- 1Bilingual Cognition and Development Lab, Center for Linguistics and Applied Linguistics, Guangdong University of Foreign Studies, Guangzhou, China
- 2Center for Brain Disorders and Cognitive Science, Shenzhen University, Shenzhen, China
- 3Center for Language and Brain, Shenzhen Institute of Neuroscience, Shenzhen, China
The neural basis of dyslexia in different languages remains unresolved, and it is unclear whether the phonological deficit as the core deficit of dyslexia is language-specific or universal. The current functional magnetic resonance imaging (fMRI) study using whole-brain data-driven network analyses investigated the neural mechanisms for phonological and orthographic processing in Chinese children with good and poor reading ability. Sixteen good readers and 16 poor readers were requested to make homophone judgments (phonological processing) and component judgments (visual-orthographic processing) of presented Chinese characters. Poor readers displayed worse performance than the good readers in phonological processing, but not in orthographic processing. Whole-brain activation analyses showed compensatory activations in the poor readers during phonological processing and automatic phonological production activation in the good readers during orthographic processing. Significant group differences in the topological properties of their brain networks were found only in orthographic processing. Analyses of nodal degree centrality and betweenness centrality revealed significant group differences in both phonological and orthographic processing. The present study supports the phonological core deficit hypothesis of reading difficulty in Chinese. It also suggests that Chinese good and poor readers might recruit different strategies and neural mechanisms for orthographic processing.
Introduction
Developmental dyslexia, or in short, dyslexia, is characterized by a severe reading acquisition disorder that cannot be explained by general intelligence impairment, lack of education opportunities, or any sensory or neurological disorders (American Psychiatric Association, 2013). It is a widespread reading disorder that affects word recognition, decoding, and spelling abilities in 5–17% of the population, regardless of cultural or language backgrounds (Shaywitz et al., 1998; Ziegler et al., 2003; Siok et al., 2008; Cao et al., 2017). Phonological deficits, including impaired phonological representation and speech sound processing, are presented in the majority of dyslexics (Ziegler and Goswami, 2005) and therefore the phonological deficit hypothesis has been the most popular hypothesis about the cause of dyslexia (Rack et al., 1992; Pennington and Lefly, 2001; for a recent review, see Paulesu et al., 2014). This hypothesis posits that dyslexics are impaired in their phonological representation and their ability to process and manipulate speech sounds (e.g., Shankweiler and Lundquist, 1992; Ziegler and Goswami, 2005), which adversely affects the development of mapping between written forms (graphemes) and speech sound (phonemes) and hinders reading development (Snowling, 1981, 1998; Muter et al., 1998; Ramus and Szenkovits, 2008; Hulme et al., 2012; Castles and Friedmann, 2014).
Phonological and Orthographic Deficits in Dyslexia
There is a tremendous amount of research on the brain mechanism of phonological processing deficits in dyslexics, and how such deficits affect reading development and might be relieved by phonological training (e.g., Shaywitz et al., 1998; Brunswick et al., 1999; Demb et al., 1999; Temple et al., 2001; Gaab et al., 2007; Cao et al., 2008; Tanaka et al., 2011; Steinbrink et al., 2012; Zhang et al., 2018). Most of the neuroimaging studies to date have investigated neural mechanism of dyslexia using visual word/pseudoword tasks and found reduced brain activation in the left temporo-parietal and temporo-occipital region in dyslexics speaking alphabetic languages (e.g., Rumsey et al., 1997; Paulesu et al., 2001, 2014; Schulz et al., 2009; van der Mark et al., 2009; Desroches et al., 2010; Pecini et al., 2011; Tanaka et al., 2011). The activation of the left inferior frontal gyrus (IFG) in dyslexics, however, increased in some studies (Shaywitz et al., 1998; Hoeft et al., 2007; MacSweeney et al., 2009) and decreased in other studies (Brambati et al., 2006; Cao et al., 2006; Booth et al., 2007; Wimmer and Schurz, 2010). Richlan et al. (2011) in a meta-analysis examined left temporo-parietal dysfunction for phonological deficits in dyslexic children and left ventral temporo-occipital dysfunction for visual-orthographic deficit in dyslexic adults. They found decreased activation of left ventral temporo-occipital region only in dyslexic adults.
Phonological deficits, however, are not the only problem in dyslexia. For example, Denckla and Rudel (1976) first reported picture naming problems in many people with dyslexia, who were slower than the normal when asked to rapidly name visual stimuli (for an overview, see Wolf et al., 2000). Wolf and Bowers, therefore, developed the double deficit hypothesis, which postulates that some people with dyslexia had a second independent naming speed deficit, which causes slower cross-modal matching of visual symbols and phonological codes, and therefore also causes reading problems (e.g., Bowers and Wolf, 1993; Wolf and Bowers, 1999; Vaessen et al., 2009).
Dyslexia is also suggested to be associated with orthographic deficits. First, rapid naming deficits seems to be quite universal among dyslexics in many languages, but phonological awareness deficit, difficulty to recognize and work with sounds in spoken language, are more common in opaque alphabetic languages (e.g., English) than transparent alphabetic languages (e.g., Italian) or non-alphabetic languages (e.g., Chinese) (e.g., Huang and Hanley, 1995; Ho et al., 2002; Ziegler et al., 2003; Tan et al., 2005; Vidyasagar and Pammer, 2010). Secondly, dyslexics exhibit deficits in processing letter strings in tasks with minimal phonological or lexical involvement, such as searching for a target letter in a string of consonants (e.g., Hawelka et al., 2006; Bosse et al., 2007; Collis et al., 2013). Ziegler et al. (2010) reported that dyslexics performed significantly worse than age-matched controls with letter and digit strings but not with symbol strings. The authors suggest that these deficits cannot be explained by weak reading experience in dyslexics, or dysfunctional visual attention processing, and reflect a deficit in processing a string of letters in parallel, probably due to difficulty in the coding of letter position. Finally, some neuroimaging studies have also found dyslexics show less activation than the normals in left fusiform gyrus, a system specialized for processing the orthographic structure of well-learned visual word forms (Rumsey et al., 1997; Brunswick et al., 1999; Temple et al., 2001; Shaywitz and Shaywitz, 2003; Cao et al., 2006; van der Mark et al., 2009; Boros et al., 2016). For example, Desroches et al. (2010) reported reduced brain activation in the fusiform gyrus in dyslexics compared with the normals during an auditory rhyming task. The brain activation in left fusiform gyrus of the dyslexics correlated significantly and positively with their non-word reading performance. The authors, therefore, suggest that dyslexics were impaired in the access to orthography and the integration of orthographic and phonological processing.
The dysfunction activation of fusiform gyrus may be secondary to a primary dysfunction of the temporo-parietal region (Boros et al., 2016). Orthographic deficits in dyslexics increase the difficulty of selecting graphemes in fusiform gyrus, which are the input to the grapheme-phoneme processing and phonological decoding system in the temporo-parietal region. Therefore dyslexia might be characterized by the co-existence of orthographic and phonological processing difficulties (Siok et al., 2009).
Dyslexia in Chinese
Siok et al. (2004) found that Chinese dyslexic children reading in Chinese did not show underactivation in the left temporo-parietal regions as typically shown in studies of alphabetic languages. They reported reduced activity at Brodmann’ area (BA) 9 in the left middle frontal gyrus (MFG), an area involved in syllabic processing of phonology (Siok et al., 2003). This study provides the first neural evidence to support previous findings of phonological awareness predicting reading development of Chinese children (e.g., McBride-Chang et al., 2008; Pan et al., 2011, 2015) and impaired phonological awareness in Chinese dyslexic children (e.g., Ho and Lai, 1999; Ziegler and Goswami, 2005), but also challenges the biological unity of dyslexia.
Unlike alphabetic languages, Chinese is a logographic language, in which the basic orthographic units, the characters, map onto morphemic meanings and to monosyllables with Chinese four tones in the spoken language. Therefore, Chinese reading needs a fine-grained visuospatial analysis to access characters’ phonology and meaning. Chinese readers must learn the character phonology at the syllabic level as a whole by rote, and they might need additional strategies like writing to learn those characters (Siok et al., 2004; Tan et al., 2005; Ziegler, 2006; Cao et al., 2013; cf. Bi et al., 2009).
Siok et al. (2009) compared Chinese dyslexic children and normal children in a decision task of Chinese character physical size. The normal showed greater activation than the dyslexic in the right inferior parietal lobe; the dyslexics, however, had more neural response than the normal participants in left inferior parietal lobe and lingual gyrus subserving visual analysis. According to the authors, phonological and orthographic disorders co-exist in the majority (83.33%) of Chinese dyslexics. The findings of Siok et al. (2009) are congruent with earlier behavioral reports of visual-orthographic deficits in Chinese dyslexics (Huang and Hanley, 1995; Ho et al., 2002).
Hu et al. (2010) examined brain activations of Chinese dyslexics, English dyslexics, English normal readers, and Chinese normal readers in a semantic decision task on written words. They found Chinese and English dyslexic adolescents had common underactivation than their normal controls in the left angular gyrus, left middle frontal, posterior temporal, and occipito-temporal regions. The authors suggest commonalities of manifestation of dyslexia in Chinese and English population, which could be influenced by readers’ cognitive ability and learning environment, as is congruent with Ziegler’s claim on the universal phonological core deficit of dyslexia (Ziegler, 2006).
Brain Connectivity in Dyslexia
A significant trend in cognitive neuroscience today is the brain connectivity approach, which explores the functional or structural connectivity patterns of brain regions that support cognitive or linguistic processing. A few studies have adopted this approach toward dyslexia.
In their pioneer work on dyslexia and connectivity, Horwitz et al. (1998), using positron emission tomography (PET) found that the dyslexics’ left angular gyrus is functionally disconnected from the extrastriate occipital and temporal lobe regions during single-word reading, compared with the normal adults. They suggest a disconnected brain network in dyslexia. More recently, Boets et al. (2013) examined whether dyslexics’ phonological deficits are caused by impaired phonological representation or by dysfunctional retrieval of phonological representations. They found that adult dyslexics have intact phonetic representations. Their functional and structural connectivity between the bilateral auditory cortices and the left IFG, however, is significantly smaller than the normal adults. Cao et al. (2017) focused on the phonological deficits of Chinese dyslexic children, who were asked to perform an auditory rhyming judgment task. They found that Chinese dyslexics were impaired in the left dorsal IFG and they had more reliance on the right precentral gyrus than the normal controls as a compensatory strategy. Their functional connectivity analyses showed that connectivity between the left STG and the left dorsal IFG was sensitive to task performance and/or reading skill rather than being dyslexic or not. In a functional connectivity study of orthographic processing of dyslexia, van der Mark et al. (2009) focused on the role of the left visual word form area in temporo-occipital area and found a significant disruption of the functional connectivity between the visual word face area (VWFA) and left inferior frontal and left inferior parietal language areas in the dyslexic children. They suggest that dyslexia is associated with impaired automatic visual word processing, along with deficits in orthographic and phonological processing. The studies mentioned above were based on the analysis of regions of interests (ROIs), and therefore their results depend on the selected regions, which are arbitrary decisions by the authors. Finn et al. (2014) adopted a whole-brain, data-driven analysis to examine the functional networks in dyslexics. They found reduced connectivity in the visual word-form areas and increased right-hemisphere connectivity in the dyslexics compared with the normal adults. However, the parcellations in both the younger reader and older reader groups were generated from their groups of normal participants with limited group size (30–45). Their data analysis focused on group differences in regional connectivity and did not compare the topological features of brain networks.
The Present Study
The present functional magnetic resonance imaging (fMRI) study investigated phonological processing and orthographic processing in Chinese children with good and poor reading ability to improve the current understanding of the universal neural mechanism for dyslexia. All participants were asked to perform a homophone judgment task (phonological processing) and a component search task (visual-orthographic processing) inside the fMRI scanner. We examined group differences in their whole-brain activation and analyzed the topological features of their functional brain networks to reveal the neural mechanisms for phonological and visual-orthographic processing in Chinese good and poor readers.
Materials and Methods
Participants
Five hundred and twenty-four 4th and 5th graders from the Beijing Yongtai Primary School in China participated in the screening for good readers and poor readers. Since there was no standardized dyslexia screening assessment or Chinese reading ability test in mainland China, we measured the children’s reading ability using a character-reading test, their Chinese teachers’ evaluation, and their school performance in the Chinese language course. This character-reading test was adapted from the reading test to evaluate Chinese children’s reading ability by Tan et al. (2005), comprised 120 Chinese characters from the textbooks for third to fifth graders (40 characters for each grade) and 40 characters beyond the primary school textbooks. The 160 characters were printed on a standard A4 sheet, listed in 16 rows and 10 columns, and arranged from easy to difficult based on grade level. Each participant was asked to read out the 160 characters as accurately and as fast as possible with a time limit of 90 s. Their name accuracy (number of characters correctly named) represented their reading performance: Poor readers had reading scores 1.5 standard deviations below the mean; good readers had reading scores 1.5 standard deviations above the mean. Their reading performance was congruent with the evaluation from their Chinese teachers and their school performance in the Chinese course. Seventeen children with dyslexia and 16 controls participated in the present fMRI study. One participant from the normal group was excluded because of neurological disease found during the fMRI scans.
The reading performance (Mean ± SD = 115.75 ± 13.57) of the 16 participants in the normal group (9 men, average age = 10 years 1 month) was significantly better than that (Mean ± SD = 35.63 ± 13.59) of the 16 participants in the dyslexic group (12 men, average age = 10 years 6 months), t30 = 16.69, p < 0.001. All participants, who were native speakers of Chinese and right-handed (Oldfield, 1971), had average and matched non-verbal intelligence according to their performance in the Raven’s Progressive Matrices (Raven et al., 1998) (good readers, Mean ± SD = 68.44 ± 15.78; poor readers, Mean ± SD = 75 ± 16.73; t30 = −1.141, p = 0.26). This fMRI study was approved by the Beijing Institutional Review Board at the Chinese Academy of Sciences. Written informed consent was obtained from each child and his/her legal guardians, mostly their parents.
Stimuli and Procedure
In this blocked-design fMRI study, both groups underwent a phonological session and a visual-orthographic session. During the phonological session, participants performed a homophone judgment task in experimental blocks: they were asked to judge whether the characters (e.g., “” sounds/yan2/and means “salt”) presented had the same pronunciation including tones with the “pinyin1” (e.g., “yán” sounds/yan2/) specified at the instruction page before each experimental block. During the visual-orthographic session, participants completed a component judgment task: they were asked to identify whether the characters (e.g., “
” sounds/shu1/and means “uncle”) presented contained a radical (e.g., “
”) specified at the instruction page before each experimental block. Chinese orthographic processing involves visuospatial analysis of Chinese characters and the application of orthographic rules (orthographic awareness). Component search task (orthographic search) asks participants to judge whether a character contained a designated a radical component and has been used as Chinese visual-orthographic processing task in previous studies (e.g., Siok and Fletcher, 2001; Ding et al., 2003).
Both sessions included four experimental blocks (homophone judgment/component search): each block began with a 2-s instruction and included eight trials; each trial started with a 500-ms presentation of Chinese character at the center of the screen, followed by a 2500-ms blank screen for responses. All the experimental blocks were interleaved with 12-s fixation blocks. Participants made “Yes” or “No” responses by clicking right or left buttons with their index fingers on a control box compatible with the fMRI scanner. The Chinese character stimuli, selected from the children’s textbooks, were matched between experimental tasks in terms of character frequency and visual complexity (strokes).
MRI Acquisition
MRI images were acquired on a Siemens Vision Magnetom 3.0-tesla scanner with a circularly polarized head coil at the Beijing MRI Imaging Center. Before the fMRI scans, all participants underwent a practice session and were visually familiarized with all the procedures and experimental conditions. They lay supine in the scanner with plastic ear-canal molds and looked up through a prism at a screen at the end of the scanner, while their heads were immobilized by a tightly fitting, vacuum pillow. A T2∗-weighted gradient-echo planar imaging (EPI) sequence was used for fMRI scans: slice thickness = 4 mm, in-plane resolution = 3.125 × 3.125 mm2, and TR/TE/flip angle = 2000 ms/30 ms/90°. The field of view (FOV) was 200 × 200 mm2, and the acquisition matrix was 64 × 64. Thirty-two contiguous axial slices were acquired parallel to the anterior commissure–posterior commissure (AC–PC) line covering the whole brain.
Data Analyses
Whole-Brain Activations
SPM 12 was used for image preprocessing and statistical analyses2. Functional images from each participant were realigned and normalized to an EPI template based on the ICBM152 stereotactic space, an approximation of canonical space (Talairach and Tournoux, 1988). The images were further re-sampled into 3 mm × 3 mm × 3 mm cubic voxels and spatially smoothed with an isotropic Gaussian kernel (6 mm full width at half-maximum). After motion-correction, the first three images (dummy images), corresponding to the period of transient hemodynamic change that occurred before the experimental trials, were discarded. The general linear model included 12 motion regressors was used to estimate the condition effect of each individual, while boxcar convolved with the canonical hemodynamic response function was selected as a reference function. Adjusted mean images were created for each condition after removing global signal and low-frequency covariates, using a high-pass filter with a cut-off of 128 s. Contrast images of homophone judgment minus fixation in phonological scanning session and component judgment minus fixation in visual-orthographic session were computed, using a Student’s group t-test, which generated the statistical parametric maps of t-values. For each session, all the contrast estimates from dyslexic and normal groups were entered into a standard SPM second-level analysis with subjects treated as a random effect, using two-samples T-test to examine possible group differences in brain activations.
All the brain activations reported below were in MNI coordinate space and survived a corrected cluster-level threshold of p < 0.05 (single voxel p = 0.005, 10000 simulations, and a minimum cluster size of 25 voxels) using AlphaSim program in REST software (Song et al., 2011).
Network Construction
Functional brain networks for good readers and poor readers were constructed at the macroscale in which nodes represent brain regions, and edges present the statistical relationships of blood oxygenation level-dependent (BOLD) signals across different regions. Here, we used the 90 regions (45 for each hemisphere) of the atlas of Automated Anatomical Labeling (AAL) (Tzourio-Mazoyer et al., 2002) as nodes of the brain network. The averaged time series of all the voxels within each ROI was extracted in each individual. Edges, or interregional functional connectivity, were calculated using Pearson correlations between these regional task-related time series of all possible pairs of the 90 regions for each participant. The correlation coefficients were then transformed to z-scores via Fisher’s transformation to improve normality (Lowe et al., 1998). Thus each participant has a 90 × 90 correlation matrix for phonological and visual-orthographic sessions, respectively.
Network Analysis
Threshold selection
We constructed binary undirected functional networks using a sparsity threshold (5% ≤ sparsity ≤ 50%, interval = 5%) to comprehensively estimate topological properties covering a wide range of sparsity and remove spurious edges as much as possible (Yang et al., 2017; Zhu et al., 2017). Because the physiological interpretation of negative correlations is ambiguous (e.g., Murphy and Fox, 2017), functional connections with negative correlation values were not considered in the present analysis.
Network metrics
Our network analyses were performed in the GRETNA toolbox (Wang et al., 2015). We calculated both the global and node network metrics at each sparsity. These metrics included: (1) The “small-world” parameters of clustering coefficient (Cp), shortest path length (Lp), normalized clustering coefficient (γ), normalized shortest path length (λ), and small-worldness (σ); (2) Network efficiency measures of the local efficiency of the whole network (Eloc) and the global efficiency of the network (Eglob); (3) Nodal centrality degree and betweenness degree that reflect functional segregation and integration (Rubinov and Sporns, 2010).
Group comparisons based on topological metrics
To examine group differences of all the network metrics mentioned in the above section, two-sample t-test analyses were used for between-subject comparisons. To correct for multiple comparisons, we used a Bonferroni corrected threshold at the significance level of 0.05. The network results were visualized using BrainNet Viewer (Xia et al., 2013).
Results
Behavioral Results
Independent-samples T-tests were conducted to compare the behavioral performance of good and poor readers in homophone judgment and component judgment tasks, respectively. As shown in Figure 1, poor readers were significantly slower (t30 = −2.08, p = 0.046) and less accurate (t30 = 3.31, p = 0.004) than the normals in the homophone judgment task. However, the two groups had similar performance in the component judgment task (ps > 0.05).

Figure 1. Reaction time (A) and accuracy rates (B) of poor readers and good readers in homophone judgment and component judgment task. ∗p < 0.05; ∗∗p < 0.005.
Whole-Brain Activations
As shown in Figure 2A, during the homophone judgment task, good readers recruited left MFG (BAs 9, 46), left IFG (pars triangularis, BA 45), and bilateral SMA (BAs 6, 8). In contrast, poor readers involved an extensive and symmetrical brain network, including the bilateral prefrontal cortex, insula, cingulate cortex, caudate nuclei, occipital regions, and cerebellum. Group comparisons showed poor readers had significantly more neural responses in the left anterior MFG, right IFG, right superior and middle temporal gyrus (MTG; Figure 2B). The good readers didn’t show more neural responses compared with the poor readers.
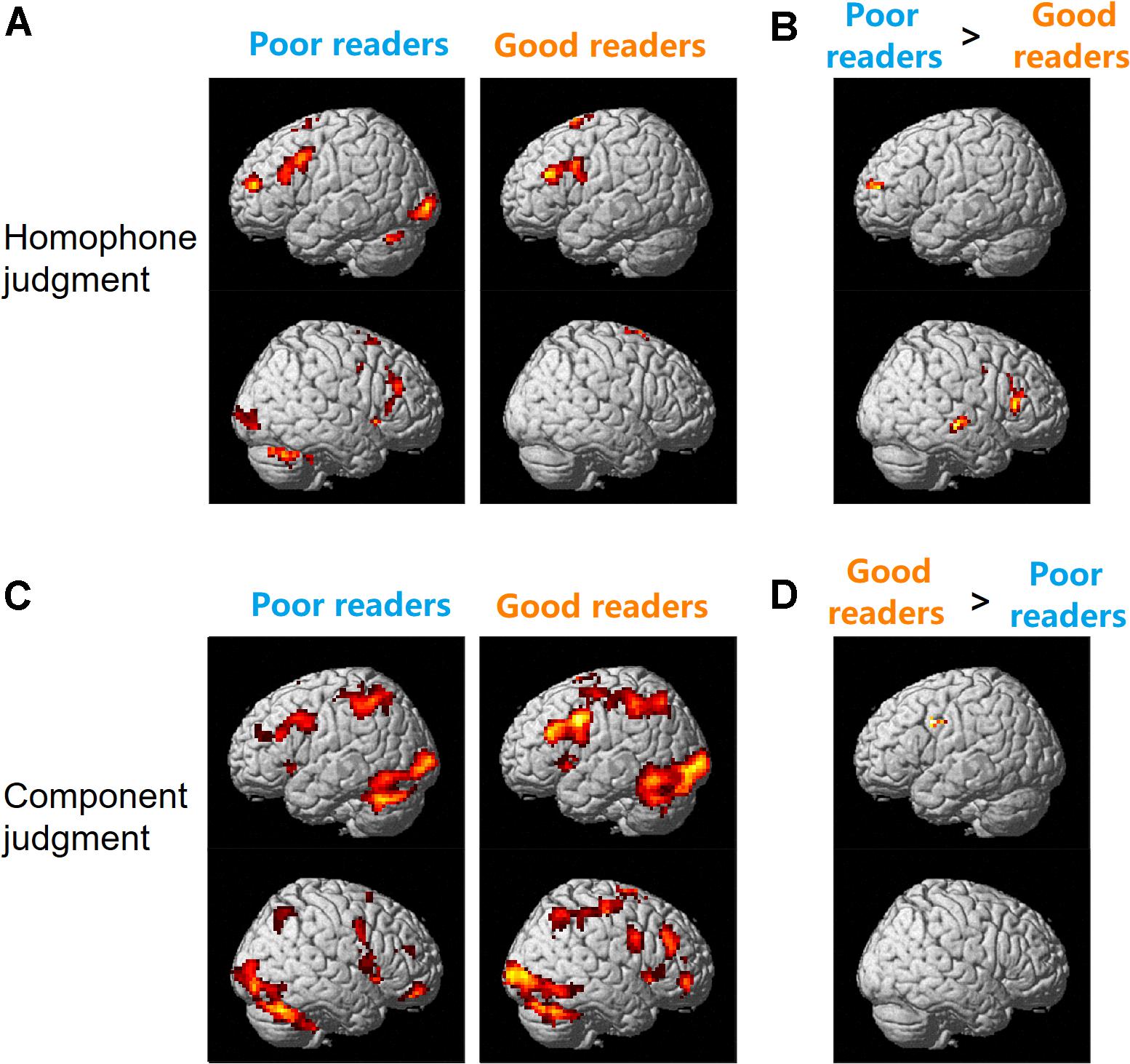
Figure 2. Brain activation for homophone judgment (A,B) and component judgment (C,D) task in Chinese poor readers and good readers. Poor readers showed more neural responses than the good readers in homophone judgment task (B) and the good readers involved more brain activation than the poor readers in component judgment task (D).
During the component judgment task, Chinese good readers showed brain activations in bilateral middle and inferior frontal gyri, precentral gyri, SMA, insula, cingulate cortex, basal ganglia, and thalamus. Bilateral superior and inferior parietal lobules, posterior temporal-occipital cortex, and cerebellum were also involved in this group. The poor readers showed neural responses in those regions similar to that of the good readers (Figure 2C). During the component judgment task (in contrast to the fixation baseline condition), the good readers had significantly more neural activity in the left premotor cortex (BA 6) than the poor readers (Figure 2D). All reported group differences in brain activation were summarized in Table 1.
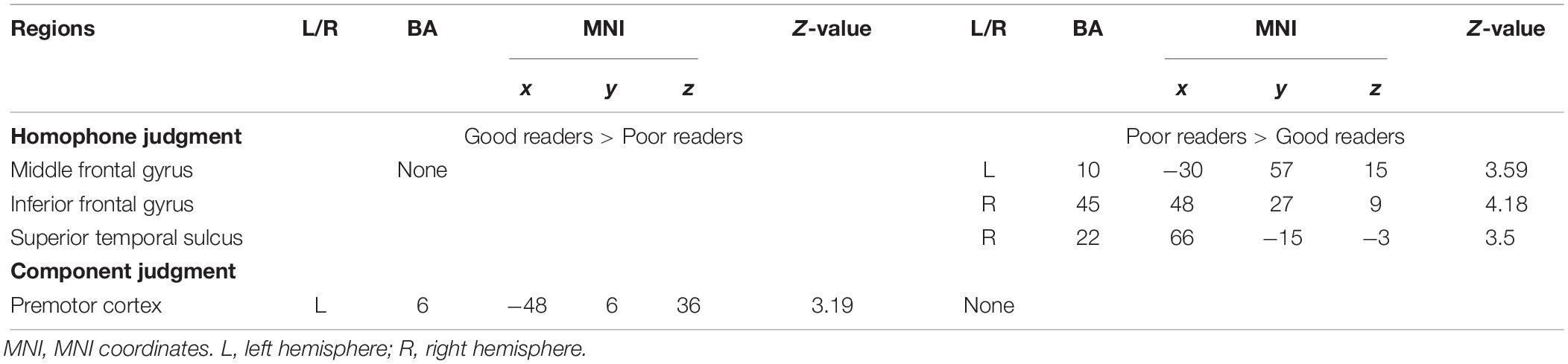
Table 1. Significant differences between Chinese poor readers and good readers in brain activations for homophone judgment and component judgment task.
Network Metrics
As shown in Figures 3A–C, significant group differences were found between their clustering coefficient (Cp), shortest path length (Lp), and normalized shortest path length (λ) of functional networks for visual-orthographic processing (component judgment task), but not for phonological processing (homophone judgment task). To be specific, during visual-orthographic processing, the brain networks of the dyslexic children displayed significantly higher Cp at the sparsity threshold of 45% (dyslexics, 0.76 ± 0.04; normal, 0.73 ± 0.02; t = −2.14, p = 0.04). They also had higher values of Lp than the normals for thresholds between 25 and 45%; the groups were significantly different in their λ at the thresholds of 30, 35, 40, and 45% (ps < 0.05).
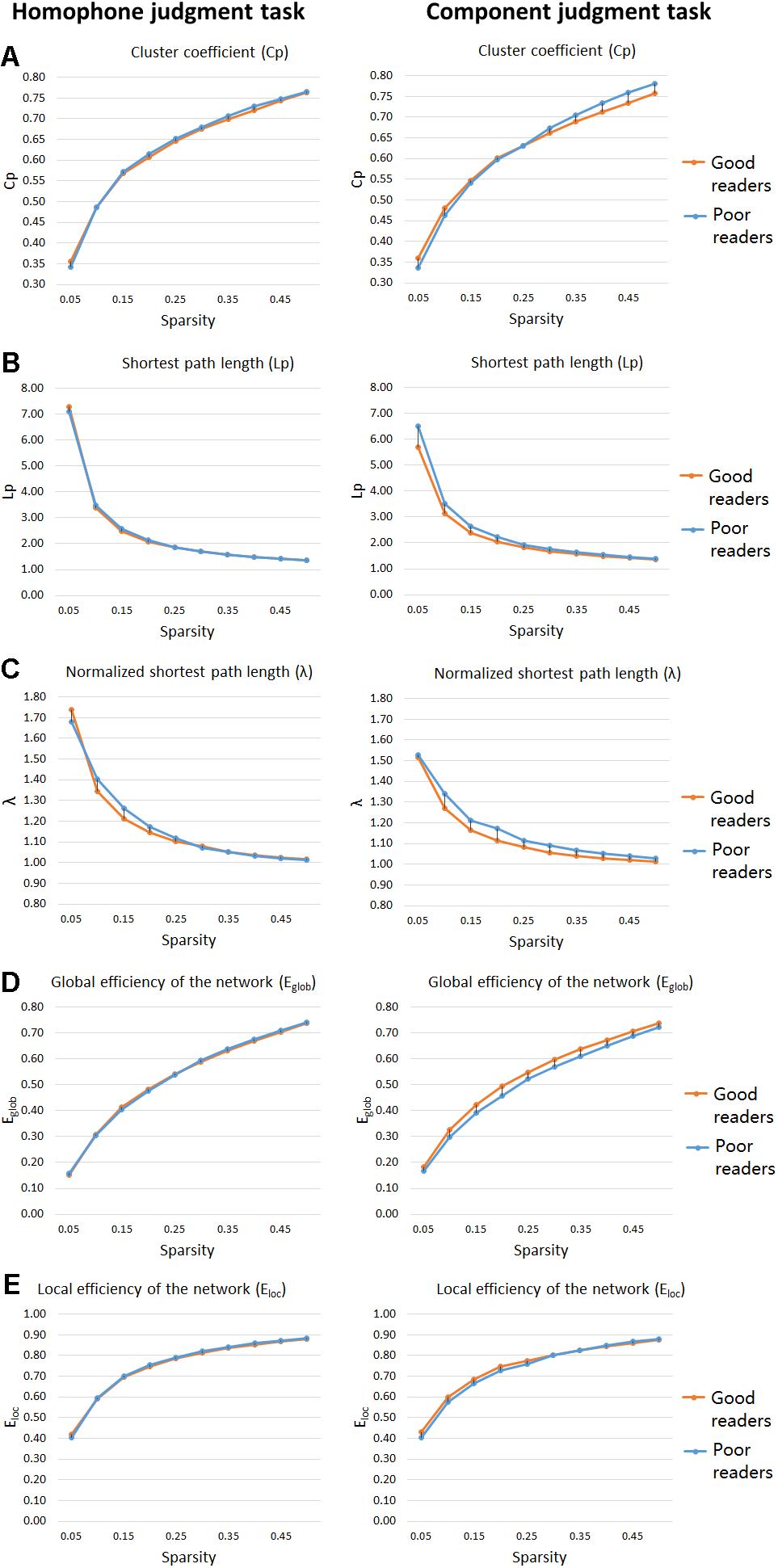
Figure 3. “Small-world” parameters and network proficiency metrics in the defined threshold range (0.05–0.5). Two-sample t-tests show that poor readers are different from the good readers in Cp (A), Lp (B), λ (C), Eglob (D), and Eloc (E) metrics of functional networks for orthographic processing (component judgment task), but not those for phonological processing (homophone judgment task). There are no group differences in Eloc, for both phonological and orthographic processing. Cp, network clustering coefficient; Lp, shortest path length; λ, normalized shortest path length, Eglob, global efficiency of the network; Eloc, local efficiency of the network.
Network Efficiency
For the homophone judgment task, there were no significant group differences in their local efficiency (Eloc) or global efficiency (Eglob). For the component judgment task, the good readers displayed higher global efficiency than the poor readers at the thresholds between 20 and 50% (ps < 0.05). No group difference was found for local efficiency in the component judgment task.
Nodal Centrality Degree
We used two-sample t-tests to examine group differences in nodal centrality measures of degree centrality and betweenness centrality at the strongest threshold (sparsity = 5%) so that all/most of the nodes were connected (Table 2). The poor readers displayed higher degree centrality in left middle temporal gyrus (MTG) during homophone judgment task compared with the good readers, who displayed higher degree centrality than the former in the right temporal pole (TP; superior and middle temporal gyri) during component judgment task (Figure 4A). As shown in Figure 4B, poor readers showed significantly higher betweenness centrality than the good readers in left calcarine fissure and right middle occipital gyrus in component judgment task. There were no significant group differences in betweenness centrality in homophone judgment task.
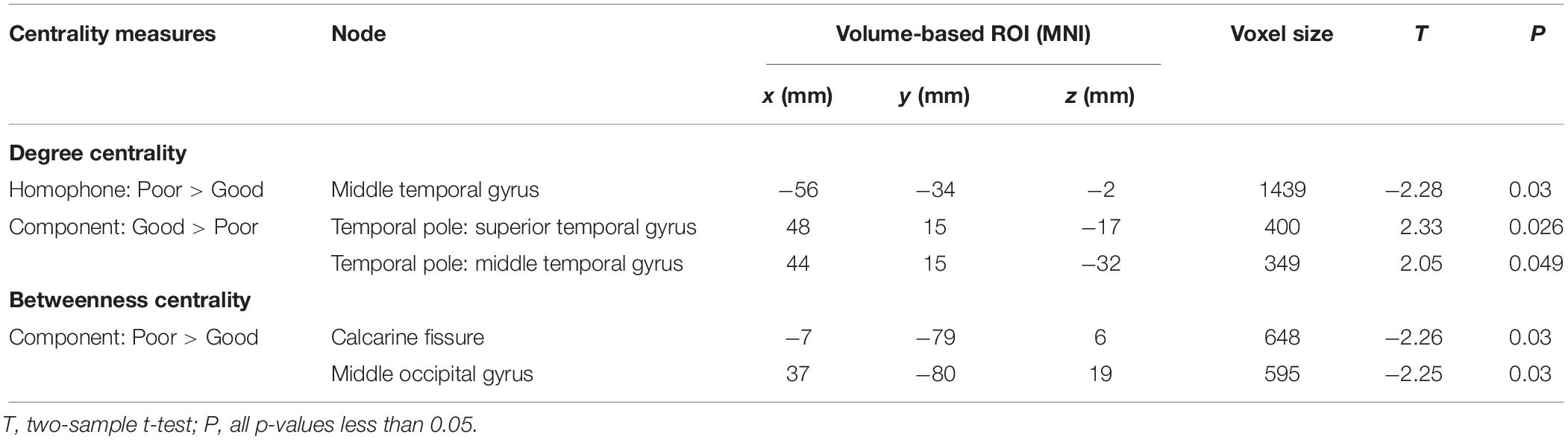
Table 2. Significant differences between Chinese poor readers (Poor) and good readers (Good) in nodal degree centrality and betweenness centrality in homophone judgment task (Homophone) and component judgment task (Component) at the sparsity threshold of 5%. MNI, MNI coordinates.
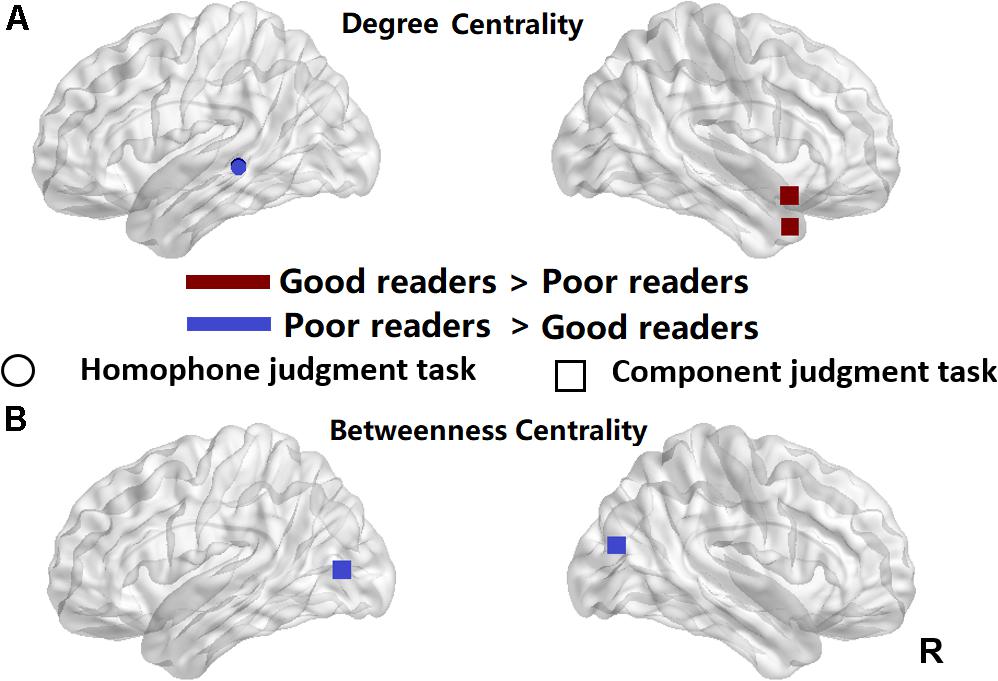
Figure 4. Significant differences between the poor readers and the good readers in their nodal degree centrality (A) and betweenness centrality at the sparsity threshold of 0.05 (B). Red, the good readers children had higher nodal centrality than the poor readers; Blue, the poor readers had higher nodal centrality than the good readers. R, right hemisphere. Circle, homophone judgment task; Square, component judgment task.
Discussion
The present fMRI study using a whole-brain data-driven network approach examined the neural correlates of phonological and visual-orthographic processing in Chinese good readers and poor readers, who were forth or fifth graders matched in age and non-verbal intelligence. Our behavioral data showed that poor readers made more errors and responded more slowly than the good readers in phonological processing (homophone judgment task). There were no group differences in orthographic processing (component judgment task) at the behavioral level. Our behavioral findings are consistent with the phonological deficit hypothesis of dyslexia and suggest no orthographic deficits in Chinese children with reading difficulties (poor readers). Whole-brain activation analyses, however, revealed the poor readers compared with the good readers had hyperactivity in left MFG (BA 10), right IFG (BA 45), and right superior temporal sulcus (STS) (BA 22) during phonological processing, and hypoactivity in the left premotor cortex (BA 6) during visual-orthographic processing. In line with poor readers’ behavioral deficits in phonological processing, the aberrant brain activations for phonological processing in Chinese poor readers suggests neurological disorder underlying the phonological processing of dyslexics. For visual-orthographic processing, the two groups might both function normally with different neural correlates. To provide a complete picture of the brain connectivity profiles of Chinese children with reading difficulties, we examined the topological features of their functional brain networks. During phonological processing, there were no significant group differences in measures of functional segregation (cluster coefficient, Cp) or functional integration (shortest path length, Lp, or the normalized shortest path length, λ). Nor were they different in their values of the global efficiency (Eglob) or local efficiency (Eloc). In visual-orthographic processing, poor readers displayed larger functional segregation (Cp) and less functional integration (Lp, λ) than good readers, who showed higher global efficiency (Eglob).
Further analyses of node centrality showed that during phonological processing, poor readers had a larger value of degree centrality at the left posterior MTG (pMTG) than the good readers, implying its more interactive role as a hub in network of dyslexics. During visual-orthographic processing, the good readers showed more centrality degree in the right TP and less betweenness centrality in the left calcarine fissure and middle occipital gyrus. Based on previous findings and our data reported above, we suggest a phonological core deficit of Chinese dyslexia and different visual-orthographic processing mechanisms in Chinese good and poor readers.
Impaired Phonological Processing, More Efforts on Cognitive Control and Semantic Processing for an Intact Functional Brain Network
Consistent with previous reports on the phonological deficits of Chinese dyslexia, the present study showed that Chinese children with reading difficulties performed worse than the good readers in the homophone judgment task. We didn’t find underactivation in either the left MFG, IFG, temporo-parietal region or fusiform gyrus in the poor readers as reported in previous studies of dyslexia, in particular, Chinese dyslexia (e.g., Shaywitz et al., 1998; Paulesu et al., 2001; Temple et al., 2001; Cao et al., 2008, 2017; Tanaka et al., 2011). Instead, hyperactivation was found in the left anterior prefrontal cortex (aPFC), right IFG, and right posterior STS (pSTS) in poor readers. The aPFC is responsible for integrating outcomes of separate cognitive operations in the pursuit of a higher behavioral goal (for a review, see Ramnani and Owen, 2004) and the right IFG is involved in cognitive control and is recruited when important cues are detected (e.g., Hampshire et al., 2010). Therefore, the larger involvement of left aPFC and right IFG might indicate Chinese poor readers recruited more cognitive control and outcome integration as a compensatory strategy, which is domain-general. Studies on dyslexia have reported reduced gray matter volume in dyslexic readers in the right STG and left STS (e.g., Richlan et al., 2013) and symmetrically distributed gray matter in STS (Dole et al., 2013). The underactivation of left temporo-parietal region is also well-documented in studies of dyslexia, especially in alphabetic languages (Rumsey et al., 1997; Paulesu et al., 2001, 2014; Schulz et al., 2009). We hypothesize that in addition to cognitive control and feedback strategies, our poor readers might have recruited the right homologous site of left pSTS for semantic association and memory, as the left pSTS is a cortical hub for semantic processing and the extraction of meaning from multiple sources of information (Liebenthal et al., 2014).
Although there were no significant group differences in the small-world properties (Cp, Lp, λ, Eglob, and Eloc) of their functional networks for phonological processing, the poor readers had a larger value of degree centrality than the good readers in the left pMTG, which contributes to controlled retrieval of conceptual knowledge (Davey et al., 2016). With this compensatory strategy, poor readers had similar global and local brain network efficiency, despite their poor performance in the phonological task.
Functioning Orthographic Processing, Less Automatic Phonological Retrieval and Multimodal Integration, More Delays in Visual Analysis Hub, and Low Efficient Brain Network
Our studies didn’t find behavioral deficits of Chinese poor readers in visual-orthographic processing. However, they engaged different brain activation and functional network to complete the same task as good readers did. Specifically, when the poor readers were fully occupied by the visual-orthographic processing task, the good readers automatically and efficiently activated the phonology of the presented character stimulus, and displayed more brain activation in the left premotor cortex, which is involved in speech production, especially articulation (e.g., Small et al., 1998; Donnan et al., 1999).
It is possible that Chinese poor readers recruited different neural mechanisms for visual-orthographic processing because they tend to have larger values of cluster coefficient and shortest path length, which also brings them a disadvantage in global efficiency compared with the good readers. The global efficiency of a network is a measure of network integration (Achard and Bullmore, 2007; Rubinov and Sporns, 2010), implying poor readers have a lower integration of functional network for visual-orthographic processing. The degree centrality analysis showed within the functional brain network of poor readers, the centrality of the right TP is less than that of the good readers. As bilateral TP are the core neural substrate for the formation of semantic representation (e.g., Lambon Ralph et al., 2009), our studies seem to suggest that the semantic representation in poor readers are not informative or complete as in the good readers. Meanwhile, betweenness centrality analysis found bilateral posterior visual cortex (calcarine fissure and middle occipital gyrus) play a more active role in information transportation of poor readers than in that of good readers during visual-orthographic processing, which indeed suggests more dependence on the visual neural correlates when poor readers perform the same visual-orthographic task as the good readers.
Our findings of the abnormal functional network for orthographic processing in Chinese children with reading difficulties are consistent with previous findings on the topological organization of brain structural network in Chinese dyslexic children (Liu et al., 2015). The authors using a similar whole-brain network analysis approach examined the structural brain network of Chinese dyslexics and found higher local specialization, a tendency of lower Eglob and prolonged characteristic path length in the dyslexic than the normal, supporting our findings of the functional networks in Chinese children with reading difficulties.
Dynamic Brain Networks in Developmental Dyslexia
Using a whole-brain approach, the current study explored the differences between Chinese good and poor readers. Compared with previous studies in alphabetic languages, this study supports the phonological core deficit hypothesis of dyslexia and pointed out that behaviorally and neurologically dyslexics had manifestations of phonological processing deficits. Meanwhile, our results also imply distinct orthographic processing between Chinese good and poor readers, especially the inefficient functional brain network in poor readers during visual-orthographic processing.
The question remains: why abnormal brain activation and the inefficient brain functional network didn’t cause orthographic processing deficits in Chinese dyslexics, as they do with phonological processing. We hypothesize that the neural mechanism for reading including the functional brain network is dynamic and developing, and behavioral performance of poor readers can be improved.
Training studies on dyslexia have provided numerous evidence on the effects of therapy or remediation on dyslexia. For example, the Tallal–Merzenich team provided intensive auditory training in dyslexic children and showed how the training rewired the children’s brain (Merzenich et al., 1996; Tallal et al., 1996). Shaywitz et al. (2004) recruited second and third graders and administered phonologically mediated reading intervention to those with reading disabilities. Children who received the experimental intervention not only improved their reading performance but also showed increased brain activation in bilateral IFG, left STS, and temporo-occipital regions. Interestingly, Krafnick et al. (2011) reported gray matter volume changes in the left anterior fusiform gyrus/hippocampus, left precuneus, right hippocampus, and right anterior cerebellum during the intervention period. Those areas did not change after the training was stopped.
As we know, learning to read is associated with changes in brain activity. For example, Turkeltaub et al. (2003) in a cross-sectional fMRI study on subjects whose ages ranged from 6 to 22 years found reading acquisition is associated with increased activity in left MTG and IFG and decreased activity in the right inferior temporal regions. Learning to read also changes brain connectivity in dyslexics. Morken et al. (2017) traced reading process of dyslexics during their reading development. In this longitudinal study, participants were scanned through Pre literacy (6 years old), Emergent Literacy (8 years old), and Literacy (12 years old) stages. This study is the first fMRI study tracing the effectivity connectivity in dyslexics. Using Dynamic Causal Modelling (DCM) approach, they found different effectivity patterns in readers with and without dyslexia at age 6 and 8, but 12, implying by age 12, dyslexics reached functional, albeit poor reading skill with normalized effectivity close to the normal.
In the current study, participants were fourth and fifth graders, who had at least 5 years of experience in Chinese character writing and their Chinese literacy is close to the Literacy stage in Morken et al. (2017). It is possible that poor readers have orthographic deficits in their early years of Chinese reading acquisition. After they begin to receive school education, they are asked to do a lot of practice on Chinese writing and spelling to memorize Chinese words by rote in school and after school. Not surprisingly, Chinese writing can predict children’s reading development (e.g., Tan et al., 2005; Cao et al., 2013). With reading development and intensive writing practice, their visual-orthographic processing, which was at a disadvantage in the beginning, might be improved to the extent that the differences between good and poor readers are not significant in terms of their behavioral performance. Only by neuroimaging techniques, we were able to reveal group differences in their neural substrates for visual-orthographic processing.
Meanwhile, the phonological deficit as the core deficit of dyslexia is not alleviated as reading skill approve. Their behavioral performance in phonological manipulation is still significantly different from good readers. Most of the intervention studies on dyslexia adopt the phonological-based training program. If more phonological-based training is used in the classroom setting, phonological deficits might be less in dyslexics as their reading literacy increases.
Conclusion
This study used a whole-brain data-driven network approach to examine the topological features of functional brain networks for phonological and visual-orthographic processing in Chinese good and poor readers. Our results suggest phonological deficits and aberrant neural mechanisms in Chinese poor readers, implying a language-universal phonological deficit in dyslexia. Our findings also indicate good and poor readers rely on different neural mechanisms or strategies in visual-orthographic processing to arrive at similar behavioral performance. To fully understand how phonological processing and visual-orthographic processing progress as reading literacy develops, we will need longitudinal studies tracking the reading development of dyslexics in typical classroom settings using brain imaging techniques.
Data Availability Statement
The datasets generated for this study are available on request to the corresponding author.
Ethics Statement
The studies involving human participants were reviewed and approved by the Key Laboratory of Brain and Cognitive Sciences at The University of Hong Kong. Written informed consent to participate in this study was provided by the participants’ legal guardian/next of kin.
Author Contributions
LT and JY conceived the presented study, collected the data, discussed the results, and contributed to the final manuscript. JY performed the data analyses.
Funding
This research was supported by the Shenzhen Basic Research Scheme (JCYJ20170818110103216 and JCYJ20170412164413575) and Shenzhen Double Chain Grant [2018(256)] awarded to LT, and Innovative School Project in Higher Education of Guangdong, China (GWTP-GC-2017-01) and Social Science Key Research Grant of Universities in Guangdong Province (2018WZDXM005) awarded to JY.
Conflict of Interest
The authors declare that the research was conducted in the absence of any commercial or financial relationships that could be construed as a potential conflict of interest.
Footnotes
- ^ “Pinyin” is an alphabetic phonetic system mainly used in Mainland China to represent pronunciation of Chinese characters in Putonghua, standard spoken language in Mainland China. All children (6–7 years old) enrolled in primary school education at Mainland China are trained in Pinyin for 6–8 weeks before starting to learn Chinese characters.
- ^ http://www.fil.ion.ucl.ac.uk/spm
References
Achard, S., and Bullmore, E. (2007). Efficiency and cost of economical brain functional networks. PLoS Comp. Biol. 3:e17. doi: 10.1371/journal.pcbi.0030017
American Psychiatric Association (2013). Diagnostic and Statistical Manual of Mental Disorders (5th edn). Arlington, VA: American Psychiatric Association.
Bi, Y., Han, Z., and Zhang, Y. (2009). Reading does not depend on writing, even in Chinese. Neuropsychologia 47, 1193–1199. doi: 10.1016/j.neuropsychologia.2008.11.006
Boets, B., de Beeck, H. P. O., Vandermosten, M., Scott, S. K., Gillebert, C. R., Mantini, D., et al. (2013). Intact but less accessible phonetic representations in adults with dyslexia. Science 342, 1251–1254. doi: 10.1126/science.1244333
Booth, J. R., Wood, L., Lu, D., Houk, J. C., and Bitan, T. (2007). The role of the basal ganglia and cerebellum in language processing. Brain Res. 1133, 136–144. doi: 10.1016/j.brainres.2006.11.074
Boros, M., Anton, J.-L., Pech-Georgel, C., Grainger, J., Szwed, M., and Ziegler, J. C. (2016). Orthographic processing deficits in developmental dyslexia: beyond the ventral visual stream. NeuroImage 128, 316–327. doi: 10.1016/j.neuroimage.2016.01.014
Bosse, M.-L., Tainturier, M. J., and Valdois, S. (2007). Developmental dyslexia: the visual attention span deficit hypothesis. Cognition 104, 198–230. doi: 10.1016/j.cognition.2006.05.009
Bowers, P. G., and Wolf, M. (1993). Theoretical links among naming speed, precise timing mechanisms and orthographic skill in dyslexia. Read. Writ. 5, 69–85. doi: 10.1007/bf01026919
Brambati, S. M., Termine, C., Ruffino, M., Danna, M., Lanzi, G., Stella, G., et al. (2006). Neuropsychological deficits and neural dysfunction in familial dyslexia. Brain Res. 1113, 174–185. doi: 10.1016/j.brainres.2006.06.099
Brunswick, N., McCrory, E., Price, C. J., Frith, C. D., and Frith, U. (1999). Explicit and implicit processing of words and pseudowords by adult developmental dyslexics: a search for Wernicke’s Wortschatz? Brain 122, 1901–1917. doi: 10.1093/brain/122.10.1901
Cao, F., Bitan, T., and Booth, J. R. (2008). Effective brain connectivity in children with reading difficulties during phonological processing. Brain Lang. 107, 91–101. doi: 10.1016/j.bandl.2007.12.009
Cao, F., Bitan, T., Chou, T.-L., Burman, D. D., and Booth, J. R. (2006). Deficient orthographic and phonological representations in children with dyslexia revealed by brain activation patterns. J. Child Psychol. Psychiatry 47, 1041–1050. doi: 10.1111/j.1469-7610.2006.01684.x
Cao, F., Vu, M., Lung Chan, D. H., Lawrence, J. M., Harris, L. N., Guan, Q., et al. (2013). Writing affects the brain network of reading in Chinese: a functional magnetic resonance imaging study. Hum. Brain Mapp. 34, 1670–1684. doi: 10.1002/hbm.22017
Cao, F., Yan, X., Wang, Z., Liu, Y., Wang, J., Spray, G. J., et al. (2017). Neural signatures of phonological deficits in Chinese developmental dyslexia. Neuroimage 146, 301–311. doi: 10.1016/j.neuroimage.2016.11.051
Castles, A., and Friedmann, N. (2014). Developmental dyslexia and the phonological deficit hypothesis. Mind Lang. 29, 270–285. doi: 10.1111/mila.12050
Collis, N. L., Kohnen, S., and Kinoshita, S. (2013). The role of visual spatial attention in adult developmental dyslexia. Quar. J. Exp. Psychol. 66, 245–260. doi: 10.1080/17470218.2012.705305
Davey, J., Thompson, H. E., Hallam, G., Karapanagiotidis, T., Murphy, C., De Caso, I., et al. (2016). Exploring the role of the posterior middle temporal gyrus in semantic cognition: integration of anterior temporal lobe with executive processes. Neuroimage 137, 165–177. doi: 10.1016/j.neuroimage.2016.05.051
Demb, J. B., Poldrack, R. A., and Gabrieli, J. D. (1999). “Functional neuroimaging of word processing in normal and dyslexic readers,” in Language, speech, and communication. Converging Methods for Understanding Reading and Dyslexia, eds R. M. Klein, and P. A. McMullen (Cambridge, MA: The MIT Press), 245–304.
Denckla, M. B., and Rudel, R. G. (1976). Naming of object-drawings by dyslexic and other learning disabled children. Brain Lang. 3, 1–15. doi: 10.1016/0093-934X(76)90001-8
Desroches, A. S., Cone, N. E., Bolger, D. J., Bitan, T., Burman, D. D., and Booth, J. R. (2010). Children with reading difficulties show differences in brain regions associated with orthographic processing during spoken language processing. Brain Res. 1356, 73–84. doi: 10.1016/j.brainres.2010.07.097
Ding, G., Perry, C., Peng, D., Ma, L., Li, D., Xu, S., et al. (2003). Neural mechanisms underlying semantic and orthographic processing in Chinese–English bilinguals. NeuroReport 14, 1557–1562. doi: 10.1097/00001756-200308260-00003
Dole, M., Meunier, F., and Hoen, M. (2013). Gray and white matter distribution in dyslexia: a VBM study of superior temporal gyrus asymmetry. PLoS One 8:e76823. doi: 10.1371/journal.pone.0076823
Donnan, G. A., Carey, L. M., and Saling, M. M. (1999). More (or less) on Broca. Lancet 353, 1031–1032. doi: 10.1016/s0140-6736(99)90052-1
Finn, E. S., Shen, X., Holahan, J. M., Scheinost, D., Lacadie, C., Papademetris, X., et al. (2014). Disruption of functional networks in dyslexia: a whole-brain, data-driven analysis of connectivity. Biol. Psychiatry 76, 397–404. doi: 10.1016/j.biopsych.2013.08.031
Gaab, N., Gabrieli, J. D. E., Deutsch, G. K., Tallal, P., and Temple, E. (2007). Neural correlates of rapid auditory processing are disrupted in children with developmental dyslexia and ameliorated with training: an fMRI study. Restorative Neurol. Neurosci. 25, 295–310.
Hampshire, A., Chamberlain, S. R., Monti, M. M., Duncan, J., and Owen, A. M. (2010). The role of the right inferior frontal gyrus: inhibition and attentional control. Neuroimage 50, 1313–1319. doi: 10.1016/j.neuroimage.2009.12.109
Hawelka, S., Huber, C., and Wimmer, H. (2006). Impaired visual processing of letter and digit strings in adult dyslexic readers. Vis. Res. 46, 718–723. doi: 10.1016/j.visres.2005.09.017
Ho, C. S.-H., Chan, D. W.-O., Tsang, S.-M., and Lee, S.-H. (2002). The cognitive profile and multiple-deficit hypothesis in Chinese developmental dyslexia. Dev. Psychol. 38:543. doi: 10.1037//0012-1649.38.4.543
Ho, C. S.-H., and Lai, D. N.-C. (1999). Naming-speed deficits and phonological memory deficits in Chinese developmental dyslexia. Learn. Individ. Differ. 11, 173–186. doi: 10.1016/s1041-6080(00)80004-7
Hoeft, F., Meyler, A., Hernandez, A., Juel, C., Taylor-Hill, H., Martindale, J. L., et al. (2007). Functional and morphometric brain dissociation between dyslexia and reading ability. Proc. Natl. Acad. Sci. U.S.A. 104, 4234–4239. doi: 10.1073/pnas.0609399104
Horwitz, B., Rumsey, J. M., and Donohue, B. C. (1998). Functional connectivity of the angular gyrus in normal reading and dyslexia. Proc. Natl. Acad. Sci. U.S.A. 95, 8939–8944. doi: 10.1073/pnas.95.15.8939
Hu, W., Lee, H. L., Zhang, Q., Liu, T., Geng, L. B., Seghier, M. L., et al. (2010). Developmental dyslexia in Chinese and English populations: dissociating the effect of dyslexia from language differences. Brain 133, 1694–1706. doi: 10.1093/brain/awq106
Huang, H.-S., and Hanley, J. R. (1995). Phonological awareness and visual skills in learning to read Chinese and English. Cognition 54, 73–98. doi: 10.1016/0010-0277(94)00641-w
Hulme, C., Bowyer-Crane, C., Carroll, J. M., Duff, F. J., and Snowling, M. J. (2012). The causal role of phoneme awareness and letter-sound knowledge in learning to read: combining intervention studies with mediation analyses. Psychol. Sci. 23, 572–577. doi: 10.1177/0956797611435921
Krafnick, A. J., Flowers, D. L., Napoliello, E. M., and Eden, G. F. (2011). Gray matter volume changes following reading intervention in dyslexic children. Neuroimage 57, 733–741. doi: 10.1016/j.neuroimage.2010.10.062
Lambon Ralph, M. A., Pobric, G., and Jefferies, E. (2009). Conceptual knowledge is underpinned by the temporal pole bilaterally: convergent evidence from rTMS. Cereb. Cortex 19, 832–838. doi: 10.1093/cercor/bhn131
Liebenthal, E., Desai, R. H., Humphries, C., Sabri, M., and Desai, A. (2014). The functional organization of the left STS: a large scale meta-analysis of PET and fMRI studies of healthy adults. Front. Neurosci. 8:289. doi: 10.3389/fnins.2014.00289
Liu, K., Shi, L., Chen, F., Waye, M. M., Lim, C. K., Cheng, P. W., et al. (2015). Altered topological organization of brain structural network in Chinese children with developmental dyslexia. Neurosci. Lett. 589, 169–175. doi: 10.1016/j.neulet.2015.01.037
Lowe, M. J., Mock, B. J., and Sorenson, J. A. (1998). Functional connectivity in single and multislice echoplanar imaging using resting-state fluctuations. Neuroimage 7, 119–132. doi: 10.1006/nimg.1997.0315
MacSweeney, M., Brammer, M. J., Waters, D., and Goswami, U. (2009). Enhanced activation of the left inferior frontal gyrus in deaf and dyslexic adults during rhyming. Brain 132, 1928–1940. doi: 10.1093/brain/awp129
McBride-Chang, C., Tardif, T., Cho, J.-R., Shu, H. U. A., Fletcher, P., Stokes, S. F., et al. (2008). What’s in a word? morphological awareness and vocabulary knowledge in three languages. Appl. Psycholinguist. 29, 437–462. doi: 10.1017/s014271640808020x
Merzenich, M. M., Jenkins, W. M., Johnston, P., Schreiner, C., Miller, S. L., and Tallal, P. (1996). Temporal processing deficits of language-learning impaired children ameliorated by training. Science 271, 77–81. doi: 10.1126/science.271.5245.77
Morken, F., Helland, T., Hugdahl, K., and Specht, K. (2017). Reading in dyslexia across literacy development: a longitudinal study of effective connectivity. Neuroimage 144, 92–100. doi: 10.1016/j.neuroimage.2016.09.060
Murphy, K., and Fox, M. D. (2017). Towards a consensus regarding global signal regression for resting state functional connectivity MRI. Neuroimage 154, 169–173. doi: 10.1016/j.neuroimage.2016.11.052
Muter, V., Hulme, C., Snowling, M., and Taylor, S. (1998). Segmentation, not rhyming, predicts early progress in learning to read. J. Exp. Child Psychol. 71, 3–27. doi: 10.1006/jecp.1998.2453
Oldfield, R. C. (1971). The assessment and analysis of handedness: the Edinburgh inventory. Neuropsychologia 9, 97–113. doi: 10.1016/0028-3932(71)90067-4
Pan, J., McBride-Chang, C., Shu, H., Liu, H., Zhang, Y., and Li, H. (2011). What is in the naming? a 5-year longitudinal study of early rapid naming and phonological sensitivity in relation to subsequent reading skills in both native Chinese and English as a second language. J. Educ. Psychol. 103:897. doi: 10.1037/a0024344
Pan, J., Shu, H., Wang, Y., and Yan, M. (2015). Parafoveal activation of sign translation previews among deaf readers during the reading of Chinese sentences. Mem. Cogn. 43, 964–972. doi: 10.3758/s13421-015-0511-9
Paulesu, E., Danelli, L., and Berlingeri, M. (2014). Reading the dyslexic brain: multiple dysfunctional routes revealed by a new meta-analysis of PET and fMRI activation studies. Front. Hum. Neurosci. 8:830. doi: 10.3389/fnhum.2014.00830
Paulesu, E., Démonet, J.-F., Fazio, F., McCrory, E., Chanoine, V., Brunswick, N., et al. (2001). Dyslexia: cultural diversity and biological unity. Science 291, 2165–2167. doi: 10.1126/science.1057179
Pecini, C., Biagi, L., Brizzolara, D., Cipriani, P., Di Lieto, M. C., Guzzetta, A., et al. (2011). How many functional brains in developmental dyslexia? When the history of language delay makes the difference. Cogn. Behav. Neurol. 24, 85–92. doi: 10.1097/WNN.0b013e318222a4c2
Pennington, B. F., and Lefly, D. L. (2001). Early reading development in children at family risk for dyslexia. Child Dev. 72, 816–833. doi: 10.1111/1467-8624.00317
Rack, J. P., Snowling, M. J., and Olson, R. K. (1992). developmental dyslexia: a review. Read. Res. Quar. 27, 29–53.
Ramnani, N., and Owen, A. M. (2004). Anterior prefrontal cortex: insights into function from anatomy and neuroimaging. Nat. Rev. Neurosci. 5:184. doi: 10.1038/nrn1343
Ramus, F., and Szenkovits, G. (2008). What phonological deficit? Quar. J. Exp. Psychol. 61, 129–141. doi: 10.1080/17470210701508822
Raven, J., Raven, J. C., and Court, J. H. (1998). Manual for Raven’sProgressive Matrices and Vocabulary Scales. San Antonio, TX: Pearson, Inc.
Richlan, F., Kronbichler, M., and Wimmer, H. (2011). Meta-analyzing brain dysfunctions in dyslexic children and adults. Neuroimage 56, 1735–1742. doi: 10.1016/j.neuroimage.2011.02.040
Richlan, F., Kronbichler, M., and Wimmer, H. (2013). Structural abnormalities in the dyslexic brain: a meta-analysis of voxel-based morphometry studies. Hum. Brain Mapp. 34, 3055–3065. doi: 10.1002/hbm.22127
Rubinov, M., and Sporns, O. (2010). Complex network measures of brain connectivity: uses and interpretations. Neuroimage 52, 1059–1069. doi: 10.1016/j.neuroimage.2009.10.003
Rumsey, J. M., Nace, K., Donohue, B., Wise, D., Maisog, J. M., and Andreason, P. (1997). A positron emission tomographic study of impaired word recognition and phonological processing in dyslexic men. Arch. Neurol. 54, 562–573. doi: 10.1001/archneur.1997.00550170042013
Schulz, E., Maurer, U., van der Mark, S., Bucher, K., Brem, S., Martin, E., et al. (2009). Reading for meaning in dyslexic and young children: distinct neural pathways but common endpoints. Neuropsychologia 47, 2544–2557. doi: 10.1016/j.neuropsychologia.2009.04.028
Shankweiler, D., and Lundquist, E. (1992). On the relations between learning to spell and learning to read. Adv. Psychol. 94, 179–192. doi: 10.1016/s0166-4115(08)62795-8
Shaywitz, B. A., Shaywitz, S. E., Blachman, B. A., Pugh, K. R., Fulbright, R. K., Skudlarski, P., et al. (2004). Development of left occipitotemporal systems for skilled reading in children after a phonologically-based intervention. Biol. Psychiatry 55, 926–933. doi: 10.1016/j.biopsych.2003.12.019
Shaywitz, S. E., and Shaywitz, B. A. (2003). Dyslexia (specific reading disability). Pediatr. Rev. 24, 147–153. doi: 10.1542/pir.24-5-147
Shaywitz, S. E., Shaywitz, B. A., Pugh, K. R., Fulbright, R. K., Constable, R. T., Mencl, W. E., et al. (1998). Functional disruption in the organization of the brain for reading in dyslexia. Proc. Natl. Acad. Sci. U.S.A. 95, 2636–2641.
Siok, W. T., and Fletcher, P. (2001). The role of phonological awareness and visual-orthographic skills in Chinese reading acquisition. Dev. Psychol. 37:886. doi: 10.1037/0012-1649.37.6.886
Siok, W. T., Jin, Z., Fletcher, P., and Tan, L. H. (2003). Distinct brain regions associated with syllable and phoneme. Hum. Brain Mapp. 18, 201–207. doi: 10.1002/hbm.10094
Siok, W. T., Niu, Z., Jin, Z., Perfetti, C. A., and Tan, L. H. (2008). A structural–functional basis for dyslexia in the cortex of Chinese readers. Proc. Natl. Acad. Sci. U.S.A. 105, 5561–5566. doi: 10.1073/pnas.0801750105
Siok, W. T., Perfetti, C. A., Jin, Z., and Tan, L. H. (2004). Biological abnormality of impaired reading is constrained by culture. Nature 431:71. doi: 10.1038/nature02865
Siok, W. T., Spinks, J. A., Jin, Z., and Tan, L. H. (2009). Developmental dyslexia is characterized by the co-existence of visuospatial and phonological disorders in Chinese children. Curr. Biol. 19, R890–R892. doi: 10.1016/j.cub.2009.08.014
Small, S. L., Flores, D. K., and Noll, D. C. (1998). Different neural circuits subserve reading before and after therapy for acquired dyslexia. Brain Lang. 62, 298–308. doi: 10.1006/brln.1998.1951
Snowling, M. (1998). Dyslexia as a phonological deficit: evidence and implications. Child Psychol. Psychiatr. Rev. 3, 4–11. doi: 10.1111/1475-3588.00201
Snowling, M. J. (1981). Phonemic deficits in developmental dyslexia. Psychol. Res. 43, 219–234. doi: 10.1007/bf00309831
Song, X.-W., Dong, Z.-Y., Long, X.-Y., Li, S.-F., Zuo, X.-N., Zhu, C.-Z., et al. (2011). REST: a toolkit for resting-state functional magnetic resonance imaging data processing. PLoS One 6:e25031. doi: 10.1371/journal.pone.0025031
Steinbrink, C., Groth, K., Lachmann, T., and Riecker, A. (2012). Neural correlates of temporal auditory processing in developmental dyslexia during German vowel length discrimination: an fMRI study. Brain Lang. 121, 1–11. doi: 10.1016/j.bandl.2011.12.003
Talairach, J., and Tournoux, P. (1988). Co-Planar Stereotaxic Atlas of the Human Brain. New York, NY: Theime.
Tallal, P., Miller, S. L., Bedi, G., Byma, G., Wang, X., Nagarajan, S. S., et al. (1996). Language comprehension in language-learning impaired children improved with acoustically modified speech. Science 271, 81–84. doi: 10.1126/science.271.5245.81
Tan, L. H., Spinks, J. A., Eden, G. F., Perfetti, C. A., and Siok, W. T. (2005). Reading depends on writing, in Chinese. Proc. Natl. Acad. Sci. U.S.A. 102, 8781–8785.
Tanaka, H., Black, J. M., Hulme, C., Stanley, L. M., Kesler, S. R., Whitfield-Gabrieli, S., et al. (2011). The brain basis of the phonological deficit in dyslexia is independent of IQ. Psychol. Sci. 22, 1442–1451. doi: 10.1177/0956797611419521
Temple, E., Poldrack, R. A., Salidis, J., Deutsch, G. K., Tallal, P., Merzenich, M. M., et al. (2001). Disrupted neural responses to phonological and orthographic processing in dyslexic children: an fMRI study. Neuroreport 12, 299–307. doi: 10.1097/00001756-200102120-00024
Turkeltaub, P. E., Gareau, L., Flowers, D. L., Zeffiro, T. A., and Eden, G. F. (2003). Development of neural mechanisms for reading. Nat. Neurosci. 6:767.
Tzourio-Mazoyer, N., Landeau, B., Papathanassiou, D., Crivello, F., Etard, O., Delcroix, N., et al. (2002). Automated anatomical labeling of activations in SPM using a macroscopic anatomical parcellation of the MNI MRI single-subject brain. Neuroimage 15, 273–289. doi: 10.1006/nimg.2001.0978
Vaessen, A., Gerretsen, P., and Blomert, L. (2009). Naming problems do not reflect a second independent core deficit in dyslexia: double deficits explored. J. Exp. Child Psychol. 103, 202–221. doi: 10.1016/j.jecp.2008.12.004
van der Mark, S., Bucher, K., Maurer, U., Schulz, E., Brem, S., Buckelmüller, J., et al. (2009). Children with dyslexia lack multiple specializations along the visual word-form (VWF) system. Neuroimage 47, 1940–1949. doi: 10.1016/j.neuroimage.2009.05.021
Vidyasagar, T. R., and Pammer, K. (2010). Dyslexia: a deficit in visuo-spatial attention, not in phonological processing. Trends Cogn. Sci. 14, 57–63. doi: 10.1016/j.tics.2009.12.003
Wang, J., Wang, X., Xia, M., Liao, X., Evans, A., and He, Y. (2015). GRETNA: a graph theoretical network analysis toolbox for imaging connectomics. Front. Hum. Neurosci. 9:386. doi: 10.3389/fnhum.2015.00386
Wimmer, H., and Schurz, M. (2010). Dyslexia in regular orthographies: manifestation and causation. Dyslexia 16, 283–299. doi: 10.1002/dys.411
Wolf, M., and Bowers, P. G. (1999). The double-deficit hypothesis for the developmental dyslexias. J. Educ. Psychol. 91:415. doi: 10.1037//0022-0663.91.3.415
Wolf, M., Bowers, P. G., and Biddle, K. (2000). Naming-speed processes, timing, and reading: a conceptual review. J. Learn. Disabil. 33, 387–407. doi: 10.1177/002221940003300409
Xia, M., Wang, J., and He, Y. (2013). BrainNet viewer: a network visualization tool for human brain connectomics. PLoS One 8:e68910. doi: 10.1371/journal.pone.0068910
Yang, X., Liu, J., Meng, Y., Xia, M., Cui, Z., Wu, X., et al. (2017). Network analysis reveals disrupted functional brain circuitry in drug-naive social anxiety disorder. Neuroimage 190, 213–223. doi: 10.1016/j.neuroimage.2017.12.011
Zhang, M., Xie, W., Xu, Y., and Meng, X. (2018). Auditory temporal perceptual learning and transfer in Chinese-speaking children with developmental dyslexia. Res. Dev. Disabil. 74, 146–159. doi: 10.1016/j.ridd.2018.01.005
Zhu, H., Qiu, C., Meng, Y., Yuan, M., Zhang, Y., Ren, Z., et al. (2017). Altered topological properties of brain networks in social anxiety disorder: a resting-state functional MRI study. Sci. Rep. 7:43089. doi: 10.1038/srep43089
Ziegler, J. C. (2006). Do differences in brain activation challenge universal theories of dyslexia? Brain Lang. 98, 341–343. doi: 10.1016/j.bandl.2005.05.002
Ziegler, J. C., and Goswami, U. (2005). Reading acquisition, developmental dyslexia, and skilled reading across languages: a psycholinguistic grain size theory. Psychol. Bull. 131:3. doi: 10.1037/0033-2909.131.1.3
Ziegler, J. C., Pech-Georgel, C., Dufau, S., and Grainger, J. (2010). Rapid processing of letters, digits and symbols: what purely visual-attentional deficit in developmental dyslexia? Dev. Sci. 13, F8–F14. doi: 10.1111/j.1467-7687.2010.00983.x
Keywords: dyslexia, phonological deficit, orthographic deficit, Chinese, functional brain network
Citation: Yang J and Tan LH (2020) Whole-Brain Functional Networks for Phonological and Orthographic Processing in Chinese Good and Poor Readers. Front. Psychol. 10:2945. doi: 10.3389/fpsyg.2019.02945
Received: 11 September 2019; Accepted: 12 December 2019;
Published: 14 January 2020.
Edited by:
Aaron J. Newman, Dalhousie University, CanadaReviewed by:
Maria Fernanda Lara-Diaz, Universidad Nacional de Colombia, ColombiaJuan C. Melendez, University of Valencia, Spain
Copyright © 2020 Yang and Tan. This is an open-access article distributed under the terms of the Creative Commons Attribution License (CC BY). The use, distribution or reproduction in other forums is permitted, provided the original author(s) and the copyright owner(s) are credited and that the original publication in this journal is cited, in accordance with accepted academic practice. No use, distribution or reproduction is permitted which does not comply with these terms.
*Correspondence: Li Hai Tan, dGFubGhAc3p1LmVkdS5jbg==