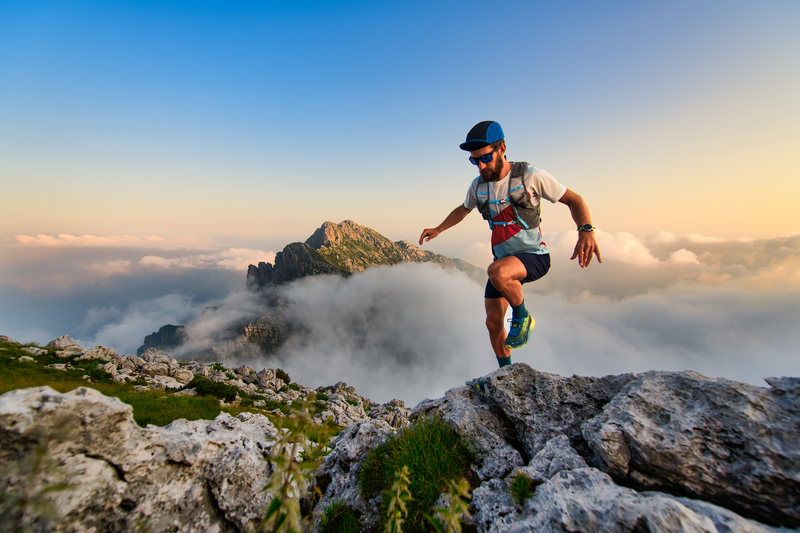
94% of researchers rate our articles as excellent or good
Learn more about the work of our research integrity team to safeguard the quality of each article we publish.
Find out more
PERSPECTIVE article
Front. Psychol. , 13 June 2018
Sec. Cognition
Volume 9 - 2018 | https://doi.org/10.3389/fpsyg.2018.00927
This article is part of the Research Topic Cognitive Aspects of Interactive Technology Use: From Computers to Smart Objects and Autonomous Agents View all 19 articles
Intelligent tutor systems (ITSs) in mobile devices take us through learning tasks and make learning ubiquitous, autonomous, and at low cost (Nye, 2015). In this paper, we describe guided embodiment as an ITS essential feature for second language learning (L2) and aphasia rehabilitation (ARe) that enhances efficiency in the learning process. In embodiment, cognitive processes, here specifically language (re)learning are grounded in actions and gestures (Pecher and Zwaan, 2005; Fischer and Zwaan, 2008; Dijkstra and Post, 2015). In order to guide users through embodiment, ITSs must track action and gesture, and give corrective feed-back to achieve the users' goals. Therefore, sensor systems are essential to guided embodiment. In the next sections, we describe sensor systems that can be implemented in ITS for guided embodiment.
Today in L2 learning, ITSs transpose classroom activities as reading, listening, and making exercises in electronic environments (Holland et al., 2013). Similarly in ARe, a virtual therapist in a tablet helps patients in the treatment of verbal anomia by presenting pictures (Lavoie et al., 2016). Virtual therapists do basically what a human therapist would do, i.e., they ask patients to name the pictures presented (Brandenburg et al., 2013; Kurland et al., 2014; Szabo and Dittelman, 2014).
Both domains, L2 and ARe, still treat language a purely mentalistic process, a manipulation of symbols in our minds (Fodor, 1976, 1983). Consequently, symbols such as written words or pictures representing the word's semantics are the base of main stream language educational and rehabilitation methods. Despite this, in the last three decades, a growing number of studies have converged to suggest that language as a cognitive capacity is grounded in our bodily experiences in the environment, in perception and action (Lakoff, 2012; Dijkstra and Post, 2015; Borghi and Zarcone, 2016). Words are not symbols any more. Instead, they have been described as “experience related brain networks” (Pulvermüller, 2002). Interestingly, not only concrete but also abstract vocabulary is rooted in the body. In a comprehensive review of neuroscientific studies, Meteyard and colleagues show that simple recognition of abstract words elicits activity in sensorimotor brain regions (Meteyard et al., 2012). This is explained by the fact that abstract concepts are also internalized by real experiences that in their turn are related to the body. Take for example the word love: it is embodied because acquired from concrete and experienced concepts, i.e., perceiving the partner physically, doing things with the partner, and so on. All these experiences converge to a metaphorical extension which is labeled as love.
In fact, first language acquisition is tightly connected to sensorimotor experiences (Inkster et al., 2016; Thill and Twomey, 2016). In infancy, the body is the main vehicle that collects experiences related to language units as nouns and verbs (Tomasello et al., 2017). Furthermore, very early in development, gestures make their appearance. They are precursors of spoken language (Mattos and Hinzen, 2015) and tightly bound to it. Language and gestures represent the two sides of the human communicative system (Kelly et al., 2010).
In adult age, the body can be used as a tool to enhance memory for verbal information (Zimmer, 2001). This is achieved by performing gestures to words or phrases that are to be memorized. The effect of gestures on memory for verbal information has been named “enactment effect” (EE) Engelkamp and Zimmer (1985) and “self-performed task effect” (Cohen, 1981). The EE is robust and has been extensively investigated with different materials, tests, and populations (Von Essen and Nilsson, 2003). In memory research, the EE effect has been reconducted to a motor trace that the gesture leaves in words' representations (Engelkamp, 1998).
Also, in second language learning, self-performed gestures accompanying words enhance memory performance compared to just reading the words and/or listening to them (Macedonia, 2014), in the short and in the long term (Macedonia and Klimesch, 2014). In a study with functional Magnet Resonance Imaging (fMRI), Macedonia and Mueller (2016) have shown that passive recognition of second language words trained with gestures activates extended sensorimotor networks. These networks involve motor cortices and subcortical structures as the basal ganglia, and the cerebellum. They all participate to a large motor network. It is thus conceivable that retention is superior because words learned with gestures might engage procedural memory in addition to declarative memory (Nilsson and Bäckman, 1989). Interestingly, recent studies on patients with impaired procedural memory have demonstrated that the patients could not take advantage of learning through gestures (Klooster et al., 2014).
In aphasia, gestures produced by patients trying to communicate can easily be observed. These gestures fulfill compensatory functions (Göksun et al., 2015; Rose et al., 2016) if the patients' language is impoverished or omitted (Pritchard et al., 2015). However, because of the high variance in lesion patterns, age of the patients, patho-linguistic profile, intensity of intervention, etc., studies employing gestures and studies employing other therapeutic instruments are difficult to compare. Hence, effects of gestures on rehabilitation can be diverging (Kroenke et al., 2013). Main stream aphasia therapy is still constrained to the verbal modality and bans gestures as tool that might help to restore language networks (Pulvermüller, 2002). Nevertheless, a growing number of studies show that action and gesture can help support the missing side of the communicative coin (Rose, 2013). Whereas simple observation of action has a positive impact on word recovery (Bonifazi et al., 2013), observation followed by execution of action leads to better recovery results (Marangolo and Caltagirone, 2014). These studies pave the way for a novel understanding of aphasia therapy in which the body helps the mind to regain language functions, as long as brain structures serving procedural memory are not compromised (Klooster et al., 2014).
This is to say that humans need the body to acquire first language, to support memory for verbal information, to learn a second language, and to reacquire language functions disrupted by brain lesions. At this point, a core issue is to stress that embodiment of language needs active experience. In enactment research, it has long been known that it is not enough to observe gestures and actions, one must perform them (Cohen, 1981; Engelkamp et al., 1994). When interacting with an ITS, the user is first presented with the language to be trained and the gestures to be performed. Thereafter, the user must perform the actions and the gestures. Monitoring can make action performance accurate in execution. Thus, one component of the ITS must detect motion and gesture, compare it with a template and give feed-back on execution accuracy. Execution monitoring needs sensor systems.
Guided embodiment requires an interaction between ITS and user: A gesture representing a concept is performed by an ITS avatar. The user observes the gesture and imitates it. The user's gesture must be sensed during performance. Performance is evaluated by the system on the base of a template. Visual, auditory and or tactile feedback is given by the ITS (please see Figure 1).
First, a written word is presented to the user on a display simultaneously with a video in which an actor performs a representational gesture. The gesture can be presented by a human through a video or by an avatar, or an agent (Bergmann, 2015). Synchronously, an audio file of the word is played via loudspeaker.
Motion is the change of body position in time. Motion capturing occurs as a two-phases process. First, a single motion is sensed generating data (motion sensing) (Moeslund et al., 2006). Secondly, the data are sampled (motion sampling) and sequenced in time into a movement path, a so-called motion trajectory model. Depending on the location of the sensors used to detect the motion, Motion capturing can be subdivided into two categories: infrastructure based or through wearables. Infrastructure-based systems rely on hardware that is rigidly mounted inside a room as high-speed infrared cameras in a gait analysis laboratory, or sensors in a blue screen environment. Infrastructure based systems use sensors with high power consumption.
Systems based on microwave, ultrasonic or radar sensors operate by emitting electromagnetic or sonic waves and sensing the echo received. Depending on the purpose of motion capturing, sensor technologies can vary. For example, ultrasonic motion detection is quite common in prenatal diagnostics (Birnholz et al., 1978). For remote vital sign detection radar-based motion detection is frequently used (Lubecke et al., 2002).
Vision-based systems (VBS), including single camera, multiple cameras, and depth camera systems, play the most important role in human motion capture. Sensors detect light which can be visible or invisible to the human eye which is emitted or reflected by the body or an object (Moeslund et al., 2006).
Single camera-based motion detection systems are present in notebooks, tablets, and mobiles. Although these systems often have a high-quality resolution, they operate with a single camera. A single camera cannot capture the motion of body parts that are occluded by other body parts. This results in an inaccurate or incomplete analysis of the motion.
Multiple camera systems with two or more cameras allow 3D capturing. Algorithms combining 2D images from the cameras calculate a 3D-resolution (Aggarwal and Cai, 1997; Cai and Aggarwal, 1999). In the 3D-resolution, the synchronized recordings are combined. The combination includes the positions of the cameras relative to each other and their angles of view. Multiple camera systems are used in rigid mounted setups, in laboratories or dedicated rooms for example in rehabilitation (gait analysis), and sports (motion analysis).
Depth cameras sense 3D-information by means of infrared light. They calculate the distance between the camera and a body in two ways. They project an invisible grid onto the scenery and sense the grid's deformations. Alternatively, they measure the distance to the scenery and they calculate the transfer time of the infrared light from the camera to the object. This second kind of depth camera is also called “Time-of-flight”-camera (ToF) (Barnachon et al., 2014; Cunha et al., 2016; Garn et al., 2016).
Depth camera systems with a single device do not overcome the problem of occluded parts (Han et al., 2013). However, they have an advantage: they provide information about the distance of each object or body within the camera's view relative to the camera's position. These systems do not rely on heuristics about proportions of the object in order to determine its distance. This information increases accuracy in calculating the position of a human body or object.
Wearables are sensors worn on the body. They are light-weighted and have low power consumption. They are often used in sports (Roetenberg et al., 2013). Among wearables, we find inertial measurement units (IMUs) and sensing textiles.
Inertial Measurement Units (IMUs) are small electronic devices that measure acceleration, angular changes and changes in the magnetic field surrounding the body or object (Roetenberg, 2006; Shkel, 2011). If the starting position is known, an approximate position at time t is can be calculated by implementing the changes in forces, angles and magnetic field from the starting position up to t. IMUs differ from camera-based systems: while the latter measure the absolute position of the body at every time point t, IMUs acquire a starting position and the movement's sequence.
IMUs are integrated into wearable objects and respond on minimal deviations of the sensors by showing a drift. This drift can sum up to false positions over time. Fusion algorithms combining filtering and validation of sensor are used to compensate, respectively minimize drifts values (Luinge and Veltink, 2005; Sabatini, 2011; Roetenberg et al., 2013).
Sensing textiles represent a novel way of capturing motion. They consist of fabrics containing enwoven pressure sensitive fibers. These fibers change their electric resistance depending on the pressure changes that they sense (Mazzoldi et al., 2002; Parzer et al., 2016). Clothes tailored with these fabrics enable to calculate movements of the body in a fine-grained way (Parzer et al., 2016). The choice of the adequate type of motion sensing technology depends on the application domain. In our case, sensing of human body movements for an ITS can be accomplished with four sensor technologies: camera, depth-camera, IMUs, and sensing textiles.
Vision-based systems (VBS) take pictures over time and analyze them in order to detect body parts. Thereafter, VBS transform the detected body parts into digital representations, into human body models. Common models are skeletal, joint-based (Badler and Smoliar, 1979; Han et al., 2017), and mesh-based (de Aguiar et al., 2007). For an overview and classification of the major techniques used for sampling 3D data, please see Aggarwal and Xia (2014).
Additionally, VBS can increase the accuracy of the human body model by markers as light-emitting diodes, passive reflectors or patterns. These markers are fixed on pre-defined body parts and map them to the according representation within the model. Marker-less systems use heuristics about shapes, dimensions, and relations between body parts estimating and calculating the model according to these constraints.
Body data are sampled and thereafter transferred into a digital form in constant periods of time. This is done in order to obtain the motion trajectory model needed. It represents the body parts and their changes in posture over the time of recording (Poppe, 2010). Hence, motion sampling results in a motion trajectory model.
In the literature, different approaches for matching motion trajectory models are discussed. Kollorz et al. (2008) ground their model on projections of image depth. Mitra and Acharya (2007) describe the use of hidden Markov models (Rabiner and Juang, 1986), finite-state machines (Marvin, 1967) and, neural networks (Lippmann, 1987). Other authors use a support-vector machine-based approach (Cristianini and Shawe-Taylor, 2000; Schuldt et al., 2004; Miranda et al., 2014). A template-based method for matching motion has been developed by Müller and Röder (2006). Stiefmeier et al. (2007) convert the motion trajectory model into strings of symbols. This is done in order to apply string matching algorithms that are faster in running analyses. Detailed reviews on vision-based human motion recognition methods are provided by Poppe (2010) and Weinland et al. (2011).
Embodiment-based ITS employed in language learning and rehabilitation need real-time processing of sensed gestures because of the immediate feedback on gesture accuracy that users need (Ganapathi et al., 2010).
Accuracy in sound reproduction is an important issue in both, second language learning and aphasia rehabilitation. Language output by the user is recorded and analyzed by different methods (Rabiner and Juang, 1993). Recent approaches employ complex models as neural networks for speech recognition (Hinton et al., 2012; Graves et al., 2013).
After a match between the sensed gesture or the voice and the template within the representing motion trajectory model has occurred, feedback can follow. It can be visual via the display, acoustical with sound through a speaker (built-in or external), and tactorial by means of a vibration given by the device. Feedback can be simple (i.e., a sound or synthesized speech).
In order to give an overview of the sensor technologies presented in the preceding sections, we created Table 1. It describes the degree of following characteristics: accuracy in motion sensing, ease of set up for an expert, mobility and size. Note that the description is done for the use of a professional (lab technician) and for an institution (language school or hospital). We do not consider ITS software, software processes, and design patterns, or aspects of user-interface design. For further reading, please see (Oppermann, 2002; Dillon, 2003; Carroll, 2006; Smith-Atakan, 2006; Preece et al., 2015).
In this paper, we describe two application domains for ITS following principles of guided embodiment: language (re-)learning and aphasia rehabilitation. So far, we have focused on the possible use of the ITS in an institution (school vs. hospital). However, considering that language learning and rehabilitation need massed practice (Pulvermüller et al., 2001; Kurland et al., 2014), ITS should accompany users during the learning task in their homes. Sensing textiles can represent an emerging field in guided embodiment for language learning and aphasia rehabilitation. A learning t-shirt could combine a few advantages: high accuracy in sensing motion, ease of use and possible vibration feedback. However, to our knowledge no such system is present to date on the market, even as a prototype.
To present, only single camera systems present in tablets and mobile phones are affordable and easy to use. Also, nearly everyone has an own device. Because of their size, single camera systems can be carried where users need them. Despite the fact that presently single cameras are not very accurate in motion capturing as described in the preceding section, they might become the instruments used in a near future.
Altogether, this brief overview highlights the fact that guided embodiment of language could be the way to enhance performance in learning and rehabilitation. However, more research in the field is needed.
MM has laid down the structure of this paper and written the sections on embodiment. FH and OW have written the sections on technologies for gesture performance monitoring.
Parts of the work of FH have been supported by the Austrian COMET-K2 program of the Linz Center of Mechatronics GmbH (LCM) and by the EU-funded H2020 ECSEL project SILENSE (ID 737487).
The authors declare that the research was conducted in the absence of any commercial or financial relationships that could be construed as a potential conflict of interest.
Aggarwal, J. K., and Cai, Q. (1997). “Human motion analysis: a review,” in IEEE Nonrigid and Articulated Motion Workshop, IEEE Comput. Soc (San Juan), 90–102.
Aggarwal, J. K., and Xia, L. (2014). Human activity recognition from 3D data : a review. Patt. Recogn. Lett. 48, 70–80. doi: 10.1016/j.patrec.2014.04.011
Badler, N. I., and Smoliar, S. W. (1979). Digital representations of human movement. ACM Comput. Surveys 11, 19–38. doi: 10.1145/356757.356760
Barnachon, M., Bouakaz, S., Boufama, B., and Guillou, E. (2014). Ongoing human action recognition with motion capture. Patt. Recogn. 47, 238–247. doi: 10.1016/j.patcog.2013.06.020
Bergmann, K. (2015). “Towards gesture-based literacy training with a virtual agent,” in Proc. Symposium on Multimodal Communication (Linköping), 113–121.
Birnholz, J. C., Stephens, J. C., and Faria, M. (1978). Fetal movement patterns: a possible means of defining neurologic developmental milestones in utero. Am. J. Roentgenol. 130, 537–540. doi: 10.2214/ajr.130.3.537
Bonifazi, S., Tomaiuolo, F., Altoè, G., Ceravolo, M. G., Provinciali, L., and Marangolo, P. (2013). Action observation as a useful approach for enhancing recovery of verb production: new evidence from aphasia. Eur. J. Phys. Rehabil. Med. 49, 473–481.
Borghi, A. M., and Zarcone, E. (2016). Grounding abstractness: abstract concepts and the activation of the mouth. Front. Psychol. 7:1498. doi: 10.3389/fpsyg.2016.01498
Brandenburg, C., Worrall, L., and Rodriguez, A. D. (2013). Mobile computing technology and aphasia: an integrated review of accessibility and potential uses. Aphasiology 27, 444–461. doi: 10.1080/02687038.2013.772293
Cai, Q., and Aggarwal, J. K. (1999). Tracking human motion in structured environments using a distributed-camera system. IEEE Trans. Patt. Anal. Mach. Intell. 21, 1241–1247. doi: 10.1109/34.809119
Carroll, J. M. (2006). “Human-computer interaction,” in Encyclopedia of Cognitive Science, ed L. Nadel (Chichester: John Wiley & Sons, Ltd), 1–4.
Cohen, R. L. (1981). On the generality of some memory laws. Scand. J. Psychol. 22, 267–281. doi: 10.1111/j.1467-9450.1981.tb00402.x
Cristianini, N., and Shawe-Taylor, J. (2000). An Introduction to Support Vector Machines and Other Kernel-based Learning Methods. Cambridge, MA: Cambridge University Press.
Cunha, J. P., Choupina, H. M., Rocha, A. P., Fernandes, J. M., Achilles, F., Loesch, A. M., et al. (2016). NeuroKinect: a novel low-cost 3Dvideo-EEG System for epileptic seizure motion quantification. PLoS ONE 11:e0145669. doi: 10.1371/journal.pone.0145669
de Aguiar, E., Theobalt, C., Stoll, C., and Seidel, H.-P. (2007). “Marker-less deformable mesh tracking for human shape and motion capture,” in 2007 IEEE Conference on Computer Vision and Pattern Recognition (IEEE), 1–8.
Dijkstra, K., and Post, L. (2015). Mechanisms of embodiment. Front. Psychol. 6:1525. doi: 10.3389/fpsyg.2015.01525
Dillon, A. (2003). “User interface design,” in MacMillan Encyclopedia of Cognitive Science, ed E. Nadel (Chichester: John Wiley & Sons, Ltd), 453–458.
Engelkamp, J., and Zimmer, H. D. (1985). Motor programs and their relation to semantic memory. German J. Psychol. 9, 239–254.
Engelkamp, J., Zimmer, H. D., Mohr, G., and Sellen, O. (1994). Memory of self-performed tasks: self-performing during recognition. Mem. Cogn. 22, 34–39. doi: 10.3758/BF03202759
Fischer, M. H., and Zwaan, R. A. (2008). Embodied language: a review of the role of the motor system in language comprehension. Q. J. Exp. Psychol. 61, 825–850. doi: 10.1080/17470210701623605
Fodor, J. A. (1983). The modularity of Mind : An Essay on Faculty Psychology. Cambridge, MA; London: MIT Press.
Ganapathi, V., Plagemann, C., Koller, D., and Thrun, S. (2010). “Real time motion capture using a single time-of-flight camera,” in 2010 IEEE Computer Society Conference on Computer Vision and Pattern Recognition (San Francisco, CA: IEEE), 755–762.
Garn, H., Member, S., Kohn, B., Dittrich, K., Wiesmeyr, C., Kloesch, G., et al. (2016). “3D Detection of Periodic Limb Movements in Sleep,” in 2016 IEEE 38th Annual International Conference of the Engineering in Medicine and Biology Society (EMBC), (Orlando, FL), 427–430.
Göksun, T., Lehet, M., Malykhina, K., and Chatterjee, A. (2015). Spontaneous gesture and spatial language: Evidence from focal brain injury. Brain Lang. 150, 1–13. doi: 10.1016/j.bandl.2015.07.012
Graves, A., Mohamed, A., and Hinton, G. (2013). Speech “Recognition with deep recurrent neural networks,” in 2013 IEEE International Conference on Acoustics, Speech and Signal Processing (Vancouver, BC), 150. 6645–6649.
Han, F., Reily, B., Hoff, W., and Zhang, H. (2017). Space-time representation of people based on 3D skeletal data : a review. Comp. Vis. Image Understanding, 158, 85–105. doi: 10.1016/j.cviu.2017.01.011
Han, J., Shao, L., Xu, D., and transactions on Shotton, - J. (2013). “Enhanced computer vision with microsoft kinect sensor: a review,” in IEEE Transactions on Cybernetics. Available online at: http://ieeexplore.ieee.org/abstract/document/6547194/
Hinton, G., Deng, L., Yu, D., Dahl, G., Mohamed, A. R., Jaitly, N., et al. (2012). Deep neural networks for acoustic modeling in speech recognition: the shared views of four research groups. IEEE Signal Process. Mag. 29, 82–97. doi: 10.1109/MSP.2012.2205597
Holland, M. V., Sams, M. R., and Kaplan, J. D. (2013). Intelligent Language Tutors: Theory Shaping Technology. Available online at: http://books.google.com/books?hl=enandlr=andid=Db9dAgAAQBAJandoi=fndandpg=PP1andots=ZVkvAFWNfzandsig=mYinl4JTfiNalluHWsiTv0BhDBs
Inkster, M., Wellsby, M., Lloyd, E., and Pexman, P. M. (2016). Development of embodied word meanings: sensorimotor effects in children's lexical processing. Front. Psychol. 7:317. doi: 10.3389/fpsyg.2016.00317
Kelly, S. D., Ozyürek, A., and Maris, E. (2010). Two sides of the same coin: speech and gesture mutually interact to enhance comprehension. Psychol. Sci. 21, 260–267. doi: 10.1177/0956797609357327
Klooster, N. B., Cook, S. W., Uc, E. Y., and Duff, M. C. (2014). Gestures make memories, but what kind? Patients with impaired procedural memory display disruptions in gesture production and comprehension. Front. Hum. Neurosci. 8:1054. doi: 10.3389/fnhum.2014.01054
Kollorz, E., Penne, J., Hornegger, J., and Barke, A. (2008). Gesture recognition with a Time-Of-Flight camera. Int. J. Intell. Syst. Technol. Appl. 5:334. doi: 10.1504/IJISTA.2008.021296
Kroenke, K. M., Kraft, I., Regenbrecht, F., and Obrig, H. (2013). Lexical learning in mild aphasia: gesture benefit depends on patholinguistic profile and lesion pattern. Cortex 49, 2637–2649. doi: 10.1016/j.cortex.2013.07.012
Kurland, J., Wilkins, A. R., and Stokes, P. (2014). iPractice: Piloting the effectiveness of a tablet-based home practice program in aphasia treatment. Semin. Speech Lang. 35, 51–63. doi: 10.1055/s-0033-1362991
Lakoff, G. (2012). Explaining embodied cognition results. Top. Cogn. Sci. 4, 773–785. doi: 10.1111/j.1756-8765.2012.01222.x
Lavoie, M., Routhier, S., Légaré, A., and Macoir, J. (2016). Treatment of verb anomia in aphasia: efficacy of self-administered therapy using a smart tablet. Neurocase 22, 109–118. doi: 10.1080/13554794.2015.1051055
Lippmann, R. (1987). An introduction to computing with neural nets. IEEE ASSP Mag. 4, 4–22. doi: 10.1109/MASSP.1987.1165576
Lubecke, O. B., Ong, P. W., and Lubecke, V. M. (2002). “10 GHz Doppler radar sensing of respiration and heart movement,” in Proceedings of the IEEE Annual Northeast Bioengineering Conference, NEBEC, Vol. 2002 (Janua, IEEE), 55–56.
Luinge, H. J., and Veltink, P. H. (2005). Measuring orientation of human body segments using miniature gyroscopes and accelerometers. Med. Biol. Eng. Comput. 43, 273–282. doi: 10.1007/BF02345966
Macedonia, M. (2014). Bringing back the body into the mind: gestures enhance word learning in foreign language. Front. Psychol. 5:1467. doi: 10.3389/fpsyg.2014.01467
Macedonia, M., and Klimesch, W. (2014). Long-term effects of gestures on memory for foreign language words trained in the classroom. Mind Brain Educ. 8, 74–88. doi: 10.1111/mbe.12047
Macedonia, M., and Mueller, K. (2016). Exploring the neural representation of novel words learned through enactment in a word recognition task. Front. Psychol. 7:953. doi: 10.3389/fpsyg.2016.00953
Marangolo, P., and Caltagirone, C. (2014). Options to enhance recovery from aphasia by means of non-invasive brain stimulation and action observation therapy. Expert Rev. Neurother. 14, 75–91. doi: 10.1586/14737175.2014.864555
Marvin, L. M. (1967). Computation : Finite and Infinite Machines. Prentice-Hall. Available online at: https://dl.acm.org/citation.cfm?id=1095587
Mattos, O., and Hinzen, W. (2015). The linguistic roots of natural pedagogy. Front. Psychol. 6:1424. doi: 10.3389/fpsyg.2015.01424
Mazzoldi, A., De Rossi, D., Lorussi, F., Scilingo, E. P., and Paradiso, R. (2002). Smart textiles for wearable motion capture systems. Autex Res. J. 2, 199–203.
Meteyard, L., Cuadrado, S. R., Bahrami, B., and Vigliocco, G. (2012). Coming of age: a review of embodiment and the neuroscience of semantics. Cortex 48, 788–804. doi: 10.1016/j.cortex.2010.11.002
Miranda, L., Vieira, T., Martínez, D., Lewiner, T., Vieira, A. W., and Mario, M. F. (2014). Online gesture recognition from pose kernel learning and decision forests. Patt. Recogn. Lett. 39, 65–73. doi: 10.1016/j.patrec.2013.10.005
Mitra, S., and Acharya, T. (2007). Gesture recognition: a survey. IEEE Trans. Syst. Man Cybernet. 37, 311–324. doi: 10.1109/TSMCC.2007.893280
Moeslund, T. B., Hilton, A., and Krüger, V. (2006). A survey of advances in vision-based human motion capture and analysis. Comput. Vis. Image Understand. 104, 90–126. doi: 10.1016/j.cviu.2006.08.002
Müller, M., and Röder, T. (2006). “Motion templates for automatic classification and retrieval of motion capture data,” in Proceedings of the ACM SIGGRAPH/Eurographics Symposium on Computer Animation (Eurographics Association), 137–146.
Nilsson, L. G., and Bäckman, L. (1989). Implicit Memory and the Enactment of Verbal Instructions. Implicit Memory: Theoretical Issues. Available online at: http://books.google.com/books?hl=enandlr=andid=xmG8XVnxlV8Candoi=fndandpg=PA173anddq=free+recall+and+enactmentandots=g7tKpUmHEnandsig=vKkzXWgReaJxwjvSc2o3j2AWPis
Nye, B. D. (2015). Intelligent tutoring systems by and for the developing world: a review of trends and approaches for educational technology in a global context. Int. J. Artif. Intell. Educ. 25:177. doi: 10.1007/s40593-014-0028-6
Oppermann, R. (2002). “User-interface design,” in Handbook on Information Technologies for Education and Training (Berlin; Heidelberg: Springer), 233–248.
Parzer, P., Probst, K., Babic, T., Rendl, C., Vogl, A., Olwal, A., et al. (2016). “FlexTiles,” in Proceedings of the 2016 CHI Conference Extended Abstracts on Human Factors in Computing Systems - CHI EA'16 (New York, NY: ACM Press), 3754–3757.
Pecher, D., and Zwaan, R. A. (2005). Grounding Cognition: The Role of Perception and Action in Memory, Language, and Thinking. Available online at: http://books.google.com/books?hl=enandlr=andid=RaxTkckBnh4Candoi=fndandpg=PP1anddq=embodiment+cognitionandots=EHGQcDOX-zandsig=xD68EMdN-rrSqWyMROqqF9mTzxU
Poppe, R. (2010). A survey on vision-based human action recognition. Image Vis. Comput. 28, 976–990. doi: 10.1016/j.imavis.2009.11.014
Preece, J., Sharp, H., and Rogers, Y. (2015). Interaction Design: Beyond Human-Computer Interaction, 4th Edn. New York, NY: Wiley.
Pritchard, M., Dipper, L., Morgan, G., and Cocks, N. (2015). Language and iconic gesture use in procedural discourse by speakers with aphasia. Aphasiology 29, 826–844. doi: 10.1080/02687038.2014.993912
Pulvermüller, F. (2002). The Neuroscience of Language : On Brain Circuits of Words and Serial Order. Cambridge; New York, NY: Cambridge University Press.
Pulvermüller, F., Neininger, B., Elbert, T., Mohr, B., Rockstroh, B., Koebbel, P., et al. (2001). Constraint-induced therapy of chronic aphasia after stroke. Stroke 32, 1621–1626. doi: 10.1161/01.STR.32.7.1621
Rabiner, L. R., and Juang, B. H. (1986). An introduction to hidden markov models. IEEE ASSP Mag. 3, 4–16. doi: 10.1109/MASSP.1986.1165342
Rabiner, L. R., and Juang, B. H. (1993). Fundamentals of Speech Recognition. PTR Prentice Hall. Available online at: http://www.citeulike.org/group/10577/article/308923
Roetenberg, D. (2006). Inertial and Magnetic Sensing of Human Motion. These de Doctorat, Ph.D., University of Twente.
Roetenberg, D., Luinge, H., and Slycke, P. (2013). Xsens MVN : Full 6DOF Human Motion Tracking Using Miniature Inertial Sensors. Technical report, Vol. 3.
Rose, M. L. (2013). Releasing the constraints on aphasia therapy: the positive impact of gesture and multimodality treatments. Am. J. Speech Lang. Pathol. 22, S227–S239. doi: 10.1044/1058-0360(2012/12-0091)
Rose, M. L., Mok, Z., and Sekine, K. (2016). Communicative effectiveness of pantomime gesture in people with aphasia. Int. J. Lang. Commun. Disord. 52, 227–237.doi: 10.1111/1460-6984.12268
Sabatini, A. M. (2011). Estimating three-dimensional orientation of human body parts by inertial/magnetic sensing. Sensors 11, 1489–1525. doi: 10.3390/s110201489
Schuldt, C., Barbara, L., and Stockholm, S.-. (2004). “Recognizing Human Actions: A Local SVM Approach * Dept. of Numerical Analysis and omputer Science,” in ICPR 2004. Proceedings of the 17th International Conference on Pattern Recognition, 2004 (Cambridge, UK), 32–36.
Shkel, A. M. (2011). “Precision navigation and timing enabled by microtechnology: are we there yet?” in IEEE Sensors 2010 Conference (Kona, HI).
Smith-Atakan, S. (2006). Human-Computer Interaction. Thomson. Available online at: https://books.google.at/books?hl=deandlr=andid=tjPHVhncBzYCandoi=fndandpg=PR9anddq=human+computer+interactionandots=mr7DY7LhEnandsig=Xf5Q_hbo_xyx8CCXOBw5Fq2c_G8#v=onepageandq=human computer interactionandf = false
Stiefmeier, T., Roggen, D., and Tröster, G. (2007). “Gestures are strings: efficient online gesture spotting and classification using string matching,” in Proceedings of 2nd International Conference on Body Area Networks (Florence), 1–8.
Szabo, G., and Dittelman, J. (2014). Using mobile technology with individuals with aphasia: native iPad features and everyday apps. Semin. Speech Lang. 35, 5–16. doi: 10.1055/s-0033-1362993
Thill, S., and Twomey, K. E. (2016). What's on the inside counts: a grounded account of concept acquisition and development. Front. Psychol. 7:402. doi: 10.3389/fpsyg.2016.00402
Tomasello, R., Garagnani, M., Wennekers, T., and Pulvermüller, F. (2017). Brain connections of words, perceptions and actions: a neurobiological model of spatio-temporal semantic activation in the human cortex. Neuropsychologia 98, 111–129. doi: 10.1016/j.neuropsychologia.2016.07.004
Von Essen, J. D., and Nilsson, L. G. (2003). Memory effects of motor activation in subject-performed tasks and sign language. Psychon. Bull. Rev. 10, 445–449. doi: 10.3758/BF03196504
Weinland, D., Ronfard, R., and Boyer, E. (2011). A survey of vision-based methods for action representation, segmentation and recognition. Comp. Vis. Image Understand. 115, 224–241. doi: 10.1016/j.cviu.2010.10.002
Keywords: tutor systems, language instruction, aphasia therapy, intelligent tutor system, gesture production, gesture recognition, learning
Citation: Macedonia M, Hammer F and Weichselbaum O (2018) Guided Embodiment and Potential Applications of Tutor Systems in Language Instruction and Rehabilitation. Front. Psychol. 9:927. doi: 10.3389/fpsyg.2018.00927
Received: 09 November 2017; Accepted: 22 May 2018;
Published: 13 June 2018.
Edited by:
Amon Rapp, Università degli Studi di Torino, ItalyReviewed by:
J. Scott Jordan, Illinois State University, United StatesCopyright © 2018 Macedonia, Hammer and Weichselbaum. This is an open-access article distributed under the terms of the Creative Commons Attribution License (CC BY). The use, distribution or reproduction in other forums is permitted, provided the original author(s) and the copyright owner are credited and that the original publication in this journal is cited, in accordance with accepted academic practice. No use, distribution or reproduction is permitted which does not comply with these terms.
*Correspondence: Manuela Macedonia, bWFudWVsYUBtYWNlZG9uaWEuYXQ=
Disclaimer: All claims expressed in this article are solely those of the authors and do not necessarily represent those of their affiliated organizations, or those of the publisher, the editors and the reviewers. Any product that may be evaluated in this article or claim that may be made by its manufacturer is not guaranteed or endorsed by the publisher.
Research integrity at Frontiers
Learn more about the work of our research integrity team to safeguard the quality of each article we publish.