- 1Department of Speech, Language, and Hearing Sciences, University of Florida, Gainesville, FL, USA
- 2Department of Neurodegenerative Disease, Dementia Research Centre, Institute of Neurology, University College London, London, UK
- 3Eleanor Saffran Center for Cognitive Neuroscience, Temple University, Philadelphia, PA, USA
- 4Department of Communication Sciences and Disorders, Temple University, Philadelphia, PA, USA
The empirical study of language has historically relied heavily upon concrete word stimuli. By definition, concrete words evoke salient perceptual associations that fit well within feature-based, sensorimotor models of word meaning. In contrast, many theorists argue that abstract words are “disembodied” in that their meaning is mediated through language. We investigated word meaning as distributed in multidimensional space using hierarchical cluster analysis. Participants (N = 365) rated target words (n = 400 English nouns) across 12 cognitive dimensions (e.g., polarity, ease of teaching, emotional valence). Factor reduction revealed three latent factors, corresponding roughly to perceptual salience, affective association, and magnitude. We plotted the original 400 words for the three latent factors. Abstract and concrete words showed overlap in their topography but also differentiated themselves in semantic space. This topographic approach to word meaning offers a unique perspective to word concreteness.
Introduction
A narrow empirical focus on concrete words yields an incomplete picture of the mental lexicon. Today, substantial gaps persist in our knowledge of the cognitive and neural underpinnings of abstract words (e.g., love, truth). Readers of English encounter abstract and concrete words with comparable frequency (Reilly, 2005; Reilly and Kean, 2007). Thus, it is difficult to justify sidestepping the abstract half of the lexicon that poses an empirical challenge.
Despite lopsided attention to concrete words, cognitive science has shown longstanding interest in abstract words (Locke, 1685). Empirical work in abstract-concrete word differences advanced rapidly in the late 1960s when psycholinguists defined concreteness and devised a means of measuring its strength. Concreteness, the extent to which a word can be perceived through the senses, is typically measured as a continuous, ratio level variable anchored by a zero point, with zero indicating no evoked perception (Paivio et al., 1968). Psycholinguists have compiled concreteness ratings for many thousands of words across numerous languages with the aim of elucidating the word concreteness effect, a term that reflects the collective advantage for concrete words in a variety of domains, including recall accuracy (Walker and Hulme, 1999), age of acquisition (Gilhooly and Logie, 1980), word list memory (Allen and Hulme, 2006), naming latency (Bleasdale, 1987), word recognition (Schwanenflugel et al., 1988), and dissociations in performance associated with neurological injury (Warrington, 1975, 1981; Breedin et al., 1994; Franklin et al., 1995; Bonner et al., 2009; Jefferies et al., 2009).
It has proven exceptionally difficult to develop a comprehensive theory accounting for the word concreteness effect (Connell and Lynott, 2012). Abstract and concrete words differ on a variety of non-semantic dimensions, including sound structure and morphological complexity (Reilly and Kean, 2007; Westbury and Moroschan, 2009; Reilly et al., 2012), polysemy and homonymy (Anderson and Nagy, 1991; Crutch and Jackson, 2011). Thus, when one observes a concreteness advantage in a particular task, it is not always clear where the locus of the effect lies (for an example see Kroll and Merves, 1986).
An intimate link between language and abstract word representation forms the backbone of today's dominant model of word concreteness. Paivio's (1991) Dual Coding Theory (DCT) offers a multiple semantics approach to word meaning based on the premise that verbal knowledge and visuoperceptual knowledge reflect two parallel but also highly interactive codes that support a word's meaning. Concrete words benefit from the support of both visual and verbal codes (i.e., they are dually coded), whereas abstract word meaning is mediated almost exclusively through a verbal code. DCT has proven its durability as a model that accounts for word concreteness effects in early childhood language learning and reading, as well as in neurological dissociations in adults (Franklin et al., 1994, 1995; Sadoski and Paivio, 2004; Sadoski, 2005).
Although DCT is compelling in scope, many psycholinguists now recognize the need for finer-grained specificity in delineating the topography of abstract and concrete words. Several approaches to concrete-abstract word representation have recently emerged to address this need. Gallese and Lakoff (2005) and Kousta et al. (2011) have proposed “embodied” approaches to abstract word representation that anchor abstract word meaning in somatic states such as emotion. These embodied approaches offer a radical departure from the dominant view that abstract words are mediated exclusively through symbolic, propositional knowledge. In one such approach, Kousta et al. (2011) argue that emotion is a powerful latent factor (with somatic and perceptual underpinnings) that underlies the meaning of abstract words (Andrews et al., 2009; Kousta et al., 2009, 2011; Newcombe et al., 2012). Kousta et al. further argued that many past studies of concreteness have confounded the constructs of imageability (i.e., the ability to evoke a mental image) and context availability and that when such confounding factors are tightly controlled, the concreteness advantage either disappears or modestly reverses such that abstract words show a processing advantage (but see Paivio, 2013).
Other theorists attribute abstract-concrete differences to the rapid access to contextual information for concrete words (i.e., context availability) (Schwanenflugel and Shoben, 1983), a greater number of semantic units to support concrete concepts (Plaut and Shallice, 1993) or greater number of semantic predicates for concrete items (Jones, 1985). An alternative formulation has suggested that abstract words have a relatively greater reliance upon associative information, whilst concrete words have a relatively greater reliance upon semantic similarity information (Crutch and Warrington, 2005). The predictions of this “different representational frameworks” hypothesis have been confirmed by a number of recent studies (Duñabeitia et al, 2009), with semantic similarity and association demonstrated to exert a graded effect across the concreteness spectrum (Crutch and Jackson, 2011).
Language researchers have long recognized the role of taxonomic hierarchies in concrete word representation (Rosch, 1973; Lakoff, 1990). For example, dog is a basic level concept that has both superordinate (e.g., animal) and subordinate distinctions (e.g., collie). Much of our knowledge of lexical category structure is derived from studies where participants generate lists of features (e.g., dog → tail) or associations (e.g., dog → leash) for concrete target words (Garrard et al., 2001, 2005; Cree and McRae, 2003; Rogers et al., 2004; Cree et al., 2006; Dilkina and Lambon Ralph, 2012). These feature listings yield distance metrics that speak to the family resemblance among concrete words. While these feature listing methods have some utility when applied to abstract words there are inherent weaknesses to this approach for abstract words. Abstract concepts, by their nature, lack the taxonomic hierarchical organization and unambiguous contextual properties imbued within concrete concepts and which make a feature listing method ideal (But see Barsalou and Wiemer-Hastings, 2005; Wiemer-Hastings and Xu, 2005 for examples of feature listing approaches for abstract concepts).
Recently a novel abstract concept feature (ACF) rating approach has been used in combination with multi-dimensional scaling techniques to examine distance metrics and cohesion among abstract words. This approach, developed by Crutch et al. (2012a,b), asks participants to rate the importance of particular types of information for the meaning of a concept. Crutch et al. originally performed this procedure on a corpus of 50 abstract words, spanning nine cognitive dimensions, including emotion, magnitude, and spatial relations. Unlike standard measures of word concreteness, this unique clustering solution revealed that concepts such as VAPOR and ILLUSION aggregate closely within semantic space. Standard semantic distance metrics gleaned through feature listing approaches or unidimensional ratings often fail to capture such similarities.
Here we performed the ACF in order to determine the clustering attributes of larger corpus of concrete and abstract concepts within a higher dimensional space than was originally employed by Crutch et al. (2012a,b). We measured each word's salience on 12 unique dimensions, including: Sensation, Action; Thought; Emotion; Social Interaction; Time; Space; Quantity; Polarity; Morality; Ease of Modifying; and Ease of Teaching.
Sensorimotor information has long been known to play an important role in the representation of concrete concepts, and a growing body of research has made the argument for the role of affective association in the representation of abstract concepts (Andrews et al., 2009; Kousta et al., 2009, 2011). We included metrics for Sensation, Action, Emotion, and Polarity based on the dominance of these variables in previous work. We also included a more nuanced set of dimensions linked to Social Interaction and Thought. Our rationale for the inclusion of these dimensions stems from the work of Borghi et al. (2011) and Barsalou (1999), who argue for the contributions of social interaction and introspection on abstract word acquisition and representation. We assessed the salience of Time in abstract and concrete word meaning due to its role in the temporal unfolding of event structure (Allman and Meck, 2012). We assessed the salience of Spatial information due to its roles both in the organization of geographical concepts, as well as more oblique contributions to metaphor (Zwaan and Yaxley, 2003; Lakoff and Johnson, 2008) We assessed Quantity with the aim of tapping the division between numerical and non-numerical semantics (e.g., mass-count distinctions) (Gathercole, 1985). The Morality dimension characterizes the social mores that govern behavior which have been hypothesized to reflect a cognitive emotional association complex which can be represented across the prefrontal cortex and limbic system (Moll et al., 2005). Ease of teaching reflects variety in both age of acquisition and learning style (e.g., experiential observation vs. explicit verbal instruction) that mark abstract and concrete words (Coltheart et al., 1988; Strain et al., 2002; Reilly et al., 2007). Ease of Modifying provides an index of the contextual availability of a word in terms of adjectival description (Schwanenflugel and Shoben, 1983; Schwanenflugel et al., 1992). It should be noted that this is not an exhaustive list of dimensions and that the inclusion of certain dimensions is more empirically/theoretically justified than others. It should also be noted that we were constrained by selecting dimensions that could be easily distinguished and comprehended by the lay participant.
Hypotheses, Aims, and Significance
The DCT is premised upon the interaction of two parallel semantic memory systems, one dedicated to sensory imagery and the other dedicated to language. We hypothesize that word concreteness might ultimately be better contextualized within one semantic system. One might specify such a system in terms of a high dimensional space where word meanings cluster along axes representing key cognitive dimensions (e.g., emotional salience, sensory salience). We hypothesize that this unitary space comprises a topography wherein the meanings of words (both concrete and abstract) are distributed. Here, we investigated the clustering behaviors of a relatively large (N = 400) set of abstract and concrete nouns within a semantic space bounded 12 dimensions, including: Sensation; Action; Thought; Emotional Valence; Social Interaction; Time; Space; Quantity; Polarity; Morality; Ease of Modifying; and Ease of Teaching.
We hypothesize that this topographic approach would produce regions of overlap, as well as distinct clusters corresponding to “concreteness” (e.g., abstract words cluster at the high end of emotional valence). Importantly, the presence of a unitary, multi-dimensional space would obviate the need for an artificial dichotomy such as concreteness by treating this and other psycholinguistic variables as continuous.
Methods
Overview
We isolated a set of abstract (N = 200) and concrete (N = 200) English nouns and obtained Likert-scale ratings for each word on 12 variables (dimensions). We then employed factor reduction and hierarchical cluster analysis to model the topography of how these words scaled.
Participants
Participants included native English speakers recruited through the online crowd-sourcing program, Mechanical Turk. Following trimming procedures aimed at eliminating spurious participants, we isolated a sample (N = 365) with an age ranging from 17 to 83 years, (mean = 40.7). Education ranged from 9 to 20 years (mean = 15.4). Sex distribution was 68.2% female.
Materials and Procedure
Stimuli included English nouns (N = 400) from the MRC Psycholinguistic Database (Coltheart, 1981). Stimuli were pure nouns in that we ensured they had no alternate grammatical class (e.g., desk but not phone). Target words were either abstract or concrete based on rated concreteness. The MRC database concreteness values reflect a 100–700 scale. In our sample, concrete words had an average concreteness rating of 589 (SD = 46.9), whereas abstract words had a rated average of 304 (SD = 47.1). There was no overlap in the distributions of abstract and concrete words, and their means were distant (Zdifference = 2.38). The list of dimensions chosen for the analysis was not an exhaustive set of dimensions. In order to provide proof of concept that this clustering procedure could prove successful, we sampled words from the tails of the concreteness spectrum (high/low).
Scale Development and Implementation
Participants rated each of the target words on the following 12 dimensions using a 7-point Likert Scale: 1. Sensation; 2. Action; 3. Thought; 4. Emotional Valence; 5. Social Interaction; 6. Time; 7. Space; 8. Quantity; 9. Polarity; 10. Morality; 11. Ease of Modifying; 12. Ease of Teaching. Table 1 reflects the wording given to participants.
Each stimulus appeared in randomized order within the context of separate surveys dedicated to each cognitive dimension. Participants were instructed to use the entire scale and to work quickly but carefully.
Data Collection
Participants completed ratings via Amazon Mechanical Turk, an online pool of workers from around the globe who perform virtual tasks (Buhrmester et al., 2011). Participants logged into Mechanical Turk, electronically consented, and then completed up to 12 individual surveys, one for each dimension.
Data Analyses
We excluded participant data that corresponded to any of the following conditions: (1) Taking less than 10 min to complete the survey (less than 1.5 s per response), (2) Using less than half of the seven point scale (i.e., 3 numbers or less) which was considered not following our directions of using the entire scale, or (3) The presence of runs of more than 20 identical consecutive responses (2.5 SD away from the average run mean; M = 3.2, SD = 6.8). We then performed intraclass correlational analyses in order to measure inter-rater reliability. We also ran correlation analyses between individual item standard deviations and concreteness in order to determine if concreteness led to greater variability in the rating of items.
We first pursued exploratory factor analysis with the goal of reducing the dimensionality and redundancy of the original set of 12 variables. We converted the original ratings into a series of factor scores using the Anderson-Rubin method (Anderson and Rubin, 1956). The factor analyses yielded three latent factors that subsequently define a three-dimensional space upon which distance metrics between any two words can be derived. We report the Euclidean squared coefficient as a metric of semantic distance (Danielsson, 1980).
Using the reduced dataset, we then conducted a hierarchical agglomerative cluster analysis using Ward's method (1963). This procedure iteratively clusters observations into groups in a bottom-up manner until only one large cluster remains. We determined the optimal clustering solution by comparing clusters from the hierarchical cluster analysis with clusters created by a partitional k-means iterative analysis using Cohen's Kappa (Aldenderfer and Blashfield, 1984). The cluster analysis allowed us to create an empirical metric of how items grouped in the semantic. In other words this allowed us to determine how items grouped on a smaller dimensions as compared to macro dimensions (i.e., Abstract-Concrete).
Results
Data Trimming
The first author and a blinded rater showed 99.3% inter-rater agreement on surveys to be excluded (see method for criteria). Of the original 545 surveys, 180 (33%) were eliminated, leaving 365 surveys for final analysis (See Supplementary Material for how many responses were removed per condition). Removal was comparable across all surveys. The intraclass correlation coefficient (ICC) was found to high throughout all 12 surveys with the lowest ICC being 0.991 (see Table 2). Table 3 displays the correlations between item standard deviations and concreteness for each survey dimension. Two of the dimensions showed greater variability for more concrete concepts, three showed no variability differences and seven showed greater variability for more abstract items.
Individual Ratings Emotion
Figure 1 reflects scatterplots of ratings for each of the 12 original dimensions plotted against the a priori concreteness values for each target word. All of the bivariate correlations were significant (α ≤ 0.01).
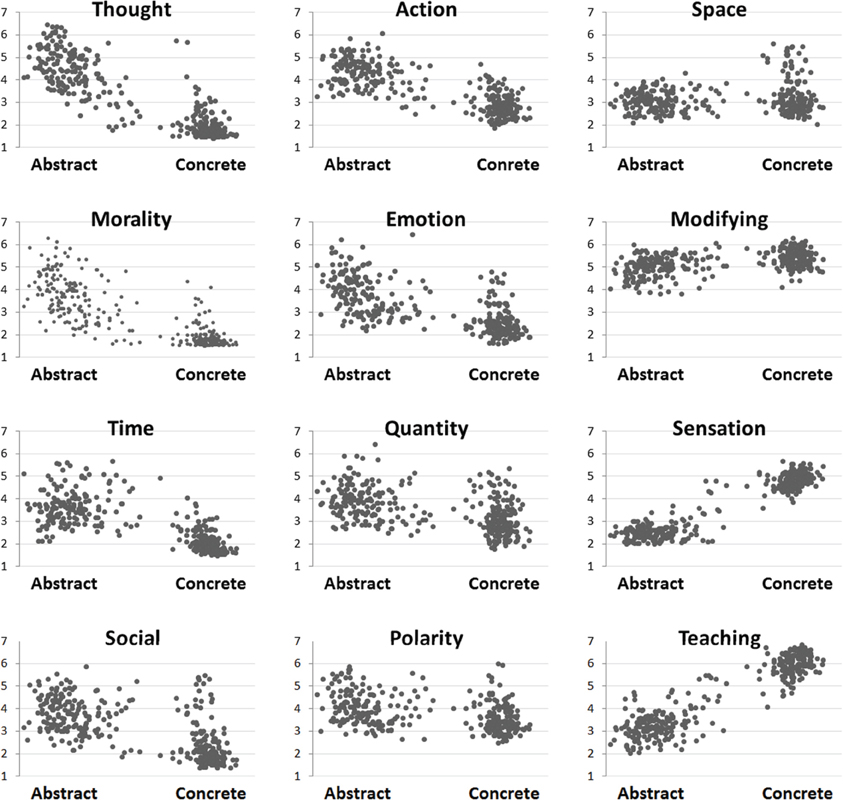
Figure 1. Scatterplots of mean Likert scale ratings (1–7; y-axis) for each of the 12 rating dimensions for words from across the concreteness spectrum (x-axis).
Exploratory Factor Analysis
We extracted three latent factors (model fit, R2 = 0.81) from the original set of 12 dimensions (see Table 1). The reduced set of factors and the constituent variables they subsume were as follows: (1) Emotion, Polarity, Social, Morality, Action, Thought; (2) Ease of Teaching, Sensation, Ease of Modifying, Time; (3) Space, Quantity (see Table 4). In terms of nomenclature, we will refer to these latent constructs hereafter as: (1) Affective Association/Social Cognition; (2) Perceptual Salience; and (3) Magnitude.
Table 5 represents relations between the three factors with other salient psycholinguistic variables (e.g., word frequency, age of acquisition). Figure 2 displays the spread between concrete and abstract words within the 3-dimensional space defined by the three factors.
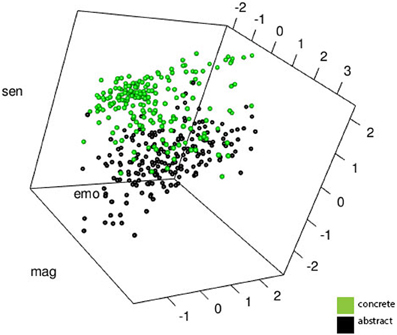
Figure 2. Three Dimensional Scatterplot Representing Abstract and Concrete Word Meaning. This view represents rotation about the axes/planes defined by the factors: Sens, sensation; Mag, magnitude; and Emo, emotion.
Hierarchical Cluster Analysis
A 12-cluster solution yielded an optimal model (Cohen's Kappa = 0.87). Figure 3 reflects a dendrogram corresponding to this optimal clustering solution. Table 6 reflects quantitative aspects of each cluster in terms of psycholinguistic attributes (e.g., lexical frequency).
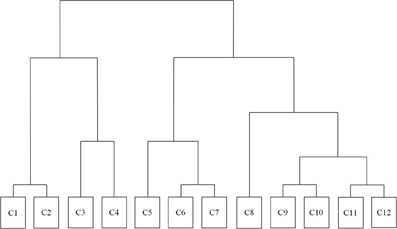
Figure 3. Dendrogram of hierarchical cluster analysis. Each cluster has been given a cluster number (e.g., C1,C2). The words inside each cluster can be found in Supplementary Material.
The dendrogram shows that most concrete words are contained in the first four clusters (C1–C4), whereas abstract words are mostly found in latter clusters (C5–C12). Focusing on the clusters of abstract words, it is apparent that the level of affective association increases from left to right on the dendrogram. Cluster 8 is also of interest as it is a cluster of concrete words (e.g., chocolate, father) that are high in affective salience and nestled within many other abstract words describing social cognition.
Discussion
Using hierarchical cluster analyses, we explored the topography of abstract and concrete nouns (N = 400). We first defined a multi-dimensional semantic space that was composed of 12 individual predictors, each with precedence as a moderator of concreteness effects. Participants subsequently rated the original set of abstract and concrete nouns on all of the individual dimensions. We then used factor analysis to examine whether the original multi-dimensional semantic space could be reduced. This approach yielded three latent constructs, corresponding roughly to affective association/social cognition, perceptual salience, and magnitude. We then calculated distance metrics for the abstract and concrete words within the semantic space defined by this reduced set of predictors. Abstract and concrete words have both unique and common regions of overlap within semantic space. Moreover, factors such as affective association/social cognition and magnitude appear to play significant roles in delineating this space.
There are two primary ways of visualizing these data. The first is at the level of the individual predictors, and the second is through a clustering analysis that considers the predictors together.
Individual Predictors
Figure 1 highlights the variability and weighting across the 12 unique dimensions in isolation prior to factor reduction. The bivariate correlations between concreteness and each predictor vary from strongly positive (e.g., r = 0.94 for sensation) to strongly negative (e.g., r = −0.87 for thought). In addition, several predictors (e.g., r = 0.10 for space) had relatively flat slopes, indicating that these variables only weakly discriminated concrete from abstract words in isolation. With respect to concreteness, we observed the strongest positive bivariate correlations with sensation (r = 0.94) and ease-of-teaching (r = 0.92). Sensation, analogous to imageability, is a construct intimately related to concreteness (R2 = 0.88) but one that captures a wider range of somatosensory states. Ease-of-teaching has a close parallel to ease of learning. A vast body of literature investigating age-of-acquisition has shown that the earliest acquired words tend be concrete (e.g., ball, mama). One common developmental explanation is that the salience of a concrete word's referent facilitates a fast and durable mapping (Gilhooly and Logie, 1980; Bloom, 1998). Abstract words, in contrast, have no physical referent and must therefore be learned through alternate means, often through nuanced experiences with concrete objects and emotions. For example, one must first learn “sad” before acquiring a more abstract state such as “melancholy.”
In addition to strong positive relationships with concreteness, we also observed several robust negative correlations, including thought (r = −0.87) and morality (r = −0.81). Participants rated thought according to the salience of ideas, opinions, judgments, and mental operations. Many words that are considered classically abstract are often defined as “the feeling of X.” Thus, the strong negative correlation between concreteness and thought reflects a logical property of abstract words (i.e., they tend to often denote unobservable mental states). Morality is similar to thought in that this construct often denotes phenomena that are not directly observable but instead reflect complex social mores that govern and denote behavior (e.g., truth, honesty).
Multidimensional Solution
The strength of this approach lies not within individual predictors but in a solution that considers all such variables simultaneously. This multi-dimensional solution yielded a dynamic structure whereby abstract and concrete words can be differentiated. We view two properties of the observed topography as particularly salient: (A) Abstract and concrete words have unique topographies within a multi-dimensional space defined by affective association/social cognition, magnitude, and perceptual salience; (B) The topography of abstract and concrete words also overlap within this space. For example, father and love load high on emotion and ultimately cluster together despite the fact that father is classically considered concrete and “love” as abstract. It should be noted that this clustering emerges despite all words being rate independently (i.e., there were no ratings of the direct association between any pairs of concepts).
The topographies of abstract and concrete words are unique
While affective association/social cognition and concreteness/perceptual salience have been regularly indicated as dimensions that underlie the representations of concrete and abstract concepts, the role of magnitude is less clear.
The factor analysis identified a latent variable reflecting a combination of space and quantity. We interpreted this amalgamation as corresponding roughly to the construct of magnitude. Magnitude in this context reflects both the scalar features of concrete words (e.g., how large?, how hot?, how loud?) but also gradations of many abstract emotions (e.g., irritated < angry < infuriated). Walsh (2003) has argued that such a magnitude system detects and appreciate such gradations. Neurological damage to regions of the parietal lobes (e.g., cortical basal degeneration) results in deficits for estimating and appreciating many magnitude distinctions, including time, physical size, and affect (i.e., emotional blunting; Gibb et al., 1989; Crutch et al., 2012a,b).
Magnitude is a construct that has previously received attention in the psycholinguistic literature, particularly with respect to spatial metaphor comprehension (Lakoff, 1990, 2012; Barsalou and Wiemer-Hastings, 2005; Jefferies et al., 2009; Connell and Lynott, 2012). During semantic relatedness tasks (e.g., match two related pictures from a field of three), both healthy adults and patients with neurological disorders (e.g., stroke aphasia) tend to take longer to match items that are more geographically distant (e.g., London:New York vs. London:Manchester; Crutch and Warrington, 2003), or items that appear in reverse-iconic order (e.g., basement:attic vs. attic:basement; Zwaan and Yaxley, 2003). Similar findings have been reported for the directionality and congruency of spatial metaphors with respect to one's own body (Zwaan and Taylor, 2006). Thus, our scaling results confirm a place of prominence and a dimension of discrimination for magnitude and related variables (e.g., polarity, valence) in supporting the meanings of both abstract and concrete words.
The topographies of abstract and concrete words also overlap
The scatterplot in Figure 2 demonstrates several regions of significant overlap in the topographies of abstract and concrete words. The area of highest overlap was apparent for words at the high end of the affective association/social cognition dimension. Concrete words that loaded high on the affective association/social cognition factor (e.g., father, chocolate) were closer via distance metrics in semantic space to abstract words (e.g., love, justice) than they were to other concrete or abstract words lacking an affective association/social cognition component (e.g., aspect, paradigm, fisherman, and banana). This underscores the importance of emotional valence in word meaning. Altarriba et al. (1999) have argued that emotional valence can be viewed as orthogonal to concreteness and should accordingly be viewed as an independent dimension of word meaning (i.e., there are abstract, concrete, and emotion words). More recently Kousta et al. (2011) have argued for an embodied theory with emotional information being the main contributor to the representation of abstract concepts (Etkin et al., 2006; Vigliocco et al., 2013).
The overlap of our topographies in areas of high affective association/social cognition suggest that while abstract concepts likely rely more on affective association/social cognition for their representation, concrete concepts can also be greatly influenced by affective association/social cognition. There is also the indication that high affective association/social cognition can lead to abstract concepts becoming more tangible, that is, more concrete, as indicated by the positive association between affective association/social cognition and imageability. This overlap may lead to a strengthening of the networks for these concepts leading to collective processing advantages that Kousta et al. (2009) found for words high in affective association. It should be noted that these areas of overlap are even more surprising as we only chose concepts that were at the extreme ends of the concreteness spectrum.
The ACF approach allowed us to create a single multidimensional semantic space. This approach obviates the need for multiple semantic systems (e.g., language for abstract words, percepts for concrete words). By treating this topography as a continuous space, word meaning can be distributed in a flexible way that is untethered to any particular artificial dichotomy (e.g., abstract-concrete, imageable-non-imageable; for another unitary semantics account see Vigliocco et al., 2004). In this approach words were rated individually, therefore words collocated in this semantic space represent similar underlying properties and not merely linguistic properties. It should be noted that early work on dimensionality in semantics by Osgood et al. Osgood et al. (1954) also found three dimensions that held importance in the evaluation of concepts: evaluation, potency, and activity. This work, however, has mostly focused on determining the connotation of a concept, object, or event.
It still remains an open question, however, whether this semantic space is neurologically real or just a product of our data. We attempted to test this question through the use of a behavioral task with a patient with aphasia (Crutch et al., 2013). The patient, a 65 years old male, had a history of global aphasia which resolved into a mixed non-fluent aphasia. This patient, SKO, displayed deficits in verbal comprehension and phonological-orthographic transcoding. The patient was given a spoken word to written word matching paradigm. This consisted of SKO being shown two words and then being asked to point to the word just spoken by the examiner. The pairs of words were varied by distance. Some of the words were close in distance in the semantic space created in the current study while others were far. As we had predicted, pairs of words closer in semantic distance lead to greater interference than those further. We also determined that ACF ratings were better at predicting deficits than another common and well researched method of determining the strength of word association, latent semantic analysis (Landauer and Dumais, 1997). We argue that these findings suggest that this semantic space is somewhat representative of the underlying representation of concepts.
While the findings here are promising more can be done to improve the current semantic space. The 12 predictors chosen do not constitute an exhaustive list of potentially relevant dimensions. The sensation dimension, for instance, could be broken up into several dimensions (Visual, Auditory, etc.), which might lead to greater differentiation across more concrete concepts. The inclusion of greater dimensionality would also help decrease the amount of unexplained variance in the model, however, this will happen to a smaller and smaller degree as more dimensions are added. Also now that we have shown proof of concept, future work would benefit from expanding the concepts across grammatical class and concreteness (e.g., more middling concreteness concepts) as this will likely create a semantic space which is more ecologically valid.
Overall, this topographic approach also readily lends itself to computational investigations whereby particular dimensions (e.g., magnitude) or individual clusters (e.g., high emotion, low magnitude) might be selectively lesioned as functions of regional brain damage. Much of the utility of this approach will depend on specifying the nature and fluidity of the topography.
Conflict of Interest Statement
The authors declare that the research was conducted in the absence of any commercial or financial relationships that could be construed as a potential conflict of interest.
Acknowledgments
We are grateful to Alison O'Donoughue for her assistance with numerous aspects of this project. This work was supported by US Public Health Service grants DC010197 (Jamie Reilly) and DC013063 (Jamie Reilly), Alzheimer Research UK Senior Research Fellowship (Sebastian Crutch), and by the NIHR Queen Square Dementia Biomedical Research Unit (Sebastian Crutch).
Supplementary Material
The Supplementary Material for this article can be found online at: http://www.frontiersin.org/journal/10.3389/fpsyg.2014.00360/abstract
References
Allen, R., and Hulme, C. (2006). Speech and language processing mechanisms in verbal serial recall. J. Mem. Lang. 55, 64–88. doi: 10.1016/j.jml.2006.02.002
Allman, M. J., and Meck, W. H. (2012). Pathophysiological distortions in time perception and timed performance. Brain 135, 656–677. doi: 10.1093/brain/awr210
Altarriba, J., Bauer, L., and Benvenuto, C. (1999). Concreteness, context availability, and imageability ratings and word associations for abstract, concrete, and emotion words. Behav. Res. Methods 31, 578–602. doi: 10.3758/BF03200738
Anderson, R. C., and Nagy, W. E. (1991). “Word meanings,” in Handbook of Reading Research, Vol. 2, eds R. Barr, M. L. Kamil, P. B. Mosenthal, and P. D. Pearson (Hillsdale, NJ: Lawrence Erlbaum Associates, Inc), 690–724.
Anderson, T. W., and Rubin, H. (1956). “Statistical inference in factor analysis,” in Proceedings of the Berkeley Symposium on Mathematical Statistics and Probability, Vol. 5 (London: Cambridge University Press), 111–150.
Andrews, M., Vigliocco, G., and Vinson, D. (2009). Integrating experiential and distributional data to learn semantic representations. Psychol. Rev. 116, 463–498. doi: 10.1037/a0016261
Barsalou, L. W. (1999). Perceptions of perceptual symbols. Behav. Brain Sci. 22, 637–660. doi: 10.1017/S0140525X99532147
Barsalou, L. W., and Wiemer-Hastings, K. (2005). “Situating abstract concepts,” in Gounding Cognition: The Role of Perception and Action in Memory, Language, and Thought, D. Pecher and R. Zwaan (New York, NY: Cambridge University Press), 129–163. doi: 10.1017/CBO9780511499968.007
Bleasdale, F. A. (1987). Concreteness-dependent associative priming: separate lexical organization for concrete and abstract words. J. Exp. Psychol. Learn. Mem. Cogn. 13, 582–594. doi: 10.1037/0278-7393.13.4.582
Bloom, L. (1998). “Language acquisition in its developmental context,” in Handbook of Child Psychology: Volume 2: Cognition, Perception, and Language ed W. Damon (Hoboken, NJ: John Wiley & Sons Inc), 309–370.
Bonner, M. F., Vesely, L., Price, C., Anderson, C., Richmond, L., Farag, C., et al. (2009). Reversal of the concreteness effect in semantic dementia. Cogn. Neuropsychol. 26, 568–579. doi: 10.1080/02643290903512305
Borghi, A. M., Flumini, A., Cimatti, F., Marocco, D., Scorolli, C., Borghi, A., et al. (2011). Manipulating objects and telling words: a study on concrete and abstract words acquisition. Front. Psychol. 2:15. doi: 10.3389/fpsyg.2011.00015
Breedin, S. D., Saffran, E. M., and Coslett, H. B. (1994). Reversal of the concreteness effect in a patient with semantic dementia. Cogn. Neuropsychol. 11, 617–660. doi: 10.1080/02643299408251987
Buhrmester, M., Kwang, T., and Gosling, S. D. (2011). Amazon's mechanical turk. Perspect. Psychol. Sci. 6, 3–5. doi: 10.1177/1745691610393980
Coltheart, M. (1981). The MRC psycholinguistic database. Q. J. Exp. Psychol. A Hum. Exp. Psychol. 33A, 497–505. doi: 10.1080/14640748108400805
Coltheart, V., Laxon, V. J., and Keating, C. (1988). Effects of word imageability and age of acquisition on children's reading. Br. J. Psychol. 79, 1–12. doi: 10.1111/j.2044-8295.1988.tb02270.x
Connell, L., and Lynott, D. (2012). Strength of perceptual experience predicts word processing performance better than concreteness or imageability. Cognition 125, 452–465. doi: 10.1016/j.cognition.2012.07.010
Cree, G. S., McNorgan, C., and McRae, K. (2006). Distinctive features hold a privileged status in the computation of word meaning: implications for theories of semantic memory. J. Exp. Psychol. Learn. Mem. Cogn. 32, 643–658. doi: 10.1037/0278-7393.32.4.643
Cree, G. S., and McRae, K. (2003). Analyzing the factors underlying the structure and computation of the meaning of chipmunk, cherry, chisel, cheese, and cello (and many other such concrete nouns). J. Exp. Psychol. Gen. 132, 163–201. doi: 10.1037/0096-3445.132.2.163
Crutch, S. J., and Jackson, E. C. (2011). Contrasting graded effects of semantic similarity and association across the concreteness spectrum. Q. J. Exp. Psychol. 64, 1388–1408. doi: 10.1080/17470218.2010.543285
Crutch, S. J., Lehmann, M., Schott, J. M., Rabinovici, G. D., Rossor, M. N., and Fox, N. C. (2012a). Posterior cortical atrophy. Lancet Neurol. 11, 170. doi: 10.1016/S1474-4422(11)70289-7
Crutch, S. J., Troche, J., Reilly, J., and Ridgway, G. R. (2013). Abstract conceptual feature ratings: the role of emotion, magnitude, and other cognitive domains in the organization of abstract conceptual knowledge. Front. Hum. Neurosci. 7:186. doi: 10.3389/fnhum.2013.00186
Crutch, S. J., and Warrington, E. K. (2003). Spatial coding of semantic information: knowledge of country and city names depends on their geographical proximity. Brain 126, 1821–1829. doi: 10.1093/brain/awg187
Crutch, S. J., and Warrington, E. K. (2005). Abstract and concrete concepts have structurally different representational frameworks. Brain 128, 615–627. doi: 10.1093/brain/awh349
Crutch, S. J., Williams, P., Ridgway, G. R., and Borgenicht, L. (2012b). The role of polarity in antonym and synonym conceptual knowledge: evidence from stroke aphasia and multidimensional ratings of abstract words. Neuropsychologia 50, 2636–2644. doi: 10.1016/j.neuropsychologia.2012.07.015
Danielsson, P.-E. (1980). Euclidean distance mapping. Comput. Graph. Image Process. 14, 227–248. doi: 10.1016/0146-664X(80)90054-4
Dilkina, K., and Lambon Ralph, M. A. (2012). Conceptual structure within and between modalities. Front. Hum. Neurosci. 6:333. doi: 10.3389/fnhum.2012.00333
Duñabeitia, J. A., Avilés, A., Afonso, O., Scheepers, C., and Carreiras, M. (2009). Qualitative differences in the representation of abstract versus concrete words: evidence from the visual-world paradigm. Cognition 110, 284–292. doi: 10.1016/j.cognition.2008.11.012
Etkin, A., Egner, T., Peraza, D. M., Kandel, E. R., and Hirsch, J. (2006). Resolving emotional conflict: a role for the rostral anterior cingulate cortex in modulating activity in the amygdala. Neuron 51, 871–882. doi: 10.1016/j.neuron.2006.07.029
Franklin, S., Howard, D., and Patterson, K. (1994). Abstract word meaning deafness. Cogn. Neuropsychol. 11, 1–34. doi: 10.1080/02643299408251964
Franklin, S., Howard, D., and Patterson, K. (1995). Abstract word anomia. Cogn. Neuropsychol. 12, 549–566. doi: 10.1080/02643299508252007
Gallese, V., and Lakoff, G. (2005). The brain's concepts: the role of the sensory-motor system in conceptual knowledge. Cogn. Neuropsychol. 22, 455–479. doi: 10.1080/02643290442000310
Garrard, P., Lambon Ralph, M. A., Hodges, J. R., and Patterson, K. (2001). Prototypicality, distinctiveness, and intercorrelation: analyses of the semantic attributes of living and nonliving concepts. Cogn. Neuropsychol. 18, 125–174. doi: 10.1080/02643290125857
Garrard, P., Lambon Ralph, M. A., Patterson, K., Pratt, K. H., and Hodges, J. R. (2005). Semantic feature knowledge and picture naming in dementia of Alzheimer's type: a new approach. Brain Lang. 93, 79–94. doi: 10.1016/j.bandl.2004.08.003
Gathercole, V. C. (1985). “He has too much hard questions:” the acquisition of the linguistic mass-count distinction in much and many. J. Child Lang. 12, 395–415. doi: 10.1017/S0305000900006504
Gibb, W., Luthert, P., and Marsden, C. (1989). Corticobasal degeneration. Brain 112, 1171–1192. doi: 10.1093/brain/112.5.1171
Gilhooly, K., and Logie, R. (1980). Age-of-acquisition, imagery, concreteness, familiarity, and ambiguity measures for 1,944 words. Behav. Res. Methods 12, 395–427. doi: 10.3758/BF03201693
Jefferies, E., Patterson, K., Jones, R. W., and Lambon Ralph, M. A. (2009). Comprehension of concrete and abstract words in semantic dementia. Neuropsychology 23, 492–499. doi: 10.1037/a0015452
Jones, G. V. (1985). Deep dyslexia, imageability, and ease of predication. Brain Lang. 24, 1–19. doi: 10.1016/0093-934X(85)90094-X
Kousta, S. T., Vigliocco, G., Vinson, D. P., Andrews, M., and Del Campo, E. (2011). The representation of abstract words: why emotion matters. J. Exp. Psychol. Gen. 140, 14–34. doi: 10.1037/a0021446
Kousta, S.-T., Vinson, D. P., and Vigliocco, G. (2009). Emotion words, regardless of polarity, have a processing advantage over neutral words. Cognition 112, 473–481. doi: 10.1016/j.cognition.2009.06.007
Kroll, J. F., and Merves, J. S. (1986). Lexical access for concrete and abstract words. J. Exp. Psychol. Learn. Mem. Cogn. 12, 92–107. doi: 10.1037/0278-7393.12.1.92
Lakoff, G. (1990). Women, Fire, and Dangerous Things: What Categories Reveal About the Mind. Chicago, IL: University of Chicago Press.
Lakoff, G. (2012). Explaining embodied cognition results. Top. Cogn. Sci. 4, 773–785. doi: 10.1111/j.1756-8765.2012.01222.x
Landauer, T. K., and Dumais, S. T. (1997). A solution to Plato's problem: the latent semantic analysis theory of acquisition, induction, and representation of knowledge. Psychol. Rev. 104, 211. doi: 10.1037/0033-295X.104.2.211
Locke, J. (1685). “An essay on human understanding,” in Of Abstract and Concrete Terms, Chapter 7, ed M. Ruse (New York, NY; Amherst, MA: Prometheus books), 384–385.
Moll, J., Zahn, R., de Oliveira-Souza, R., Krueger, F., and Grafman, J. (2005). The neural basis of human moral cognition. Nat. Rev. Neurosci. 6, 799–809. doi: 10.1038/nrn1768
Newcombe, P. I., Campbell, C., Siakaluk, P. D., and Pexman, P. M. (2012). Effects of emotional and sensorimotor knowledge in semantic processing of concrete and abstract nouns. Front. Hum. Neurosci. 6:275. doi: 10.3389/fnhum.2012.00275
Osgood, C. E., Sebeok, T. A., Gardner, J. W., Carroll, J. B., Newmark, L. D., Ervin, S. M., et al. (1954). Psycholinguistics: a survey of theory and research problems. J. Abnorm. Soc. Psychol. 49(4 pt 2), i. doi: 10.1037/h0063655
Paivio, A. (1991). Dual coding theory: retrospect and current status. Can. J. Psychol. 45, 255–287. doi: 10.1037/h0084295
Paivio, A. (2013). Dual coding theory, word abstractness, and emotion: a critical review of Kousta et al. (2011). J. Exp. Psychol. Gen. 142, 282–287. doi: 10.1037/a0027004
Paivio, A., Yuille, J. C., and Madigan, S. A. (1968). Concreteness, imagery, and meaningfulness values for 925 nouns. J. Exp. Psychol. 76(1 pt 2), 1–25. doi: 10.1037/h0025327
Plaut, D. C., and Shallice, T. (1993). Deep dyslexia: a case study of connectionist neuropsychology. Cogn. Neuropsychol. 10, 377–500. doi: 10.1080/02643299308253469
Reilly, J. (2005). A tale of two imageabilities: An interaction of sound and meaning in natural language perception. (66), Dissertation Abstracts International: Section B: The Sciences and Engineering, US. Available online at: https://search.ebscohost.com/login.aspx?direct=true&db=psyh&AN=2005-99024-143&site=ehost-live
Reilly, J., Chrysikou, E. G., and Ramey, C. H. (2007). Support for hybrid models of the age of acquisition of English nouns. Psychon. Bull. Rev. 14, 1164–1170. doi: 10.3758/BF03193107
Reilly, J., and Kean, J. (2007). Formal distinctiveness of high- and low-imageability nouns: analyses and theoretical implications. Cogn. Sci. 31, 157–168. doi: 10.1080/03640210709336988
Reilly, J., Westbury, C., Kean, J., and Peelle, J. E. (2012). Arbitrary symbolism in natural language revisited: when word forms carry meaning. PLoS ONE 7:e42286. doi: 10.1371/journal.pone.0042286
Rogers, T. T., Lambon Ralph, M. A., Garrard, P., Bozeat, S., McClelland, J. L., Hodges, J. R., et al. (2004). Structure and deterioration of semantic memory: a neuropsychological and computational investigation. Psychol. Rev. 111, 205–235. doi: 10.1037/0033-295X.111.1.205
Rosch, E. H. (1973). Natural categories. Cogn. Psychol. 4, 328–350. doi: 10.1016/0010-0285(73)90017-0
Sadoski, M. (2005). A dual coding view of vocabulary learning. Read. Writing Q. 21, 221–238. doi: 10.1080/10573560590949359
Sadoski, M., and Paivio, A. (2004). A dual coding theoretical model of reading. Theor. Models Processes Read. 5, 1329–1362. doi: 10.1598/0872075028.47
Schwanenflugel, P. J., Akin, C., and Luh, W.-M. (1992). Context availability and the recall of abstract and concrete words. Mem. Cognit. 20, 96–104. doi: 10.3758/BF03208259
Schwanenflugel, P. J., Harnishfeger, K. K., and Stowe, R. W. (1988). Context availability and lexical decisions for abstract and concrete words. J. Mem. Lang. 27, 499–520. doi: 10.1016/0749-596X(88)90022-8
Schwanenflugel, P. J., and Shoben, E. J. (1983). Differential context effects in the comprehension of abstract and concrete verbal materials. J. Exp. Psychol. Learn. Mem. Cogn. 9, 82–102. doi: 10.1037/0278-7393.9.1.82
Strain, E., Patterson, K., and Seidenberg, M. S. (2002). Theories of word naming interact with spelling-sound consistency. J. Exp. Psychol. Learn. Mem. Cogn. 28, 207–214. discussion: 215–220. doi: 10.1037/0278-7393.28.1.207
Vigliocco, G., Kousta, S.-T., Della Rosa, P. A., Vinson, D. P., Tettamanti, M., Devlin, J. T., et al. (2013). The neural representation of abstract words: the role of emotion. Cereb. Cortex. doi: 10.1093/cercor/bht025. [Epub ahead of print].
Vigliocco, G., Vinson, D. P., Lewis, W., and Garrett, M. F. (2004). Representing the meanings of object and action words: the featural and unitary semantic space hypothesis. Cogn. Psychol. 48, 422–488. doi: 10.1016/j.cogpsych.2003.09.001
Walker, I., and Hulme, C. (1999). Concrete words are easier to recall than abstract words: evidence for a semantic contribution to short-term serial recall. J. Exp. Psychol. Learn. Mem. Cogn. 25, 1256–1271. doi: 10.1037/0278-7393.25.5.1256
Walsh, V. (2003). A theory of magnitude: common cortical metrics of time, space and quantity. Trends Cogn. Sci. 7, 483–488. doi: 10.1016/j.tics.2003.09.002
Ward, J. H. Jr. (1963). Hierarchical grouping to optimize an objective function. J. Am. Stat. Assoc. 58, 236–244. doi: 10.1080/01621459.1963.10500845
Warrington, E. K. (1975). The selective impairment of semantic memory. Q. J. Exp. Psychol. 27, 635–657. doi: 10.1080/14640747508400525
Warrington, E. K. (1981). Concrete word dyslexia. Br. J. Psychol. 72, 175–196. doi: 10.1111/j.2044-8295.1981.tb02175.x
Westbury, C., and Moroschan, G. (2009). Imageability x phonology interactions during lexical access: effects of modality, phonological neighbourhood, and phonological processing efficiency. Ment. Lex. 4, 115–145. doi: 10.1075/ml.4.1.05wes
Wiemer-Hastings, K., and Xu, X. (2005). Content differences for abstract and concrete concepts. Cogn. Sci. 29, 719–736. doi: 10.1207/s15516709cog0000_33
Zwaan, R. A., and Taylor, L. J. (2006). Seeing, acting, understanding: motor resonance in language comprehension. J. Exp. Psychol. Gen. 135, 1–11. doi: 10.1037/0096-3445.135.1.1
Keywords: semantic memory, concreteness, abstract concepts, embodied cognition, emotion, magnitude
Citation: Troche J, Crutch S and Reilly J (2014) Clustering, hierarchical organization, and the topography of abstract and concrete nouns. Front. Psychol. 5:360. doi: 10.3389/fpsyg.2014.00360
Received: 10 January 2014; Accepted: 06 April 2014;
Published online: 28 April 2014.
Edited by:
Guy Dove, University of Louisville, USACopyright © 2014 Troche, Crutch and Reilly. This is an open-access article distributed under the terms of the Creative Commons Attribution License (CC BY). The use, distribution or reproduction in other forums is permitted, provided the original author(s) or licensor are credited and that the original publication in this journal is cited, in accordance with accepted academic practice. No use, distribution or reproduction is permitted which does not comply with these terms.
*Correspondence: Joshua Troche, University of Florida, 336 Dauer Hall, PO Box 100174, Gainesville, FL 32610, USA e-mail:amV0Z2F0b3JAcGhocC51ZmwuZWR1