
The authors declare that the research was conducted in the absence of any commercial or financial relationships that could be construed as a potential conflict of interest.
References
Acosta-Prado, J. C., Romero, A. K., and Tafur-Mendoza, A. A. (2020). Conditions of knowledge management, innovation capability and firm performance in colombian NTBFs: a measurement scale. VINE J. Inform. Knowledge Manag. Syst. doi: 10.1108/VJIKMS-09-2019-0142 [Epub ahead of print].
Adnan, Z., Abdullah, H., and Ahmad, J. (2016). Assessing the moderating effect of competition intensity on HRM practices and organizational performance link: the experience of malaysian r&D companies. Proc. Econ. Finance 35, 462–467. doi: 10.1016/s2212-5671(16)00057-5
Ahmed, P. K. (1998). Culture and climate for innovation. Eur. J. Innov. Manag. 1, 30–43. doi: 10.1108/14601069810199131
Akingbola, K. (2013). Resource-Based View (RBV) of unincorporated social economy organizations. Can. J. Nonprofit Soc. Econ. Res 4, 66–85. doi: 10.22230/cjnser.2013v4n1a133
Aktar, A., and Pangil, F. (2017). The relationship between employee engagement, hrm practices and perceived organizational support: evidence from banking employees. Int. J. Hum. Resour. Stud. 7, 1–22. doi: 10.5296/ijhrs.v7i3.11353
Ali, F., Rasoolimanesh, M., Sarstedt, C., Ringle, S., and Ryu, K. (2018). An assessment of the use of partial least squares structural equation modeling (PLS-SEM) in hospitality research. Int. J. Contemp. Hospital. Manag. 30, 514–538. doi: 10.1108/IJCHM-10-2016-0568
Angeli, F., and Jaiswal, A. (2016). Business model innovation for inclusive health care delivery at the bottom of the pyramid. Organ. Environ. 29, 486–507. doi: 10.1177/1086026616647174
Ariza-Montes, A., and Lucia-Casademunt, A. M. (2016). Nonprofit versus for-profit organizations: a european overview of employees’ work conditions. Hum. Serv. Organ. Manag. Leadersh. Gov. 40, 334–351. doi: 10.1080/23303131.2015.1134742
Atkinson, C., and Lucas, R. (2013). Worker responses to HR practice in adult social Care in England. Hum. Res. Manag. J. 23, 296–312. doi: 10.1111/j.1748-8583.2012.00203.x
Ato, M., López, J., and Benavente, A. (2013). A Classification System for Research Designs in Psychology. Anal. Psicol. 29, 1038–1059. doi: 10.6018/analesps.29.3.178511
Ayob, N., Teasdale, S., and Fagan, K. (2016). How social innovation ‘came to be’: tracing the evolution of a contested concept. J. Soc. Policy 45, 635–653. doi: 10.1017/S004727941600009X
Baines, D. (2010). ‘If We Don’t get back to where we were before’: working in the restructured non-profit social services. Br. J. Soc. Work 40, 928–945. doi: 10.1093/bjsw/bcn176
Bal, Y., Bozkurt, S., and Ertemsir, E. (2014). A study on determining the relationship between strategic HRM practices and creating innovation in organizations. Współczesne Zarza̧dzanie. 13:23.
Ballesteros-Rodríguez, J. L., de Saá-Pérez, P., and Domínguez-Falcón, C. (2012). The role of organizational culture and HRM on training success: evidence from the canarian restaurant industry. Int. J. Hum. Resour. Manag. 23, 1–18. doi: 10.1080/09585192.2011.637071
Baluch, A. M. (2017). Employee perceptions of HRM and well-being in nonprofit organizations: unpacking the unintended. Int. J. Hum. Resour. Manag. 28, 1912–1937. doi: 10.1080/09585192.2015.1136672
Barney, J. (1991). Firm resources and sustained competitive advantage. J. Manag. 17, 99–120. doi: 10.1177/014920639101700108
Brandsen, T., Evers, A., Cattacin, S., and Zimmer, A. (2016). “Social innovation: a sympathetic and critical interpretation,” in Social Innovations in the Urban Context. Nonprofit and Civil Society Studies (An International Multidisciplinary Series), eds T. Brandsen, S. Cattacin, A. Evers, and A. Zimmer (Cham: Springer), doi: 10.1007/978-3-319-21551-8_1.
Brimhall, K. C. (2019). Inclusion and commitment as key pathways between leadership and nonprofit performance. Nonprofit Manag. Leadersh. 30, 31–49. doi: 10.1002/nml.21368
Brown, W., Andersson, F., and Suyeon, J. (2016). Dimensions of capacity in nonprofit human service organizations. Voluntas. 27, 2889–2912. doi: 10.1007/s11266-015-9633-8
Christensen, C. M., Raynor, M., Rory, M., and McDonald, R. (2015). What is disruptive innovation? Harvard Bus. Rev. 33, 7–8. doi: 10.1353/abr.2012.0147
Cohen, J. (1988). Statistical Power Analysis for the Behavioral Sciences. 2nd Edn. Hillsdale, NJ: Lawrence Erlbaum Associates.
Colbert, B. A. (2004). The complex resource-based view: implications for theory and practice in strategic human resource management. Acad. Manag. Rev. 29, 341–358. doi: 10.5465/AMR.2004.13670987
Collis, D. J., and Montgomery, C. (1995). Competing on resources: strategy in the 1990s competing on resources? Harvard Bus. Rev. 77, 118–128.
Conner, K. R. (1991). A historical comparison of resource-based theory and five schools of thought within industrial organization economics: do we have a new theory of the firm? J. Manag. 17, 121–154. doi: 10.1177/014920639101700109
Diaz-Fernandez, M., Bornay-Barrachina, M., and Lopez-Cabrales, A. (2017). HRM practices and innovation performance: a panel-data approach. Int. J. Manpower 38, 354–372. doi: 10.1108/IJM-02-2015-0028
Echevarría, J. (2008). The Oslo manual and the social innovation. Arbor 184:732. doi: 10.3989/arbor.2008.i732.210
Faul, F., Erdfelder, E., Buchner, A., and Lang, A.-G. (2009). Statistical power analyses using GPower 3.1: tests for correlation and regression analyses. Behav. Res. Methods 41, 1149–1160. doi: 10.3758/BRM.41.4.1149
Faulkner, A., and Kent, J. (2001). Innovation and regulation in human implant technologies: developing comparative approaches. Soc. Sci. Med. 53, 895–913. doi: 10.1016/S0277-9536(00)00389-0
Fondas, N., and Denison, D. R. (1991). Corporate Culture and Organizational Effectiveness. Hoboken, NJ: Wiley, doi: 10.2307/258613.
Fornell, C., and Larcker, D. (1981). Evaluating structural equation models with unobservable variables and measurement error. J. Mark. Res. 18, 39–50. doi: 10.2307/3151312
Franke, G., and Sarstedt, M. (2019). Heuristics versus statistics in discriminant validity testing: a comparison of four procedures. Internet Res. 29, 430–447. doi: 10.1108/IntR-12-2017-0515
George, G., Rao-Nicholson, R., Corbishley, C., and Bansal, R. (2015). Institutional entrepreneurship, governance, and poverty: insights from emergency medical response servicesin India. Asia Pacific J. Manag. 32, 39–65. doi: 10.1007/s10490-014-9377-9
Gile, P. P., Buljac-Samardzic, M., and De Klundert, J. (2018). The effect of human resource management on performance in hospitals in sub-saharan africa: a systematic literature review. Hum. Resour. Health 16:34. doi: 10.1186/s12960-018-0298-4
Grant, R. M. (1991). The resource-based theory of competitive advantage: implications for strategy formulation. California Manag. Rev. 33, 114–135. doi: 10.2307/41166664
Gurrutxaga, A. (2011). Condiciones y condicionamientos de La innovación social. Arbor 184, 1045–1064. doi: 10.3989/arbor.2011.752n6003
Hair, J. F., Black, W., Babin, B., and Anderson, R. (2019a). Multivariate Data Analysis. 8th Edn. Hampshire: Cengace Learning.
Hair, J. F., Hult, G. T. M., Ringle, C. M., and Sarstedt, M. (2017). A Primer on Partial Least Squares Structural Equation Modeling (PLS-SEM). 2nd Edn. Thousand Oaks, CA: Sage.
Hair, J. F., Ringle, C. M., and Sarstedt, M. (2011). PLS-SEM: Indeed a Silver Bullet. J. Mark. Theor. Pract. 19, 139–152. doi: 10.2753/MTP1069-6679190202
Hair, J. F., Ringle, C. M., and Sarstedt, M. (2013). Partial least squares structural equation modeling: rigorous applications, better results and higher acceptance. Long Range Plan. 46, 1–12. doi: 10.1016/j.lrp.2013.01.001
Hair, J. F., Risher, J. J., Sarstedt, M., and Ringle, C. M. (2019b). When to use and how to report the results of PLS-SEM. Eur. Bus. Rev. 31, 2–24. doi: 10.1108/EBR-11-2018-0203
Hartog, D., Den, N., and Verburg, R. M. (2004). High performance work systems, organisational culture and firm effectiveness. Hum. Resour. Manag. J. 14, 55–78. doi: 10.1111/j.1748-8583.2004.tb00112.x
Henseler, J., Dijkstra, T., Sarstedt, M., Ringle, C. M., Diamantopoulos, A., Straub, D. W., et al. (2014). Common beliefs and reality about PLS: comments on rönkkö and evermann (2013). Organ. Res. Methods 17, 182–209. doi: 10.1177/1094428114526928
Henseler, J., Ringle, C. M., and Sarstedt, M. (2015). A new criterion for assessing discriminant validity in variance-based structural equation modeling. J. Acad. Mark. Sci. 43, 115–135. doi: 10.1007/s11747-014-0403-8
Henseler, J., Ringle, C., and Sinkovics, R. (2009). “The use of partial least squares path modeling in international marketing,” in New Challenges to International Marketing, eds R. Sinkovics and P. Ghauri (Bingley: Emerald Group Publishing Limited), doi: 10.1108/S1474-797920090000020014.
Höck, C., Ringle, C. M., and Sarstedt, M. (2010). Management of multi-purpose stadiums: importance and performance measurement of service interfaces. Int. J. Serv. Technol. Manag. 14, 188–207. doi: 10.1504/IJSTM.2010.034327
Hwang, J., and Christensen, C. M. (2008). Disruptive innovation in health care delivery: a framework for business-model innovation. Health Affairs 27, 1329–1335. doi: 10.1377/hlthaff.27.5.1329
Jarvis, C. B., MacKenzie, S. B., and Podsakoff, P. M. (2003). A critical review of construct indicators and measurement model misspecification in marketing and consumer research. J. Cons. Res. 30, 199–218. doi: 10.1086/376806
Jaskyte, K. (2004). Transformational leadership, organizational culture, and innovativeness in nonprofit organizations. Nonprofit Manag. Leadersh. 15, 153–168. doi: 10.1002/nml.59
Jaskyte, K. (2011). Predictors of administrative and technological innovations in nonprofit organizations. Public Admin. Rev. 71, 77–86. doi: 10.1111/j.1540-6210.2010.02308.x
Jaskyte, K. (2015). Board of directors and innovation in nonprofit organizations model: preliminary evidence from nonprofit organizations in developing countries. Voluntas 26, 1920–1943. doi: 10.1007/s11266-014-9505-7
Jaskyte, K. (2018). Board attributes and processes, board effectiveness, and organizational innovation: evidence from nonprofit organizations. Voluntas 29, 1098–1111. doi: 10.1007/s11266-017-9945-y
Jaskyte, K. (2020). Technological and organizational innovations and financial performance: evidence from nonprofit human service organizations. Voluntas 31, 1–11. doi: 10.1007/s11266-019-00191-8
Jaskyte, K., and de Riobó, R. M. (2004). Characteristics of innovative nonprofit organizations in Argentina. Voluntas 15, 71–79. doi: 10.1023/B:VOLU.0000023634.07861.70
Jaskyte, K., and Dressler, W. (2005). Organizational culture and innovation in nonprofit human service organizations. Admin. Soc. Work 29, 23–41. doi: 10.1300/J147v29n02_03
Jiang, J., Wang, S., and Zhao, S. (2012). Does HRM facilitate employee creativity and organizational innovation? a study of chinese firms. Int. J. Hum. Resour. Manag. 23, 1–23. doi: 10.1080/09585192.2012.690567
Jiménez-Jiménez, D., and Sanz-Valle, R. (2011). Innovation, organizational learning, and performance. J. Bus. Res. 64, 408–417. doi: 10.1016/j.jbusres.2010.09.010
Kang, S. C., Morris, S. S., and Snell, S. A. (2007). Relational archetypes, organizational learning, and value creation: extending the human resource architecture. Acad. Manag. Rev. 32, 236–256. doi: 10.5465/amr.2007.23464060
Kerlinger, F. N., and Lee, H. B. (2000). Foundations of Behavioral Research, 4th Edn. Fort Worth, TX: Harcourt College Publishers.
Kusluvan, S., Kusluvan, Z., Ilhan, I., and Buyruk, L. (2010). The human dimension: a review of human resources management issues in the tourism and hospitality industry. Cornell Hospital. Q. 51, 171–214. doi: 10.1177/1938965510362871
Langer, J., and LeRoux, K. (2017). Developmental culture and effectiveness in nonprofit organizations. Public Perform. Manag. Rev. 40, 457–479. doi: 10.1080/15309576.2016.1273124
Leal-Rodríguez, A. L., Ariza-Montes, J., Roldán, J., and Leal-Millán, A. G. (2014). Absorptive capacity, innovation and cultural barriers: a conditional mediation model. J. Bus. Res. 67, 763–768. doi: 10.1016/j.jbusres.2013.11.041
Li, Y., Zhao, Y., and Liu, Y. (2006). The relationship between HRM, technology innovation and performance in China. Int. J. Manpower 27, 679–697. doi: 10.1108/01437720610708284
Liao, K. -H., and Huang, I. -S. (2016). Impact of vision, strategy, and human resource on nonprofit organization service performance. Proc. Soc. Behav. Sci. 224, 20–27. doi: 10.1016/j.sbspro.2016.05.395
Long, D., and Fahey, L. (2000). Diagnosing cultural barriers to knowledge management. Acad. Manag. Exec. 14, 113–127. doi: 10.5465/ame.2000.3979820
López, O. H., Villegas, H. C., and Cantú-Mata, J. L. (2018a). Instrument to determine the predictors of innovation capacities in the context of health organizations. assessment of your reliability. Espacios 39:28.
López, O. H., Villegas, H. C., and Catica, J. R. (2018b). Validity of the instrument to determine the triggers of innovation capabilities in health care organizations. Gaceta Méd. Caracas 126, 160–169.
Mateos-Aparicio, G. (2011). Partial Least Squares (PLS) methods: origins, evolution, and application to social sciences. Commun. Stat. Theor. Methods 40, 2305–2317. doi: 10.1080/03610921003778225
McDonald, R. E. (2007). An investigation of innovation in nonprofit organizations: the role of organizational mission. Nonprofit Voluntary Sec. Q. 36, 256–281. doi: 10.1177/0899764006295996
Mesch, D. J. (2010). Management of human resources in 2020: the outlook for nonprofit organizations. Public Admin. Rev. 70, s173–s174. doi: 10.1111/j.1540-6210.2009.02124.x
Métailler, T. (2016). The role of the HRM in the construction of KM for the innovation in technological SMEs. Electr. J. Knowledge Manag. 14:772.
Meyer, M., and Leitner, J. (2018). Slack and innovation: the role of human resources in nonprofits. Nonprofit Manag. Leadersh. 29, 181–201. doi: 10.1002/nml.21316
Michelini, L. (2012). Social Innovation and New Business Models. Berlin: Springer, doi: 10.1007/978-3-642-32150-4.
Moral, J. (2019). Revisión de los criterios para validez convergente estimada a través de la varianza media extraída. Psychologia 13, 25–41. doi: 10.21500/19002386.4119
Narapareddy, V., and Berte, E. (2019). Entrepreneurship in a Non-Profit Healthcare Organization. Entrepreneursh. Educ. Pedag. 2, 123–132. doi: 10.1177/2515127418805207
Nickson, D., Warhurst, C., Dutton, E., and Hurrell, S. (2008). A job to believe in: recruitment in the scottish voluntary sector. Hum. Resour. Manag. J. 18, 20–35. doi: 10.1111/j.1748-8583.2007.00056.x
Nigel, S. (1994). The importance-performance matrix as a determinant of improvement priority. Int. J. Operat. Product. Manag. 14, 59–75. doi: 10.1108/01443579410056803
Nitzl, C., Roldán, J. L., and Cepeda, G. (2016). Mediation analysis in partial least squares path modeling: helping researchers discuss more sophisticated models. Indus. Manag. Data Syst. 116, 1849–1864. doi: 10.1108/IMDS-07-2015-0302
Nunnally, J. C., and Bernstein, I. H. (1994). Psychometric Theory. 3rd ed. New York, NY: McGraw-Hill.
OECD/Eurostat (2005). Oslo Manual: Guidelines for Collecting and Interpreting Innovation Data, 3rd edition, the Measurement of Scientific and Technological Activities Communities. Paris: OECD. doi: 10.1787/9789264013100-en
Oliveira, S. B., and Toda, F. A. (2013). O Planejamento estratégico e a visão baseada Em Recursos (RBV): Uma Avaliação Da Tecnologia Da Informação Na Gestão Hospitalar. Rev. Eletr. Ciência Admin. 12, 39–57. doi: 10.5329/recadm.2013006
Omachonu, V. K., and Einspruch, N. (2010). Innovation in healthcare delivery systems: a conceptual framework. Innovat J. 15, 1–20.
Peters, T., and Waterman, R. H. Jr. (1982). “Search of excellence: simultaneous loose-tight properties,” in Search of Excellence: Lessons from America’s Best-Run Companies, (Manhattan, NY: Harper & Row).
Phillips, W., Lee, H., Ghobadian, A., O’Regan, N., and James, P. (2015). Social innovation and social entrepreneurship: a systematic review. Group Organ. Manag. 40, 428–461. doi: 10.1177/1059601114560063
Prada-Ríos, S. I., Pérez-Castaño, A. M., and Rivera-Triviño, A. F. (2017). Classification of health services providing institutions according to the system of health accounts of the organization for economic cooperation and development: the Colombia case. Rev. Gerencia Polit. Salud 16, 51–65. doi: 10.11144/Javeriana.rgps16-32.cips
Rigdon, E. E. (2012). Rethinking partial least squares path modeling: in praise of simple methods. Long Range Plan. 45, 341–358. doi: 10.1016/j.lrp.2012.09.010
Sanzo-Perez, M. J., Álvarez-González, L., and Rey-García, M. (2015). How to encourage social innovations: a resource-based approach. Serv. Indus. J. 35, 430–447. doi: 10.1080/02642069.2015.1015517
Sarstedt, M., and Mooi, E. (2019). A Concise Guide to Market Research: The Process, Data, and Methods Using IBM SPSS Statistics. 3rd Edn. Berlin: Springer-Verlag Berlin Heidelberg. doi: 10.1007/978-3-662-56707-4.
Shier, M. L., Handy, F., and Jennings, C. (2019). Intraorganizational conditions supporting social innovations by human service nonprofits. Nonprofit Voluntary Sec. Q. 48, 173–193. doi: 10.1177/0899764018797477
Streukens, S., and Leroi-Werelds, S. (2016). Bootstrapping and PLS-SEM: a step-by-step guide to get more out of your bootstrap results. Eur. Manag. J. 34, 618–632. doi: 10.1016/j.emj.2016.06.003
Svensson, G., Mahoney, T., and Hambrick, M. (2019). What does innovation mean to nonprofit practitioners? International insights from development and peace-building nonprofits. Nonprofit Voluntary Sec. Q. 49, 380–398. doi: 10.1177/0899764019872009
Teece, D. J., Pisano, G., and Shuen, A. (1997). Dynamic capabilities and strategic management. Strat. Manag. J. 18, 509–533. doi: 10.1002/(SICI)1097-0266(199708)18:7<509::AID-SMJ882<3.0.CO;2-Z
Vecchio, M. D., and Rappini, V. (2011). “Low cost in sanità,” in Rapporto OASI 2011. L’aziendalizzazione Della Sanità in Italia, Ed. E. Cantù (Milan: Egea).
Wernerfelt, B. (1984). A resource-based view of the firm. Strat. Manag. J. 5, 171–180. doi: 10.1002/smj.4250050207
Keywords: human resource management, innovative performance, organizational culture, PLS-SEM, non-profit hospitals
Citation: Acosta-Prado JC, López-Montoya OH, Sanchís-Pedregosa C and Zárate-Torres RA (2020) Human Resource Management and Innovative Performance in Non-profit Hospitals: The Mediating Effect of Organizational Culture. Front. Psychol. 11:1422. doi: 10.3389/fpsyg.2020.01422
Received: 26 March 2020; Accepted: 27 May 2020;
Published: 19 June 2020.
Edited by:
Antonio Ariza-Montes, Universidad Loyola Andalucía, SpainReviewed by:
Cristian Geldes, Alberto Hurtado University, ChileJhon Wilder Zartha Sossa, Pontificia Universidad Javeriana Cali, Colombia
Copyright © 2020 Acosta-Prado, López-Montoya, Sanchís-Pedregosa and Zárate-Torres. This is an open-access article distributed under the terms of the Creative Commons Attribution License (CC BY). The use, distribution or reproduction in other forums is permitted, provided the original author(s) and the copyright owner(s) are credited and that the original publication in this journal is cited, in accordance with accepted academic practice. No use, distribution or reproduction is permitted which does not comply with these terms.
*Correspondence: Carlos Sanchís-Pedregosa, c.sanchisp@up.edu.pe; csanchis@us.es