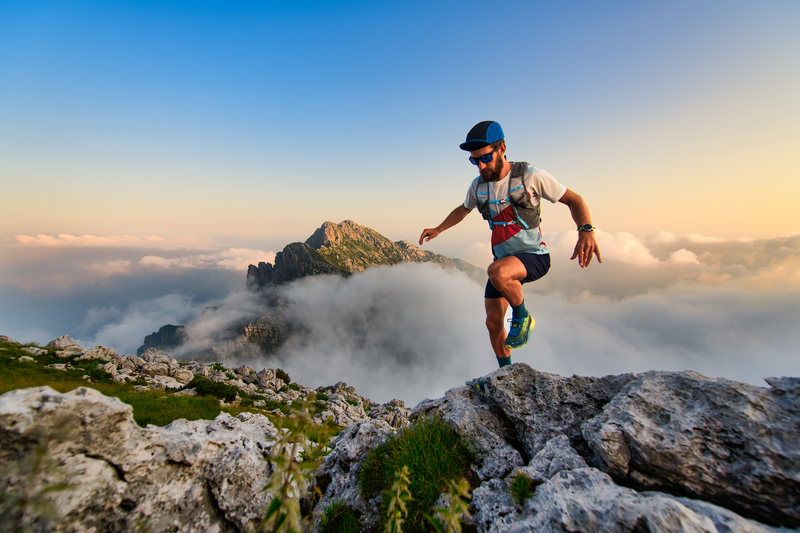
95% of researchers rate our articles as excellent or good
Learn more about the work of our research integrity team to safeguard the quality of each article we publish.
Find out more
HYPOTHESIS AND THEORY article
Front. Psychol. , 09 July 2019
Sec. Organizational Psychology
Volume 10 - 2019 | https://doi.org/10.3389/fpsyg.2019.01596
A commentary has been posted on this article:
Commentary: Principles, Approaches and Challenges of Applying Big Data in Safety Psychology Research
Big data is now widely used in many fields and is also widely applied to the integration of disciplines. Traditional methods of safety psychology are not well suited for analyzing psychological states, especially in the management of human factors in industrial production. Also, big data now becomes a new way to excavate related insight by analyzing a large amount of psychological data. So, this paper is to propose the concept of big data of safety psychology (BDSP) and to illustrate the challenges of applying big data in safety psychology. First, this paper puts forward the concept of BDSP and analyzes the difference between BDSP and traditional sample data. Subsequently, this paper summarizes the classification standard and basic characteristic of BDSP, explores the framework of BDSP and then constructs a three-dimensional structure of BDSP. Lastly, this paper discusses the challenges of using BDSP. This study is of great help to safety practitioners to solve psychological issues in the safety domain, and points out one of the research trends of human factor in industrial safety.
Psychology plays an important role in work safety (Cree and Kelloway, 1997). In the long period of productive practice, safety psychology became a subject of improving safety consciousness of workers in China. With the emergence of big data, disruptive technologies have transformed commerce, science and many aspects of society (Lin, 2015), evidently when Nature and Science published special issues dedicating to discuss the opportunities and challenges brought by big data. It marks the possibility that we can apply big data in various fields and reveals that thinking of data processing is correlation rather than causation. Meanwhile, there was a link between the psychological state of people and the tendency of behavior. Once the patterns of human acts (e.g., data record of text, images, and video) are analyzed by big data technology, personal psychological state can be inferred (Huyghebaert et al., 2017). Accordingly, we can apply big data to solve psychological issues related to safety. The aim is to analyze the correlation between psychological data and workplace risk, which provides valuable information of psychology for users (e.g., government, enterprises, and employees) to reduce the risk of the working environment.
Big data practitioners in academia, industry and community have built a comprehensive base of tools and knowledge that makes big data accessible to researchers in a broad range of fields (Chen and Wojcik, 2016). Thus, big data offer fresh ways to perfect and extend theoriesof safety psychology. Many studies analyze safety big data from perspectives of concepts, models and empirical analysis. For example, Ouyang et al. (2018) put forward the methodologies, principles and prospects of applying big data in safety science research, Huang et al. (2018b) constructed a conceptual framework of big-data-driven safety decision-making, and Marvin et al. (2017) explained a few examples of the new developments that big data was contributing to in early warning systems in food safety problems. In addition, crisis-related incidents generate data in the course of practice, especially when joined with data originating from other sources, create giant psychological related datasets (Guzzo et al., 2015). For one thing, job insecurity leads to stressful workplace conditions, which decrease people’s psychological well-being and mental health, and are even linked to the development of mental illness (Giorgi et al., 2016; Mucci et al., 2016). For another, psychosocial risk management during times of crisis is a strategic topic because crisis may be accompanied by an increase in mortality (Giorgi et al., 2015). As psychological data sets grow, they become increasingly valuable for safety policymakers to interfere with collective climate of fear and develop optimism in the workplace.
It is important to make an effective safety decision based on reliable and enough psychological related information in safety management (Wang et al., 2017). Various scholars have access to the massive quantities of information produced by people, things and their interactions in the era of big data (Boyd and Crawford, 2012). Thus, big data provides credible evidence based on psychological information analyzing, forecasting and managing people’s behavior. However, big data technology is not mature enough and thus leads to some data dilemmas, such as noise accumulation, spurious correlation, and incidental homogeneity (Fan et al., 2014). Also, another difficulty is how to manage the large quantity of psychological data efficiently and address the shortcomings of data in the current technological environment, such as semantic analysis, multimode data reduction, data plugging, data separation, slow data, and data missing. Despite the fact that there are still some problems, it is a fascinating outlook that unsafe psychology of human was intervened by evidence of safety information based on psychological knowledge and rules.
In China, safety psychology is almost a compulsory course for safety engineering students. The aim of safety psychology is that it can infer the psychological state by analyzing behavior, then explain, predict and intervene the human’s act, thus improve the human’s ability to handle risk factors in enterprises. Sample surveys of traditional safety psychology are a main way to discover the psychological knowledge and rule in the safety domain, including in the research methods of observation, survey, test, experimental and case analysis, as well as the research tool of psychological questionnaires and psychological scales (Zhu et al., 2015). However, the volume of study objects is limited, with it difficult to obtain thousands or more. The selection of study objects should meet the essential qualification of statistics, namely that samples selected are random with equal chances. As such, sample volume can reflect the situation of the entire study object.
Some psychological factors are often ignored in the entire research due to the difference in education, culture, environment, cognition, and work experience of study object (Fisher et al., 2017), as well as the limitations of sample volume and questionnaire design. The repeatability and reliability of research results are relatively low, which presents difficulties to large-scale promotion and application in the field of industrial safety. Since traditional methods of safety psychology can’t meet the complex requirement of safety activities, big data applied to safety psychology research in such a context is nurtured and hygienic, providing safety managers with evidence-based services of behavior management and task disassembling.
With the era of big data coming, especially when Internet companies have accumulated the application and optimization experience of data mining in the long run, it has achieved the objectives in term of automatic and real-time storage, data processing and analyzing, and personalized service provided according to user’s psychological characteristics and behavioral habits. For example, e-commerce companies send messages to his customers about various products likely to interest him to the app homepage according to the user’s psychological characteristics indicated by online shopping history.
Big data is a well-known data processing technology in use today which has been employed in various fields. With the view that the background for the current study circumstances is the cross-integration trend of the subject area, many researchers and practitioners use big data technology to explore and verify psychological issues in recent years as shown in Table 1. Accordingly, new psychological knowledge and rules were excavated by big data, are also applied to address the challenge faced by traditional safety psychology.
Interdisciplinary research is one of the popular research fields in organizational psychology, as is also determined by the characteristic of psychological issues in industrial safety. It is necessary to the disciplinary intercrossing and fusion of safety science, data science and psychology, which solve the psychological issue in the safety domain from theoretical innovation and practical application.
Data science is a discipline using data to learn knowledge, including applied mathematics, pattern recognition, machine learning, data warehouse and high-performance computing. One of its aims is to reveal the phenomena and laws of human behaviors. The emergence of big data has spawned a new research paradigm and provided a bright prospect to numerous disciplines. Hence, we can use big data to address safety issues.
Safety psychology is the intersection of the disciplines of safety science and psychology. Basically, the knowledge and skills from safety psychology need to be mastered by safety practitioners, which is the result of long-term application and practice in the enterprise. Also, safety psychology is a mature discipline in China. With the rapid development of information technologies, a large amount of data have been produced for almost every aspect of people’s lives and work, and these data can be used to solve safety problems. Big data applies in the safety domain to make a large amount of theoretical innovation and expansion, including safety big data proposal (Ouyang et al., 2018), accidents analysis paradigm (Huang et al., 2018a), and big-data-driven safety decision-making (Huang et al., 2018b). Big data can also be used in coal mine safety (Abou El-Nasr and Shaban, 2015), construction safety (Guo et al., 2016), traffic safety (Shi and Abdel-Aty, 2015), and food safety (Wang et al., 2015). Furthermore, the massive quantities of data covering human behaviors and moods offer psychology an unprecedented opportunity to conduct innovative theory field study (Chen and Wojcik, 2016). Applying big data in psychology will also gradually mature, and many scholars have carried out lots of theoretical studies (Cheung and Jak, 2016; Harlow and Oswald, 2016; Adjerid and Kelley, 2018) and practice studies (Dhar, 2013; Vie et al., 2013; Paxton and Griffiths, 2017; Leivada et al., 2019).
According to what has been discussed above, safety science, data science and psychology are no longer independent but linked to each other (e.g., safety psychology, safety big data, big data in psychology), which has developed into a new disciplinary field, namely big data of safety psychology (BDSP), as shown in Figure 1. Also, a basic definition of BDSP is described according to the content of safety science, psychology, and big data.
Big data of safety psychology refers to structured, semi-structured and unstructured data set formed by psychological index parameters and behavior, which provide potential and valuable psychological knowledge and rules to solve the psychological issue related to safety with the help of big data technology.
The concepts of BDSP are further analyzed as follows:
(1) Data volume is beyond the maximum handling capability of the traditional computational technology within a given time period (Manyika et al., 2011), and the computing model and storage pattern of current computers levels can’t meet data analyzing requirements, thus it shall depend on distributed processing, distributed database, cloud storage, and virtualization technology based on cloud computing.
(2) BDSP is a set of various psychological data, including structured data (e.g., number, symbol), semi-structured data (e.g., XML document, HTML document) and unstructured data (e.g., text, image, video, and sound). The data types are complex, and the value density of information is quite low.
(3) BDSP does not mean a large amount of psychological related data in the safety domain, which can provide valuable psychological information to solve the psychological issue related to safety. In addition, the mining results need to satisfy the actual application requirement of the current permit condition of big data mining cost and information value density.
The aims of BDSP are as follows:
(1) The relationship between psychological factors (e.g., mood, ability, personality, gender, age, experience, and environment) and workplace risk were analyzed by a large amount of multi-modal data and collected accident cases.
(2) Human’s behavior simulations in the early, middle and late stages of the accident were performed by big data technology, which constantly optimized personal mode of psychological cognition and reactions, and then provided evidence-based information for the improvement and development of 3E countermeasures (e.g., engineering, education, and enforcement).
(3) It can contribute to reducing safety psychological information asymmetry among community, industry, enterprise and individual level, sequentially exerts an imperceptible influence on people’s safety consciousness.
Through literature analysis, it is not hard to find out that big data has plenty of differences from sample data (Ouyang et al., 2018). Differences from 12 aspects between BDSP and traditional sample data were analyzed briefly (Kang et al., 2017a), as shown in Table 2. Both BDSP and traditional sample data have advantages and disadvantages in different situations. Rationally choosing corresponding research methods is a scientific approach to solve psychological issues in the safety domain. Also, the purposes of both are to find knowledge and rules and further to reduce accidents and to create a better working environment.
Managing a large amount of psychological data is complex system engineering. Besides, BDSP are studied from different perspectives, so that twice as much can be accomplished with half the effort. The classification of BDSP help better understand its study content and development trend, which could be targeted to solve psychological problems and avoid detours (Ouyang et al., 2018). The types of BDSP are divided into seven categories, as shown in Table 3. It is noteworthy that the classification is not immutable but may change accordingly with the development of safety science, psychology, and big data.
The goal of characteristics can be viewed as a deeper understanding of BDSP application and a guidance of how to apply BDSP appropriately. Data collected has hidden information of psychological states of people, which may be of little value to safety practitioners without big data mining technology. Thus, an interdisciplinary approach is needed to deal with psychological problems in industrial safety (Huang et al., 2018b). BDSP is the development trend for information technology applied to psychology. The application value of big data comes from three sources, according to three elements of big data (Mayer-Schönberger and Cukier, 2013). On this basis, the dataset serves as a basis, technology as a support, and application as a guide. Therefore, seven basic characteristics can be concluded, as Figure 2 shows. To further clarify the connotations of each characteristic, more detailed explanations can be seen as follows.
(1) Safety psychological data overall advantages. In the area of big data, psychological data are not obtained from samples selected randomly, but covers the whole study object, excavated from the underlying psychological knowledge and rules to improve the safety climate of organizations well. In addition, the traditional psychology study has been simplified as several important factors to analyze the relationship between behavior and psychological states. It is sometimes difficult to find the latent psychological rules from the heavy industry, light industry, and agriculture industry due to the limited study object volume. By contrast, related insight can be excavated from a large amount of psychological data since BDSP cover the whole study object. Meanwhile, the whole data produced by simple algorithms are more accurate than the sample data produced by complex algorithms (Halevy et al., 2009; Sacristán and Dilla, 2015). In practice, we should set a fault-tolerant standard of psychological data and quickly gain general outlines of psychological knowledge related to safety. For example, in 2015, occupations in China were divided into 1481 types, leading to the difficulty of recognizing the characteristics of each occupation by using traditional methods. But, it is quick to excavate the general rules of those occupations according to a large amount of psychological data in safety activities.
(2) Safety psychological data correlation. The correlation of psychological data is the core of BDSP (Ouyang et al., 2018), therefore many data mining methods have been emerging. Correlation thinking is a prior logic way in which the relevant insight is excavated from the stream of big data. Also, the major advantages of correlation study are to explore the unknown knowledge and rules related to safety psychology, to broaden the study areas of safety psychology, then to check the reliability of traditional sample results. For example, Golder and Macy (2011) applied big data to psychology to excavate the correlation of data acquisition where emotional information more than 500 million Twitter data from approximately 2.4 million users in 84 countries from February 2008 to January 2010. The results showed a volatility pattern of positive emotions and negative emotions.
(3) Safety psychological technology integration. The formation of BDSP benefits from the integration of cutting-edge information technology, and its application relies on the information technology level currently. It is a critical component of how to solve the technological problem of massive scale auto-implement of data acquisition, storage, processing, and visualization. So far, capturing the streams of big data in psychology mainly concentrates on Internet behavior records (e.g., Twitter, Weibo), which has achieved some success in psychological knowledge and rules. Real-time record and trace psychological data can be applied in a large scale in industrial production with the evolution of information technology.
(4) Safety psychological data-driven. Data-driven has become a popular and promising study method, incorporating almost all disciplines (Huang et al., 2018b). It excavates information value from a large amount of data, and integrates and refines the rules of information, thus forming an automated decision-making model. The system automatically makes decisions according to the previously established model without manual assistance, when raw psychological data is input. The bias information from the model analysis between the data-driven results and the actual results will give feedback to machine learning, and the model will self-improve in the subsequent machine learning iterative process. The complete data-driven value system is constructed from the data acquisition, management and mining, which play an important role in BDSP.
(5) Safety psychological information need. Due to the sectors and scales of enterprises differences, they have a practical need in the type and direction of psychological information, especially in high-frequency accidents industry and near-zero accidents service industry. Enterprises choose the level of big data mining technology according to its own safety condition, which intervenes the unsafe climate of formal and informal group. The real-time processing and analyzing of psychological data should satisfy the need of psychological information critical in safety evidence-based decisions. Therefore, the need for psychological information in the safety domain is the driving force for the technological innovation and development of BDSP.
(6) Safety psychological state prediction. With the development and popularity of wearables, psychological data be tracked and recorded without interfering with the activity of the worker, with electronic data automatically acquired, storage, and updated by smart devices, memory technology, and communication technology. According to the models (e.g., active learning, semi-supervised learning, transfer learning, and multitask learning) and algorithms (e.g., Apriori, C4.5, and AdaBoost) of big data, users instantly predict the psychological condition of individual, group and even the industry when combining data with a visual information system, providing practical evidence for safety decision-making and management. For example, Candás et al. (2014) used the data mining technology to predict people with mental disorders according to personal data gathered from wearables.
(7) Safety psychological information value. BDSP does not mean that a large amount of psychological data is related to safety. Its core is that psychological data can be transformed into valuable information for safety practitioners. Because the psychological state of workers is difficult to quantify, it requires managers to have relatively reliable psychological information to prevent workplace risk. Enterprises selects proper methods and tools to excavate the psychological rule to meet information value requirements in practical product. For example, the system real-time monitoring and recording information of miners’ psychological states by wearables, and they further correlate with storage data, which automatically alert miners of the attention against danger.
A reasonable framework of research will be critical to master BDSP. The framework of BDSP will standardize and guide safety psychological application in the big data age. From the perspective of data requirement, study method, study tool and processing step, they have formed the framework of BDSP, as shown in Figure 3. To further clarify the framework of BDSP, more detailed explanations can be seen as follows.
Data acquisition is the basic condition for big data technology to study the psychological issues in the safety domain. Data acquisition methods are diverse and data quality is closely related to data sources, and on this basis, data acquisition tools are constantly emerging accordingly. Web crawling is one of the widely used ways for data acquisition automatically in recent years. The user sets the crawler software parameter to improve the data quality, ensuring the authenticity, reliability, originality and integrity of the raw data acquisition. Data management is performed, such as multimodality data management, text data processing and analysis, and feature system design and extraction, which come to some reliable conclusions. The user cannot delete, filter and clean the raw data without authorization to avoid error or bias.
Data mining aims to reveal hidden, unknown and potentially valuable information from a large quantity of psychologically related data. According to the cost of using data mining tools and the fault-tolerant standard of psychological data, the data mining methods includes classification, regression analysis, clustering analysis, association rules, feature analysis, variance analysis and web page mining. Also, the data mining algorithm includes neural networks, genetic algorithm, decision tree and fuzzy set. Every method has its own merits, demerits and limiting conditions. Data collected have contained information related to the temperament, characteristics and emotions of personnel psychological characteristics, which need to establish semantic analysis dictionary to explain the connections between psychological data and the risks. Selecting and optimizing data mining methods need the joint efforts of the safety practitioners, data managers and data analysts, thus extracting the hidden and valuable psychological knowledge from the stream of big data will be faster and more efficient.
Psychological related data acquisition and processing has become more convenient and intelligent as devices are tending to be smaller and smarter. Also, the development of information technology and its own software and hardware component gradually mature. Study tools of safety psychology have changed from data acquisition to results visualization, especially behavioral data acquisition of study objects at the individual and group level. With the arrival of the era of big data, it has reached the basic condition in analysis visualizations, data mining, predictive analytic, semantic search, data management and data storage. The data logging from wearables, smart devices and virtual reality automatically store in a data warehouse to improve the authenticity and accuracy of raw data, which interact with the cloud in the form of electronic data transmission to form data-driven decision-making models, then the results have displayed in visualization ways.
The rising complexity of safety psychological problems means it is now imperative that we take scientific and rigorous steps to ensure BDSP can be better applied to people’s lives and work. On the basis of the step of big data mining and the characteristic of safety psychology, the models of BDSP were concluded as follows: (i) Data acquisition. Data acquisition is the application foundation of big data and its methods are now include three categories: device sensors, system logs and web crawlers. (ii) Data preprocessing. We need to take preprocessed methods due to data imperfection of missing, repetition, noise, inconsistency and high dimension, including data cleaning, data integration, data transformation and data reduction. (iii) Data analysis and mining. This step is a key of big data processing which can be simplified into psychological data annotation, feature design and extraction, safety psychological model established and model improvement continuous. (iv) Data description. This step is the description of the psychological knowledge and rule from a large amount of data in a visual form, which enables users to observe and analyze the results more efficiently.
Application of BDSP is a complex activity which integrates theory level, information technology and application range into an organic whole. On this basis, we attempt to construct a three-dimensional structure of BDSP, as shown in Figure 4. To further clarify the level of BDSP, more detailed explanations can be seen as follows.
The promotion, development, dissemination and application of BDSP are the theoretical cornerstones, including the application characteristics of safety psychology and the technological superiority of big data. The theoretical basis of BDSP is divided into four levels. (i) The first level is the theoretical construction of the definition, connotation and attribution of BDSP, namely what is BDSP. (ii) The second level is the theoretical algorithm for the statistics, analysis and mining of BDSP, namely that classical algorithms are optimized to accommodate semantics types or new algorithms are developed for psychological characteristics. (iii) The third level is the theoretical practice for the information service of BDSP, namely the impact of psychological state of the worker on the risk evolution, which improves industrial facilities and work environments by psychological knowledge and rules. (iv) The fourth level is the theoretical innovation for the current challenge and future development trend of BDSP. The theoretical content of BDSP will inevitably change and adjust as the big data technology tends to mature.
Platform construction of big data mainly includes the module of data acquisition, storage, process and visualization. Every module is supported by its own hardware or software technology. The information technology of data acquisition, memory, processing and visualization will now be summarized as follows: (i) Currently, big data acquisition platforms include Apache Flume, Fluentd, Logstash, Chukwa, Scribe and Splunk Forwarder, which provide reliable and scalable data acquisition ways. (ii) The database system is a warehouse to organize, store and manage data, including relational database (e.g., Oracle, DB2, MySQL) and non-relational database (e.g., NoSql, Cloudant). Data warehouse, as one of the data integration frameworks, is an effective way to solve the problems of data analysis and to apply big data. (iii) Many data statistical and analytical techniques have emerged to solve the problem of massive data, data consistency, high concurrency and high availability in big data mining. Data algorithms should satisfy the technological and practical needs. (iv) Data visualization tools (e.g., Jupyter, Tableau, Google Chart, D3.js) will help users to identify future trends and patterns of psychological issues so as to make evidence-based safety decisions.
The advantages of BDSP are to provide users with potential, worthwhile and practical information to solve safety psychological issues. Application range can be classified into individual, small-group, large-group and industrial levels according to the volume of study object and the requirement of information services (Kang et al., 2017b). Individual psychology refers to the psychological phenomena and behavioral pattern at the individual level, focusing on people’s psychological process, state and feature. Group psychology and industrial psychology focus on the psychological insight at the statistical level, which guides significance to intervene climates in safety activities. It should be noted that there is no clear-cut distinction between the small-group psychology and the large-group psychology. Users will obtain help from psychological insights, for example, (i) Individual BDSP provide the worker with an early warning service when they are faced with danger. (ii) Group BDSP provide the enterprise or department with feedback or correction services in safety education and safety management. (iii) Industrial BDSP provide a safety climate to intervene in the crisis incident.
With the growing amount of psychological related data, the good news is that the path is relatively straightforward to extract psychological information from big data, but the challenge lies in how to effectively capture, manage and extract them (Demystifying Big Data, 2012). Despite this, big data will help researchers to model, analyze and predict the psychological influence mechanism in the safety domain, some challenges still need to be outlined due to big data constraints.
The inherent defects of data bring challenges to the development of BDSP:
(1) Data are obtained from the third-party data source in the present situation. The contents of those third-party data sometimes differ from each other, which may lead to invalidity and even faults in the mining results.
(2) The system has collected data that cannot completely reflect people’s psychological states due to the limitation of software input. In addition, multimodal, semantic analysis data needs to be considered. Semantic analysis techniques analyzing and categorizing psychological texts are relatively mature, with the support of the semantic dictionary (e.g., LIWC, Textmind), but the semantic analysis of video and audio is far from maturity.
(3) The deviation of personal characteristics has affected the representativeness of raw data (Kern et al., 2016), for example, some people hide their extraversion in the social network while they reveal an introversion characteristic in real life. Besides, online water armies, especially internet robots, can release false information by imitating human network activities, which decrease the reliability of the semantic analytic results.
(4) A large amount of data does not mean that they include the entire study objects (Weeg et al., 2015). The software presents an inevitable feature of users’ age level, such as young, middle-aged or aged, which will bring about a phenomenon of survivorship bias. In addition, it is ignored that phenomenon like the late report, the false report and the missing report exist during data collection, which will affect the reliability of case analysis methods.
(5) Under current circumstances, it is difficult to take on real-time collection and processing people’s psychological data on a large scale in the industrial field, especially in developing countries, since employers haven’t realized the responsibility of paying attention to employees’ psychological states.
(6) Traditional database methods are optimized for faster access and summarization of data users that they want to query. It has caused a situation where data satisfy this pattern rather than the opposite (Dhar, 2013), which is not able to discover new psychological knowledge and rules.
Big data of safety psychology cannot replace the traditional method of safety psychology at current stages. First, BDSP currently focuses on the theoretical aspects and is now at the initial development stage, unable to resolve all psychological problems of safety domain. BDSP has become an essential complement to traditional safety psychology. Moreover, the traditional method of safety psychology includes the experimental method, sampling statistics and case analysis, which are adequate to address safety psychological issue at a micro level. It can verify the accuracy of psychological knowledge excavated by big data to improve the reliability of the conclusion. Lastly, as a study hotspot of safety psychology, advantages and defects of BDSP will gradually emerge with deeper research and study as well as the development of information technology.
Ethical issues of BDSP deserve serious consideration. Some studies have revealed that possible violations in big data are against user privacy (Barnaghi et al., 2013; Sivarajah et al., 2017). On the one hand, data collection and mining inevitably involves users’ private information, especially as users’ characteristics and habits are under analysis by big data, increasing the frequency of harassment services and causing privacy intrusions and invasive marketing. On the other hand, data are viewed as a commercial resource for organizations and not for the public. Unlicensed web crawlers will interfere with the normal running of the network system, and anti-crawling programs of system setup also affect the quality of data collected.
Traditional safety psychology is not developed enough to solve human psychological problems in complex industrial production sometimes. Fortunately, there is an imminent need to convert such data into useful information and knowledge in industrial safety due to the wide availability of huge amounts of psychological data. Big data now becomes a new way to analyze people’s psychological states and behavior tendencies by processing a large amount of data. It is of great significance to discuss how big data is applied in the field of safety psychology. As an innovative approach aiming to excavate psychology related insight in industrial safety domain, BDSP offers some potential and valuable psychological knowledge and rules for safety practitioners to reduce the accident and to create a better working environment. In conclusion, BDSP significantly contributes to industrial safety and deserves thorough research by related scholars.
However, it is not enough for big data to be viewed as an important driving force to solve the psychological issues in the safety domain because it lacks supporting theories for guiding the application big data in safety psychology field. Under these circumstances, the main aim of this work is how to integrate big data into safety psychology and how to use it as a tool in the safety domain, providing the theoretical foundation of big data application in safety psychology field.
According to a literature review and comparative methods, we analyzed BDSP according to four aspects: (i) What BDSP are. First, the defect of the traditional study of safety psychology was analyzed and how big data extracts psychological rules were briefly reviewed. Then, the feasibility of BDSP was verified and the concept and aim of BDSP were proposed. Lastly, the differences between BDSP and traditional sample data were analyzed from 12 aspects. (ii) What the types and characteristics of BDSP are. First, seven classification standards of BDSP were analyzed to better understand its future development trends. Then, seven basic characteristics of BDSP were put forward according to the layer of dataset, technology and application. (iii) What the framework and structure of BDSP are. First, the framework of BDSP was constructed from four perspectives which are data requirements, study method, study tool, and processing step, which effectively manage the stream of big data. Then, a three-dimensional structure of BDSP was constructed at a theoretical level, information technology, and application range, by which its contents were clarified. (iv) What the challenges of BDSP are. It has outlined the challenge in terms of data inherent defect, BDSP insufficiency and ethical issues. These issues certainly require attention when applying big data in safety psychology research.
It is clear that big data plays an important role in the field of safety psychology. This study provides guidance to the evidence-based services in behavior management, which is valuable to help safety practitioners solve psychological issues in the aspects of safety management, safety education and safety enforcement.
No datasets were generated or analyzed for this study.
LK contributed to the conception of the study. LK, CW, and BW contributed significantly to the manuscript preparation. LK wrote the manuscript. CW and BW helped to perform the analysis with constructive discussions.
This work was supported by the National Natural Science Foundation of China (Grant No. 51534008) and the Fundamental Research Funds for the Central Universities of Central South University (Grant No. 2019zzts304).
The authors declare that the research was conducted in the absence of any commercial or financial relationships that could be construed as a potential conflict of interest.
Abou El-Nasr, M., and Shaban, H. (2015). Low-power and reliable communications for UWB-based wireless monitoring sensor networks in underground mine tunnels. Int. J. Distrib. Sens. Netw. 11, 1–11. doi: 10.1155/2015/456460
Adjerid, I., and Kelley, K. (2018). Big data in psychology: a framework for research advancement. Am. Psychol. 73, 899–917. doi: 10.1037/amp0000190
Barnaghi, P., Sheth, A., and Henson, C. (2013). From data to actionable knowledge: big data challenges in the web of things [guest editors’ introduction]. IEEE Intell. Syst. 28, 6–11. doi: 10.1109/MIS.2013.142
Boyd, D., and Crawford, K. (2012). Critical questions for big data: provocations for a cultural, technological, and scholarly phenomenon. Inf. Commun. Soc. 15, 662–679. doi: 10.1080/1369118X.2012.678878
Candás, J. L. C., Peláez, V., López, G., Fernández, M. Á.,Álvarez, E., and Díaz, G. (2014). An automatic data mining method to detect abnormal human behaviour using physical activity measurements. Pervasive Mob. Comput. 15, 228–241. doi: 10.1016/j.pmcj.2014.09.007
Chen, E. E., and Wojcik, S. P. (2016). A practical guide to big data research in psychology. Psychol. Methods 21, 458–474. doi: 10.1037/met0000111
Cheung, M. W. L., and Jak, S. (2016). Analyzing big data in psychology: a split/analyze/meta-analyze approach. Front. Psychol. 7:738. doi: 10.3389/fpsyg.2016.00738
Cree, T., and Kelloway, E. K. (1997). Responses to occupational hazards: exit and participation. J. Occup. Health Psychol. 2, 304–311. doi: 10.1037/1076-8998.2.4.304
Curini, L., Iacus, S., and Canova, L. (2015). Measuring idiosyncratic happiness through the analysis of twitter: an application to the italian case. Soc. Indic. Res. 121, 525–542. doi: 10.1007/s11205-014-0646-2
Demystifying Big Data (2012). A Practical Guide to Transforming the Business of Government. Washington, DC: TechAmerica Foundation’s Federal Big Data Commission.
Fan, J., Han, F., and Liu, H. (2014). Challenges of big data analysis. Natl. Sci. Rev. 1, 293–314. doi: 10.1093/nsr/nwt032
Fisher, G. G., Chaffee, D. S., Tetrick, L. E., Davalos, D. B., and Potter, G. G. (2017). Cognitive functioning, aging, and work: a review and recommendations for research and practice. J. Occup. Health Psychol. 22, 314–336. doi: 10.1037/ocp0000086
Giorgi, G., Arcangeli, G., Mucci, N., and Cupelli, V. (2015). Economic stress in the workplace: the impact of fear of the crisis on mental health. Work 51, 135–142. doi: 10.3233/WOR-141844
Giorgi, G., Montani, F., Fiz-Perez, J., Arcangeli, G., and Mucci, N. (2016). Expatriates’ multiple fears, from terrorism to working conditions: development of a model. Front. Psychol. 7:1571. doi: 10.3389/fpsyg.2016.01571
Golder, S. A., and Macy, M. W. (2011). Diurnal and seasonal mood vary with work, sleep, and daylength across diverse cultures. Science 333, 1878–1881. doi: 10.1126/science.1202775
Guo, S. Y., Ding, L. Y., Luo, H. B., and Jiang, X. Y. (2016). A Big-Data-based platform of workers’ behavior: observations from the field. Accid. Anal. Prev. 93, 299–309. doi: 10.1016/j.aap.2015.09.024
Guzzo, R. A., Fink, A. A., King, E., Tonidandel, S., and Landis, R. S. (2015). Big data recommendations for industrial–organizational psychology. Ind. Organ. Psychol. 8, 491–508. doi: 10.1017/iop.2015.40
Halevy, A., Norvig, P., and Pereira, F. (2009). The unreasonable effectiveness of data. IEEE Intell. Syst. 24, 8–12. doi: 10.1109/MIS.2009.36
Harlow, L. L., and Oswald, F. L. (2016). Big data in psychology: introduction to the special issue. Psychol. Methods 21, 447–457. doi: 10.1037/met0000120
Huang, L., Wu, C., Wang, B., and Ouyang, Q. (2018a). A new paradigm for accident investigation and analysis in the era of big data. Process Saf. Prog. 37, 42–48. doi: 10.1002/prs.11898
Huang, L., Wu, C., Wang, B., and Ouyang, Q. (2018b). Big-data-driven safety decision-making: a conceptual framework and its influencing factors. Saf. Sci. 109, 46–56. doi: 10.1016/j.ssci.2018.05.012
Huyghebaert, T., Gillet, N., Fernet, C., Lahiani, F. J., Chevalier, S., and Fouquereau, E. (2017). Investigating the longitudinal effects of surface acting on managers’ functioning through psychological needs. J. Occup. Health Psychol. 23, 207–222. doi: 10.1037/ocp0000080
Jones, N. M., Wojcik, S. P., Sweeting, J., and Silver, R. C. (2016). Tweeting negative emotion: an investigation of Twitter data in the aftermath of violence on college campuses. Psychol. Methods 21, 526–541. doi: 10.1037/met0000099
Kang, L., Huang, R., Wu, C., and Zhang, W. (2017a). Research on fundamental problems of safety psychology big data. J. Saf. Sci. Technol. 13, 5–10. doi: 10.11731/j.issn.1673-193x.2017.07.001
Kang, L., Wu, C., and Huang, R. (2017b). Research on foundation of similarity safety psychology. Chin. Saf. Sci. J. 27, 19–24. doi: 10.16265/j.cnki.issn1003-3033.2017.04.004
Kern, M. L., Park, G., Eichstaedt, J. C., Schwartz, H. A., Sap, M., Smith, L. K., et al. (2016). Gaining insights from social media language: methodologies and challenges. Psychol. Methods 21, 507–525. doi: 10.1037/met0000091
Leivada, E., D’Alessandro, R., and Grohmann, K. K. (2019). Eliciting big data from small, young, or non-standard languages: 10 experimental challenges. Front. Psychol. 10:313. doi: 10.3389/fpsyg.2019.00313
Lin, J. (2015). On building better mousetraps and understanding the human condition: reflections on big data in the social sciences. Ann. Am. Acad. Pol. Soc. Sci. 659, 33–47. doi: 10.1177/0002716215569174
Manyika, J., Chui, M., Brown, B., Bughin, J., Dobbs, R., Roxburgh, C., et al. (2011). Big Data: The Next Frontier for Innovation, Competition, and Productivity. New York, NY: McKinsey Global Institute.
Marvin, H. J., Janssen, E. M., Bouzembrak, Y., Hendriksen, P. J., and Staats, M. (2017). Big data in food safety: an overview. Crit. Rev. Food Sci. Nutr. 57, 2286–2295. doi: 10.1080/10408398.2016.1257481
Mayer-Schönberger, V., and Cukier, K. (2013). Big Data–A Revolution that Will Transform How We Live, Think and Work. London: John Murray.
Mucci, N., Giorgi, G., Roncaioli, M., Perez, J. F., and Arcangeli, G. (2016). The correlation between stress and economic crisis: a systematic review. Neuropsychiatr. Dis. Treat. 12, 983–993. doi: 10.2147/NDT.S98525
Ouyang, Q., Wu, C., and Huang, L. (2018). Methodologies, principles and prospects of applying big data in safety science research. Saf. Sci. 101, 60–71. doi: 10.1016/j.ssci.2017.08.012
Paxton, A., and Griffiths, T. L. (2017). Finding the traces of behavioral and cognitive processes in big data and naturally occurring datasets. Behav. Res. Methods 49, 1630–1638. doi: 10.3758/s13428-017-0874-x
Sacristán, J. A., and Dilla, T. (2015). No big data without small data: learning health care systems begin and end with the individual patient. J. Eval. Clin. Pract. 21, 1014–1017. doi: 10.1111/jep.12350
Shi, Q., and Abdel-Aty, M. (2015). Big data applications in real-time traffic operation and safety monitoring and improvement on urban expressways. Transp. Res. Part C Emerg. Technol. 58, 380–394. doi: 10.1016/j.trc.2015.02.022
Sivarajah, U., Kamal, M. M., Irani, Z., and Weerakkody, V. (2017). Critical analysis of big data challenges and analytical methods. J. Business Res. 70, 263–286. doi: 10.1016/j.jbusres.2016.08.001
Vaitla, B., Bosco, C., Alegana, V., Bird, T., Pezzulo, C., Hornby, G., et al. (2017). Big Data and The Well-Being of Women and Girls: Applications On The Social Scientific Frontier. Washington, DC: Data2x.
Vie, L. L., Griffith, K. N., Scheier, L. M., Lester, P. B., and Seligman, M. E. (2013). The person-event data environment: leveraging big data for studies of psychological strengths in soldiers. Front. Psychol. 4:934. doi: 10.3389/fpsyg.2013.00934
Wang, B., Wu, C., Shi, B., and Huang, L. (2017). Evidence-based safety (EBS) management: a new approach to teaching the practice of safety management (SM). J. Saf. Res. 63, 21–28. doi: 10.1016/j.jsr.2017.08.012
Wang, J., Gan, S., Zhao, N., Liu, T., and Zhu, T. (2016). Chinese mood variation analysis based on sina weibo. J. Univ. Chinese Acad. Sci. 33, 815–824. doi: 10.7523/j.issn.2095-6134.2016.06.014
Wang, Y., Yang, B., Luo, Y., He, J., and Tan, H. (2015). The application of big data mining in risk warning for food safety. Asian Agric. Res. 7, 83–86.
Weeg, C., Schwartz, H. A., Hill, S., Merchant, R. M., Arango, C., and Ungar, L. (2015). Using twitter to measure public discussion of diseases: a case study. JMIR Public Health Surveill. 1:e6. doi: 10.2196/publichealth.395
Ye, Y., Xu, Y., Zhu, Y., Liang, J., Lan, T., and Yu, M. (2016). The characteristics of moral emotions of chinese netizens towards an anthropogenic hazard: a sentiment analysis on weibo. Acta Psychol. Sin. 48, 290–304. doi: 10.3724/SP.J.1041.2016.00290
Keywords: big data, multidisciplinary psychology, big data of safety psychology, organizational psychology, industrial safety
Citation: Kang L, Wu C and Wang B (2019) Principles, Approaches and Challenges of Applying Big Data in Safety Psychology Research. Front. Psychol. 10:1596. doi: 10.3389/fpsyg.2019.01596
Received: 02 May 2019; Accepted: 25 June 2019;
Published: 09 July 2019.
Edited by:
Giulio Arcangeli, University of Florence, ItalyReviewed by:
Gabriele Giorgi, Università Europea di Roma, ItalyCopyright © 2019 Kang, Wu and Wang. This is an open-access article distributed under the terms of the Creative Commons Attribution License (CC BY). The use, distribution or reproduction in other forums is permitted, provided the original author(s) and the copyright owner(s) are credited and that the original publication in this journal is cited, in accordance with accepted academic practice. No use, distribution or reproduction is permitted which does not comply with these terms.
*Correspondence: Liangguo Kang, a2FuZ2xpYW5nZ3VvQGNzdS5lZHUuY24=; Bing Wang, c2FmZWJveUBjc3UuZWR1LmNu
Disclaimer: All claims expressed in this article are solely those of the authors and do not necessarily represent those of their affiliated organizations, or those of the publisher, the editors and the reviewers. Any product that may be evaluated in this article or claim that may be made by its manufacturer is not guaranteed or endorsed by the publisher.
Research integrity at Frontiers
Learn more about the work of our research integrity team to safeguard the quality of each article we publish.