- 1 Department of Brain and Cognitive Sciences, Massachusetts Institute of Technology, Cambridge, MA, USA
- 2 Computer Science and Artificial Intelligence Lab, Massachusetts Institute of Technology, Cambridge, MA, USA
According to common wisdom in the field of visual perception, top-down selective attention is required in order to bind features into objects. In this view, even simple tasks, such as distinguishing a rotated T from a rotated L, require selective attention since they require feature binding. Selective attention, in turn, is commonly conceived as involving volition, intention, and at least implicitly, awareness. There is something non-intuitive about the notion that we might need so expensive (and possibly human) a resource as conscious awareness in order to perform so basic a function as perception. In fact, we can carry out complex sensorimotor tasks, seemingly in the near absence of awareness or volitional shifts of attention (“zombie behaviors”). More generally, the tight association between attention and awareness, and the presumed role of attention on perception, is problematic. We propose that under normal viewing conditions, the main processes of feature binding and perception proceed largely independently of top-down selective attention. Recent work suggests that there is a significant loss of information in early stages of visual processing, especially in the periphery. In particular, our texture tiling model (TTM) represents images in terms of a fixed set of “texture” statistics computed over local pooling regions that tile the visual input. We argue that this lossy representation produces the perceptual ambiguities that have previously been as ascribed to a lack of feature binding in the absence of selective attention. At the same time, the TTM representation is sufficiently rich to explain performance in such complex tasks as scene gist recognition, pop-out target search, and navigation. A number of phenomena that have previously been explained in terms of voluntary attention can be explained more parsimoniously with the TTM. In this model, peripheral vision introduces a specific kind of information loss, and the information available to an observer varies greatly depending upon shifts of the point of gaze (which usually occur without awareness). The available information, in turn, provides a key determinant of the visual system’s capabilities and deficiencies. This scheme dissociates basic perceptual operations, such as feature binding, from both top-down attention and conscious awareness.
Introduction
Our senses gather copious amounts of data, seemingly far more than our minds can fully process at once. At any given instant we are consciously aware of only a small fraction of the incoming sensory input. We seem to have a limited capacity for awareness, for memory, and for the number of tasks we can simultaneously perform. For example, our conscious experience when looking at a street scene (e.g., Figure 1C) consists of first noticing, perhaps, a one-way sign, then a pedestrian, then a tree next to the sidewalk. Subjectively, it seems as if we switch our awareness between them. The mechanism behind this experience of shifting the focus of our awareness has been called selective attention. Traditionally, selective attention has been intimately linked with conscious awareness. James (1890) said of attention that “focalization, concentration, of consciousness are of its essence.” However, the precise relationship between consciousness and attention has remained unclear.
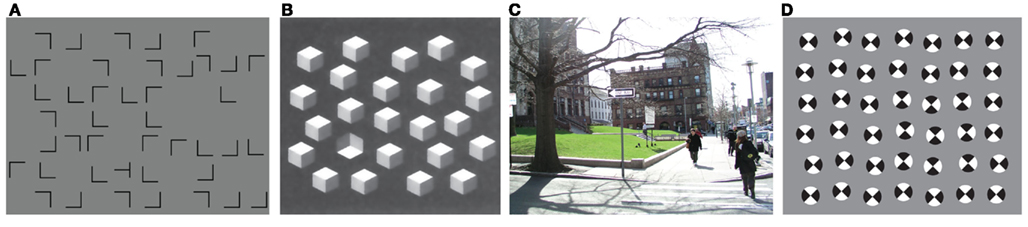
Figure 1. Challenges for a model of vision. (A) Search is sometimes difficult, even when target (T) and distractors (L) are quite discriminable. (B) Yet search is sometimes easy for fairly complex shapes, such as shaded cubes (adapted by permission from Macmillan Publishers Ltd: Nature 379: 165–168, copyright 1996). Furthermore, it is easy to get the gist of a scene (C) or of an array of items (D).
Theories of selective attention vary, but in general it is presumed to operate by alternately selecting one of a number of competing subsets of the incoming sensory data for further processing. Unselected information is momentarily either unavailable, or available only for very limited processing. (Attentional modulation, by contrast, refers to effects of attention on existing visual processing, e.g., attenuation or enhancement of processing, or changes in the tuning or contrast sensitivity of a neuron.) Selective attention can be bottom-up, in which salient items draw attention by virtue of being unusual when compared to nearby items (Rosenholtz, 1999, 2000). Bottom-up selective attention is generally assumed to be largely automatic and independent of task (Wolfe, 2007). Much of selective attention, however, is assumed to be top-down, driven by the tasks and goals of the individual.
This framework raises the question which has occupied much of the study of attention for the last 50 years: at what stage does selective attention operate? In other words, what processing occurs prior to selection – and is available to guide that selection process – and what processing occurs later, operating only on the selected information?
In vision, visual search has proved a rich experimental paradigm for investigating attention (Figures 1A,B). An observer’s task consists of finding a target among competing distractor items. If attentional selection operates late in the processing pipeline, then all items in the display might be processed to the point of identification, but an observer might only be able to concentrate their awareness on one item at a time. Presumably if this were the case, visual search would be easy, so long as the target was visually distinct from the distractors; preattentive identification of the items would direct attention to the target. However, visual search is often quite difficult: it’s surprisingly hard to find a rotated “T” among rotated “L”s, or search for a red vertical bar among red horizontal and green vertical distractors. These results have led to the conclusion that attention operates with early selection, i.e., that top-down selective attention is necessary even for so simple an operation as the binding together of pairs of features into a “T,” “L,” or red vertical bar. This conclusion dates back to Treisman and Gelade (1980). Despite some issues, discussed in the next section, this conclusion continues to pervade our thinking about visual perception.
The intimate relationship between consciousness and attention, coupled with the notion that attention strongly influences perception through early selection, is problematic. For one thing, consider the intertwined nature of consciousness and attention. It would seem straightforward to suggest that selective attention might be required as a gate to awareness (Treisman, 2003). However, an argument could also be made for the converse. “Top-down” implies goal-directed, volitional, and intentional, suggesting that some sort of conscious awareness might be a necessary precursor to top-down selective attention (Itti and Koch, 2001; Cavanagh, 2004). If selective attention, in turn, is required for feature binding, this is cause for concern. Surely so expensive (and possibly human) a resource as conscious awareness is not required for basic low-level perception. In fact, humans can perform many complex tasks, apparently with neither consciousness nor attention, such as driving home on a familiar route. Such “zombie behaviors” (Koch and Crick, 2001) would seem to imply that one can remove awareness and attention without a huge impact on task performance.
If awareness were required for top-down selective attention, could the visual system get around a need for consciousness by primarily processing “salient” regions of the image through bottom-up selective attention, and occasionally applying conscious, top-down selective attention? As Nakayama (1990) has previously argued, this does not seem like a viable strategy.
Dissociating awareness from attention (Nakayama, 1990; Levin and Simons, 1997; Koch and Tsuchiya, 2006; Wyart and Tallon-Baudry, 2008) can help resolve the issue of awareness being improbably coupled to basic perception. The notion of “unconscious inferences” (von Helmholtz, 1867) has certainly been popular in the study of human vision. This theory suggests that the brain might continuously, automatically, and often unconsciously postulate interpretations of the visual world (see Koenderink, 2011, for a recent formulation). Testing those hypothesized interpretations would involve some sort of top-down mechanisms, perhaps driving selective attention without awareness.
However, there are other issues with the awareness–attention–perception triad as well. As we will argue in the Section “Discussion,” early selection may be incompatible with a number of theories of consciousness. Furthermore, the historical link between consciousness and attention may have biased us to think of limited capacity in a particular way, which is incompatible with reasoning about perception. We will argue that this has led to a complicated story about perception in a limited capacity system, where a simpler story will suffice.
We begin by reviewing research on early vs. late attentional selection in vision: the logic behind the experiments and conclusions, as well as issues that complicate the story. Next, we will review recent work on the nature of the lossy representation in early vision. This research attributes significant information loss not to a lack of selective attention, but rather to limitations of peripheral vision. Such a lossy representation predicts difficulties in visual search previously attributed to a lack of top-down selective attention (Rosenholtz et al., under review), including perceptual ambiguities often interpreted as a lack of feature binding. Nonetheless, this representation is sufficiently rich to explain performance in such complex tasks as scene gist recognition, pop-out search, and navigation. The result is a simple, coherent account of much of the evidence used to study selective attention in vision.
First, a bit of terminology: in this paper we use the term selection to refer to the momentary choice of a subset of the sensory input, with the intent later, perhaps, to select a different subset. While, for instance, having only three cone types in the retina might be thought of as representing only a subset of the input, we do not call this “selection,” as there is no plan to later use cones with different responsivity. On the other hand, moving ones’ eyes to direct the highest density of photoreceptors to a particular location should certainly be thought of as involving a form of selection, but we do not refer to this as selective attention. Attention involves separate mechanism(s), a focus that may not agree with the point of fixation, and possibly different effects upon perception than shifting the point of gaze.
Early or Late Attentional Selection in Visual Processing?
The visual system subjects the visual input to stages of processing, from basic feature measurements in early vision, through mid-level grouping, recognition, memory, and higher-level cognition. A number of initial processing stages are assumed to occur preattentively and in parallel across the visual field. At some point in the processing stream, selection occurs. What is selected is determined by the information available from the preattentive processing stages, as well as any task-relevant information such as likely location of a target (see also “Guided Search,” Cave and Wolfe, 1990). In the most straightforward version of the story, the selected information passes through a limited capacity channel to higher processing, e.g., semantic analysis, whereas the unselected information becomes unavailable for further processing and conscious awareness (Broadbent, 1958).
At what stage does attentional selection occur, i.e., what computations can occur without attention? To answer this question, one must first run experiments in which attentional selection is likely to matter, i.e., situations in which the sensory input contains multiple components competing for limited processing resources. For example, dichotic listening experiments simultaneously present two auditory stimuli, such as speech, one to each ear, and ask participants to attend to one or both. Easy tasks presumably use computations that happen before selection, whereas difficult tasks use computations that happen after attentional selection.
In audition, the early vs. late selection story at first seemed straightforward. Listeners can easily distinguish, in the unattended ear, tones from speech, and male from female voices. However, they have difficulty identifying even a single word or phrase presented to the unattended ear, determining whether the language is English or German, and even distinguishing forward speech from reversed (Cherry, 1953). Broadbent (1958) took these results to demonstrate early selection, in which only low-level “physical” characteristics – e.g., the frequency spectrum – of the signal can be processed without attention.
However, a number of empirical findings are at odds with Broadbent’s early selection theory, including the classic demonstration by Moray (1959) that people can recognize subjectively important “messages,” such as their own names, in the unattended stream of conversation. To accommodate these findings, Treisman (1960) proposed attenuation theory, which posits that unattended information, rather than being excluded from further processing, instead has attenuated signal strength. At later stages processing occurs only if the signal falls above some threshold. An important message such as the listener’s name will be semantically processed, in this scheme, because even its attenuated signal strength will often fall above-threshold. By this theory, attentional attenuation happens early. The mere attenuation of unattended information does not obviously resolve issues of limited capacity at this early processing stage, though it does facilitate later selection of above-threshold signals for further processing.
In vision, the dominant experimental paradigm for studying early vs. late selection has been visual search, where the target and distractors are presumed to compete for limited processing resources. As discussed in the Section “Introduction,” initial results in visual search led researchers to conclude that selective attention operated early in visual processing, and to develop the highly influential feature integration theory (FIT, Treisman and Gelade, 1980). FIT suggests that spatially organized “maps” of basic features such as orientation, color, and size, can be preattentively extracted in parallel across the visual scene. However, any further processing requires attention, including the binding together of basic features. This theory predicts that searching for a target defined by a basic feature is efficient, parallel, and does not require attention. However, search for a target defined by conjunction or configuration of basic features requires the serial deployment of selective attention.
Although a number of search results support the early selection story, a number of issues arise; here we focus on only a few of the most critical. For one thing, the level at which attentional selection operates in visual search has seemed inconsistent. Studies have shown that some properties related to extraction of 3-D shape, direction of lighting, and apparent reflectance can be processed in parallel across the visual scene and thus enable easy search (Enns and Rensink, 1990; Sun and Perona, 1996). How could it be that processing of 3-D shape, lighting, and/or reflectance occurs preattentively, but not simple feature binding?
Furthermore, different paradigms have led to different conclusions about what processing occurs preattentively. Search for a scene containing an animal among non-animal scenes (VanRullen et al., 2004) or for a navigable scene among non-navigable scenes (Greene and Wolfe, 2011) seems to require a serial, attentive process. This is not surprising for FIT, since no single basic feature can identify an animal or a navigable path; search for these targets should require feature binding, which requires attention. FIT would also seem compatible with evidence from change-blindness that without attention the details of the scenes are murky (Rensink et al., 1997; Simons and Levin, 1997).
However, a number of studies have shown that natural scenes can be perceived preattentively in a dual-task paradigm. In this paradigm, the observer is given fewer competing sensory inputs than in a typical search display, but must complete both central and peripheral tasks. In this paradigm, observers can perform a peripheral task in which they identify whether a scene contains an animal or not, while simultaneously specifying whether letters presented at the center of the display are all “T”s, all “L”s, or mixed (Li et al., 2002). Furthermore, this result agrees with outcomes of rapid perception experiments. Rapid perception paradigms allow for brief attention to a scene, but minimal time to select multiple regions of that scene for further processing. Yet observers can discern much information about the gist of a scene, sufficient to identify general scene categories and properties (Rousselet et al., 2005; Greene and Oliva, 2009) and perform basic object detection, for example determining whether an image contains an animal (Thorpe et al., 1996; Kirchner and Thorpe, 2006), vehicle (VanRullen and Thorpe, 2001), or human face (Crouzet et al., 2010). A brief (26 ms) glance at a scene is also sufficient to allow observers to distinguish specific types of animals (birds or dogs) from other animal distractors (Mace et al., 2009). How is this possible with minimal attention, when attention seems necessary to tell a “T” from an “L”?
The dual-task paradigm has also provided conflicting results for preattentive processing of 3-D shape. As mentioned above, findings from visual search have suggested that some 3-D properties can be processed preattentively. However, discriminating between an upright, shaded cube and an inverted one is a difficult, attention-demanding task in a dual-task paradigm.
In order to reconcile the above results, a number of researchers have postulated that certain visual computations, such as recognizing the gist of a scene, do not require selective attention (Rensink, 2001; Treisman, 2006), perhaps occurring in a separate pathway with no bottleneck limitations (Wolfe, 2007). Others have postulated a hierarchy of preattentive features, which includes not only simple features like color and orientation but also complex conjunctive features that respond to specific object or scene categories, like “animal,” “vehicle,” or “face” (VanRullen et al., 2004; Reddy and VanRullen, 2007). These authors reason that since the more complex features are processed by higher levels of the visual stream, which have larger receptive fields, they cannot preattentively guide visual search in the way that a low-level feature like color can. However, they do allow for preattentive processing of scenes in dual tasks. This theory, too, gives special status to processing of scenes; complex conjunctive features exist if and only if the brain has cells or brain regions specific to the particular type of discrimination. VanRullen et al. (2004) then, should predict easy dual-task performance for scene, face, and place tasks.
However, it is not merely scenes, faces, and places that afford easy preattentive processing of gist. It is easy to get the gist of a set of items (Treisman, 2006). Figure 1D can easily be seen to contain an array of circles split into quarters, alternating black and white. We have a rough guess as to their number. Yet it is surprisingly difficult to tell that a 3 × 3 sub-array consists of white “bowties,” whereas the rest are black bowties. How do we get a sense of the complex array of shapes, yet have difficulty discriminating between black and white bowties? Recently, researchers have suggested that, even without attention, the visual system can compute “ensemble statistics” of a set of items, such as mean size and mean orientation (see Alvarez, 2011, for a review). Clearly, however, the limited set of ensemble statistics which have been proposed is insufficient to capture the gist of Figure 1D.
As another way out of these conundrums, some researchers (e.g., Allport, 1993; Tsotsos et al., 1995) have argued that selection does not have a single locus of operation, but can occur throughout visual processing. Similarly, Nakayama (1990) and Treisman (2006) have suggested that one can attend to regions of varying size and complexity, and that the available processing depends upon the nature of the attended region. Attend to an object, and identify that object, but perhaps not others. Attend to a set of objects, and extract set (“ensemble”) properties, but perhaps not the properties of individual objects. Attend to a scene, and get the scene gist.
Theories with ensemble statistics, special status for scene processing, or flexible representations which depend upon task may well prove correct (the last is certainly difficult to disprove). Until these theories are more fully specified, we fundamentally do not know what they can and cannot predict.
In this paper we propose a simpler, unified explanation, by re-conceptualizing early visual processing steps. Discriminating between early and late attentional selection fundamentally requires knowledge of the stages of processing, and that knowledge remains incomplete. In particular, if early stages include significant information loss not attributable to selective attention, this will profoundly affect our interpretation of the experimental results. We next review a recent model of just such an information loss in peripheral vision, and show that this lossy representation may be responsible for many of the puzzling results described above.
Recent Work: Peripheral Vision
Peripheral vision is, as a rule, worse than foveal vision, and often much worse. Only a finite number of nerve fibers can emerge from the eye, and rather than providing uniformly mediocre vision, the eye trades off sparse sampling in the periphery for sharp, high resolution foveal vision. If we need finer detail (for example for reading), we move our eyes to bring the fovea to the desired location. This economical design continues into the cortex: the cortical magnification factor expresses the way in which cortical resources are concentrated in central vision at the expense of the periphery. However, acuity loss is not the entire story, as made clear by the visual phenomena of crowding. An example is given in Figure 2. A reader fixating the central cross will likely have no difficulty identifying the isolated letter on the left. However, the same letter can be difficult to recognize when flanked by additional letters, as shown on the right. An observer might see the letters on the right in the wrong order, perhaps confusing the word with “BORAD.” They might not see an “A” at all, or might see strange letter like shapes made up of a mixture of parts from several letters. This effect cannot be explained by the loss of acuity, as the reduction in acuity necessary to cause flankers to interfere with the central target on the right would also completely degrade the isolated letter on the left. (Lettvin, 1976, makes similar points about both the subjective experience and the infeasibility of acuity loss as an explanation.)

Figure 2. Visual crowding. The “A” on the left is easy to recognize, if it is large enough, whereas the A amidst the word “BOARD” can be quite difficult to identify. This cannot be explained by a mere loss of acuity in peripheral vision.
What mechanism could account for crowding? Recent research has suggested that the representation in peripheral vision consists of summary statistics computed over local pooling regions (Parkes et al., 2001; Levi, 2008; Pelli and Tillman, 2008; Balas et al., 2009). In particular, we have proposed that the visual system might measure a fixed set of summary statistics: the marginal distribution of luminance; luminance autocorrelation; correlations of the magnitude of responses of oriented V1-like wavelets across differences in orientation, neighboring positions, and scale; and phase correlation across scale. This perhaps sounds complicated, but really is not: computing a given second-order correlation merely requires taking responses of a pair of V1-like filters, pointwise multiplying them, and taking the average over a “pooling region.” These summary statistics have been shown to do a good job of capturing texture appearance (Portilla and Simoncelli, 2000; Balas, 2006). Discriminability based on these summary statistics has been shown to predict performance recognizing crowded letters in the periphery (Balas et al., 2009).
What do we know about the pooling regions over which the summary statistics are computed? Work in crowding suggests that they grow linearly with eccentricity – i.e., with distance to the center of fixation – with a radius of ∼0.4–0.5 the eccentricity. This has been dubbed “Bouma’s law,” and it seems to be invariant to the contents of the stimulus (Bouma, 1970). The pooling regions are elongated radially outward from fixation. Presumably overlapping pooling regions tile the entire visual input. We call our model, which represents images in terms of a fixed set of hypothesized “texture” statistics, computed over local pooling regions that tile the visual input in this fashion, the texture tiling model (TTM).
Representation in terms of a fixed set of summary statistics provides an alternative tool for dealing with a limited processing capacity in vision. Limited capacity, rather than implying a need for selective attention (Broadbent, 1958), may require our perceptual systems to “describe nature economically” (Attneave, 1954). Attneave suggested that “a major function of the perceptual machinery is to strip away some of the redundancy of stimulation, to describe or encode incoming information in a form more economical than that in which it impinges on the receptors.” Representation in terms of summary statistics provides a compressed representation of the visual input, which can capture detailed information at the expense of uncertainty about the locations of those details. Figure 3 gives a demonstration. Figure 3B shows an image synthesized to have the same summary statistics as the original image in Figure 3A, using the texture synthesis algorithm of Portilla and Simoncelli (2000). This algorithm starts with an image – usually random noise – and iteratively coerces it until it has approximately the same summary statistics as the original. We call these synthesized images “mongrels.” The results are intriguing. In order to coerce the noise “seed” image to share the same statistics as the original, apparently one must start making quadrisected circles! However, the statistics are not sufficient to distinguish between circles with black vs. white bowties; Figure 3B has the same statistics as Figure 3A, yet it contains both bowtie patterns, and the original contained only black. This may explain the difficulty segmenting the array in Figure 1D.
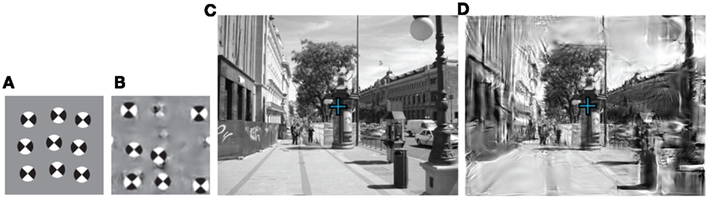
Figure 3. (A) Original image. (B) We can visualize the information available in a set of summary statistics by synthesizing a new “sample” with the same statistics as the original. Here we constrain the statistics for a single pooling region (the whole image). (C) Original photograph. (D) A new “sample,” which has the same local summary statistics as the original. The local regions overlap, tile the visual field, and grow linearly with distance from the fixation (blue cross).
Figures 3C,D shows another example, in which we have synthesized a scene to have the same local summary statistics as the original. The statistics seem sufficient to categorize the scene, and even navigate down the sidewalk. The details – such as the number of cars on the street – are murky, in line with results from change-blindness.
While additional work is required to pin down the right statistical measurements, our present set provide a good initial guess. Certainly they seem quite plausible as a visual system representation. Early stages of standard models of object recognition typically measure responses of oriented, V1-like feature detectors, as does our model. They then build up progressively more complex features by looking for co-occurrence of simple structures over a small pooling region (Fukushima, 1980; Riesenhuber and Poggio, 1999; Deco and Rolls, 2004). These co-occurrences, computed over a larger pooling region, can approximate the correlations computed by our model.
Second, our summary statistics appear to be quite close to sufficient. Balas (2006) showed that observers are barely above chance at parafoveal discrimination between a grayscale texture synthesized with this set of statistics and an original patch of texture. More recent results have shown a similar sufficiency of these summary statistics for capturing the appearance of real scenes. Freeman and Simoncelli (2011) synthesized full-field versions of natural scenes. These syntheses were generated to satisfy constraints based on local summary statistics in regions that tile the visual field and grow linearly with eccentricity. When viewing at the appropriate fixation point, observers had great difficulty discriminating real from synthetic scenes. That the proposed statistics are close to sufficient for capturing both texture and scene appearance is impressive; much information has been thrown away, and yet observers have difficulty telling the difference between an original image and a noise image coerced to have the same statistics.
Finally, significant subsets of the proposed summary statistics are also necessary. If a subset of statistics is necessary, then textures synthesized without that set should be easily distinguishable from the original texture. Balas (2006) has shown that observers become much better at parafoveal discrimination between real and synthesized textures when the syntheses do not make use of either the marginal statistics of luminance, or of the correlations of magnitude responses of V1-like oriented filters.
To test the TTM, we make use of texture synthesis techniques (Portilla and Simoncelli, 2000, for local patches; Rosenholtz, 2011, for complex images) to generate new images – “mongrels” – that share approximately the same summary statistics as each original stimulus. Mongrels enable intuitions and the generation of testable predictions from our model. The general logic is essentially this: we can generate a number of mongrels (e.g., Figure 3B) which share the same local summary statistics as each original stimulus (e.g., Figure 3A). The model cannot tell these mongrels apart from the original, nor from each other. If these images are indistinguishable, how hard would a given task be? If we could not tell an image with all black bowtie circles from one with both white- and black- bowtie circles, it would be quite difficult to, say, find a white bowtie circle among black bowtie circles.
By synthesizing mongrel images which are equivalent to the original image, according to the model, we can generate testable model predictions for a wide range of tasks. Most powerfully, we can predict performance on higher-level visual tasks without needing a model of higher-level vision. We do not need to build a black vs. white bowtie discriminator to tell from mongrels (like Figure 3B) that our model predicts this task will be difficult. We do not need to model scene classification to tell from mongrels (like Figure 3D) that the model predicts easy discrimination between a street scene and a beach. In practice, we ask subjects to perform a discrimination task with a number of synthesized images, and we measure their task performance with those mongrels as a measure of the informativeness of the summary statistics for a given task (see Materials and Methods.) We have previously used this methodology to make testable predictions of the model for a number of visual crowding tasks, and shown that the model can predict performance on these tasks (Balas et al., 2009).
Results: The Model Makes Sense of Diverse Phenomena
Feature, Conjunction, and Configuration Search
Rethinking visual search in light of recent understanding of peripheral vision provides immediate insight. If early visual representation is in terms of a fixed set of summary statistics, computed over pooling regions that grow with eccentricity, then for typical search displays many of those pooling regions will contain more than a single item. This suggests that the visual system’s real task as it confronts a search display is to discriminate between peripheral patches containing a target (plus distractors) from those containing only distractors. This is quite different from the usual formulation, in which the key determinant of search performance is whether an individual target is preattentively discriminable from an individual distractor.
In Figure 4, the target (“Q”) is not visible near the current fixation (red crosshairs), so the subject continues searching. Where to look next? A reasonable strategy is to seek out regions that have promising statistics. The green and blue disks represent two hypothetical pooling regions in the periphery, one containing the target (plus distractors), the other containing only distractors. If the statistics in a target-present patch are noticeably different from those of target-absent patches, then this can guide the subject’s eyes toward the target. However, if the statistics are inadequate to make the distinction, then the subject must proceed without guidance.
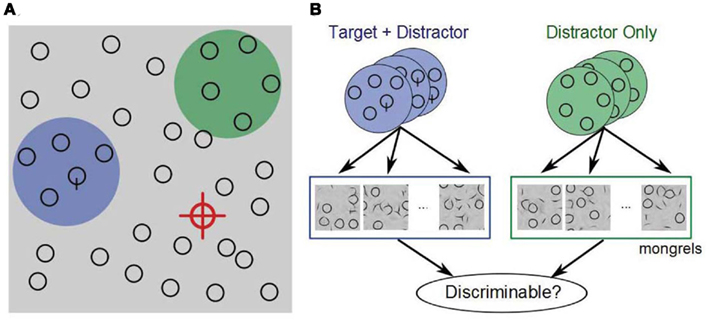
Figure 4. (A) In visual search, we propose that on each fixation (red cross), the visual system computes a fixed set of summary statistics over each local patch. Some patches contain a target and distractors (blue), whereas most contain only distractors (green). The job of the visual system is to distinguish between promising and unpromising peripheral patches and to move the eyes accordingly. (B) We hypothesize, therefore, that peripheral patch discriminability, based on a rich set of summary statistics, critically limits search performance. To test this, we select a number of target + distractor and distractor-only patches, and generate a number of patches with the same statistics (“mongrels”). We then ask observers to discriminate between target + distractor and distractor-only synthesized patches, and examine whether this discriminability predicts search difficulty.
The prediction is that to a first approximation, search will be easy only if the visual statistics of target-present patches are sufficiently different from those of target-absent patches. (Two conditions with the same statistical discriminability might nonetheless lead to different performance due to peculiarities of later processing; e.g., stimuli like letters might be more effectively processed than non-letters at a later stage.) We can generate mongrels of target-present and target-absent patches, which share the same summary statistics as the corresponding original patches. Figure 5 shows examples for three conditions. To our model, these mongrels are indistinguishable from the original patches. How difficult would we expect a given search task to be?
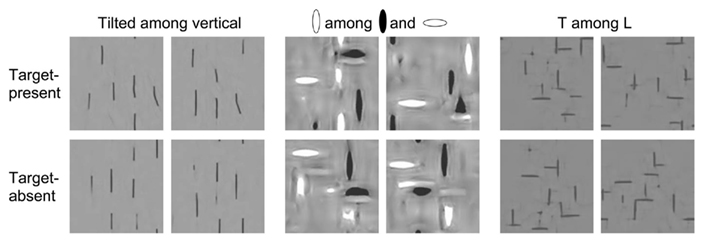
Figure 5. Example mongrels for target-present (row 1) and target-absent (row 2) patches, for three classic search conditions. (A) tilted among vertical; (B) orientation–contrast conjunction search; (C) T among L. How discriminable are target-present from target-absent mongrels? Inspection suggests that the summary statistic model correctly predicts easy search for tilted among vertical, more difficult conjunction search, and yet more difficult search for T among L, as validated by results in Figure 6.
Search for a tilted line among vertical is easy (Treisman and Gelade, 1980). The target-present mongrels for this condition clearly show a target-like item, whereas the distractor-only mongrels do not. Patch discrimination based upon statistics alone should be easy, predicting easy search.
Conjunction search for a white vertical among black verticals and white horizontals shows some intriguing “illusory conjunctions” (Treisman and Gelade, 1980; Treisman and Schmidt, 1982) – white verticals – in the distractor-only mongrels. This apparent lack of binding has previously been attributed to a need for selective attention for feature binding, but in our model is due to representation in terms of a rich set of image statistics. The inherent ambiguity in this representation makes it more difficult to discriminate between target-present and target-absent patches, and correctly predicts more difficult search.
Search for a “T” among “L”s is known as a difficult “configuration search” (Wolfe et al., 1989). In fact, the mongrels for this condition show “T”-like items in some of the distractor-only patches, and no “T”-like items in some of the target + distractor mongrels. Again, we note that the model predicts confusions which have previously been attributed to a lack of preattentive “binding.” Patch discrimination based upon summary statistics looks difficult, predicting difficult search.
Figure 6 plots search performance for five classic search tasks, vs. the discriminability of target-present vs. target-absent mongrels (see Materials and Methods). Results agree with the above intuitions. The data shows a clear relationship between search performance and visual discriminability of patch statistics as measured by human discrimination of the mongrels (R2 = 0.99, p < 0.01; Rosenholtz et al., under review). Crucially, one can predict classic differences between feature, conjunction, and configuration search, with a model with no attentional selection.
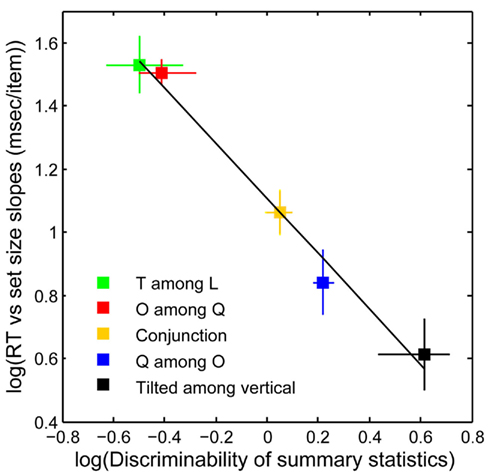
Figure 6. Search performance vs. statistical discriminability. y-Axis: search performance for correct target-present trials, as measured by log 10 (search efficiency), i.e., the mean number of milliseconds (ms) of search time divided by the number of display items. x-Axis: “statistical discriminability” of target-present from target-absent patches based on the empirical discriminability, d′, of the corresponding mongrels. There is a strong relationship between search difficulty and mongrel discriminability, in agreement with our predictions. [y-axis error bars = SE of the mean; x-axis error bars = 95% confidence intervals for log 10 (d′)].
Search and Dual-Task Performance on Shaded Cubes
The previous section demonstrated that the TTM can predict search results previously attributed to an early attentional selection mechanism. What about search results which are more problematic for early selection? Enns and Rensink (1990) demonstrated that searching for a side-lit cube among top-lit shaded cubes is quite efficient (∼8 ms/item), particularly when compared with search using “equivalent” 2-D targets and distractors (>20 ms/item). This would seem to suggest that direction of illumination might be available prior to operation of selective attention. Other results from Enns and Rensink (1990) and Sun and Perona (1996) have suggested that 3-D orientation might be available preattentively. This work calls into question the early selection story, as surely 3-D orientation and lighting direction do not occur earlier in visual processing than piecing together vertical and horizontal bars to make a T-junction. The story has been further complicated by evidence that observers have difficulty distinguishing upright from inverted cubes in a dual-task setting (VanRullen et al., 2004).
We examined whether the TTM can shed light on these puzzling results. We generated a number of mongrels for target-present and target-absent patches for search for a side-lit cube among top-lit (Enns and Rensink’s Experiment 3A), as well as for some of their “equivalent” 2-D targets and distractors (Experiments 2B and 2C). As described in Section “Materials and Methods,” observers judged whether each mongrel came from an original patch that contained or did not contain the target.
Preliminary results demonstrate that the TTM can predict easier search for the 3-D condition (d′ = 2.44) than for the 2-D conditions (mean d′ = 1.78). Essentially what this means is that there are 2-D pattern differences between the 3-D condition and the 2-D conditions, which show up in the summary statistics and make it easier in the 3-D condition to discriminate target-present from target-absent patches. The summary statistic information provides better search guidance in the 3-D case than in the 2-D conditions.
We then asked why distinguishing between an upright and inverted cube was difficult under dual-task conditions. For our model, this is actually an unsurprising result. Our summary statistic representation, within a single pooling region, is theoretically unable to tell an upright from an inverted figure (see Figures 7A,B), though constraints from multiple pooling regions may be able to do the discrimination. This is an odd consequence of our model, which nonetheless has correctly predicted performance in a peripheral discrimination task (Balas et al., 2009).
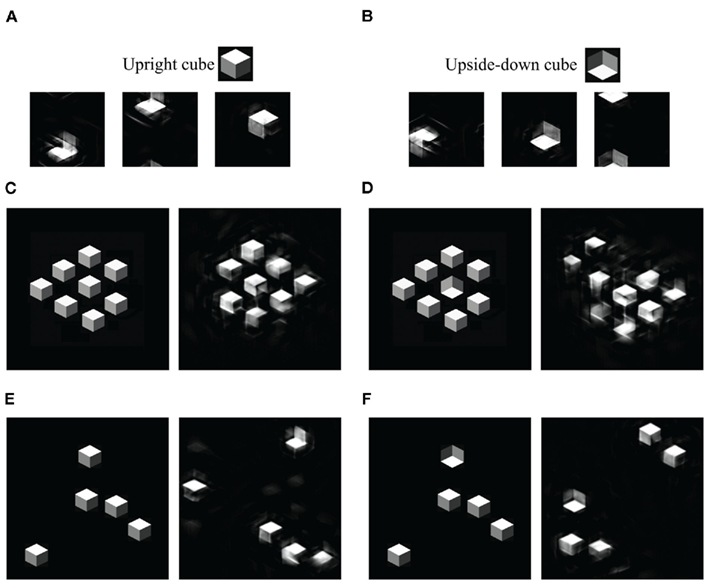
Figure 7. Mongrels of shaded cubes. (A) Mongrels synthesized from an image containing a single upright cube (inset). (B) Mongrels of an image with a single inverted cube (inset). The statistics have difficulty discriminating an upright from inverted cube. (C–F) Original (left) and mongrel (right) pairs. (C,D) Patches from a dense, regular display. (E,F) Patches from a sparse display. For the dense display, the target-absent mongrel shows no sign of a target, while the target-present mongrel does. For the sparse display, both mongrels show signs of a target. (Single pooling region mongrels wrap around both horizontally and vertically, so a cube may start at the top and end at the bottom of the image. The mongrels in (C–F) have been shifted to the middle, for easy viewing.)
What sense can we make, then, of easy search (Enns and Rensink, 1990; Sun and Perona, 1996) for an inverted cube among upright cubes? Enns and Rensink reported slopes of 8 ms/item. For visual search, it matters not only what the target and distractor look like, but also what the search display looks like. Figure 1B shows an example display, adapted from Sun and Perona (1996). The cubes are so densely packed that they are almost regularly aligned with one another (Enns and Rensink (1990) used similar but less dense displays). The dense, regular array of cubes may have introduced emergent features that could serve as cues to facilitate search. Figures 7C,D shows mongrels of dense vs. sparse displays. It appears from this demo that the dense regular arrangement contains features that favor recognition of homogeneous, distractor-only patches. (Future work is required to test whether the TTM can predict effects of item arrangement.)
To test the possibility of emergent features in the dense displays, we re-ran search conditions with upright and inverted cubes similar to those described by Sun and Perona (1996), but with the same random, less dense arrangement of elements as used in our previous search tasks (e.g., Figure 8A). As we expected, less dense and regular displays led to far less efficient search (Figure 8B). We conclude that earlier results demonstrating efficient search in these particular cube search conditions were efficient due to yet-unspecified emergent features of the displays.
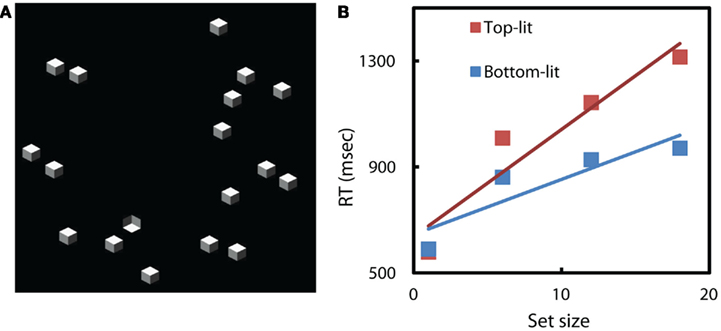
Figure 8. (A)An example of our search displays. Target is an inverted cube; distractors are upright cubes. (B) More irregular search displays leads to less efficient search, for this task. Average response times on correct target-present trials vs search set size. RT slope of searching for upright is 40 ms/item, the slope of searching for inverted cube is 21 ms/item.
Our TTM explains not only the basic visual search results, but also easier search for some 3-D cube stimuli than for “equivalent” 2-D stimuli. These results were problematic for an early selection story. Furthermore, we predict difficult dual-task performance discriminating upright vs. inverted cubes. Insights gained from the model led us to re-run search experiments on upright vs. inverted cubes, and to the conclusion that the original search displays may have enabled easy search due to an emergent feature.
Search, Dual-Task, and Rapid Perception of Scenes
Other problematic results for the early selection story have involved scene perception. Scene perception is very fast, and people can do scene discrimination tasks, such as animal vs. non-animal, when attention is engaged elsewhere. However, searching for an animal scene among non-animal distractors is a slow, serial search that requires attention. If animals can be detected preattentively in a dual-task situation, why do not they “pop-out” in a search task (Li et al., 2002; VanRullen et al., 2004)?
VanRullen et al. (2004) suggest that any discrimination task can be preattentive if there is a dedicated population of neurons in visual cortex that performs that task. With simple tasks, such as color discrimination, the dedicated neurons are located early in the visual system and these neurons can also guide visual search, producing “pop-out” effects. For more complex discriminations, such as animal vs. non-animal, the dedicated neurons are located higher in the visual stream, probably in inferotemporal cortex. These neurons cannot guide visual search, because their receptive fields are so large that they typically contain multiple items, so there is neural competition between target and distractors (Reddy and VanRullen, 2007).
Here we ask whether our model can explain the dichotomy between search performance and rapid/dual-task performance without needing to rely upon special neuronal populations dedicated to particular scene discrimination tasks. Earlier in this paper, we argued that the real task for the visual system in visual search is not to discriminate between a single target and a single distractor, but rather is often to discriminate between target-present and target-absent patches which may contain information from multiple items. With this reconceptualization of search, one expects search performance often to conflict with performance of tasks involving single items. We hypothesize that typical scene discrimination tasks (such as animal vs. non-animal) are easy with rapid presentation, even in a dual-task situation, because the summary statistic representation is sufficient to distinguish a single target from a distractor. However, when multiple images are presented in a crowded search display, the summary statistics mix features from nearby images, and it is no longer possible to clearly identify the region of the array which contains the target.
To test this hypothesis, we first had subjects perform one of two go/no-go rapid scene perception tasks (animal vs. non-animal or vehicle vs. non-vehicle) with image presentation either at fixation or 11° to the left or right of fixation (see Materials and Methods). Subjects were asked to respond to target images (animals or vehicles) as quickly as possible. We also synthesized “mongrel” versions of each of the images from the go/no-go tasks, using the TTM with fixation of the synthesis procedure set as in the go/no-go task (either in the center of each image, or 11° to the left or right of center). Examples of target images from the go/no-go task and their corresponding mongrels are shown in Figure 9. A separate group of subjects performed one of two mongrel-classification tasks: discriminating animal mongrels from non-animals or vehicle mongrels from non-vehicles.
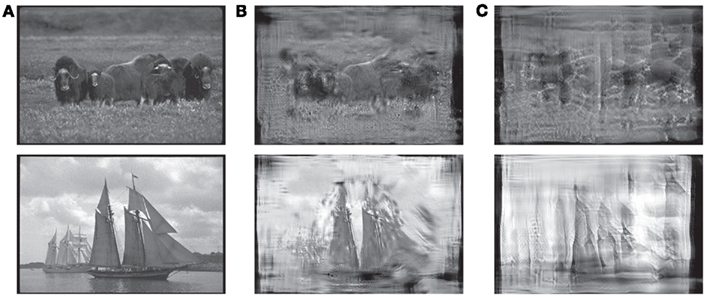
Figure 9. Example stimuli from animal- and vehicle-detection tasks. (A) Target images used in the go/no-go task. (B) Mongrels synthesized with fixation in the center of the image. (C) Mongrels synthesized with fixation 11° left of the image center.
Overall, subjects perform very well in the go/no-go tasks: averaging 94% correct detection at fixation, and 74 and 76% correct when detecting animals or vehicles, respectively, at 11° eccentricity. Performance is considerably lower with the mongrels: subjects average 85% correct in distinguishing mongrel animals from non-animals and 81% correct in distinguishing vehicles from non-vehicles. Performance with the peripheral mongrels is even lower, but still above chance: subjects average 60% correct in the animal/non-animal task and 62% correct in the vehicle/non-vehicle mongrel task. More work remains to determine the cause of this difference in performance, particularly on the peripheral tasks. These peripheral mongrels are challenging for our synthesis procedure, in terms of converging to a solution with the same statistics as the original. It is also possible that our model throws out a bit too much information, and that this was apparent in the scene task but not on crowding and search tasks with simpler displays.
Despite the overall difference in accuracy between the two tasks, target detection in the go/no-go rapid perception tasks correlates with responses to the mongrel images. Figure 10 shows a comparison of the responses to images in each task: images have been binned according to the proportion of target responses (“animal” or “vehicle”) they received in the mongrel sorting task, and points represent the average proportion of target responses to each image bin in the go/no-go task. The more strongly a mongrel is classified as “animal,” the more “animal” responses it receives in the go/no-go task, and the same is true of vehicles. The linear relationship between mongrel and go/no-go responses holds both when the image is presented centrally and when it is presented in the periphery. The fact that mongrel animal images can be distinguished from mongrel non-animals does not mean that search for animal among non-animal distractors should be an easy pop-out search. When multiple images are presented in a search display, features of the distractors may be combined with features of the target to mask its location, or combined features from two different distractors may create an illusory target. Figure 11 illustrates this with a “mongrel” version of a scene search array, similar to the animal among non-animal search arrays used by VanRullen et al. (2004). The location of the animal image is not obvious in the mongrel array, even though this particular animal image’s mongrel is fairly easy to identify as an animal when it is synthesized as an isolated image in the periphery.
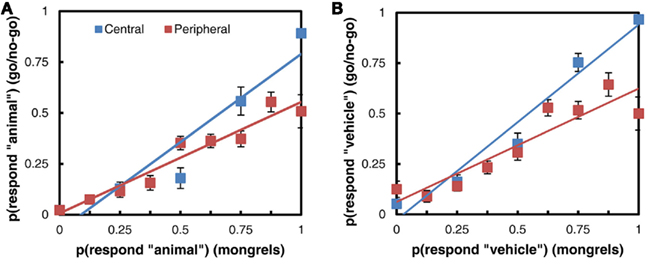
Figure 10. Comparison of mongrel and go/no-go responses. (A) Animal vs. non-animal task. (B) Vehicle vs. non-vehicle task.
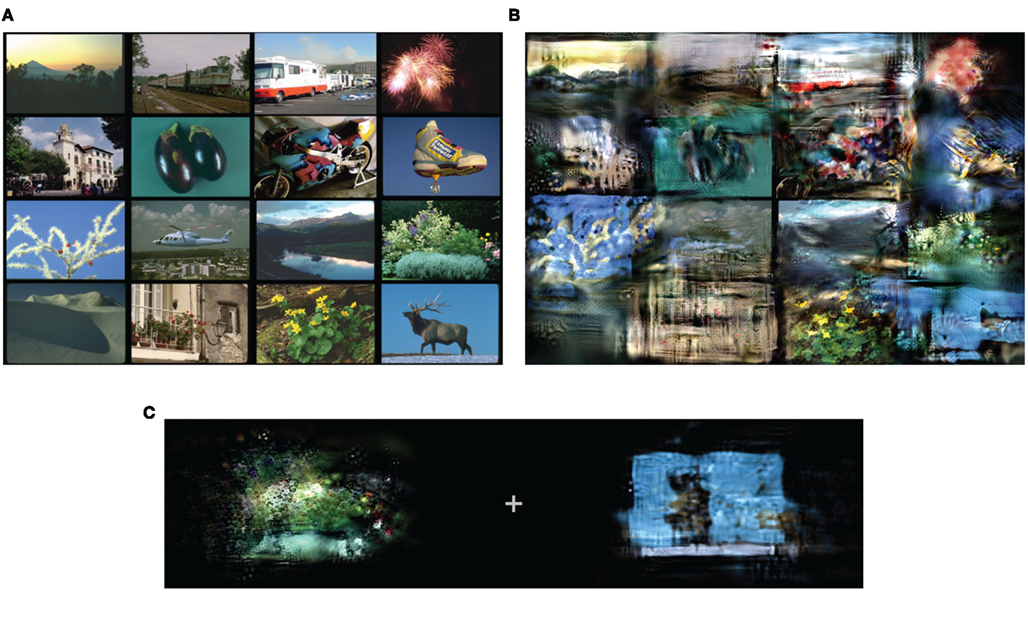
Figure 11. (A) A scene search array in the style of VanRullen et al. (2004). (B) A mongrel version of the array, fixation at center. It is difficult to determine, from the mongrel, whether there is an animal. (C) Mongrels generated from two scenes from the array (the elk and the hedge), shown in isolation in the periphery. In this case, it is easy to determine which image contains the animal.
Discussion
This paper has focused on re-evaluating the role of top-down selective attention on perceptual processing. In the standard story, based on studies of visual search, such attentional selection occurs early in the processing stream. This conclusion was drawn from reasonable assumptions – at the time and even today – about early stages of processing, and reasonable experimental logic based upon those assumptions. Yet the resulting theories have been problematic, and had difficulty predicting a number of basic effects, such as extraction of gist from scenes and other displays, search for 3-D shaded cubes among differently lit cubes, zombie behaviors, and results from different experimental paradigms. We have suggested that these results can be explained more simply by a newer model of the processing in early vision, in which the visual system represents its inputs by a rich set of summary statistics.
For clarity, it is worth reformulating both the old and new ways of thinking in terms of strategies for dealing with limited capacity. The previous story assumes that the mechanism for operating with a limited capacity channel is selective attention. By this account, various parts of the input can be thought of as competing for use of that channel. These parts might be objects (e.g., the images of a one-way sign, a pedestrian, and a tree), feature bands (e.g., “red” or “vertical”), or locations (“upper left”). Selective attention is presumed to enable the different parts of the input to share the limited capacity channel by taking turns using that channel. In digital communications – from which the “limited capacity” terminology in psychology derives – strategies for splitting up use of the channel so that multiple competing “senders” can access it are known as multiplexing, and the particular strategy of having the senders take turns using the channel is known as time-division multiplexing. (A number of other strategies exist; cell phone systems, for example, use an entirely different kind of multiplexing.) “Selection,” as defined in the Section “Introduction,” is equivalent to multiplexing, and common use of the term refers to time-division multiplexing.
When it comes to conscious awareness, the analogy to time-division multiplexing seems natural. We become aware, in a street scene (Figure 1C), of the one-way sign, then the pedestrian, then the tree; subjectively, we experience different objects, features, or locations competing for awareness. In perceptual processing, the analogy to multiplexing is far less obvious. A digital communications engineer, faced with the task of transmitting a street scene along a limited capacity channel, would be surprised at the suggestion that one should first transmit the one-way sign, then the pedestrian, then the tree. For one thing, finding each of the component objects in order to transmit their information requires a great deal of complicated processing. In terms of dealing with a limited capacity channel, there is lower-hanging fruit.
A more obvious choice to the engineer would be compression, also known as source coding1. Compression consists of representing the input with as few “bits” as possible, while retaining as much fidelity of the original signal as possible. By compressing the input, one can push more information through a limited capacity channel, in less time. Compression can be lossless, i.e., such that one could perfectly reconstruct the original signal. Simply taking into account regularities of the world (e.g., in English text, some letter combinations are more likely than others) and redundancy in the signal (a patch of bright pixels in an image increases the chance of more bright pixels nearby) can reduce the number of bits necessary. Compression can also be lossy, in which one typically throws away “unimportant” information in order to obtain greater savings in the number of bits required. For example, JPEG image compression, in addition to taking into account regularities and redundancies in the input, typically represents high spatial frequency information more coarsely than low spatial frequency, as moderate loss of high spatial frequency information may be difficult for an observer to detect. Lossy compression “selects” what information to keep and throw away, but is theoretically distinct from selection as defined here, i.e., multiplexing. (Lossless compression, on the other hand, facilitates communication through a limited capacity channel, while involving no “selection” whatsoever.)
The notion of dealing with limited capacity by compressing the input has not been lost on perception researchers. Even as Broadbent (1958) was essentially talking about multiplexing, Attneave (1954), Miller (1956), and Barlow (1961) were talking about various forms of compression. However, the association between consciousness and attention may have biased the way that many researchers thought about limited capacity. In the attention literature, it is often stated without proof that limited capacity implies the need for selection. Certainly limited capacity does not obviously require selective attention, i.e., multiplexing. Multiplexing is necessary in digital communications only for certain situations; should images obviously be thought of as containing multiple senders competing for limited capacity? On the other hand, redundancies and regularities in the world make compression a clear choice of strategy for dealing with limited capacity.
If the visual system implements a lossy compression strategy, this creates problems for reasoning about early vs. late selection. In behavioral experiments, one can observe only the inputs to the visual system (images of the world), and the outputs (performance). If information loss due to compression is misattributed to multiplexing (selective attention), it becomes difficult to determine the stage at which selective attention operates.
Many of the hypothesized “fixes” to the standard early selection story amount to lossy compression strategies. Consider, for example, suggestions that the statistics of a set of items might be available preattentively (Treisman, 2006; see Alvarez, 2011 for a review), and that image statistics might underlie preattentive recognition of the gist of a scene (Oliva and Torralba, 2006).
Our TTM incorporates both multiplexing and compression. The multiplexing mechanism consists of shifting one’s eyes in order to control what information gets through the “channel” at a given moment. For a given fixation location, the visual system has devised a general-purpose compression scheme, which represents the input with a fixed, rich set of local summary statistics, computed over regions that tile the visual field and grow with eccentricity. We have shown that this model can predict the difficulty of visual search tasks; it predicts the binding errors that have previously led researchers to conclude that attentional selection occurs early, while also predicting the ease of search for shaded cubes, which seems antithetical to early selection. Our model also shows promise in resolving a number of the conundrums surrounding the locus of attentional selection: the fact that observers can easily judge the gist of a scene or display, while being murky on the details, and the difference between scene search and dual-task performance. The TTM can more parsimoniously explain these phenomena than an early selection mechanism.
If selective attention occurs later, then there is no reason to assume that consciousness would be required for basic perceptual processing. This is some relief, and fits well with a number of functional theories of consciousness. Crick and Koch (1990), for instance, suggest that consciousness involves an attentional mechanism, and that “one of the functions of consciousness is to present the results of various underlying computations.” If one is presenting the results of only a select few computations, presumably one would want other useful computations to continue unconsciously, not stop at the stage of feature maps.
As another example, Dennett (1991) has proposed the Multiple Drafts theory of consciousness, in which multiple channels of “specialist circuits,” processes of interpretation, operate in parallel. Many of the “drafts” produced by these processes are short-lived, but some are “promoted to further functional roles.” It is unclear what role, if any, attention need play, unless perhaps it acts as a probe which asks questions of the parallel processing streams. Regardless, surely in this framework one would not want to be restricted to promoting drafts at such an early stage of interpretation as basic feature maps. Such “specialists” would not be very specialized, and would leave a great deal of interpretation to some other processing module. Both points seem antithetical to Multiple Drafts theory.
Finally, in Global Workspace theory, consciousness comes into play when information needs to be accessed by multiple brain systems, such as memory, motor control, verbal systems, and high-level decision-making systems (Baars, 2005). If we view selective attention as the mechanism that puts information into the “workspace,” then we would hardly expect attention to involve early selection. The visual system should not have to call a conference of multiple brain systems just to decide whether an image contains a corner.
Our rethinking of early visual representation seems to have eliminated a large role for attention in visual search, and perhaps in other tasks as well. Clearly attention does have measurable effects in both physiology and behavior (e.g., dual-task experiments). What might attention do? Attention seems to be able to modulate neuronal responses to produce increased firing rates, increase signal-to-noise, and narrow neuronal tuning curves (see Reynolds and Heeger, 2009, for a review). These effects, by themselves, seem unlikely to explain the difference between single- and dual-task performance. We suggest that different tasks (e.g., performing a covert discrimination of a peripheral stimulus with or without a simultaneous discrimination task at the fovea) allow more or less complicated communication within a population of neurons, enabling more or less complicated inferences. With minimal attention, the visual system might have access to local statistics from individual pooling regions across the visual field, but not be able to combine information from overlapping pooling regions to make more complex inferences. Intersecting constraints from overlapping pooling regions may not be needed for certain tasks, such as recognizing the general category of a scene (see Oliva and Torralba, 2006). However, more complex inference on the outputs of multiple pooling regions might make it possible to tell if an isolated cube were upright or inverted. Comparing the information from multiple overlapping pooling regions might explain the modest decrease in psychophysical pooling region size with attention (Yeshurun and Rashal, 2010), enable identification of an attended object when two are present within the receptive field of a given neuron (Desimone and Duncan, 1995), or allow an item to be localized with more precision than might be expected from a single large pooling region (as suggested by Rousselet et al., 2005).
The arguments presented in this paper should have a significant impact on discussions of the association between attention and awareness. If one attributes performance in a number of tasks to mechanisms of attention, when in fact performance is limited by lossy representation in early vision, this muddies questions of whether attention and awareness are the same thing and how they are linked. Just as one needs to properly understand representation to understand the impact of attention, one needs to understand attention to understand its relationship to awareness.
We have contributed to this discussion by presenting a predictive model of peripheral vision. Image synthesis techniques enable a methodology for making concrete, testable predictions of this model for a wide range of tasks. In developing such a model, it is important to understand not only that crowding occurs, perhaps because of “competition” between stimuli present in a receptive field (Desimone and Duncan, 1995), but also what information is available to the visual system in a crowded display. This information may be the elements from which perception is made, and be predictive of performance on a wide range of visual tasks.
Materials and Methods
Visual Search Experiments and Corresponding Mongrel Experiments
Subjects
Ten subjects (six male) participated in feature, conjunction, and configuration search experiments. The mongrel discrimination task for five classic search conditions was carried out by five other subjects (four male). A different group of nine subjects participated in the 3-D cube search experiment. The mongrel discrimination of 3-D cubes was carried out by a different group of eight subjects. Subjects’ ages ranged from 18 to 45 years. All reported normal or corrected-to normal vision and were paid for their participation.
Stimuli and procedure: Visual search experiments
Our visual search experiments resemble classic search experiments in the literature. We tested five search conditions: conjunction (targets defined by the conjunction of luminance contrast and orientation), rotated T among rotated Ls, O among Qs, Q among Os, and feature search for a tilted line among vertical lines. For 3-D cube search, we tested search for an inverted cube among upright, and vice versa.
Stimuli were presented on a 40-cm × 28-cm monitor, with subjects seated 75 cm away in a dark room. We ran our experiments in MATLAB, using the Psychophysics Toolbox (Brainard, 1997). The search displays consisted of either all distractors (target-absent trial) or one target and the rest distractors (target-present trial). Target-present and target-absent displays occurred with equal probability.
Each search task had four levels of the number of items in the display (the “set size”): 1, 6, 12, or 18. Stimuli were randomly placed on four concentric circles, with added positional jitter (up to one-eighth degree). The radii of the circles were 4°, 5.5°, 7°, and 8.5° of visual angle (v.a.).
Each search display remained on screen until subjects responded. Subjects indicated with a key press whether each stimulus contained or did not contain a target, and were given auditory feedback. Each subject finished 144 trials per search condition (72 target-present and 72 target-absent), evenly distributed across four set sizes. The order of the search conditions was counterbalanced across subjects, and blocked by set size.
Stimuli and procedure: Mongrel discrimination of target-present vs. target-absent patches
To measure the informativeness of summary statistics for the search tasks, we first generated 10 target-present and 10 target-absent patches for each search condition described above. Then, for each patch, we synthesized 10 new image patches with approximately the same summary statistics as the original patch, using Portilla and Simoncelli’s (2000) texture synthesis algorithm. This algorithm first measures a set of wavelet-based features at multiple spatial scales, then computes a number of summary statistics, including joint statistics that describe local relative orientation, relative phase, and wavelet correlations across position and scale. To synthesize a new texture, the algorithm then iteratively adjusts an initial “seed” image (often, as in this experiment, white noise, but any starting image may be used) until it has approximately the same statistics as the original image patch. The resulting “mongrel” is approximately equivalent to the original input in terms of the summary statistics measured by the model. Figures 3B, 4, 5, and 7 all show mongrels generated using this procedure.
During each trial, a mongrel was presented at the center of the computer screen until subjects made a response. Each mongrel subtended 3.8° × 3.8° v.a. at a viewing distance of 75 cm. Subjects were shown examples of original patches, and examples of mongrels, and asked to categorize each mongrel according to whether the mongrel was synthesized from a target-present or target-absent patch. Subjects were instructed that they should look for any cues to help them perform the task, and that the target-present mongrels, for instance, might not actually contain a target. Subjects had unlimited time to freely view the mongrels.
Each of the conditions (corresponding to a search task, including the five classic search and four cube search-related conditions) had a total of 100 target + distractor and 100 distractor-only patches to be discriminated in this mongrel task, with the first 30 trials (15 target + distractor and 15 distractor-only) serving as training, to familiarize observers with the nature of the stimuli. Observers received auditory feedback about the correctness of their responses throughout the experiment.
Go/No-Go Rapid Scene Perception Task and Corresponding Mongrel Classification Task
Subjects
Twenty-four subjects participated in the rapid perception scene task, all 18–35 years old and reporting normal or corrected-to-normal vision. All subjects were paid for their participation.
A second group of 24 subjects participated in an online mongrel classification task on Amazon’s Mechanical Turk service. All subjects gave written informed consent and were paid for their participation.
Stimuli and procedure: Go/no-go task scene discrimination
Subjects were randomly assigned to either the animal-detection or vehicle-detection task (12 subjects completed each task). The stimuli were a randomly selected subset of the images used by Li et al. (2002). The target images for the animal-detection task were 240 scenes containing animals (including mammals, birds, reptiles, fish, and insects). The target images for the vehicle-detection task were 240 scenes containing vehicles (including cars, trains, boats, planes, and hot-air balloons). The distractor set for each task included 120 images from the other target category, plus 120 scenes which contained neither vehicles nor animals (which included images of plants, food, landscapes, and buildings). Stimuli were presented in grayscale at 384 by 256 pixels (8.9° × 6.0°) on a 34-cm × 60-cm monitor, with subjects seated 75 cm away in a dark room.
We ran our experiments in MATLAB, using the Psychophysics Toolbox (Brainard, 1997). Subjects were instructed to hold down the left mouse button throughout the experiment. At the start of a trial, a central fixation cross appeared for 300 ± 100 ms, and was followed by an image presented for 20 ms. The image appeared either at the center of the screen or left or right of the fixation (center of the image at 11° eccentricity). If the image contained a target (animal or vehicle), subjects were to respond by releasing the left mouse button as quickly as possible (subjects made no response to non-target images). Subjects were given 1000 ms to make their response.
Subjects completed 10 blocks of 48 trials, with a break after each block. Each block contained an equal number of target and non-target images, and an equal number of images in each of the three presentation locations (left, center, and right).
Stimuli and procedure: Mongrel scene classification
For the scene stimuli, we synthesized full-field mongrels based on the TTM. Given a fixation point, the full-field synthesis algorithm tiles the image with overlapping pooling regions. The size of the pooling regions increases with distance from fixation according to Bouma’s Law. Within each pooling region, the model computes summary statistics using procedures similar to those described above for single pooling region mongrels, and as described in Portilla and Simoncelli (2000). Synthesis is initiated by assuming the foveal region (a small circle about fixation) is reconstructed perfectly. Then, moving outward, each subsequent pooling region is synthesized using the previous partial synthesis result as the seed for the texture synthesis process. The process iterates a number of times over the entire image. We use a coarse-to-fine strategy to speed convergence. Figures 3D, 9B,C, and 11B,C show example mongrels generated using this full-field procedure.
We generated mongrels for each image used in the rapid perception experiment. Pooling regions were placed to simulate fixation in either the center of the image or 11° left or right of center, to match the rapid perception task.
Subjects completed the task on their own computer, using a web interface on the Amazon Mechanical Turk website (www.mturk.com). The experiment consisted of 480 trials which exactly matched one the 24 sessions of the rapid perception experiment. On each trial, subjects were shown a mongrel version of an image from the rapid perception task. Mongrel images were always presented in the center of the screen, but had been synthesized to simulate the image’s position in the rapid perception task (left of, right of, or at fixation). Subjects responded with a key press to indicate whether or not the mongrel corresponded to the target category for the experimental session (“animal” or “vehicle”). Instruction was otherwise similar to that in the above mongrel experiments. Subjects received feedback after each response. Subjects could study the mongrels for as long as they wished before making a response.
Conflict of Interest Statement
The authors declare that the research was conducted in the absence of any commercial or financial relationships that could be construed as a potential conflict of interest.
Acknowledgments
Thanks to Benjamin Balas and Ted Adelson for useful discussions. This work was supported by NIH grant 1-R21-EY019366-01A1 to Dr. Rosenholtz, an NSF Graduate Fellowship to K. Ehinger, and by Qualcomm and Google.
Footnote
- ^A third part of the strategy for dealing with limited capacity is what digital communications refers to as “channel coding.” This is less relevant for the present discussion, and involves questions of how the system converts the information into a form which can be sent on the physical medium of the channel, be that wires, air, or neurons, in order to minimize transmission error. In the brain, details of spike rates and spike timing fall into this category.
References
Allport, A. (1993). Attention and control: have we been asking the wrong question? Atten. Perform. 14, 183–219.
Alvarez, G. A. (2011). Representing multiple objects as an ensemble enhances visual cognition. Trends Cogn. Sci. (Regul. Ed.) 15, 122–131.
Baars, B. J. (2005). Global workspace theory of consciousness: toward a cognitive neuroscience of human experience. Prog. Brain Res. 150, 45–53.
Balas, B. (2006). Texture synthesis and perception: using computational models to study texture representations in the human visual system. Vision Res. 46, 299–309.
Balas, B. J., Nakano, L., and Rosenholtz, R. (2009). A summary statistic representation in peripheral vision explains visual crowding. J. Vis. 9, 1–18.
Barlow, H. B. (1961). Possible principles underlying the transformation of sensory messages. Sens. Commun. 217–234.
Cavanagh, P. (2004). “Attention routines and the architecture of selection,” in Cognitive Neuroscience of Attention, ed. M. Posner (New York: Guilford Press), 13–28.
Cave, K. R., and Wolfe, J. M. (1990). Modeling the role of parallel processing in visual search. Cogn. Psychol. 22, 225–271.
Cherry, E. C. (1953). Some experiments on the recognition of speech, with one and with two ears. J. Acoust. Soc. Am. 25, 975–979.
Crick, F., and Koch, C. (1990). Towards a neurobiological theory of consciousness. Semin. Neurosci. 2, 263–275.
Crouzet, S. M., Kirchner, H., and Thorp, S. J. (2010). Fast saccades toward faces: face detection in just 100 ms. J. Vis. 10, 16.1–16.17.
Deco, G., and Rolls, E. T. (2004). A neurodynamical cortical model of visual attention and invariant object recognition. Vision Res. 44, 621–642.
Desimone, R., and Duncan, J. (1995). Neural mechanisms of selective visual attention. Annu. Rev. Neurosci. 18, 193–222.
Enns, J. T., and Rensink, R. A. (1990). Influence of scene-based properties on visual search. Science 247, 721–723.
Freeman, J., and Simoncelli, E. P. (2011). Metamers of the ventral stream. Nat. Neurosci. 14, 1195–1201.
Fukushima, K. (1980). Neocognitron: a self-organizing neural network model for a mechanism of pattern recognition unaffected by shift in position. Biol. Cybern. 36, 193–202.
Greene, M. R., and Oliva, A. (2009). Recognition of natural scenes from global properties: seeing the forest without representing the trees. Cogn. Psychol. 58, 137–176.
Greene, M. R., and Wolfe, J. M. (2011). Global image properties do not guide visual search. J. Vis. 11, 18.
Itti, L., and Koch, C. (2001). Computational modeling of visual attention. Nat. Rev. Neurosci. 2, 194–203.
Kirchner, H., and Thorpe, S. J. (2006). Ultra-rapid object detection without saccadic eye movements: visual processing speed revisited. Vision Res. 46, 1762–1776.
Koch, C., and Tsuchiya, N. (2006). Attention and consciousness: two distinct brain process. Trends Cogn. Sci. (Regul. Ed.) 11, 16–22.
Koenderink, J. J. (2011). “Vision as a user interface,” in Human Vision and Electronic Imaging XVI, Vol. 7865, eds B. E. Rogowitz and T. N. Pappas (Bellingham, WA: SPIE Press), 786504-1–786504-13.
Levi, D. M. (2008). Crowding – an essential bottleneck for object recognition: a mini-review. Vision Res. 48, 635–654.
Levin, D. T., and Simons, D. J. (1997). Failure to detect changes to attended objects in motion pictures. Psychon. Bull. Rev. 4, 501–506.
Li, F.-F., VanRullen, R., Koch, C., and Perona, P. (2002). Rapid natural scene categorization in the near absence of attention. Proc. Natl. Acad. Sci. 99, 9596–9601.
Mace, M. J.-M., Joubert, O. R., Nespoulous, J., and Fabre-Thorp, M. (2009). The time-course of visual categorizations: you spot the animal faster than the bird. PLoS ONE 4, e5927. doi:10.1371/journal.pone.0005927
Miller, G. A. (1956). The magical number seven, plus or minus two: some limits on our capacity for processing information. Psychol. Rev. 63, 81–97.
Moray, N. (1959). Attention in dichotic listening: affective cues and the influence of instructions. Q. J. Exp. Psychol. 11, 56–60.
Nakayama, K. (1990). “The iconic bottleneck and the tenuous link between early visual processing and perception,” in Vision: Coding and Efficiency, eds C. Blakemore, K. Adler, and M. Pointon (Cambridge: Cambridge University Press), 411–422.
Oliva, A., and Torralba, A. (2006). Building the gist of a scene: the role of global image features in recognition. Prog. Brain Res. 155, 23–36.
Parkes, L., Lund, J., Angelucci, A., Solomon, J. A., and Morgan, M. (2001). Compulsory averaging of crowded orientation signals in human vision. Nat. Neurosci. 4, 739–744.
Pelli, D. G., and Tillman, K. A. (2008). The uncrowded window of object recognition. Nat. Neurosci. 11, 1129–1135.
Portilla, J., and Simoncelli, E. (2000). A parametric texture model based on joint statistics of complex wavelet coefficients. Int. J. Comput. Vis. 40, 49–71.
Reddy, L., and VanRullen, R. (2007). Spacing affects some but not all visual searches: implications for theories of attention and crowding. J. Vis. 7, 1–17.
Rensink, R. A. (2001). “Change blindness: implications for the nature of attention,” in Vision and Attention, eds M. R. Jenkin, and L. R. Harris (New York: Springer), 169–188.
Rensink, R. A., O’Regan, J. K., and Clark, J. J. (1997). To see or not to see: the need for attention to perceive changes in scenes. Psychol. Sci. 8, 368–373.
Reynolds, J. H., and Heeger, D. J. (2009). The normalization model of attention. Neuron 61, 168–185.
Riesenhuber, M., and Poggio, T. (1999). Hierarchical models of object recognition in cortex. Nat. Neurosci. 2, 1019–1025.
Rosenholtz, R. (1999). A simple saliency model predicts a number of motion popout phenomena. Vision Res. 39, 3157–3163.
Rosenholtz, R. (2000). “Significantly different textures: a computational model of pre-attentive texture segmentation,” in Proceedings of European Conference on Computer Vision, ed. D. Vernon (Dublin: Springer Verlag), 197–211.
Rosenholtz, R. (2011). “What your visual system sees where you are not looking,” in Human Vision and Electronic Imaging XVI, Vol. 7865, eds B. E. Rogowitz and T. N. Pappas 786510-1–786510-14.
Rosenholtz, R., Huang, J., Raj, A., and Balas, B. J. (under review). Mechanisms of peripheral vision explain visual search. J. Vis.
Rousselet, G. A., Joubert, O. R., and Fabre-Thorp, M. (2005). How long to get to the “gist” of real-world natural scenes? Vis. Cogn. 12, 857–877.
Sun, J. Y., and Perona, P. (1996). Preattentive perception of elementary three-dimensional shapes. Vision Res. 36, 2515–2529.
Thorpe, S. J., Fize, D., and Marlot, C. (1996). Speed of processing in the human visual system. Nature 381, 520–522.
Treisman, A. (2003). “Consciousness and perceptual binding,” in The Unity of Consciousness: Binding, Integration, and Dissociation, ed. A. Cleeremans (New York: Oxford University Press), 95–113.
Treisman, A. (2006). How the deployment of attention determines what we see. Vis. Cogn. 14, 411–443.
Treisman, A., and Gelade, G. (1980). A feature-integration theory of attention. Cogn. Psychol. 12, 97–136.
Treisman, A., and Schmidt, H. (1982). Illusory conjunctions in the perception of objects. Cogn. Psychol. 14, 107–141.
Tsotsos, J. K., Culhane, S. M., Wai, W. Y. K., Lai, Y., Davis, N., and Nuflo, F. (1995). Modeling visual attention via selective tuning. Artif. Intell. 78, 507–545.
VanRullen, R., Reddy, L., and Koch, C. (2004). Visual search and dual-tasks reveal two distinct attentional resources. J. Cogn. Neurosci. 16, 4–14.
VanRullen, R., and Thorpe, S. J. (2001). Is it a bird? Is it a plane? Ultra-rapid visual categorization of natural and artifactual objects. Perception 30, 655–668.
Wolfe, J. M. (2007). “Guided search 4.0: current progress with a model of visual search,” in Integrated Models of Cognitive Systems, ed. W. Gray (New York: Oxford), 99–119.
Wolfe, J. M., Cave, K. R., and Franzel, S. L. (1989). Guided search: an alternative to the feature integration model for visual search. J. Exp. Psychol. Hum. Percept. Perform. 15, 419–433.
Wyart, V., and Tallon-Baudry, C. (2008). Neural dissociation between visual awareness and spatial attention. J. Neurosci. 28, 2667–2679.
Keywords: selective attention, limited capacity, search, scene perception, model, peripheral vision, compression
Citation: Rosenholtz R, Huang J and Ehinger KA (2012) Rethinking the role of top-down attention in vision: effects attributable to a lossy representation in peripheral vision. Front. Psychology 3:13. doi: 10.3389/fpsyg.2012.00013
Received: 15 October 2011;
Paper pending published: 16 November 2011;
Accepted: 11 January 2012;
Published online: 06 February 2012.
Edited by:
Naotsugu Tsuchiya, RIKEN, JapanReviewed by:
Guillaume A, Rousselet, University of Glasgow, UKRyota Kanai, University College London, UK
Copyright: © 2012 Rosenholtz, Huang and Ehinger. This is an open-access article distributed under the terms of the Creative Commons Attribution Non Commercial License, which permits non-commercial use, distribution, and reproduction in other forums, provided the original authors and source are credited.
*Correspondence: Ruth Rosenholtz, 32 Vassar St., D32-532, Cambridge, MA, USA. e-mail: rruth@mit.edu