- 1Department of Psychology, The Catholic University of America, Washington, DC, USA
- 2Department of Psychology, Georgetown University, Washington, DC, USA
It is often held that although explicit learning declines in the course of normal aging, implicit learning is relatively preserved. Here we summarize research from our group which leads us to argue that some forms of implicit learning do decline with adult age. In particular, we propose that there are age-related declines in implicit learning of probabilistic sequential relationships that occur across the adult lifespan, and that they reflect, at least in part, age-related striatal dysfunction. We first review behavioral evidence supporting this age-related decline and then evidence from patient groups, genetics, and neuroimaging supporting this striatal dysfunction hypothesis.
People are remarkably sensitive to spatial and temporal relationships among objects and events. These relationships are typically probabilistic and sequential, in that events often, but not always, predict later ones. Learning about these relationships is important throughout life because it enables people to perceive the world efficiently, to learn and use language, to make decisions based on experience, and even to engage in social interaction (Saffran et al., 1996; Lieberman, 2000; Kuhl, 2004; Conway and Pisoni, 2008). An intriguing characteristic of such probabilistic sequence learning is that it often takes place outside of awareness in that we need not make any special effort to learn, and are unable to articulate what we have learned. As a result of such learning, we know that a sentence is ungrammatical without being able to cite the syntactic rules it violates or we have a bad feeling about a new acquaintance without being able to express what social cues led to that impression. Reber (1967) termed this implicit learning to distinguish it from deliberate, effortful explicit/declarative learning.
It is often claimed, particularly in general reviews of cognitive aging, that implicit, unlike explicit, learning and memory are age invariant. But there is a wide range of implicit processes, and different forms of implicit learning. It appears that some of these are spared with aging, including repetition priming as well as some skills learned early and then practiced throughout life (Ikier et al., 2008; Morrow et al., 2008; Laver, 2009). Nonetheless, none of these involves picking up subtle, sequential probabilistic regularities. In fact, one skill that does call, at least in part, on implicit learning, second-language acquisition (Hedenius et al., 2011; Morgan-Short et al., 2012a,b; Frost et al., 2013), apparently declines in a linear fashion with adult age (Hakuta et al., 2003).
This perspective paper does not offer a review of the growing literature on implicit learning and aging (see review by King et al., 2013). Instead, we aim to summarize findings from our own group, including our students and collaborators, supporting two claims. First, we propose that implicit probabilistic sequence learning is not spared in healthy aging; rather there are persistent age-related learning deficits that can be detected as early as middle age. Second, we propose that these declines reflect changes in the brain that occur with aging, specifically, declines in a striatal-based learning system.
Age-related Differences in Learning
Most studies of implicit learning and aging have examined perceptual/motor based sequence learning, using the Serial Response Time (SRT) task (Nissen and Bullemer, 1987). In the original SRT people respond as quickly as possible to each of a series of events that, unbeknownst to them, follow a regular repeating spatial pattern. With practice, people become faster at responding overall, reflecting general improvement. More importantly, sequence-specific learning is revealed by the fact that response times increase abruptly if the sequential regularity is removed. Virtually all SRT studies find that older adults do show sequence-specific learning, but studies differ in whether age deficits are detected. The reason for the different results is unclear. Most studies revealing age equality in learning have used relatively simple deterministic regularities, in that each event or sequence of events perfectly predicts a subsequent one (Howard and Howard, 1989, 1992; Frensch and Miner, 1994; Cherry and Stadler, 1995; Salthouse et al., 1999; Dennis et al., 2006; Gaillard et al., 2009).
In contrast, our work using probabilistic versions of the SRT consistently reveals age-related deficits. For example, as illustrated in Figure 1A, we developed an Alternating Serial Response Time (ASRT) task, which differs from the traditional SRT in that predictable and random events occur on alternate trials, thereby introducing probabilistic 2nd order structure (i.e., event t predicts event t + 2 with high probability). For example, a given participant might encounter the regularity 1r2r4r3r1r2r4r3r …., where 1–4 represent different events and r represents any one of these. Thus, some runs of three events or triplets, occur with high probability because they conform to the regularity (e.g., 1×2 here where × is any event), whereas others occur infrequently (e.g., 2×1), thereby manipulating the conditional probabilities of successive events. Our findings suggest that people do not learn the alternating regularity per se, but rather become sensitive to the predictive relationships (Howard and Howard, 1997); with practice, RT and accuracy for these two triplet types diverge, with increasingly faster and more accurate responding to high relative to low probability triplets.
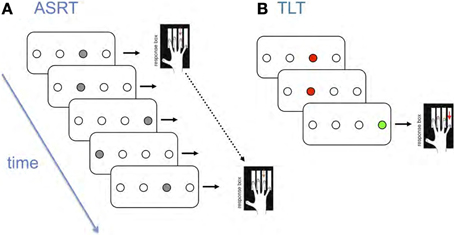
Figure 1. Schematic representation of (A) the Alternating Serial Reaction Time Task (ASRT) and (B) The Triplets Learning Task (TLT).
We have reported that young, middle-aged and old adults do learn 2nd order probabilistic sequences in the ASRT, but there are age deficits especially as training progresses (Howard and Howard, 1997; Howard et al., 2004). We have also shown that age-related deficits occur for spatial and non-spatial stimuli, persisting even with extended practice distributed over as much as 10 h of training (Dennis et al., 2003, 2006; Negash et al., 2003; Howard et al., 2004). In fact, what is most striking is that while old adults learn as much as young early in training, they asymptote well below younger people.
These age deficits in learning are detectable in middle-age (aged 34–53) (Feeney et al., 2002) and are more pronounced in healthy old-old than young-old adults (aged 65–73 and 76–80, respectively) (Howard and Howard, 1997), suggesting there might be a gradual decline in learning across the adult lifespan. Janacsek et al. (2012) have replicated adult age deficits in ASRT learning using a lifespan sample, but longitudinal data are needed. Age deficits are even more pronounced when a 3rd-order regularity is used (i.e., event t predicts event t + 3), and under some conditions older adults do not learn 3rd-order associations even with extended training (Howard et al., 2004; Bennett et al., 2007).
To investigate whether the age deficits in the ASRT are due to older adults' difficulties with motor sequencing, we developed a Triplets Learning Task (TLT, Figure 1B). It is similar to the ASRT, but rather than responding to every item in a stream, participants observe two red cues and then respond to a third green target on each of a series of three-event trials (Howard et al., 2008). We then vary the relation between the cue and target events; for example, for 2nd-order regularities (comparable to the ASRT) the first cue probabilistically predicts the target, and for 1st-order regularities the second cue predicts the target. The TLT yields learning data that are very similar to the ASRT; young and old adults learn equally well early in training, but old adults asymptote at a lower level (Howard et al., 2008; Simon et al., 2010). These TLT findings indicate that the age-related deficits in ASRT learning are not due solely to motor sequencing deficits.
Age deficits appear in the TLT for 1st as well as for 2nd order regularities (Howard et al., 2008; Stillman et al., 2012), suggesting that even simple first-order probabilistic structure can lead to age differences. This is important, because given the slower processing and reduced capacity that accompanies aging (Salthouse, 1996), deficits in 2nd and higher-order learning might reflect a reduced availability of the events to be associated, rather than a learning deficit per se. The fact that older adults are poorer than young at learning 1st order structure, where only immediately adjacent events need to be associated, suggests a deficit in associative learning, and also indicates that age related deficits are not limited to learning higher order structure.
There is always concern that observed age deficits might reflect explicit “contamination” such that the young are better, not because of superior implicit learning, but because they have gained more explicit/declarative knowledge than older people. However, the age deficits observed in the ASRT and TLT are unlikely due to age differences in explicit knowledge for several reasons. First, sensitive tests indicate people are not aware of the regularity. For example, people are unable to differentiate between high and low probability triplets in a subsequent recognition test even with substantial learning during the ASRT and TLT tasks (Howard et al., 2004, 2008). In addition, using an “implicit/explicit” variation of the ASRT that enables people to gain explicit knowledge, we found that implicit learning occurs independent of any explicit knowledge that is acquired (Song et al., 2007). There is also evidence that learning in the TLT, unlike explicit learning, is not capacity demanding; both old and young people learn 2nd order regularities even in the presence of a working memory load, with little or no effect on learning (Gamble et al., in press). Finally, both old and young adults show near-perfect retention of an ASRT sequence over at least 1 year, consistent with the excellent long-term retention characteristic of implicit/procedural skills (Romano et al., 2010).
We have focused here on our own group's work using the ASRT and TLT, but we are not alone in finding age-related deficits in implicit sequence learning. For example, Curran (1997) found age deficits when 12-element 2nd-order deterministic SRT sequences were intermixed with blocks of random sequences whereas Daselaar et al. (2003) found age equivalence using a similar procedure. Maddox and colleagues (Filoteo and Maddox, 2004; Maddox et al., 2010) reported age deficits in information-integration learning on a classification task known to rely on the striatal system. Further, Weiermann (Weiermann and Meier, 2012) has reported age deficits in a study requiring simultaneous learning of both a repeating task and response sequence.
In summary, there is accumulating evidence that not all forms of implicit learning are spared the negative effects of aging, suggesting that there is a fundamental deficit in the system underlying learning of probabilistic sequential regularities. Some of our more recent work investigates the neural bases of these age differences.
Biological Foundations of Age Differences in Learning
Recent research indicates that in young adults, implicit sequence learning involves two interacting neural systems; one based primarily on striatal (including caudate) and the other on medial temporal lobe (MTL, including hippocampus) networks (Henke, 2010; Dennis and Cabeza, 2011). The MTL system is characterized by the relatively fast acquisition of associations, and predominates early in training, whereas the striatal system learns more slowly, gradually building up associations among events (Poldrack and Packard, 2003) and becoming increasingly important as training progresses.
There is cross-sectional and longitudinal evidence that the striatum undergoes substantial age related declines in structure and function beginning in early adulthood, including declines in the overall level of striatal dopamine and in dopamine receptor density (Raz et al., 2005; Backman et al., 2010). In contrast, recent research suggests that the MTL is relatively spared in healthy aging, at least until very advanced age, even though it is affected early in Alzheimer's disease (Hedden and Gabrieli, 2005; Raz et al., 2005).
Thus, our recent work has been guided by a striatal aging hypothesis which proposes that age-related deficits in implicit probabilistic sequence learning reflect declines in a striatal-based learning system (Howard and Howard, 2012), similar to proposals advanced by others (Filoteo and Maddox, 2004; Poldrack and Foerde, 2008; Rieckmann and Bäckman, 2009). This hypothesis is consistent with the pattern of age deficits in the TLT and ASRT described above. This includes the evidence suggesting a gradual decline in learning across the adult years, similar to the gradual decline in the striatum, and the finding that age deficits are greater late in practice, when the striatum is known to dominate learning. To obtain more direct evidence, we have used patient and genetics studies as well as structural and functional neuroimaging with healthy young and older adults as illustrated below.
Consistent with the striatal aging hypothesis, we have demonstrated selective impairment of ASRT learning in individuals with corticobasal syndrome (CBS), a progressive neurological disorder characterized by atrophy of striatal regions with symptomatology similar to Parkinson's Disease (Negash et al., 2007). We have also shown that TLT learning is related to the dopamine transporter gene, DAT1, which plays an important role in striatal dopamine signaling. A variant of this gene, the 9-repeat allele, is associated with higher synaptic dopamine levels and with greater caudate volume and activity (Bertolino et al., 2006). We observed more learning in college-aged 9-carriers compared to 10/10 homozygotes on the TLT (Simon et al., 2011). Furthermore, this advantage only emerged late in training when responding would be expected to depend largely on the striatal system for young people. Taken together, these data suggest the importance of striatal dopamine for learning in the ASRT and TLT.
We have used fMRI to demonstrate that striatal activation is related to implicit sequence learning in the TLT for both young and older adults (Simon et al., 2012). Simon contrasted activation to high vs. low probability target events, i.e., a “response to predictability,” and found that early in training, younger adults displayed a greater caudate response than older adults. Furthermore, in late training, the degree of learning correlated positively with caudate response across young, but not older adults, suggesting that late in training young adults relied more on striatal networks than did old. In contrast, the degree of late learning correlated positively with hippocampal activation (response to predictability) for older adults, but not younger. Taken together, these findings suggest that the balance between the two neural systems differs in young and older adults. This is consistent with the striatal aging hypothesis and with recent evidence that in the face of this striatal deficit, older adults rely to a greater degree on the MTL system for implicit learning (Rieckmann and Bäckman, 2009; Dennis and Cabeza, 2011; Simon et al., 2012).
Although most neuroimaging research has focused on age-related changes in the structure and function of gray matter, there are also widespread declines in the integrity of white matter (Sullivan and Pfefferbaum, 2007; Madden et al., 2009; Bennett et al., 2010). We have used diffusion tensor imaging (DTI) tractography to show that the integrity of white matter tracts in cortico-striatal networks relates to age-deficits in the ASRT thereby further implicating these networks in implicit learning (Bennett et al., 2011). Bennett assessed the integrity of several tracts including a caudate-dorsolateral prefrontal cortex (DLPFC) tract in both young and older adults, using a measure of fractional anisotropy (FA) such that higher FA indicates high white matter integrity. Consistent with the striatal aging hypothesis, older adults showed lower FA for the caudate-DLPFC tracts than younger adults, whereas FA did not differ between groups for the other tracts we examined (including a hippocampal-DLPFC tract). More importantly, individuals with greater caudate-DLPFC tract FA revealed more learning. This positive correlation was observed for both young and older adults, but with older adults showing lower FA and less learning overall than young. In addition, left caudate-DPLFC tract integrity mediated the age differences in late learning, with tract FA attenuating the age group effect on learning by 93%. These findings complement studies of gray matter substrates and suggest that age-related white matter loss in striatal tracts contributes to age deficits in learning.
Summary and Future Directions
The above studies show that there are age deficits in implicit probabilistic sequence learning, and they point to striatal aging as a contributing factor. However, important questions remain. As we indicated above, the characteristics of an implicit learning task that yield age differences remain unclear, and the exact conditions under which the striatum is important for learning are not fully known (see, e.g., Schultz, 2007; Gallistel and Matzel, 2013). We have argued here that age deficits are observed consistently with probabilistic sequences, whether simple first-order or more complex higher-order structure, because such sequences are optimally learned via the striatal system. However, to the extent the striatum is needed for optimal learning of other relationships, including perhaps complex deterministic ones, age deficits will also be observed.
In addition, we focused here on age group differences, but there are substantial differences in sequence learning among individuals of the same age (e.g., Janacsek et al., 2012). Recent studies have begun to identify the source of these differences, including the evidence above that within-group sequence learning is related to dopamine genotype (Simon et al., 2011) and to white matter integrity (Bennett et al., 2011). We have also found relationships with life experience, including musical training and videogaming (Romano-Bergstrom et al., 2011).
Additional work should also address the implications of these learning differences for domains such as reading, decision-making, second language learning, and social cognition. For example, ASRT and TLT learning are associated with reading ability as assessed with standardized diagnostic tests in young (Howard et al., 2006) and older adults (Bennett et al., 2008) and may contribute to adult age differences in learning a second language (Schwab et al., 2013). Age-deficits in experience-based decision making may also reflect a learning impairment (Mata et al., 2011; Seaman et al., 2013).
Beyond this, additional work should investigate the neural dynamics that accompany implicit learning. We have recently used functional connectivity methods to show that intrinsic connectivity between the caudate and MTL is correlated with sequence learning in young adults (Stillman et al., in press), and we have used EEG/ERP to examine within-trial processes (Kiser et al., 2012).
To summarize, throughout life people are able to learn implicitly about regularities in their environment, enabling them to adapt to a changing world. Although some kinds of implicit learning are spared, we have reported evidence that implicit probabilistic sequence learning is not spared, likely reflecting age-related changes to the striatal system. Future work should further investigate the role of the striatum in individual differences in brain/behavior relationships as well as the implications for a broad range of important everyday tasks.
Author Contributions
The authors have contributed equally to the work.
Conflict of Interest Statement
The authors declare that the research was conducted in the absence of any commercial or financial relationships that could be construed as a potential conflict of interest.
Acknowledgments
This work was supported by NIA grants R01AG036863 and R37AG15450, and by several NIH NRSA predoctoral fellowships. We thank our collaborators for their support and wisdom, especially Chandan Vaidya, David Madden, and Xiong Jiang as well as our past and present graduate students for their substantial contributions to the work summarized here: Illana Bennett, Nancy Dennis, John Feeney, Katherine Gamble, Karin Japikse, Sarah Halpert, Seth Kiser, Selamawit Negash, Jennifer Romano-Bergstrom, Kendra Seaman, Jessica Simon, Sunbin Song, Chelsea Stillman, and Helen Yankovich. We are also grateful to Vinciane Gaillard and Axel Cleeremans for organizing this special issue and the very stimulating conference that led to it.
References
Backman, L., Lindenberger, U., Li, S. C., and Nyberg, L. (2010). Linking cognitive aging to alterations in dopamine neurotransmitter functioning: recent data and future avenues. Neurosci. Biobehav. Rev. 34, 670–677. doi: 10.1016/j.neubiorev.2009.12.008
Bennett, I. J., Howard, J. H. Jr., and Howard, D. V. (2007). Age-related differences in implicit learning of subtle third-order sequential structure. J. Gerontol. B Psychol. Sci. Soc. Sci. 62, P98–P103. doi: 10.1093/geronb/62.2.P98
Bennett, I. J., Madden, D. J., Vaidya, C. J., Howard, D. V., and Howard, J. H. Jr. (2010). Age-related differences in multiple measures of white matter integrity: a diffusion tensor imaging study of healthy aging. Hum. Brain Mapp. 31, 378–390. doi: 10.1002/hbm.20872
Bennett, I. J., Madden, D. J., Vaidya, C. J., Howard, J. H. Jr., and Howard, D. V. (2011). White matter integrity correlates of implicit sequence learning in healthy aging. Neurobiol. Aging 32, 2317.e1–2317.e12. doi: 10.1016/j.neurobiolaging.2010.03.017
Bennett, I. J., Romano, J. C., Howard, J. H. Jr., and Howard, D. V. (2008). Two forms of implicit learning in young adults with dyslexia. Ann. N.Y. Acad. Sci. 1145, 184–198. doi: 10.1196/annals.1416.006
Bertolino, A., Blasi, G., Latorre, V., Rubino, V., Rampino, A., Sinibaldi, L., et al. (2006). Additive effects of genetic variation in dopamine regulating genes on working memory cortical activity in human brain. J. Neurosci. 26, 3918–3922. doi: 10.1523/JNEUROSCI.4975-05.2006
Cherry, K. E., and Stadler, M. A. (1995). Implicit learning of a nonverbal sequence in younger and older adults. Psychol. Aging 10, 379–394. doi: 10.1037/0882-7974.10.3.379
Conway, C. M., and Pisoni, D. B. (2008). Neurocognitive basis of implicit learning of sequential structure and its relation to language processing. Ann. N.Y. Acad. Sci. 1145, 113–131. doi: 10.1196/annals.1416.009
Curran, T. (1997). Effects of aging on implicit sequence learning: accounting for sequence structure and explicit knowledge. Psychol. Res. 60, 24–41. doi: 10.1007/BF00419678
Daselaar, S. M., Rombouts, S. A., Veltman, D. J., Raaijmakers, J. G., and Jonker, C. (2003). Similar network activated by young and old adults during the acquisition of a motor sequence. Neurobiol. Aging 24, 1013–1019. doi: 10.1016/S0197-4580(03)00030-7
Dennis, N. A., and Cabeza, R. (2011). Age-related dedifferentiation of learning systems: an fmri study of implicit and explicit learning. Neurobiol. Aging 32, 2318.e17–2318.e30. doi: 10.1016/j.neurobiolaging.2010.04.004
Dennis, N. A., Howard, J. H. Jr., and Howard, D. V. (2003). Age deficits in learning sequences of spoken words. J. Gerontol. B Psychol. Sci. Soc. Sci. 58, P224–P227. doi: 10.1093/geronb/58.4.P224
Dennis, N. A., Howard, J. H. Jr., and Howard, D. V. (2006). Implicit sequence learning without motor sequencing in young and old adults. Exp. Brain Res. 175, 153–164. doi: 10.1007/s00221-006-0534-3
Feeney, J. J., Howard, J. H. Jr., and Howard, D. V. (2002). Implicit learning of higher order sequences in middle age. Psychol. Aging 17, 351–355. doi: 10.1037/0882-7974.17.2.351
Filoteo, J. V., and Maddox, W. T. (2004). A quantitative model-based approach to examining aging effects on information-integration category learning. Psychol. Aging 19, 171–182. doi: 10.1037/0882-7974.19.1.171
Frensch, P. A., and Miner, C. S. (1994). Effects of presentation rate and individual differences in short-term memory capacity on an indirect measure of serial learning. Mem. Cogn. 22, 95–110. doi: 10.3758/BF03202765
Frost, R., Siegelman, N., Narkiss, A., and Afek, L. (2013). What predicts successful literacy acquisition in a second language. Psychol. Sci. 24, 1243–1252. doi: 10.1177/0956797612472207
Gaillard, V., Destrebecqz, A., Michiels, S., and Cleeremans, A. (2009). Effects of age and practice in sequence learning: a graded account of ageing, learning, and control. Eur. J. Cogn. Psychol. 21, 255–282. doi: 10.1080/09541440802257423
Gallistel, C. R., and Matzel, L. D. (2013). The neuroscience of learning: beyond the hebbian synapse. Annu. Rev. Psychol. 64, 169–200. doi: 10.1146/annurev-psych-113011-143807
Gamble, K. R., Howard, J. H. Jr., and Howard, D. V. (in press). Does a simultaneous memory load affect older and younger adults' implicit associative learning. Neuropsychol. Dev. Cogn. B Aging Neuropsychol. Cogn. 37–41.
Hakuta, K., Bialystok, E., and Wiley, E. (2003). Critical evidence: a test of the critical-period hypothesis for second-language acquisition. Psychol. Sci. 14, 31–38. doi: 10.1111/1467-9280.01415
Hedden, T., and Gabrieli, J. D. E. (2005). Healthy and pathological processes in adult development: new evidence from neuroimaging of the aging brain. Curr. Opin. Neurol. Neurosurg., 18, 740–747. doi: 10.1097/01.wco.0000189875.29852.48
Hedenius, M., Persson, J., Tremblay, A., Adi-Japha, E., Veríssimo, J., Dye, C. D., et al. (2011). Grammar predicts procedural learning and consolidation deficits in children with specific language impairment. Res. Dev. Disabil. 32, 2362–2375. doi: 10.1016/j.ridd.2011.07.026
Henke, K. (2010). A model for memory systems based on processing modes rather than consciousness. Nat. Rev. Neurosci. 11, 523–532. doi: 10.1038/nrn2850
Howard, D. V., and Howard, J. H. Jr. (1989). Age differences in learning serial patterns: direct versus indirect measures. Psychol. Aging 4, 357–364. doi: 10.1037/0882-7974.4.3.357
Howard, D. V., and Howard, J. H. Jr. (1992). Adult age differences in the rate of learning serial patterns: evidence from direct and indirect tests. Psychol. Aging 7, 232–241. doi: 10.1037/0882-7974.7.2.232
Howard, D. V., and Howard, J. H. Jr. (2012). “Dissociable forms of implicit learning in aging,” in Memory and Aging: Current Issues and Future Directions, eds M. Naveh-Benjamin and N. Ohta (New York, NY: Psychology Press), 125–151.
Howard, D. V., Howard, J. H. Jr. Japikse, K., DiYanni, C., Thompson, A., and Somberg, R. (2004). Implicit sequence learning: effects of level of structure, adult age, and extended practice. Psychol. Aging 19, 79–92. doi: 10.1037/0882-7974.19.1.79
Howard, J. H. Jr., and Howard, D. V. (1997). Age differences in implicit learning of higher order dependencies in serial patterns. Psychol. Aging 12, 634–656. doi: 10.1037/0882-7974.12.4.634
Howard, J. H. Jr. Howard, D. V., Dennis, N. A., and Kelly, A. J. (2008). Implicit learning of predictive relationships in three-element visual sequences by young and old adults. J. Exp. Psychol. Learn. Mem. Cogn. 34, 1139–1157. doi: 10.1037/a0012797
Howard, J. H. Jr. Howard, D. V., Japikse, K. C., and Eden, G. F. (2006). Dyslexics are impaired on implicit higher-order sequence learning, but not on implicit spatial context learning. Neuropsychologia 44, 1131–1144. doi: 10.1016/j.neuropsychologia.2005.10.015
Ikier, S., Yang, L., and Hasher, L. (2008). Implicit proactive interference, age, and automatic versus controlled retrieval strategies. Psychol. Sci. 19, 456–461. doi: 10.1111/j.1467-9280.2008.02109.x
Janacsek, K., Fiser, J., and Nemeth, D. (2012). The best time to acquire new skills: age-related differences in implicit sequence learning across the human lifespan. Dev. Sci. 15, 496–505. doi: 10.1111/j.1467-7687.2012.01150.x
King, B. R., Fogel, S. M., Albouy, G., and Doyon, J. (2013). Neural correlates of the age-related changes in motor sequence learning and motor adaptation in older adults. Front. Hum. Neurosci. 7:142. doi: 10.3389/fnhum.2013.00142
Kiser, S. A., Fuller, R., O'Neil, K., Howard, D. V., and Howard, J. H. Jr. (2012). “ERP topography reveals learning-related changes in N400 effect to implicit stimulus probabilities,” in Society for Neuroscience Conference meeting (New Orleans, LA).
Kuhl, P. K. (2004). Early language acquisition: cracking the speech code. Nat. Rev. Neurosci. 5, 831–843. doi: 10.1038/nrn1533
Laver, G. D. (2009). Adult aging effects on semantic and episodic priming in word recognition. Psychol. Aging 24, 28–39. doi: 10.1037/a0014642
Lieberman, M. D. (2000). Intuition: a social cognitive neuroscience approach. Psychol. Bull. 126, 109–137. doi: 10.1037/0033-2909.126.1.109
Madden, D. J., Bennett, I. J., and Song, A. W. (2009). Cerebral white matter integrity and cognitive aging: contributions from diffusion tensor imaging. Neuropsychol. Rev. 19, 415–435. doi: 10.1007/s11065-009-9113-2
Maddox, W. T., Pacheco, J., Reeves, M., Zhu, B., and Schnyer, D. M. (2010). Rule-based and information-integration category learning in normal aging. Neuropsychologia 48, 2998–3008. doi: 10.1016/j.neuropsychologia.2010.06.008
Mata, R., Josef, A. K., Samanez-Larkin, G. R., and Hertwig, R. (2011). Age differences in risky choice: a meta-analysis. Ann. N.Y. Acad. Sci. 1235, 18–29. doi: 10.1111/j.1749-6632.2011.06200.x
Morgan-Short, K., Finger, I., Grey, S., and Ullman, M. T. (2012a). Second language processing shows increased native-like neural responses after months of no exposure. PLoS ONE 7:e32974. doi: 10.1371/journal.pone.0032974
Morgan-Short, K., Finger, I., Grey, S., and Ullman, M. T. (2012b). Explicit and implicit second language training differentially affect the achievement of native-like brain activation patterns. J. Cogn. Neurosci. 24, 933–947. doi: 10.1162/jocn_a_00119
Morrow, D. G., Miller, L. M., Ridolfo, H. E., Mangor, C., Fischer, U. M., Kokayeff, N. K., et al. (2008). Expertise and age differences in pilot decision making. Aging Neuropsychol. Cogn. 16, 33–35. doi: 10.1080/13825580802195641
Negash, S., Boeve, B. F., Geda, Y. E., Smith, G. E., Knopman, D. S., Ivnik, R. J., et al. (2007). Implicit learning of sequential regularities and spatial contexts in corticobasal syndrome. Neurocase 13, 133–143. doi: 10.1080/13554790701401852
Negash, S., Howard, D. V., Japikse, K. C., and Howard, J. H. Jr. (2003). Age-related differences in implicit learning of non-spatial sequential patterns. Aging Neuropsychol. Cogn. 10, 108–121. doi: 10.1076/anec.10.2.108.14462
Nissen, M. J., and Bullemer, P. (1987). Attentional requirements of learning: evidence from performance measures. Cogn. Psychol. 19, 1–32. doi: 10.1016/0010-0285(87)90002-8
Poldrack, R. A., and Foerde, K. (2008). Category learning and the memory systems debate. Neurosci. Biobehav. Rev. 32, 197–205. doi: 10.1016/j.neubiorev.2007.07.007
Poldrack, R. A., and Packard, M. G. (2003). Competition among multiple memory systems: converging evidence from animal and human brain studies. Neuropsychologia 41, 245–251. doi: 10.1016/S0028-3932(02)00157-4
Raz, N., Lindenberger, U., Rodrigue, K. M., Kennedy, K. M., Head, D., Williamson, A., et al. (2005). Regional brain changes in aging healthy adults: general trends, individual differences and modifiers. Cereb. Cortex 15, 1676–1689. doi: 10.1093/cercor/bhi044
Reber, A. S. (1967). Implicit learning of artificial grammars. J. Verbal Learn. Verbal Behav. 6, 855–863. doi: 10.1016/S0022-5371(67)80149-X
Rieckmann, A., and Bäckman, L. (2009). Implicit learning in aging: extant patterns and new directions. Neuropsychol. Rev. 19, 490–503. doi: 10.1007/s11065-009-9117-y
Romano, J. C., Howard, J. H. Jr., and Howard, D. V. (2010). One-year retention of general and sequence-specific skills in a probabilistic, serial reaction time task. Memory 18, 427–441. doi: 10.1080/09658211003742680
Romano-Bergstrom, J. C., Howard, J. H. Jr., and Howard, D. V. (2011). Enhanced implicit sequence learning in college−age video game players and musicians. Appl. Cogn. Psychol. 26, 91–96. doi: 10.1002/acp.1800
Saffran, J. R., Newport, E. L., and Aslin, R. N. (1996). Word segmentation: the role of distributional cues. J. Mem. Lang. 35, 606–621. doi: 10.1006/jmla.1996.0032
Salthouse, T. A. (1996). The processing-speed theory of adult age differences in cognition. Psychol. Rev. 103, 403–428. doi: 10.1037/0033-295X.103.3.403
Salthouse, T. A., McGuthry, K. E., and Hambrick, D. Z. (1999). A framework for analyzing and interpreting differential aging patterns: application to three measures of implicit learning. Aging Neuropsychol. Cogn. 6, 1–18. doi: 10.1076/anec.6.1.1.789
Schultz, W. (2007). Behavioral dopamine signals. Trends Neurosci. 30, 203–210. doi: 10.1016/j.tins.2007.03.007
Schwab, J. F., Schuler, K. D., Stillman, C. M., Howard, J. H. Jr. Newport, E. L., and Howard, D. V. (2013). “Aging and the statistical learning of grammatical form classes,” in Association for Psychological Science Conference Meeting (Washington, DC).
Seaman, K. L., Howard, D. V., and Howard, J. H. Jr. (2013). Adult age differences in learning on a sequentially cued prediction task. J. Gerontol. B Psychol. Sci. Soc. Sci. doi: 10.1093/geronb/gbt057. (in press).
Simon, J. R., Howard, J. H. Jr., and Howard, D. V. (2010). Age differences in implicit learning of probabilistic unstructured sequences. J. Gerontol. B Psychol. Sci. Soc. Sci. 66B, 32–38. doi: 10.1093/geronb/gbq066
Simon, J. R., Stollstorff, M., Westbay, L. C., Vaidya, C. J., Howard, J. H. Jr., and Howard, D. V. (2011). Dopamine transporter genotype predicts implicit sequence learning. Behav. Brain Res. 216, 452–457. doi: 10.1016/j.bbr.2010.08.043
Simon, J. R., Vaidya, C. J., Howard, J. H. Jr., and Howard, D. V. (2012). The effects of aging on the neural basis of implicit associative learning in a probabilistic triplets learning task. J. Cogn. Neurosci. 24, 1–13. doi: 10.1162/jocn_a_00116
Song, S., Howard, J. H. Jr., and Howard, D. V. (2007). Implicit probabilistic sequence learning is independent of explicit awareness. Learn. Mem. 14, 167–176. doi: 10.1101/lm.437407
Stillman, C. M., Feldman, H. G., Howard, J. H. Jr., and Howard, D. V. (2012). “Effects of age and structure on the acquisition of probabilistic sequential regularities,” in Cognitive Aging Conference Meeting (Atlanta, GA).
Stillman, C. M., Gordon, E. M., Simon, J. R., Vaidya, C. J., Howard, D. V., and Howard, J. H. Jr. (in press). Caudate resting connectivity predicts implicit probabilistic sequence learning. Brain Connectivity.
Sullivan, E. V., and Pfefferbaum, A. (2007). Neuroradiological characterization of normal adult ageing. Br. J. Radiol. 80 Spec No: S99–S108. doi: 10.1259/bjr/22893432
Keywords: aging, implicit learning, cognition, striatal dysfunction, cognitive neuroscience
Citation: Howard JH Jr. and Howard DV (2013) Aging mind and brain: is implicit learning spared in healthy aging? Front. Psychol. 4:817. doi: 10.3389/fpsyg.2013.00817
Received: 25 July 2013; Accepted: 14 October 2013;
Published online: 07 November 2013.
Edited by:
Vinciane Gaillard, Université Libre de Bruxelles, BelgiumReviewed by:
Rachael D. Seidler, University of Michigan, USAAnna Rieckmann, Karolinska Institute, Sweden
Copyright © 2013 Howard and Howard. This is an open-access article distributed under the terms of the Creative Commons Attribution License (CC BY). The use, distribution or reproduction in other forums is permitted, provided the original author(s) or licensor are credited and that the original publication in this journal is cited, in accordance with accepted academic practice. No use, distribution or reproduction is permitted which does not comply with these terms.
*Correspondence: James H. Howard Jr., Department of Psychology, The Catholic University of America, 620 Michigan Ave NE, District of Columbia, Washington, DC 20064, USA e-mail:aG93YXJkQGN1YS5lZHU=