- 1 Institute of Neurology and Institute of Cognitive Neuroscience, University College London, London, UK
- 2 Department of Experimental Psychology, University of Oxford, Oxford, UK
- 3 Department of Psychology, Royal Holloway, University of London, Egham, UK
- 4 Centre for Functionally Integrative Neuroscience, University of Aarhus, Aarhus, Denmark
Impulsivity is a feature of many brain disorders. Although often defined as the predisposition to act with an inadequate degree of deliberation, forethought, or control, it has proven difficult to measure. This may in part be due to the fact that it is a multifaceted construct, with impulsive decisions potentially arising as a result of a number of underlying mechanisms. Indeed, a “functional” degree of impulsivity may even promote effective behavior in healthy participants in a way that can be advantageous under certain circumstances. Although many tasks have been developed to study impulsivity, few examine decisions made rapidly, for time-sensitive rewards. In the current study we examine behavior in 59 adults on a manual “Traffic Light” task which requires participants to take risks under time pressure, if they are to maximize reward. We show that behavioral variables that index rapid anticipatory responding in this paradigm are correlated with one, specific self-report measure of impulsivity: “lack of premeditation” on the UPPS Impulsive Behavior Scale. Participants who scored more highly on this subscale performed better on the task. Moreover, anticipatory behavior reduced significantly with age (18–79 years), an effect that continued to be upheld after correction for potential age differences in the ability to judge the timing of responses. Based on these findings, we argue that the Traffic Light task provides a parametric method to study one aspect of impulsivity in health and disease: namely, rapid decision-making in pursuit of risky, time-sensitive rewards.
Introduction
Impulsivity has been defined as the predisposition to act with a low or inadequate degree of deliberation, forethought, or control (Moeller et al., 2001). It is clear, however, that the term means different things to different researchers. Operationally, impulsivity is considered to be a multidimensional construct, with impulsive behavior potentially arising from several different mechanisms, underpinned by distinct neural, and cognitive systems (Evenden, 1999; Gray, 2000; Whiteside and Lynam, 2001; Dalley et al., 2011).
An established self-report instrument, the UPPS Impulsive Behavior Scale (Whiteside and Lynam, 2001), for example, distinguishes four cognitive dimensions that predispose to impulsive acts: (1) lack of premeditation or a tendency to act without deliberation; (2) lack of perseverance or inability to complete dull or difficult tasks; (3) urgency or acting rashly whilst upset; and (4) sensation-seeking or need for novelty and excitement. These four factors relate differentially to distinct psychiatric disorders characterized by impulsive behavior (Whiteside et al., 2005; Miller et al., 2009), as well as to the risk of engaging in impulsive acts (Klonsky and May, 2010).
Although impulsivity is often used to refer to a dysfunctional state, it has been argued that an impulsive style of responding does not always have negative consequences. Indeed, if the task demands are relatively simple but participants are under time pressure, people classified as being highly impulsive can sometimes perform better than those with low trait impulsivity (Dickman and Meyer, 1988). Such observations led Dickman to propose that there might be two general classes of impulsivity: “dysfunctional” and “functional” (Dickman, 1990). The former is considered to be associated with classical views of impulsivity: lack of forethought when deliberation would be better. By contrast, functional impulsivity refers to the tendency to act rapidly with little forethought when this style of responding might be optimal.
Dickman showed these two tendencies are not correlated across individuals, and they have different cognitive correlates. Thus, while high “functionally impulsive” individuals were faster and more accurate to complete a rapid task requiring basic perceptual judgments compared to low “functionally impulsive” individuals, there was no such distinction between individuals with high and low dysfunctional traits (Dickman, 1990). Thus “functional impulsivity” can be advantageous under certain circumstances. Conversely, recent experimental data has revealed that “dysfunctional impulsivity” correlates (inversely) with the ability to inhibit actions indexed by the STOP signal reaction time task, but “functional impulsivity” measures do not (Colzato et al., 2010). These considerations might be important for the development of new experimental tools to measure impulsive traits.
In addition to self-report instruments such as the UPPS (Whiteside and Lynam, 2001) or the longer-established Barratt Impulsiveness Scale (BIS-11; Patton et al., 1995), several experimental cognitive tasks have been used to measure impulsive decision-making. These include delay discounting (Kirby and Herrnstein, 1995; Pine et al., 2010), probabilistic gambling (Bechara et al., 1994), and information sampling tasks (Clark et al., 2006). However, in none of these tasks are participants compelled to respond rapidly. In fact, on such tasks swift “impulsive” decisions are always suboptimal.
The only widely used task that does require rapid responses is the Stop Signal task (Logan et al., 1997), deployed to measure inhibitory control. In that paradigm, responses need to be made rapidly in response to an imperative cue. In real life, however, decisions are sometimes made quickly in the absence of a direct, external signal instructing us to do so. For example, when cycling in a highly congested city, negotiating between traffic and pedestrians, we often have to make rapid decisions without overtly being instructed to do so. Similarly, in many modern gaming environments, people do not have time to ponder over the possible choices.
Under such circumstances prolonged, careful deliberation might seem to be “safe and sure” but it can also be detrimental to the overall result, or lead to missed opportunities. Therefore, to address these issues, we developed a manual version of a “Traffic Light” paradigm that measures rapid decision-making in pursuit of time-sensitive, risky rewards. Our aim was to produce a task that captures the range of functional impulsivity in healthy people and is also capable of tracking its change with aging, as well as in pathological states.
On each trial in the Traffic Light paradigm, participants view a red light that successively turns amber, then green. Participants are instructed to respond rapidly following the onset of the green “go” signal, in order to obtain reward; responding prior to green incurs a small, fixed penalty. Reward value declines precipitously with increasing reaction time from green onset, so participants should aim to make responses with the minimum possible latency following green onset. However, the duration of amber is variable so the temporal onset of green cannot be confidently predicted. Moreover, because human sensorimotor decision-making is subject to substantial delays (simple motor decisions take around 200 ms to initiate in response to a go signal; Reddi and Carpenter, 2000; Taylor et al., 2006), participants cannot attain the highest rewards if they merely wait, and respond reactively to green onset.
Instead, as time unfolds during the amber foreperiod, participants must decide whether to continue to wait, or to take a risk and initiate in advance an anticipatory response. Such “risky” decisions can lead to responses being executed just prior to the green light (in which case they will be penalized) or just after it (in which case they will be highly rewarded). Thus, to do well in this paradigm, participants have to demonstrate a degree of “functional impulsivity” and be prepared to make some risky, anticipatory responses.
We evaluated evidence for impulsive, opportunistic responding in our paradigm by measuring the frequency of penalized trials (responses made prior to green onset) and the overall reward obtained, and we also applied a probabilistic model to characterize decision-making behavior. Using these variables we were able to investigate whether there is a relationship between anticipatory behavior in the Traffic Light task and self-reported impulsivity, as well as changes with aging since healthy aging is associated with reduced risk-taking and increased cautiousness (Deakin et al., 2004; Dohmen et al., 2005).
It could be argued that good performance on this task relates simply to how well people judge time. Successful early, anticipatory responses before the green light might rely solely upon the ability to perceive the passage of time, and to judge whether the current duration of amber is longer, or shorter than average. Therefore, we also administered a control task which measures time judgment, or more formally temporal duration discrimination (Bueti et al., 2008). We predicted that performance on this task would contribute to, but not entirely account for individual differences in Traffic Light behavior. Finally, we administered Raven’s Advanced Progressive Matrices (APM) and a demographical questionnaire as control measures, to evaluate the extent to which younger and older participants in our cross-sectional sample could be said to arise from similar underlying populations.
Materials and Methods
Participants
The sample consisted of 59 participants (31 female) aged 18.5–79.1 years (mean 34.2 years, SD 15.0) recruited from the local participant database. Detailed participant information is available on request. Participants gave informed consent according to the Declaration of Helsinki. The study was approved by the local ethics committee.
Measures
Participants completed the following measures:
• Traffic Light task.
• Temporal duration discrimination task.
• UPPS Impulsive Behavior Scale (Whiteside and Lynam, 2001).
• BIS-11 (Patton et al., 1995).
• Raven’s 12-item APM (Raven, 2000).
• Demographical, lifestyle, and psychiatric questionnaire.
The protocol was as follows. Participants completed a practice task, followed by three blocks of the Traffic Light task, the BIS-11, three additional blocks of the Traffic Light task, the UPPS, temporal duration discrimination task, Raven’s 12-APM and the demographical questionnaire. The duration of testing was 90 min per participant. A small number of participants did not complete the full battery. Participants were paid £ per hour for their time.
Individual measures, and their respective methods of analysis, are described in detail below. We identified outliers >2.5 SD from the mean of each measure. One participant was an outlier on multiple measures (time bias, self-reported impulsivity and measures of response time), and therefore we excluded this dataset in its entirety. After this dataset was excluded, the following individually outlying data points were excluded: one high and one low outlier on time bias/bias magnitude, one high outlier on a measure of post-error slowing and one low outlier on BIS total score. For linear analyses of age we conservatively excluded two high outliers on age. Statistical significance was p < 0.05 unless otherwise specified.
Traffic Light task
The Traffic Light task assesses rapid, opportunistic decision-making in pursuit of risky, time-sensitive rewards. Participants decide when to execute a response based on when they expect the analog of a traffic light to change from amber to green.
On each trial participants view a red light which turns amber, then green (Figure 1). The duration of the amber traffic light is drawn at random on each trial from a Gaussian distribution with mean 750 ms and SD 125 ms, and is therefore (boundedly) unpredictable. However, reward value per trial declines very steeply with increasing reaction time (RT) from green onset, whereas responses occurring prior to green incur only a small, fixed penalty. Participants must decide whether to prepare in advance an anticipatory response, based on when they expect the green light to appear (which could result in a high reward, but risks a penalty), or to wait for the green light and then respond reactively (resulting in a small, certain reward).
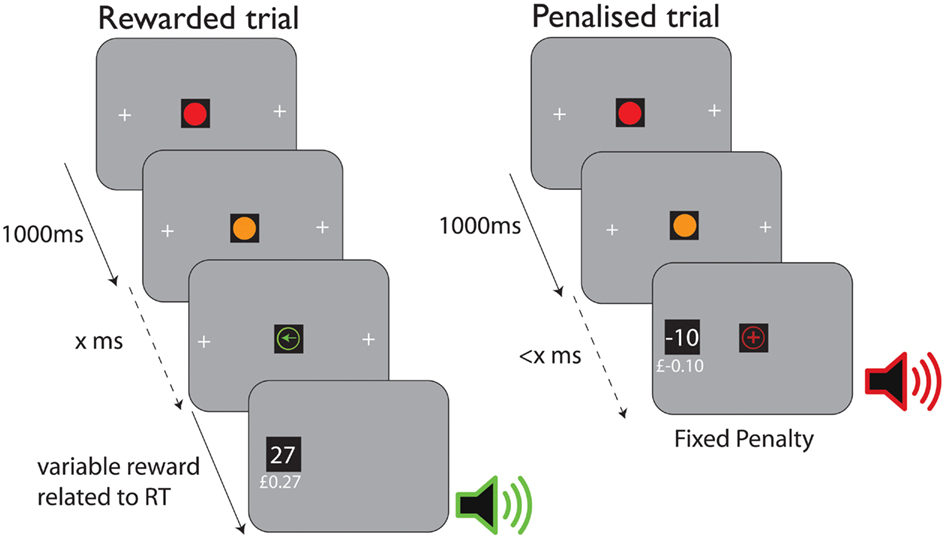
Figure 1. Traffic Light Task. On each trial a traffic light signal turns successively red, then amber, then green. Amber duration (x ms) varies randomly, whereas reward per trial is a function of how rapidly a response is made in relation to the onset of green (RT ms). Responses made subsequent to the onset of green onset are rewarded (left stream) whereas responses made prior to the onset of the green light are penalized (right stream).
Participants are rewarded with hypothetical money for making a manual response to a white target cross to the left or right of the screen, using a Cedrus RB-830 response pad (Cedrus Corporation, 2010). The target alternates predictably from left to right across trials, as indicated by the green “go” arrow. Responses to the leftward target are made with the index finger of the left hand, and responses to the rightward target are made with the index finger of the right hand. RT data are used to provide real-time reward feedback on each trial.
Reward per trial is calculated according to an inverse exponential temporal discount function: for a reaction time greater than zero ms, with respect to green onset
where R is reward value per trial (pence) and RT is reaction time with respect to the onset of green. Thus, disproportionately high rewards are obtained for extremely rapid responses: A RT of 300 ms generates £0.075, 200 ms yields £0.20, 100 ms yields £0.55, and 0 ms results in £1.50. Responses occurring prior to green onset are subject to a fixed penalty of £0.10 (10 p).
Feedback is given both visually and aurally. On each trial, participants are shown the amount they have won on the current trial, as well as a running total. Trial reward values between £0.01 and 0.20 are accompanied by a “ping” sound whereas trial reward values greater than £0.20 are accompanied by a more rewarding “ker-ching” cash-register sound. Penalized early responses are accompanied by low-pitched beep sound. Prior to performing the task, participants were given full and veridical instructions via a standardized demo.
Each block of the Traffic Light task consists of 50 trials. In order to maintain motivation, participants are encouraged to pay attention to their total winnings in each block, and on the next block to try and “beat their record.”
Analysis of traffic light data. For each participant we calculated the percentage of penalized trials, mean reward per block and mean reward per rewarded trial. These were interrogated for hypothesized effects of self-reported impulsivity, age and temporal duration discrimination, using regression and partial correlation as appropriate. For effects across blocks, which could reflect learning, we used repeated measures ANOVA (within-subjects factor: block), entering self-reported impulsivity, age, and temporal duration discrimination variables as covariates.
Next, we applied a two-horse linear rise-to-threshold model to RT distributions from the Traffic Light task (Adam et al., 2011). This method probabilistically assigns trial RTs to populations elicited from an anticipatory vs. reactive decision process (Reddi and Carpenter, 2000). In our model, these processes compete in a “two-horse race” toward the threshold for triggering a response (see Figure 2). In this model, Traffic Light response distributions are described as the product of two linear rise-to-threshold decision processes which “race” to trigger a response. The first, anticipatory decision process is evoked prior to green onset, and rises slowly to threshold from amber onset (with mean rate of rise μa and SD σa). The second, reactive decision process is triggered by the onset of green, and rises sharply to response initiation threshold (with mean rate of rise μr and SD σr).
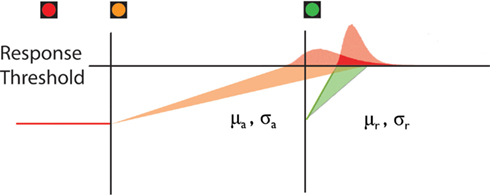
Figure 2. Two-horse linear rise-to-threshold model of response time data in the Traffic Light task. Group and individual response time data were fit to a two-horse linear rise-to-threshold model. Response time distributions arise probabilistically from one of two linear rise-to-threshold processes, and the first process to reach the threshold (horizontal line) on a given trial will trigger a response: (1) An anticipatory decision process, triggered by the onset of the amber Traffic Light, with mean rate of rise μa and SD rate of rise σa. This process has a small mean rate of rise and large variance, and therefore results in a wide distribution of RTs, of which some are elicited prior to green. (2) A reactive decision process, triggered by the onset of the green Traffic Light, with mean rate of rise μr and SD rate of rise σr. This process has a high mean rate of rise and small variance, and therefore results in a narrow distribution of RTs with median shortly after green onset.
To implement our model, we assumed that the distribution of anticipatory responses (amber distribution, Figure 2) could be described as the product of a rise-to-threshold process triggered by amber onset (at time t = 0), and that the distribution of reactive responses (green distribution, Figure 2) could be described as the product of a rise-to-threshold process triggered by green onset (at time t0, on average 750 ms after amber onset). Hence, the probability that a response had occurred by time t following amber onset (i.e., the cumulative probability distribution) was given by:
ΨA and ΨR indicate cumulative recinormal distributions describing anticipatory and reactive processes, respectively (Reddi and Carpenter, 2000). The mean μ and variance σ2 of each distribution can be interpreted as describing how rapidly and variably, on average, each of the two decision processes rises toward the threshold for initiating a response, and the multiplicative inverse of the mean rate of rise is equal to the median of the resultant anticipatory or reactive RT distribution. We assumed constant response initiation threshold (horizontal line, Figure 2) and fit the four parameters to individual participant RT distributions using maximum likelihood estimation (i.e., fminsearch in Matlab).
Model parameters were interrogated for hypothesized effects of self-reported impulsivity, age, and temporal duration discrimination1. Of central interest was whether there is a relationship between anticipatory behavior in the Traffic Light task, and self-reported impulsivity. We predicted an association between Traffic Light anticipation variables (penalized trials; anticipatory rate of rise μa) and lack of premeditation subscale on the UPPS, since this subscale encapsulates the tendency to act rapidly, without deliberation.
Furthermore, we reasoned that if anticipatory Traffic Light behavior reflects “functional impulsivity” (Dickman, 1990), then self-reported impulsivity in healthy controls should be associated with higher mean reward and with well-judged anticipations (and higher mean reward), rather than simple pre-emptiveness or “jumping the gun.” We investigated whether the relationship between the frequency of penalized trials and self-reported impulsivity was more pronounced on penalized trials for which amber duration was longer (≥750 ms) vs. shorter (<750 ms) than average. A task-optimal, opportunistic strategy should entail selective anticipation only on longer than average amber durations.
We reasoned that the degree to which participants adapt their responses following a penalty might yield a partially independent measure of their level of cautiousness. Post-error/penalty slowing is often used as a measure of such adaptive control (Botvinick et al., 2001). The magnitude of post-penalty slowing was interrogated for effects of self-reported impulsivity, age, and overall task performance (reward).
Finally, we were interested to examine the effects of age on behavior. Healthy aging is associated with reduced risk-taking, and an increase in cautiousness (Deakin et al., 2004; Dohmen et al., 2005). Therefore, if the Traffic Light task indexes impulsive, opportunistic responding, anticipatory behavior (penalized responses, anticipatory rate of rise μa) would be hypothesized to reduce with age. However, if the effects of age on Traffic Light performance are simply due to cognitive or sensorimotor slowing, we should instead observe a relationship with reactive model parameters (reactive rate of rise μr). Since model parameters were not Normally distributed across participants, we used Kendall’s tau.
Temporal duration discrimination task
It is important to investigate to what extent performance on the Traffic Light task relates simply to the discrimination of time durations. We used a two-alternative forced choice temporal duration discrimination task adapted from Bueti et al. (2008), task B. Time durations were selected to assess sensitivity to trial-to-trial differences in amber duration that occur within the Traffic Light task. This enabled us to control for the potential impact of age and individual differences in time perception on the frequency of fast, “anticipatory” Traffic Light responses.
Stimuli were green circles presented sequentially on a gray screen. Each trial required a judgment as to which of the two circles was presented for longer. On each trial, one of the two circle durations was 750 ms (the “standard” duration), equivalent to the mean duration of amber on the Traffic Light task. The other circle duration was a “comparison” duration drawn from a set of eight durations, four of which were longer and four shorter than the standard. The difference between standard and comparison durations on each trial was ±50, ±100, ±150, or ±300 ms. That is, four out of eight comparison durations differed from the standard duration by <1 SD amber, and the other four differed by >1 SD amber.
The order of standard/comparison duration was fully randomized across trials, and the eight comparison durations were randomly ordered between trials. Participants used the index and middle fingers of the dominant hand on a computer keyboard to indicate whether the first or second duration was longer. Feedback was provided on each trial. Participants completed four continuous blocks of the task consisting of 48 trials per block.
Raw data from the temporal duration discrimination task consisted of the frequency of standard > comparison perceptual decisions at each standard minus comparison interval (psychometric curves). Individual participant psychometric curves were fitted to a cumulative Gaussian function with parameters bias, b, and variance, σ2 using standard psychophysical procedures implemented in Matlab, and used to calculate the maximum slope of the psychometric function. We used the slope and the mean perceptual bias magnitude in group summary and inferential statistics (i.e., correlation between slope/bias and Traffic Light reward, penalties and μa).
UPPS, BIS-11, Raven’s 12-APM, and demographical questionnaire
Two established self-report measures of impulsivity, the UPPS Impulsive Behavior Scale (Whiteside and Lynam, 2001) and the Barratt Impulsiveness Scale (BIS-11; Patton et al., 1995), were administered. We hypothesized that the lack of premeditation subscale of the UPPS would predict anticipatory behavior on the Traffic Light task (penalized responses, modeled anticipatory rate of rise μa).
All BIS-11 subscales load onto UPPS lack of premeditation, with the strongest factor loading for BIS-11 motor impulsivity (Whiteside and Lynam, 2001). Therefore, to explore convergent validity, we investigated the relationship between Traffic Light anticipation variables and BIS-11 motor impulsivity (Whiteside and Lynam, 2001).
Raven’s 12-APM and the demographical questionnaire were used to evaluate the extent to which younger and older participants in our cross-sectional sample could be said to arise from similar underlying populations. Thus, we checked whether the correlation between age and Raven score in our sample was comparable with correlation coefficients reported in previous studies (e.g., Babcock, 1994, r ≈ 0.4); age effects on demographic variables were investigated to gauge deviance from typical lifespan and population trends. We checked for any differences in employment, computer use, and psychiatric illness which could limit generalizability of our findings. The results were satisfactory. Data are available on request.
Results
Traffic Light Performance
Performance varied considerably between individuals (see Table 1). Overall, 14.3% of trials were penalized early trials (in which responses occurred prior to green onset) but the range across individuals varied widely from 0 to 34.6%. Similarly, overall group mean reward per block was £11.58, but the range was large, varying between £3.32 and £16.84. The corresponding group mean reward per rewarded trial was 28 p, with range 7–47 p. This variability is important because we wanted to develop a task that has a wide dynamic range across the population.
A second key motivation for developing this paradigm was to produce a task in which making a decision to go early could be functionally advantageous, even though it might sometimes lead to penalties. By contrast, simply waiting for a cue to respond (green light) would be “safe” (incurring no penalties) but lead to small cumulative earnings overall. Importantly, we found that participants who incurred more penalties on the Traffic Light task accumulated higher mean reward ( p < 0.001). Thus, making early responses was functionally advantageous on this task.
Effects across blocks, which could reflect learning, were investigated using repeated measures ANOVA. These are discussed in full in a separate Section “Learning Effects.”
Modeling Performance in Terms of Anticipatory and Reactive Processes
We first fit our double recinormal model (Figure 2) to pooled group Traffic Light RT data (Figure 3). This suggested RTs followed a bimodal distribution, and were satisfactorily described as resulting from the combination of two decision processes:
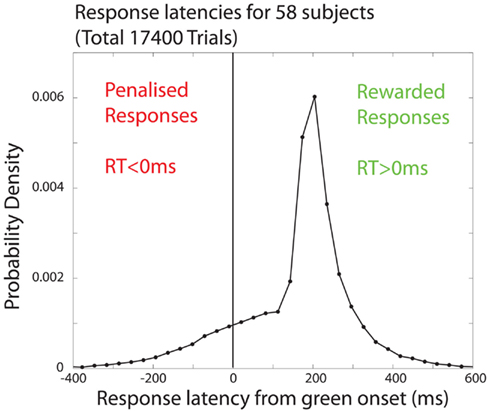
Figure 3. Response time distribution in the Traffic Light task (N = 58). RT data, pooled across participants, shown with respect to green onset at t = 0.
1. An anticipatory linear rise-to-threshold decision process triggered by amber light onset (with mean rate of rise μa 0.725 “decision signal” units per second and SD σa 0.618 s−1)
2. A reactive linear rise-to-threshold decision process triggered by green light onset (with mean rate of rise μr of 4.52 “decision signal” units per second and SD σr 1.27 s−1).
The multiplicative inverse of the mean rate of rise is equal to the median of the resultant recinormal RT distribution:
• for the anticipatory decision process, this was 1380 ms following amber onset, and
• for the reactive decision process, this was 221 ms following green onset (corresponding well to previous estimates of simple reactive RT; Reddi and Carpenter, 2000; Taylor et al., 2006).
The model reproduced the sensitivity of RT to the duration of amber (Figure 4, compare left data panels with right model results). Thus with increasing durations of amber, a greater number of “anticipatory” responses are elicited, leading to an increased likelihood of penalized, as well as highly rewarded trials, depending on the duration of amber.
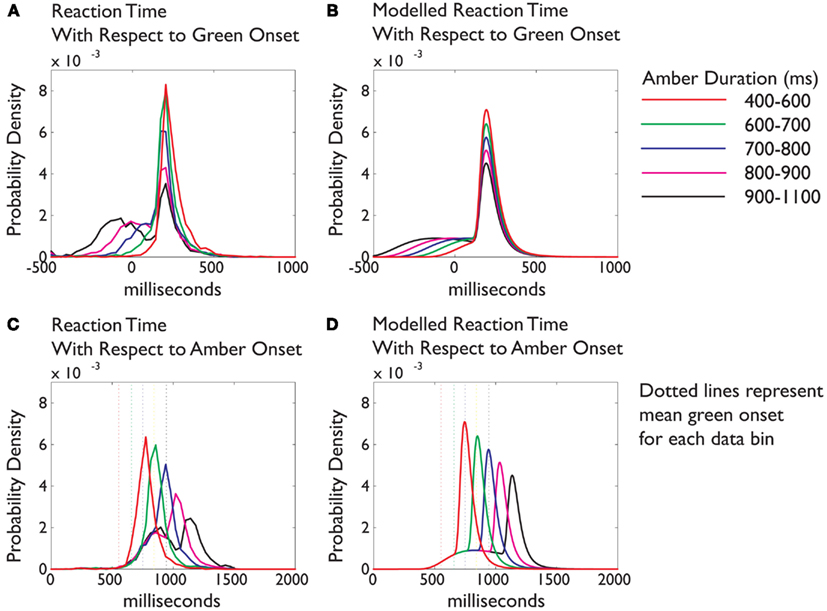
Figure 4. Observed and modeled response time distributions partitioned by amber duration. Response time data (top and bottom left) pooled across participants and partitioned by amber duration, shown with respect to green onset (top) and amber onset (bottom). The proportion of anticipations increases as amber duration increases. Importantly, our model (top and bottom right) reproduces the observed increase in anticipatory responses with increasing durations of amber: Penalized early responses tend to be elicited on trials for which amber duration is longer than average.
For hypothesis testing, we next fitted our model to individual participant RT distributions (see Table 1). The anticipatory rate of rise varied substantially between individuals (see range values in Table 1), exerting a minimal contribution to task behavior in some participants and a substantial contribution in others. This finding mirrors the fact that across individuals there was wide variation in the propensity to make anticipations. By contrast, the reactive decision process was relatively less variable both within and between participants.
Importantly, likelihood ratio tests showed that the two-horse linear rise-to-threshold model fit single-participant data better than a one-horse model with a single “reactive” cumulative recinormal distribution in all 43 datasets for which a one-horse solution could be found (minimum χ2 value 16.6, p < 0.001).
Relationship to Self-reported Impulsivity
If anticipatory Traffic Light behavior reflects “functional impulsivity” (Dickman, 1990), then self-reported impulsivity should be associated with well-judged anticipations (and higher mean reward), rather than simple pre-emptiveness or “jumping the gun.” Our analysis revealed significant relationships between scores on one sub-section of the UPPS and two variables on the Traffic Light task that can be considered to index anticipation. Specifically, there were significant correlations between UPPS lack of premeditation scores and:
• penalized trials ( p = 0.003).
• mean anticipatory rate of rise, μa (τ = 0.264, p = 0.010).
Moreover, there were also significant correlations between UPPS lack of premeditation scores and:
• mean reward per block ( p = 0.013).
• mean reward per rewarded trial ( p = 0.003).
Thus, not only did UPPS lack of premeditation scores predict anticipatory behavior, they also predicted overall outcome. The more impulsive an individual was on this subscale of the UPPS, the more functionally advantageous they were on the Traffic Light task. Importantly, mean rate of rise, μr of the modeled reactive decision process showed no association with UPPS lack of premeditation (p > 0.4). Furthermore, these correlations were not observed for the remaining UPPS subscales (p > 0.1 for all).
To evaluate convergent validity, we also examined the pattern of association between BIS-11 motor impulsivity and the aforementioned Traffic Light variables (penalized trials, reward, μa). This showed a similar pattern: BIS-11 motor impulsivity was significantly associated with penalties ( p = 0.003), mean reward per block ( p = 0.014), mean reward per rewarded trial ( p = 0.003), and anticipatory mean rate of rise μa (τ = 0.262, p = 0.006). Again, there was no association with reactive mean rate of riseμr (p > 0.6).
Next, we investigated whether the relationship between the frequency of penalized trials and self-reported impulsivity was more pronounced on penalized trials for which amber duration was longer (≥750 ms) vs. shorter (<750 ms) than average. We predicted that a task-optimal, opportunistic strategy should entail selective anticipation only on longer than average amber durations. This prediction was upheld: UPPS lack of premeditation predicted the percentage of penalties incurred on long amber durations ( p < 0.001) but not on short amber durations (p > 0.05) on the Traffic Light task. Similarly, BIS-11 motor impulsivity predicted the percentage of penalties incurred on long ( p < 0.001) but not short amber durations (p > 0.1). These results suggest that self-reported non-premeditative impulsivity is significantly associated with the tendency to commit “functionally impulsive” anticipatory responses on the Traffic Light task.
Post-Penalty Slowing
The degree to which people adapt their responses following an error or penalty is often used as an index of adaptive control. Here, we calculated this in two ways:
• difference in RT between post-penalty trials and all other trials, and
• difference in RT between post-penalty trials and all other trials except penalty trials (since penalized RTs might be faster than average).
Participants were significantly slower in the first trial following a penalty, calculated according to both methods (mean difference 86.6 ms, SD 78.4 ms, t-test: t56 = 8.33, p < 0.001 for first method; 60.9 ms, SD 79.8 ms, t-test: t56 = 5.75, p < 0.001 for second method).
However, post-penalty slowing did not assist task performance, since the degree of post-penalty slowing was negatively correlated with mean reward per block (ρ = −0.422, p = 0.001 for first method; ρ = −0.324, p = 0.014 for second method), and mean reward per rewarded trial (ρ = −0.468, p < 0.001 for first method; ρ = −0.362, p = 0.006 for second method). Thus the more sensitive an individual was to a penalty by slowing down on the next trial, the less overall reward they obtained.
We hypothesized that the degree to which participants adapt their responses following a penalty might index performance monitoring and the level of deliberation or control. Indeed, post-penalty slowing was significantly negatively correlated with UPPS lack of premeditation ( p = 0.003 for first method; p = 0.017 for second method). The extent of post-penalty slowing was substantially lower (and in some cases absent) in participants scoring highly on UPPS lack of premeditation. Indeed, a greater degree of post-penalty slowing, which characterizes more cautious, deliberative participants, is not functionally adaptive to the task.
Effect of Age on Model Parameters
We hypothesized that if the Traffic Light task indexes impulsive, opportunistic responding, then anticipatory responding should reduce with age, because aging is associated with reduced risk-taking, and increased cautiousness (Deakin et al., 2004; Dohmen et al., 2005). By contrast, if the effects of age are simply due to cognitive or sensorimotor slowing, we should instead observe a relationship with reactive model parameters (reactive rate of rise μr).
Our analysis revealed that the anticipatory rate of rise μa was significantly negatively associated with age (τ = −0.257, p = 0.005), whereas μr was not associated with age (p > 0.1). Moreover, the percentage of penalized trials showed a near-significant negative correlation with age (ρ = −0.263, p = 0.050). In addition, mean reward per block correlated negatively with age (ρ = −0.338, p = 0.011), as did mean reward per rewarded trial (ρ = −0.323, p = 0.015). Thus functionally useful responding declined with age. Participant age was unrelated to UPPS lack of premeditation (p > 0.7). The observed relationship between the mean rate of rise, μa of the modeled anticipatory decision process and UPPS lack of premeditation remained significant after partialing out age (τ = 0.379, p = 0.010).
Contribution of Time Duration Discrimination
It was important to quantify the extent to which anticipatory behavior on the Traffic Light task is related simply to differences in time duration discrimination. We found that variance in the slope of the temporal duration discrimination stimulus-response function (see Table 1) predicted a small proportion of variance in Traffic Light mean reward per block ( p = 0.037; adding mean bias magnitude to the model did not increase predictive power), but there was no significant relationship to modeled anticipatory rate of rise (μa; p > 0.6) or frequency of penalized early trials (p > 0.9).
This pattern of results is consistent with the view that temporal duration discrimination ability contributes to, but does not entirely account for successful Traffic Light task performance. We would argue that an additional variable, such as individually varying impulsivity or motivation, contributes to anticipatory responses in the task.
There was no relationship between the slope of the temporal duration discrimination stimulus-response function and either participant age (p > 0.3), or any self-report impulsivity subscale (all p > 0.09). Thus, although performance on the Traffic Light task is sensitive to changes across age and self-reported impulsivity, this cannot be accounted for by an effect mediated via discriminant sensitivity to short durations of time.
Learning Effects
We investigated effects across blocks, which could reflect learning, using repeated measures ANOVA (within-subjects factor: five blocks since a large minority (18%) of participants completed 5 blocks) with UPPS lack of premeditation, age, and temporal duration discrimination slope as covariates.
Although in numerical terms performance improved across blocks, the effect was not significant: either for mean reward per block, mean reward per rewarded trial or percentage penalized trials (p > 0.1 for all). However, there was an interaction between age and mean reward per block across blocks (F4,176 = 2.602, p = 0.038). Contrasts revealed the effect was quadratic (F1,44 = 2.634, p = 0.031; see Figure 5).
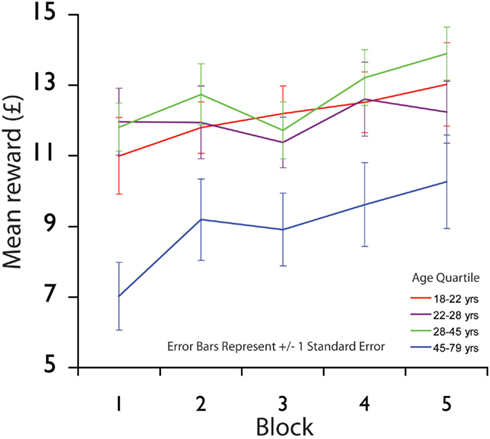
Figure 5. Mean reward per block across blocks. Mean reward per block across blocks, shown by age quartiles in order to graphically illustrate the quadratic effect of age and block number on mean reward per block. In numerical terms performance improved across blocks, although the effect was not significant. Older participants showed steeper improvement in performance than younger participants during early blocks.
There was no evidence for an interaction between performance across blocks and either UPPS lack of premeditation (for mean reward per block, mean reward per rewarded trial, or simply number of penalized trials: p > 0.1 for all) or temporal duration discrimination slope (p > 0.1 for all). Therefore, the effect of UPPS lack of premeditation reflects stable individual differences in strategy present from the outset, i.e., willingness to take a risk and anticipate green onset.
Discussion
The current study investigated behavior in a manual “Traffic Light” task which requires participants to take risks under time pressure, if they are to maximize reward. We argue that our task captures “functional impulsivity,” which is adaptive within the current environment and balances the benefits of careful premeditation with those conferred by rapid, opportunistic responding (Dickman, 1990). Participants who responded in a more anticipatory manner, and committed more penalties, also accumulated higher mean reward. Anticipatory behavior on the Traffic Light task was related to a specific subscale on the UPPS Impulsive Behavior Scale subscale labeled “lack of premeditation.” Participants with high “lack of premeditation” scores behaved in a more anticipatory manner, and accumulated higher mean reward.
Anticipatory Behavior on the Traffic Light Task
In the Traffic Light paradigm, participants respond rapidly following onset of a green “go” signal, in order to obtain reward (Figure 1). Reward value declines precipitously with response speed from green onset, whereas responding during the preceding amber signal incurs a small, fixed penalty. Since the duration of amber is variable, the onset of the green “go” signal cannot be confidently predicted. Moreover, since sensorimotor and decision delays are substantial, participants cannot attain the highest rewards if they merely wait, and respond reactively to green onset. Instead, participants should choose to sometimes risk incurring a penalty, and initiate in advance an anticipatory response.
Our descriptive analysis suggested that many participants did indeed adopt an anticipatory strategy on a proportion of trials. This strategy could be labeled “functionally” impulsive (Dickman and Meyer, 1988; Dickman, 1990), since participants who incurred more penalties also accumulated higher reward. Moreover, anticipation increased with increasing amber duration (Figure 4). This suggests participants tailored their degree of anticipation to the current trial environment. On average, participants incurred penalties on 14% trials (Figure 3). For some individuals, this figure was as high as 35%, whereas for others (particularly older participants) it was close to zero. To better understand the mechanisms underlying this variation, we modeled Traffic Light RT distributions as arising probabilistically from two linear rise-to-threshold processes (Adam et al., 2011).
In our model, trial RTs were assigned probabilistically to populations triggered by an anticipatory vs. a reactive decision process, which on each trial compete in a “two-horse race” to response threshold (Figure 2). The early, anticipatory process is triggered before the green light by amber onset, and tends to result in either penalized or highly rewarded responses. The later, reactive process is triggered by green light onset, and tends to result in slower, more modestly rewarded responses. Importantly, our model reproduced the observed increase in anticipatory responding with increasing durations of amber (Figure 4).
Across participants, the modeled reactive decision process had a rapid rate of rise from green onset and small variance, commensurate with the unequivocal status of green onset as a signal for immediate action. The reactive rise-to-threshold process thus corresponded to a relatively narrow RT distribution with median 230 ms after green onset. This value is in broad agreement with RT estimates from previous studies that have studied simple reactive responses to a go signal (Reddi and Carpenter, 2000; Taylor et al., 2006). Importantly, our analyses suggest the reactive decision process is unaffected by individual differences in age and self-reported impulsivity.
This was not the case for the anticipatory decision process. Across participants, the anticipatory decision process had a slow rate of rise from amber onset and large variance, commensurate with the uncertain status of time elapsed during amber as a signal for action. The corresponding amber RT distribution had a large, late median and large variance. As predicted, the anticipatory decision process, and therefore the penalties and rewards to which it contributed, was sensitive to a number of individual difference variables: namely age, and specific dimensions of self-reported impulsivity. We therefore suggest that it is the anticipatory response component specifically that reflects “functional impulsivity” in our task.
Relationship to Self-Reported Lack of Premeditation
We observed an association between Traffic Light anticipation (anticipatory rate of rise; penalties; reward) and UPPS lack of premeditation. This was replicated in the statistically related motor impulsivity subscale of the BIS-11. More highly impulsive individuals (according to self-report) were more likely to make anticipatory decisions, and in consequence they incurred more penalties. Overall, however, these individuals accumulated higher mean reward, since many of their responses occurred very briefly after green onset rather than just before it.
We argue that this tendency reflects a task-optimal, “functionally impulsive” mode of responding. High levels of UPPS lack of premeditation were not associated with a large number of penalties committed indiscriminately across durations of amber, but rather were related to “functional” or well-judged early responses, elicited when amber duration was longer than average. Note that highly non-premeditative individuals (as indexed by UPPS lack of premeditation scores) did not differ on reactive rate of rise (they were not faster to respond to a go signal), and neither did they show superior time perception.
The association between Traffic Light anticipation variables and UPPS lack of premeditation (and its statistical relation on the BIS-11) suggests that the Traffic Light task shows sensitivity to a particular dimension of impulsivity. UPPS lack of premeditation encapsulates the tendency to act rapidly, without careful forethought, and it has been argued that in certain contexts this is adaptive (Dickman, 1990). Many existing behavioral measures of impulsivity favor a careful, deliberative response strategy (Bechara et al., 1994; Kirby and Herrnstein, 1995; Clark et al., 2006), and are therefore not capable of measuring a rapid, functional aspect of impulsivity. One potential exception is the Stop Signal task, which indexes rapid motor responses and their withholding following a stop signal (Chamberlain and Sahakian, 2007; Liddle et al., 2009). Alternatively, it may be the case that UPPS lack of premeditation reflects a general risk-seeking tendency. Comparison of Traffic Light with Iowa Gambling Task performance, and the relationship to self-reported impulsivity, will reveal whether UPPS lack of premeditation predicts rapid, accurately timed responses specifically, or the propensity to take risks in order to accumulate reward more generally. Indeed, if we are to fractionate distinct cognitive contributions to impulsive behavior, it will be important to use a range of experimental measures.
Sensitivity to Penalties on a Trial-to-Trial Basis
Participants were slower to respond in the trial immediately following a penalty. However, post-penalty slowing did not assist performance, since it was negatively correlated with mean reward. Individuals who were less “sensitive” to incurring a penalty – as indexed by demonstrating a lesser degree of post-penalty slowing – tended to accumulate more reward. In addition, the extent of post-penalty slowing was substantially lower (and in some cases absent) in participants scoring highly on UPPS lack of premeditation, again underlying this important association with performance on the task and one aspect of impulsivity.
Effects of Age on Traffic Light anticipation
Previous research has highlighted normative lifespan changes in impulsivity, risk-seeking, and motivation (Deakin et al., 2004; Dohmen et al., 2005; Steinberg et al., 2009; Brodaty et al., 2010). Therefore, to the extent to which our Traffic Light task indexes impulsive, opportunistic responding, we expected to observe a relationship with cross-sectional age. This prediction was upheld. With increasing age, participants committed fewer penalized early responses, showed a slower anticipatory mean rate of rise and accumulated lower mean reward. Importantly, our modeling analysis suggests that this was not due to sensorimotor slowness per se. No effect of age was observed on the reactive decision process (cf. reactive slowing; Cerella, 1985); the effect was specific to the anticipatory decision process. Furthermore, there was no relationship between age and performance on the temporal duration discrimination task. Interpretation of this pattern of findings within an established framework for decision-making (Reddi and Carpenter, 2000) prompts the conclusion that older participants show reduced urgency or motivation to pursue risky, time-sensitive rewards (Ratcliff et al., 2000). This effect may interact with learning effects across blocks (see Learning Effects).
Conclusion
Impulsivity can be defined as a predisposition to act with an inadequate degree of deliberation, forethought or control. However, in certain contexts – such as when an opportunity is available for a limited period of time – a degree of “functional” impulsivity might actually be adaptive (Dickman, 1990). Here, we present data from a Traffic Light paradigm in which it is beneficial to respond in an “impulsive,” anticipatory manner. Participants scoring more highly on a specific dimension of self-reported impulsivity (UPPS lack of premeditation) show more anticipatory behavior and in consequence, accumulate higher reward.
The Traffic Light task could be applied in research investigating functional vs. dysfunctional impulsivity in healthy individuals, including during pharmacological cognitive enhancement (Academy of Medical Sciences, 2008; Husain and Mehta, 2011). Our findings show the utility of the Traffic Light paradigm for dissociating the effects of individual differences in anticipatory responding and certain forms of impulsivity from the effects of reactive slowness per se. As such, our task may be valuable for testing impulsive patients who also show psychomotor slowing (Voon and Dalley, 2011).
Conflict of Interest Statement
The authors declare that the research was conducted in the absence of any commercial or financial relationships that could be construed as a potential conflict of interest.
Acknowledgments
This research was supported by the Wellcome Trust and the NIHR CBRC at UCL/UCLH. The authors are grateful to Dr. Davina Hensman for contributing to data collection.
Footnote
- ^Note that we did not investigate model parameters for effects across the session, for example an increase in anticipatory rate-of-rise between the first and second three blocks, since 150 trials is not sufficient for model fitting.
References
Academy of Medical Sciences. (2008). Brainscience, Addiction and Drugs. Working Group Report Chaired by Professor Sir Gabriel Horn, Foresight Brain Science, Addiction and Drugs Project. London: Office of Science and Technology.
Adam, R., Bays, P. M., and Husain, M. (2011). Rapid decision-making under risk. Cogn. Neurosci. 3, 52–61.
Babcock, R. L. (1994). Analysis of adult age differences on the Raven’s Advanced Progressive Matrices Test. Psychol. Aging 9, 303–314.
Bechara, A., Damasio, A. R., Damasio, H., and Anderson, S. W. (1994). Insensitivity to future consequences following damage to human prefrontal cortex. Cognition 50, 7–15.
Botvinick, M. M., Braver, T. S., Barch, D. M., Carter, C. S., and Cohen, J. D. (2001). Conflict monitoring and cognitive control. Psychol. Rev. 108, 624–652.
Brodaty, H., Altendorf, A., Withall, A., and Sachdev, P. (2010). Do people become more apathetic as they grow older? A longitudinal study in healthy individuals. Int. Psychogeriatr. 22, 426–436.
Bueti, D., Bahrami, B., and Walsh, V. (2008). Sensory and association cortex in time perception. J. Cogn. Neurosci. 20, 1054–1062.
Cedrus Corporation (2010). San Pedro, CA. Available at: http://www.governmentcontractswon.com/department/defense/cedrus-corporation-196339803.asp?yr=10
Chamberlain, S. R., and Sahakian, B. J. (2007). The neuropsychiatry of impulsivity. Curr. Opin. Psychiatry 20, 255–261.
Clark, L., Robbins, T., Ersche, K., and Sahakian, B. (2006). Reflection impulsivity in current and former substance users. Biol. Psychiatry 60, 515–522.
Colzato, L. S., van den Wildenberg, W. P. M., Van der Does, A. J. W., and Hommel, B. (2010). Genetic markers of striatal dopamine predict individual differences in dysfunctional, but not functional impulsivity. Neuroscience 170, 782–788.
Dalley, J. W., Everitt, B. J., and Robbins, T. W. (2011). Impulsivity, compulsivity, and top–down cognitive control. Neuron 69, 680–694.
Deakin, J., Aitken, M., Robbins, T., and Sahakian, B. J. (2004). Risk taking during decision–making in normal volunteers changes with age. J. Int. Neuropsychol. Soc. 10, 590–598.
Dickman, S. J. (1990). Functional and dysfunctional impulsivity: personality and cognitive correlates. J. Pers. Soc. Psychol. 58, 95–102.
Dickman, S. J., and Meyer, D. E. (1988). Impulsivity and speed-accuracy tradeoffs in information processing. J. Pers. Soc. Psychol. 54, 274–290.
Dohmen, T., Falk, A., Huffman, D., Sunde, U., Schupp, J., and Wagner, G. (2005). Individual Risk Attitudes: New Evidence from a Large, Representative, Experimentally-Validated Survey. Available at: http://ideas.repec.org/p/feb/wpaper/2096.html
Husain, M., and Mehta, M. A. (2011). Cognitive enhancement by drugs in health and disease. Trends Cogn. Sci. (Regul. Ed.) 15, 28–36.
Kirby, K. N., and Herrnstein, R. J. (1995). Preference reversals due to myopic discounting of delayed reward. Psychol. Sci. 6, 83–89.
Klonsky, E. D., and May, A. (2010). Rethinking impulsivity in suicide. Suicide Life Threat. Behav. 40, 612–619.
Liddle, E. B., Scerif, G., Hollis, C. P., Batty, M. J., Groom, M. J., Liotti, M., and Liddle, P. F. (2009). Looking before you leap: a theory of motivated control of action. Cognition 112, 141–158.
Logan, G. D., Schachar, R. J., and Tannock, R. (1997). Impulsivity and inhibitory control. Psychol. Sci. 8, 60–64.
Miller, D. J., Derefinko, K. J., Lynam, D. R., Milich, R., and Fillmore, M. T. (2009). Impulsivity and attention deficit-hyperactivity disorder: subtype classification using the UPPS impulsive behavior scale. J. Psychopathol. Behav. Assess. 32, 323–332.
Moeller, F. G., Barratt, E. S., Dougherty, D. M., Schmitz, J. M., and Swann, A. C. (2001). Psychiatric aspects of impulsivity. Am. J. Psychiatry 158, 1783–1793.
Patton, J. H., Stanford, M. S., and Barratt, E. S. (1995). Factor structure of the Barratt impulsiveness scale. J. Clin. Psychol. 51, 768–774.
Pine, A., Shiner, T., Seymour, B., and Dolan, R. J. (2010). Dopamine, time, and impulsivity in humans. J. Neurosci. 30, 8888–8896.
Ratcliff, R., Spieler, D., and McKoon, G. (2000). Explicitly modeling the effects of aging on response time. Psychon. Bull. Rev. 7, 1–25.
Raven, J. (2000). The Raven’s progressive matrices: change and stability over culture and time. Cogn. Psychol. 41, 1–48.
Reddi, B. A. J., and Carpenter, R. H. S. (2000). The influence of urgency on decision time. Nat. Neurosci. 3, 827–830.
Steinberg, L., Graham, S., O’Brien, L., Woolard, J., Cauffman, E., and Banich, M. (2009). Age differences in future orientation and delay discounting. Child Dev. 80, 28–44.
Taylor, M. J., Carpenter, R. H. S., and Anderson, A. J. (2006). A noisy transform predicts saccadic and manual reaction times to changes in contrast. J. Physiol. (Lond.) 573, 741–751.
Voon, V., and Dalley, J. W. (2011). Parkinson disease: impulsive choice– Parkinson disease and dopaminergic therapy. Nat. Rev. Neurol. 7, 541–542.
Whiteside, S. P., and Lynam, D. R. (2001). The five factor model and impulsivity: using a structural model of personality to understand impulsivity. Pers. Individ. Dif. 30, 669–689.
Keywords: impulsivity, decision-making, reward, risk, age, reaction time
Citation: Burnett Heyes S, Adam RJ, Urner M, van der Leer L, Bahrami B, Bays PM and Husain M (2012) Impulsivity and rapid decision-making for reward. Front. Psychology 3:153. doi: 10.3389/fpsyg.2012.00153
Received: 23 February 2012; Accepted: 27 April 2012;
Published online: 22 May 2012.
Edited by:
Andreas B. Eder, University of Wuerzburg, GermanyReviewed by:
Luis Jimenez, University of Santiago de Compostela, SpainAna M. Franco-Watkins, Auburn University, USA
Copyright: © 2012 Burnett Heyes, Adam, Urner, van der Leer, Bahrami, Bays and Husain. This is an open-access article distributed under the terms of the Creative Commons Attribution Non Commercial License, which permits non-commercial use, distribution, and reproduction in other forums, provided the original authors and source are credited.
*Correspondence: Stephanie Burnett Heyes, Department of Experimental Psychology, University of Oxford, South Parks Road, Oxford OX1 3UD, UK. e-mail: burstephanie@gmail.com