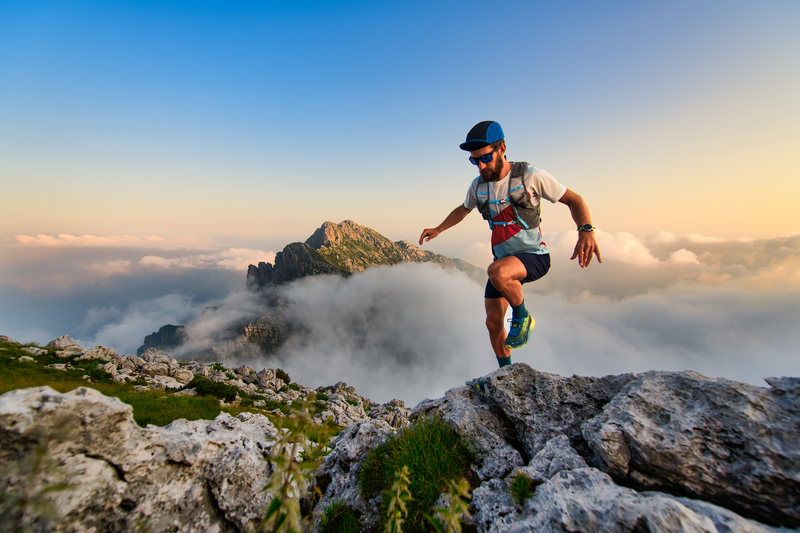
95% of researchers rate our articles as excellent or good
Learn more about the work of our research integrity team to safeguard the quality of each article we publish.
Find out more
GENERAL COMMENTARY article
Front. Psychol. , 27 September 2017
Sec. Educational Psychology
Volume 8 - 2017 | https://doi.org/10.3389/fpsyg.2017.01715
This article is part of the Research Topic Epistemological and Ethical Aspects of Research in the Social Sciences View all 13 articles
A commentary on
Psychological Science's Aversion to the Null
by Heene, M., and Ferguson, C. J. (2017). Psychological Science under Scrutiny: Recent Challenges and Proposed Solutions, eds S. O. Lilienfeld and I. D. Waldman (Chichester: John Wiley & Sons), 34–52.
Heene and Ferguson (2017) contributed important epistemological, ethical and didactical ideas to the debate on null hypothesis significance testing, chief among them ideas about falsificationism, statistical power, dubious statistical practices, and publication bias. Important as those contributions are, the authors do not fully resolve four confusions which we would like to clarify.
One confusion is equating the null hypothesis (H0) with randomness when “chance” actually resides in the sample. We can, indeed, read three different instances of randomness in the text: associated with the sample on pages 36 (trial performance) and 37; associated with the alternative hypothesis (HA) on page 41 (“less likely to observe mean differences…far off the true…mean difference of 0.7”); and associated with H0 throughout the text, starting on page 36. In reality, H0 simply claims a population non-effect (H0: Δ = 0) while HA claims a constant effect (e.g., HA: Δ = 0.7), their corresponding distributions assuming random sampling variation in both cases. It is in the (random) sample where “chance” resides, as by chance we may pick a sample which shows a given effect (e.g., δ = 0.3) when the true effect in the population is either “0” (H0) or “0.7” (HA). Frequentist tests only assess the probability of getting the observed sample effect under H0 while Bayesian statistics also assesses the probability of such effect under HA (e.g., Rouder et al., 2009). Therefore, the p-value does not inform about a hypothesis of chance but about the probability of the data under H0 (Fisher, 1954).
A second issue confuses power with missing true effects, something explicitly expressed on page 42 but also suggested when discussing sample sizes throughout the text (p. 36 onwards). The underlying argument is that larger sample sizes allow for achieving statistical significance so that a true effect may not be missed—something which is, at the same time, portrayed as unethical, e.g., p. 36, and ludicrous, e.g., p. 44. In reality, “we cannot manipulate population effect sizes” (p. 41), as they are deemed constant in the population (e.g., HA: Δ = 0.7), and a significant result at 50% power will not be missed at 80% power. As Heene and Ferguson's Figures 3.1A,C show, power simply moves the goalposts on the real line, reducing the Type II error (β), while the larger sample size also reduces the standard error. By moving the goalposts, smaller (by chance) sample effects get associated with HA, which is a correct association as long as there is a true population effect. Thus, power is there not to prevent missing effects due to small sample sizes but to be able to justify whether we could plausibly accept H0 when results are not significant (Neyman, 1955; Cohen, 1988).
A third issue is about falsificationism (pp. 35–37), which the authors argue cannot happen in psychology because we never accept H0, only reject it or fail to reject it. In reality, frequentist tests are logically based on modus tollens, the valid argument form for the falsification of statements (Perezgonzalez, 2017a). H0 is simply the contrapositive of our research hypothesis, and denying H0 allows us to affirm the latter. Therefore, frequentist tests are eminently falsificationist, attempting to disprove H0 via reductio arguments (p, α; Mayo, 2017). Indeed, H0 does not even need to be “zero” in the population: We could perfectly substitute the actual value of our HA, so that we may prove the theory false with a significant result (the “strong” test purported by Meehl, 1997).
A fourth issue is whether we always need to be in the position of accepting H0 (something argued on pages 36–37). This is not necessarily so. Just testing H0 as for rejecting it is suitable when we are only interested in learning about our research hypothesis (e.g., does the treatment have an effect?—Perezgonzalez, 2016). In such context, H0 provides a precise statistical hypothesis for carrying out the test and, because the actual parameter (Δ) is unknown, it only provides informative value via its rejection (Fisher, 1954), H0 acting merely as a “straw man” (Cortina and Dunlap, 1997). This testing procedure was not only developed in the context of small samples (Fisher, 1954) but the lack of a specific HA precludes the control of Type II errors and of power. (A way forward would be to assess the effects warranted under H0—Mayo and Spanos, 2006—or to control sample size via a sensitiveness analysis—Perezgonzalez, 2017b).
If we wish to be able to accept H0, then we are stating that we are also interested in the potential demise of our intervention (i.e., if the treatment has no effect, we want to make sure it is akin to placebo; Perezgonzalez, 2016). This testing seems similar to Fisher's, but it requires active control of the severity with which the alternative hypothesis is to be tested (ideally, ≥80% power; Neyman, 1955; Cohen, 1988). Such control necessarily means more information—a precise alternative hypothesis (e.g., HA: μ1 – μ2 = 0.7, vs. H0: μ1 – μ2 = 0) and a specified Type II error for HA (e.g., β = 0.20)—so that the power of the test can be managed (given α, β, and N). This approach not only allows for accepting H0 but also illustrates that power is only relevant for such purpose, not for rejecting H0. Such approach, and similar ones, have also been available since Fisher's tests of significance (e.g., Neyman and Pearson, 1928; Jeffreys, 1939).
As final note, frequentist approaches only deal with the probability of data under H0 [p(D|H0)]. If we want to say anything about the (posterior) probability of the hypotheses, then a Bayesian approach is needed in order to confirm which hypothesis is most likely given both the likelihood of the data and the prior probabilities of the hypotheses themselves (Jeffreys, 1961; Gelman et al., 2013).
JDP initiated and drafted the general commentary. DF and JP contributed theoretical background and feedback. All authors approved the final version of the manuscript for submission.
The authors declare that the research was conducted in the absence of any commercial or financial relationships that could be construed as a potential conflict of interest.
Cohen, J. (1988). Statistical Power Analysis for the Behavioral Sciences, 2nd Edn. New York, NY: Psychology Press.
Cortina, J. M., and Dunlap, W. P. (1997). On the logic and purpose of significance testing. Psychol. Methods 2, 161–172. doi: 10.1037/1082-989X.2.2.161
Fisher, R. A. (1954). Statistical Methods for Research Workers, 12th Edn. Edinburgh: Oliver and Boyd.
Gelman, A., Carlin, J. B., Stern, H. S., Dunson, D. B., Vehtari, A., and Rubin, D. B. (2013). Bayesian Data Analysis, 3rd Edn. Boca Raton, FL: CRC Press.
Heene, M., and Ferguson, C. J. (2017). “Psychological science's aversion to the null, and why many of the things you think are true, aren't,” in Psychological Science under Scrutiny: Recent Challenges and Proposed Solutions, eds S. O. Lilienfeld and I. D. Waldman (Chichester: John Wiley & Sons), 34–52.
Mayo, D. G. (2017). If you're Seeing Limb-Sawing in p-Value Logic, You're Sawing Off the Limbs of Reductio Arguments [Web log post]. Available online at: https://errorstatistics.com/2017/04/15/if-youre-seeing-limb-sawing-in-p-value-logic-youre-sawing-off-the-limbs-of-reductio-arguments/.
Mayo, D. G., and Spanos, A. (2006). Severe testing as a basic concept in a Neyman-Pearson philosophy of induction. Br. J. Philos. Sci. 57, 323–357. doi: 10.1093/bjps/axl003
Meehl, P.E. (1997). “The problem is epistemology, not statistics: replace significance tests by confidence intervals and quantify accuracy of risky numerical predictions,” in What If There Were No Significance Tests? eds L. L. Harlow, S. A. Mulaik, and J. H. Steiger (Mahwah: Erlbaum), 393–425.
Neyman, J. (1955). The problem of inductive inference. Commun. Pure Appl. Math. 8, 13–45. doi: 10.1002/cpa.3160080103
Neyman, J., and Pearson, E. S. (1928). On the use and interpretation of certain test criteria for purposes of statistical inference: part I. Biometrika 20A, 175–240. doi: 10.2307/2331945
Perezgonzalez, J. D. (2016). Commentary: how Bayes factors change scientific practice. Front. Psychol. 7:1504. doi: 10.3389/fpsyg.2016.01504
Perezgonzalez, J. D. (2017a). Commentary: the need for Bayesian hypothesis testing in psychological science. Front. Psychol. 8:1434. doi: 10.3389/fpsyg.2017.01434
Perezgonzalez, J. D. (2017b). Statistical Sensitiveness for the Behavioral Sciences. Available online at: https://osf.io/preprints/psyarxiv/qd3gu.
Keywords: data testing, hypothesis testing, null hypothesis significance testing, effect size, falsificationism, statistics
Citation: Perezgonzalez JD, Frías-Navarro D and Pascual-Llobell J (2017) Commentary: Psychological Science's Aversion to the Null. Front. Psychol. 8:1715. doi: 10.3389/fpsyg.2017.01715
Received: 30 May 2017; Accepted: 19 September 2017;
Published: 27 September 2017.
Edited by:
Hannes Schröter, German Institute for Adult Education (LG), GermanyReviewed by:
Daniel Bratzke, Universität Tübingen, GermanyCopyright © 2017 Perezgonzalez, Frías-Navarro and Pascual-Llobell. This is an open-access article distributed under the terms of the Creative Commons Attribution License (CC BY). The use, distribution or reproduction in other forums is permitted, provided the original author(s) or licensor are credited and that the original publication in this journal is cited, in accordance with accepted academic practice. No use, distribution or reproduction is permitted which does not comply with these terms.
*Correspondence: Jose D. Perezgonzalez, ai5kLnBlcmV6Z29uemFsZXpAbWFzc2V5LmFjLm56
Disclaimer: All claims expressed in this article are solely those of the authors and do not necessarily represent those of their affiliated organizations, or those of the publisher, the editors and the reviewers. Any product that may be evaluated in this article or claim that may be made by its manufacturer is not guaranteed or endorsed by the publisher.
Research integrity at Frontiers
Learn more about the work of our research integrity team to safeguard the quality of each article we publish.