- 1 Roxelyn and Richard Pepper Department of Communication Sciences and Disorders, Northwestern University, Evanston, IL, USA
- 2 Department of Music, University of Arkansas, Fayetteville, AR, USA
- 3 Department of Otolaryngology – Head and Neck Surgery, Feinberg School of Medicine, Northwestern University, Chicago, IL, USA
Research on music and language in recent decades has focused on their overlapping neurophysiological, perceptual, and cognitive underpinnings, ranging from the mechanism for encoding basic auditory cues to the mechanism for detecting violations in phrase structure. These overlaps have most often been identified in musicians with musical knowledge that was acquired explicitly, through formal training. In this paper, we review independent bodies of work in music and language that suggest an important role for implicitly acquired knowledge, implicit memory, and their associated neural structures in the acquisition of linguistic or musical grammar. These findings motivate potential new work that examines music and language comparatively in the context of the implicit memory system.
Introduction
Music has been called the universal language of mankind (Longfellow, 1835) reflecting longstanding curiosity on the relationship between music and language. Both share many traits including being perceived primarily through the auditory system, having similar acoustic attributes and reflecting analogous generative syntactic systems. This has led to decades of scientific research, exemplified by the papers included in this volume, exploring their overlapping neurophysiological, perceptual, and cognitive underpinnings. These range from the mechanism for encoding basic auditory cues (Wong et al., 2007; Kraus and Chandrasekaran, 2010), to mechanisms supporting acquisition (Slevc and Miyake, 2006; Schön et al., 2008) to the mechanism for detecting violations in predicted structure (Slevc et al., 2009).
Much of this research with respect to music has made use of trained musicians, in part to look for evidence that the cognitive and neural correlates of specialization for music are similar to the human specialization for language (e.g., Besson and Faita, 1995; Patel et al., 1998a; Maess et al., 2001; Schön et al., 2008; Kraus and Chandrasekaran, 2010). While using trained musicians has led to great strides in our understanding of how music is processed, it has obscured another important similarity between music and language: both may be acquired implicitly, without the aid of explicit instruction. In this paper, we review independent bodies of research exploring the role of implicitly acquired knowledge and associated neural structures in the acquisition of language and musical grammar.
We first consider the role of implicit memory in language by looking at both natural and artificial language learning studies. The studies discussed in the Section “Implicit Memory and Language” show that the implicit memory system plays an important role in acquiring the grammar, or rules, of language at all levels of linguistics structure (Table 1). Similarly, implicit learning in music is found in the acquisition of rhythm, pitch, and melodic structures. The studies discussed in the Section “Implicit Memory and Music” suggest a potentially common learning mechanism shared by both music and language that allows for the acquisition of these complex systems without the need for instruction (Table 1).
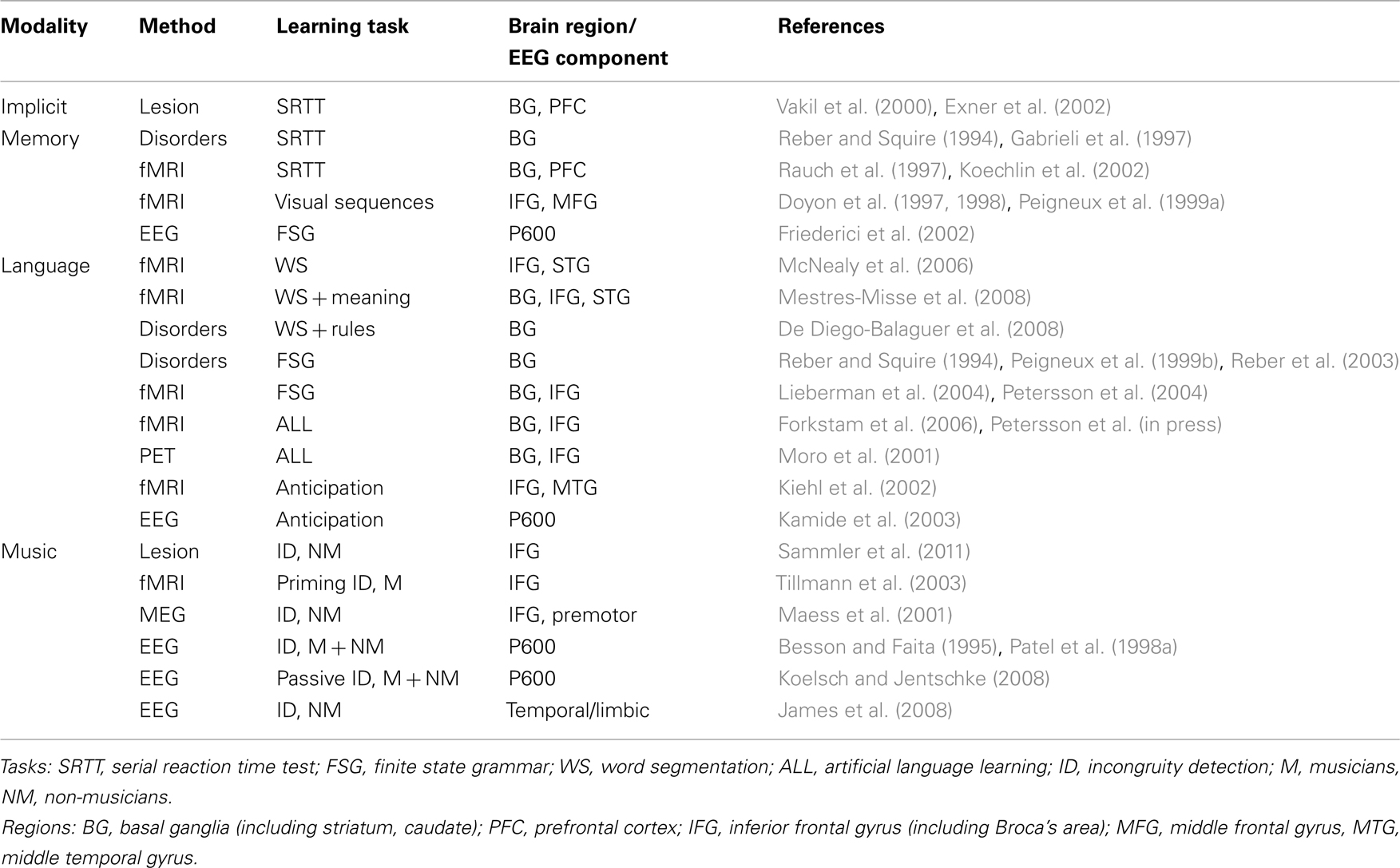
Table 1. Summary of representative neurological findings associating implicit memory, language, and music.
The studies we discuss below help us understand this mechanism by highlighting the fact that both music and language involve expectation and the tracking of dependencies between sequential elements. Neurally, there is a significant three-way overlap of the brain structures implicated in implicit memory and those involved in learning language and learning music. This convergence encourages new work that juxtaposes music and language in the context of the implicit memory system. Given the known relationship between dopamine and the implicit memory system, we may also consider more directly the genomic and molecular bases of music and language abilities.
Implicit Memory
Implicit memory is generally defined as acquired knowledge that is not available to conscious access (Schacter and Graf, 1986; Schacter, 1987). This contrasts with explicit memory, which is characterized by knowledge that involves conscious recollection, recall, or recognition. The majority of behavioral evidence for an implicit memory system is based on experiments wherein experience leads to altered performance on some task without participants being aware of having learned anything.
One type of implicit memory stems from perceptual learning, which involves changes to the perceptual system and to perceptual categories (e.g., phonemes, chords) due to experience. For example, in one study (Wade and Holt, 2005), participants played a video game that involved navigating through a maze. A non-critical feature of the game was that certain non-speech auditory cues were associated with certain events. After playing the game, participants were better able to distinguish the sounds and reliably learned the sound-event patterns. Importantly, learning was qualitatively different, and in some cases better, than explicit training on these same patterns. While explicit attention has been shown to facilitate this sort of perceptual learning (e.g., Ranganath and Rainer, 2003) it is also well established that perceptual learning can be subliminal and implicit (Goldstone, 1998; Seitz and Watanabe, 2003).
Another type of implicit memory involves the implicit learning of sequences (e.g., sentences, melodies). A commonly used paradigm to test implicit memory for sequences is the serial reaction time test (SRTT; Nissen and Bullemer, 1987). In this test, participants are exposed to some stimuli (e.g., objects appearing sequentially at different points on a screen) cuing participants to respond (e.g., by indicating where the stimuli appear) as quickly as possible. While the sequences of stimuli appear to be random to the participant, embedded within the random sequences is a fixed pattern, repeatedly interspersed throughout the random sequences. Over the course of the experiment, response times and accuracy on the fixed sequences improves relative to the random sequences, presumably because the participants are learning this repeated sequence. Crucially, participants do not exhibit an improved ability to explicitly recall this repeated sequence as compared to recalling random non-repeated sequences. The fact that participants show implicit learning without explicit knowledge suggests that these memory systems can operate independently, and that people can learn about the sequencing of some stimuli without being explicitly aware of it. While implicit memory is relevant for both sequence learning and category learning and both sequence learning and category learning are relevant to language and music, we focus primarily on implicit memory in the context of sequence learning.
A more specific kind of implicit sequence learning often discussed in the context of language and music is statistical learning (e.g., Saffran et al., 1996a). Statistical learning involves the same basic idea that participants can learn sequences without explicit awareness, but adds an additional component of tracking statistics over these sequences1. For example, in a series of studies, Saffran et al. (1996a,b, 1999) showed that adults, children, and infants are able to track transitional probabilities between syllables and tones. Participants were exposed to seemingly random sequences of syllables obscuring consistent differences in the probability that certain syllables followed others (see below for more details). Participants were sensitive to these differences in transitional probability, and subsequent work has explored what types of statistics and what types of dependencies can be implicitly tracked (Knowlton and Squire, 1996; Aslin et al., 1998; Gomez, 2002).
More recently, neurological studies have, for the most part, supported this dissociation between implicit and explicit memory systems for tasks like the SRTT (Curran, 1997) and other similar sensory–motor learning tasks. Evidence includes both lesion studies (Vakil et al., 2000; Exner et al., 2002; Peach and Wong, 2004) and functional imaging (Rauch et al., 1997; Koechlin et al., 2002) and implicate the striatum, and more specifically the caudate, in implicit learning. For example, Alzheimer’s patients, characterized by degeneration of the medial temporal lobe, have little trouble with the SRTT despite exhibiting problems with declarative memory, while learning in the same task is impaired for people with diseases characterized by degeneration of the basal ganglia, including in Parkinson’s patients (Reber and Squire, 1994; Jackson et al., 1995) and Huntington’s patients (Gabrieli et al., 1997). More generally, the basal ganglia have been implicated in implicit learning across a number of different tasks (Squire and Knowlton, 2000; Eichenbaum and Cohen, 2001). In addition to implicit memory, the basal ganglia, and the caudate specifically, have also been implicated in motor learning (Knowlton et al., 1996), general learning plasticity (Graybiel, 2005) and learning from feedback (Packard and Knowlton, 2002). There is also some evidence that the inferior frontal gyrus, and in particular, Broca’s area and its right homolog, are also involved in learning sequences (Doyon et al., 1997, 1998; Peigneux et al., 1999a). More generally, Broca’s area has also been associated with a wide range of linguistic functions (Grodzinsky and Santi, 2008) including hierarchical processing (Musso et al., 2003), recursion, binding (Hagoort, 2005), and speech articulation (see Bookheimer, 2002 for a review).
Finally, by virtue of the fact that dopamine receptors are found in the basal ganglia, and in particular, the striatum (which includes the caudate, putamen, and nucleus accumbens), implicit memory has been associated with dopamine. This has been supported by studies showing that increasing dopamine levels in the brain can lead to improved implicit learning (de Vries et al., 2010b), that dopamine deficiencies, as in Parkinson patients, result in poor implicit learning though explicit learning is intact (Shohamy et al., 2009), and that dopaminergic neurons in primates show a burst of activity when learning implicitly (see Shohamy and Adcock, 2010 for a review).
Implicit Memory and Language
Language learning shares a number of important similarities with the learning of sensory–motor sequences, which have been classically associated with implicit memory and which, as will be discussed below, are also implicated in acquiring a musical system. As with the tasks used in implicit learning experiments (e.g., the SRTT), people are often unaware of, or unable to articulate many of the rules of their language (Fodor, 1983). People can also learn language without any explicit instruction (Chomsky, 1957). This is particularly true before school age when children learn language with relative ease (Lenneberg, 1967), which has been argued to be, in part, due to children’s good implicit memory capacity as compared to adults (DiGiulio et al., 1994; DeKeyser and Larson-Hall, 2005). Finally, certain aspects of linguistic knowledge, namely the rules of combination, may be represented probabilistically or as information about the distributional relationships at different levels of linguistic structure (e.g., phonemes, morphemes, words, and sentences; Redington and Chater, 1997). This knowledge is generally not consciously accessible to speakers of a language and is similar in nature to the probabilistic knowledge acquired in implicit learning.
Implicitly Learned Artificial Grammars
The use of implicitly learned distributional information for language learning has been demonstrated at many different levels of linguistic structure. For example, at the level of word segmentation, Saffran et al. (1996a) exposed 8-month-old infants to a stream of running speech consisting of four three-syllable words without any breaks or pauses indicating word-hood. Thus, the only cue to word segmentation was the transitional probability between syllables, where within-word transitional probability of syllables was 1.0 and between-word transitional probability of syllables was 0.33 (no word followed itself). Infants showed a significant ability to discriminate words from part-words (formed by combining the final syllable from one word with the first two syllables of another). Adults performed similarly (Saffran et al., 1996b) in what is argued to reflect implicit learning of word segmentation (Evans et al., 2009). Importantly, this ability is suggested to be domain general as it also applies to tones (Saffran et al., 1999 and below in the discussion on implicit memory and music) and visual stimuli (Fiser and Aslin, 2001).
Analogous behavior is also found with respect to the acquisition of phonotactics. Phonotactics are the restrictions on where phonemes can occur in a word in a language (e.g., English prohibits ng starting a word or h ending one). In one study (Onishi et al., 2002), adults briefly exposed to pseudo-words reflecting some non-English phonotactic generalization showed speeded repetition to words that adhered to the generalization as compared to words that did not.
Another study on implicit phonotactic learning (Dell et al., 2000) found that when participants are tasked with repeating sets of words reflecting some phonotactic generalization, their speech errors tend to reflect these newly learned generalizations, as is true of one’s native language. The authors assessed the implicitness of learning using something they call the “ask-tell technique.” This involved asking all participants whether they had noticed anything about the words they were pronouncing; the experimenters also told half the participants, explicitly, what the phonotactics would be before starting. Neither the uninformed nor informed participants were able to identify any regularities in the experimental materials. These results, in addition to the fact that the speech errors were not intentional, suggest that this learning is, in fact, implicit.
Another important component of learning the phonology of a language, acquiring phonological rules, has been shown to relate to non-linguistic implicit learning as well (Ettlinger et al., in press). In this study, participants took both an artificial grammar learning experiment and a test of implicit learning. The artificial grammar learning task involved exposure to words that reflected a set of rules for forming plural and diminutive variants (e.g., dog, dogs, doggie, doggies). The test of implicit learning was a modified version of the Tower of London task (Shallice, 1982). In this task, participants were required to solve puzzles, increasing in difficulty, which involved virtually moving colored balls on three sticks to match a predetermined pattern. Embedded within the puzzles were repeated sequences of moves, and participants were asked to think through their moves before starting, to minimize the effects of motor coordination, unlike the SRTT. Implicit learning was measured by looking at the improvement in performance on the repeated sequences (Phillips et al., 1999). Results showed a strong correlation between learning the artificial language and performance on the Tower of London task, suggesting that implicit memory and language learning are linked.
In another set of experiments exploring the possible implicit learning of syntactic structure, Reber (1967) taught participants an artificial finite state grammar for sequences of letters (Figure 1). After exposure to strings of letters generated by the grammar, participants were asked to judge the grammaticality of novel sequences of letters. Participants were able to successfully distinguish what constituted a valid sequence without being able to explicitly describe the rules of the grammar.
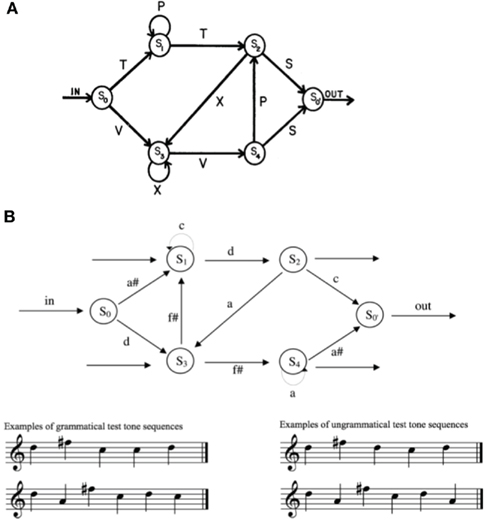
Figure 1. Examples of finite state grammars used in language (A) and music (B) learning experiments. (A) is a finite state grammar used to generate sequences of letters that participants are exposed to in implicit language learning experiments (from Reber, 1967); (B) shows a similar structure, using notes instead of letters (from Tillmann and Poulin-Charronnat, 2010). Participants can acquire grammars of this sort and identify valid versus invalid sequences without being explicitly aware of any specific aspects of the grammar for both music and language.
In addition to these associative studies, more concrete evidence on the role of implicit memory in language learning is provided by recent imaging studies.
McNealy et al. (2006) adapted a version of Saffran et al.’s (1996) word-segmentation paradigm for functional imaging by presenting participants with three speech streams: one containing no regularities, one containing the statistical regularities as detailed above, and a third containing statistical regularities plus a standard phonetic word-segmentation cue. Greater activation was found in inferior frontal gyrus for the statistical cue and statistical cue plus phonetic cue conditions as compared to the random condition. Additional activation was found in the superior temporal gyrus, which is associated with the processing of speech (Geschwind, 1970). Another study found that when word meaning is introduced to this experimental paradigm, greater activation is also found in the basal ganglia, specifically the caudate (Mestres-Misse et al., 2008), plus the thalamus, which serves as a relay between subcortical (e.g., basal ganglia) and cortical networks and is involved in sensory perception (Steriade et al., 1997). This has led to the hypothesis that the basal ganglia, and therefore, presumably, implicit memory, is important for the integration of multiple information sources during the process of language learning (Rodriguez-Fornells et al., 2009). Similarly, patients with early stage Huntington’s disease and striatal damage also do poorly on tasks of this sort (De Diego-Balaguer et al., 2008).
As with the SRTT and word segmentation, a fronto-striatal network is implicated in acquiring the finite state grammars described above. Alzheimer’s and amnesic patients, with degeneration or lesions of the temporal cortices can still successfully learn artificial grammars of this sort while having trouble with more explicit language tasks (Reber and Squire, 1994; Knowlton and Squire, 1996; Reber et al., 2003). The ability of Parkinson’s patients with degeneration of the basal ganglia to learn artificial grammars is less clear, however, with conflicting evidence present (Peigneux et al., 1999b; Witt et al., 2002). Similar findings are found using functional imaging, with the basal ganglia, and inferior frontal gyrus supporting the acquisition of implicit knowledge of an underlying pattern governing a sequence of letters, while the medial temporal lobe supports the recall of specific sequences (Lieberman et al., 2004; Petersson et al., 2004). Activation of the caudate is also found in a study of syntactic processing (Moro et al., 2001). In this latter study, participants were exposed to a version of Italian (the participants’ native language) where all content words were replaced with pseudo-words, with function words left intact, which served to eliminate any semantic component of processing. Syntactic (word order), morphological (determiner agreement), and phonotactic violations were juxtaposed using PET. The results reflected Broca’s and right IFG activation for the morphological and syntactic conditions, which has long been associated with syntactic processing (Embick et al., 2000; Grodzinsky, 2000) and may be part of a basal ganglia thalamocortical circuit (Ullman, 2006). Greater activation was also found in the left caudate, which is associated with implicit memory (see above). This result had been replicated a number of times with different types of artificial syntactic grammar, with activation consistently found in Broca’s area and the caudate (Forkstam et al., 2006; Petersson et al., in press).
Implicit Learning and Natural Language
With respect to language learning in more ecologically valid settings, a few behavioral studies have shown a relationship between natural language processing and implicit learning. Misyak et al. (2010) created an implicit learning task that combined the SRTT and artificial grammar learning and showed that performance correlated with participants’ ability to comprehend complex English sentences. Evans et al. (2009) looked at children with specific language impairment, ages five to seven, and showed that these children also performed worse on the word-segmentation task from Saffran et al. (1996) as compared to a control group with the same non-verbal IQ. They conclude that specific language impairment may not, in fact, be specific to language, but rather reflects an impairment of implicit learning, which is crucial for language learning but distinct from other measures of intelligence (see also Kaufman et al., 2010 for a similar view). Furthermore, performance on the word-segmentation task correlated with vocabulary size within each participant group, suggesting implicit learning facilitates word learning. Finally, research looking into language processing in more realistic settings has also considered language processing in noise (e.g., Wong et al., 2008, 2009a, 2010; Harris et al., 2009). In particular, Conway et al. (2010) showed a relationship between an ability to perceive speech in noise and implicit sensory–motor sequence learning. Participants who were good at an SRTT-like task were similarly good at perceiving sentences embedded in noise when the last word in the sentence had high-predictability (e.g., Her entry should win first prize), even when controlling for working memory and intelligence. The correlation disappeared for sentences ending in low-predictability words (e.g., The arm is riding on the beach).
This suggests that an important way in which implicit memory is related to language is through prediction and anticipation. A number of studies using eye-tracking (see Kamide, 2008 for a review) and event-related potentials (ERPs; see Van Berkum, 2008 for a review) have shown that people make significant use of context to facilitate processing. For example, participants look more often at a picture of beer than a doll when hearing the beginning of the sentence the man will taste the… (Kamide et al., 2003). A violation of an anticipated sentence completion will also yield a specific ERP response, either N400 for semantic incongruency or P600 for syntactic. The same ERP response is elicited on encountering anomalies in predicted outcome for artificial grammars similar to Reber (1967, above; Friederici et al., 2002) and music (Patel et al., 1998a).
Additional neural evidence comes from functional imaging, showing a significant overlap in the brain regions associated with implicit memory and language. As mentioned above, Broca’s area has been implicated in implicit memory tasks, and Broca’s area has a longstanding association with language learning and language processing (Embick et al., 2000; Grodzinsky, 2000; Sahin et al., 2009). Broca’s area has also been implicated in prediction, and the expectations that yield the N400 or P600, above, result in activation of the bilateral inferior frontal gyrus (i.e., Broca’s area, nearby regions and their right homologs) in addition to the middle temporal gyrus (Kiehl et al., 2002).
Thus, there is a wide range of similarities between language and implicit knowledge both in terms of their neural substrates (the fronto-striatal system) and in their cognitive underpinnings (sequential knowledge, expectation). These similarities have motivated myriad theories in linguistic processing positing that the dissociation between the words and rules of a language is homologous to the dissociation of explicit and implicit memory, respectively (Paradis, 1994, 2009; Pinker, 1999; Pinker and Ullman, 2002). Evidence for this dissociation is discussed below, and is based on the idea that we can explicitly recall and conceptualize the words of our language, which is declarative in nature, whereas the application of the rules of language (when speaking naturally, as contrasted with attempting to adhere to a style guide, for example) is generally more difficult, if not impossible to articulate.
To conclude, there is extensive and convergent evidence for a close relationship between the cognitive and neurophysiological underpinnings of language learning and implicit memory. Language learning involves cognitive abilities that are generally learned implicitly, including tracking dependencies and developing expectations regarding adjacent linguistic structures. Language and implicit memory are also both supported by a set of neural structures including the anterior portion of the inferior frontal gyrus and the basal ganglia. As will be reviewed below, music shares many of these same associations with implicit memory and these shared associations are not restricted to musicians with formal musical training, but extend to everyday music listeners.
Implicit Memory and Music
Although music is sometimes held to be the domain of specialists, its near-ubiquity in daily life, from mp3 players to Internet radio, cinema, and advertising, shows that affinity for music is widespread. Indeed, music has frequently been postulated by anthropologists to be a human universal, present in all known cultures (Blacking, 1973; Zatorre and Peretz, 2001). Although the ability to perform music skillfully is not evenly distributed and often relies on years of formal training, the ability to listen, process, and respond emotionally to music is shared across most of the population and seems to depend only on implicit exposure. For example, Bigand et al. (2005) showed that people with and without formal training responded largely interchangeably to non-vocal classical music. Other deep musical abilities in people without explicit training, such as the ability to perceive the relationship between a theme and its variations and to learn new compositional systems, are chronicled in Bigand and Poulin-Charronnat (2006). With little to no explicit training, how is it possible for people to develop the ability to represent and respond appropriately to the complex syntactic structures of music?
Desain and Honing (1999) demonstrate that even a seemingly simple and near-universal ability like tapping to a beat depends on complex internal representations of harmonic and syntactic musical structures. Indeed, research summarized in Krumhansl (1990) shows that implicit exposure to Western tonal music is sufficient for listeners to develop internal representations of the pitch relationships that music theorists hold to underlie tonality. Given a tonal context, such as a scale or chord progression, listeners without formal training can accurately judge how well a given continuation fits the established tonality.
One of the mechanisms by which passive exposure can ultimately yield sophisticated internal representations is statistical learning. Saffran et al. (1999) constructed long isochronous tone sequences out of 6 three-note “figures” repeated in random order, with no breaks or other indication of boundaries between the figures, and constrained so that the same figure never appeared twice in succession. When infants were exposed to this series of tones over a 20-min period, they were able to abstract the constituent three-note figures, despite the fact that nothing but the reduced transition probabilities between them delineated the figures in the continuous stream of the musical surface. The infants, it seemed, had carefully tracked continuation probabilities in the sequence, despite the fact that their exposure to it was entirely passive. This ability to track common outcomes in musical repertoires may seem arbitrary, but in fact has been held by music theorists and psychologists since Meyer (1956) to form the basis of affective responses to music (see Huron and Margulis, 2010 for a summary). Continuations that are recognized, even implicitly, as unusual are thought to result in perceptions of special expressivity or esthetic charge. In this way, the ability to implicitly track statistics about continuations may form the fundamental scaffolding for the widespread ability to respond emotionally to music, even in the absence of formal training.
Implicit memory for music also reveals itself in various well-documented priming effects. Priming is generally defined as an implicit memory effect in which exposure to a stimulus influences responses to later stimulus without awareness of or an ability to recall the specific prime (Tulving et al., 1982). For example, Hutchins and Palmer (2008) showed that participants were more accurate in singing back the last tone of a short melody if that tone had appeared previously in the melody. Musical priming can also evidence itself in the form of faster and more accurate judgments about pitches or chords that are normative and expected given the tonal context. This kind of tonal priming has been documented in responses to melodic continuations (Margulis and Levine, 2006), and harmonic continuations (Bigand and Pineau, 1997) by listeners with no formal training. fMRI studies have implicated suppressed activity in bilateral inferior frontal regions of the brain during harmonic priming (Tillmann et al., 2000, 2003). It has even been documented in children (Schellenberg et al., 2005). Bharucha and Stoeckig(1986, 1987) provide evidence that harmonic priming is cognitive (based on the implicit abstraction of regularities in the musical environment) rather than sensory (based on psychoacoustic relationships) in nature. Tillmann et al. (2000) propose a self-organizing network model that can account for the kind of implicit learning of tonal structure revealed by priming studies. These priming effects are also observed to reflect the acquisition of musical grammars implicitly learned in the same fashion as in the implicit language learning experiments above (Figure 1; Tillmann and Poulin-Charronnat, 2010).
It is not only continuation statistics that listeners track implicitly. Duple and quadruple meters are more common than triple meters in Western music, and Brochard et al. (2003) confirmed that when presented with an ambiguous stimulus, listeners assume a binary division of the beat. Relatedly, the major mode is more common than the minor mode in Western music, and Huron (2006) confirmed that when presented with an ambiguous stimulus, listeners assume the major mode. And although absolute pitch perception is restricted to a tiny fraction of the population, Levitin (1994) demonstrated that ordinary listeners generally sing familiar songs within a semitone or so of their actual pitch level, suggesting that people have some implicit sense of pitch even in the absence of formal training on scales, producing notes, performing in key, or tuning an instrument. It is clear that mere exposure, independent of formal training, or active use (such as performance or participation) is sufficient to engender highly structured and highly specific memory traces in ordinary listeners.
Implicit memory for music emerges consistently in preference effects. Halpern and O’Connor (2000) showed that although explicit recognition memory for melodies deteriorated with age, implicit memory was retained, in the form of elevated preference (the mere exposure effect first documented in Zajonc, 1968). A battery of studies over the past several decades (summarized nicely in Szpunar et al., 2004) illustrate that listeners’ preference increases for music that has been encountered before. This effect is even stronger for music that is complex or ecologically valid (Bornstein, 1989). Halpern and Mullensiefen (2008) exploit this preference toward previously encountered music as a measure of implicit memory, showing that when melodies that are encountered in an exposure phase are later replayed in new timbres, participants continue to report increased liking for them, even when explicit memory of the music is obscured (i.e., the timbre change prevented them from recognizing explicitly that they had heard the excerpts before). Similarly, Peretz et al. (1998) found that explicit recognition memory was more susceptible to decay over time than implicit memory measured by elevated preference. They concluded that, in contrast with explicit memory, implicit memory as manifested in affective judgments operates obligatorily, in an automatic and unconscious fashion. Samson and Peretz (2005) further conclude, based on an analysis of patients with temporal lobe lesions on either the right or left side, that the right temporal lobe is more active in the formation of representations that underlie implicit musical memory, and the left temporal lobe is more active in processes related to explicit retrieval of musical memories.
In addition to the implicit learning of normative patterns in a particular musical style, many people are able to gain competence in more than one musical system through mere passive exposure, independent of any experience performing or producing the sound, as well as independent of any explicit instruction (formal musical training) about the style. Wong et al. (2009b) illustrate that passive exposure to the music from two cultures can result in the development of true bimusicals who approach both styles with affective and cognitive competence lacking in monomusicals of similar age and background. Wong et al. (in press) used structural equation modeling to investigate fMRI data from bimusical and monomusical listeners, finding more connectivity, and larger differentiation between the musical systems in bimusicals. These differences imply that even the implicit learning of multiple musical systems can result in fundamental changes to the way the brain approaches expressive sound.
Electrophysiological evidence also supports this conclusion. Violations of expected harmonic, melodic, and rhythmic patterns result in a late positive component (LPC) characteristic of the detection of an incongruity, even when the participants lacked formal training and were unable to explicitly identify the surprises (Besson and Faita, 1995). The elicitation of ERP components related to syntactic violations in music seem to be independent of the task relevance of unexpected chords, and provides strong evidence for important implicit components to musical ability (Koelsch et al., 2000). Patel et al. (1998b) were the first to show that the P600 – a known marker of syntactic violations in language – extended to syntactic violations in music grammars that are abstracted implicitly by listeners. Generally, these responses have been found even when the musical exposure is entirely passive, as in Koelsch and Jentschke (2008), when participants were watching a silent movie. Koelsch (2010) emphasizes that the early right anterior negativity (ERAN) that emerges in response to syntactic violations in music depends on the long-term extraction of statistical regularities in music, not from short-term exposure to particular sequences.
Predictions based on these abstractions of musical syntax are thought to be localized in the premotor cortex and the inferior frontal gyrus (particularly Broca’s area). Evidence for localization to the IFG comes from MEG (Maess et al., 2001), fMRI (Tillmann et al., 2003), and lesion studies (Sammler et al., 2011) exploring participants’ responses to ungrammatical or incongruent musical stimuli (see Koelsch, 2006 for a review). There is also some evidence that the source of the ERP component responding to expectation violation may have origins in the right temporal–limbic areas, which is associated with affect and emotive processing (James et al., 2008).
The processing of syntactic violations in music has also been shown to interfere with the processing of syntactic violations in language, suggesting overlap for these two functions. When participants read garden path sentences while hearing chord progressions, they took longer to process syntactically unexpected words when they appeared at the same time as syntactically unexpected harmonies; however, no such interference occurred when the musical surprise was not syntactic in nature (e.g., when a chord sounded in a different timbre; Slevc et al., 2009). So, implicit memory seems to play an important role in syntactic processing in both language and music.
Implicit Memory in Language and Music
We have reviewed above independent sets of empirical studies implicating the role of the implicit memory system in music and language, summarized in Table 1. In particular, we have discussed the fact that explicit training is not required for processing of language or music. It is important to note that these studies examined music or language alone. To ascertain common pathways in processing and/or representation, music and language should be examined in tandem. In terms of processing, studies could be conducted such as those performed by Patel and Slevc and colleagues (Patel et al., 1998b; Slevc et al., 2009) in which musical and linguistic stimuli were combined. However, it is preferable that everyday music listeners should be examined to ascertain that the results are not due to formal musical training alone or trained musicians possessing a genetic difference.
Studies examining the dependence and independence of musical and linguistic functions sometimes yield conflicting results. In particular, the lesion literature favors independence while studies on neurologically normal subjects favor dependence. It is beyond the scope of this proposal to extensively discuss the nature of this debate, except to mention that a reconciliation has been proposed by imposing a distinction between representation and processing at least for syntax (Patel, 2008). In his Shared Syntactic Integration Resource Hypothesis, Patel (2003) postulates that while musical and linguistic syntactic representations are maintained separately, the processing of both musical and linguistic syntactic structures overlapped in neural resources. While the processing aspect of this hypothesis has much support (Patel et al., 1998b) and is conceivably more feasible to test, representations are difficult to examine. However, neural repetition-suppression/enhancement paradigms have been used recently to examine mental representations in humans (Grill-Spector et al., 2006) and can potentially be used to test whether musical and linguistic representations overlap in neural regions. More specifically related to the implicit memory system, we believe such experiments could be conducted with both music and language studied side-by-side.
Major divisions of the dopaminergic system contain neurons from the substantia nigra pars compacta and ventral tegmental area projecting to divisions of the striatum and prefrontal cortex, and other regions (see Seamans and Yang, 2004 for a review). As discussed above, these brain regions are also associated with the implicit memory system. Recent studies in humans, including pharmacological (de Vries et al., 2010b), molecular imaging (e.g., McNab et al., 2009), and genomic (e.g., Klein et al., 2007a,b) studies have examined the role of dopamine and related genes in a variety of implicit behaviors, such as acquiring an artificial grammar (de Vries et al., 2010a) and learning from feedback in a statistical learning paradigm (Klein et al., 2007b). Future research into the role of the implicit memory system in music and language could employ similar methods to more directly examine their potentially shared molecular neurobiological mechanisms.
Conflict of Interest Statement
The authors declare that the research was conducted in the absence of any commercial or financial relationships that could be construed as a potential conflict of interest.
Acknowledgments
The authors would like to thank Lionel Newman for assistance in editing this manuscript. Support provided by grant T32 NS047987 to Marc Ettlinger and NIH grants R01DC008333, R21DC007468, and NSF BCS-1125144 to Patrick C. M. Wong.
Footnote
- ^“Statistical learning” is also sometimes used to refer to certain types of perceptual learning (e.g., Maye et al., 2002). Here, we use it to refer to sequence-based learning only.
References
Aslin, R. N., Saffran, J. R., and Newport, E. L. (1998). Computation of conditional probability statistics by 8-month-old infants. Psychol. Sci. 9, 321–324.
Besson, M., and Faita, F. (1995). An event-related potential (ERP) study of musical expectancy: comparison of musicians with nonmusicians. J. Exp. Psychol. Hum. Percept. Perform. 21, 1278–1296.
Bharucha, J. J., and Stoeckig, K. (1986). Reaction time and musical expectancy: priming of chords. J. Exp. Psychol. Hum. Percept. Perform. 12, 403–410.
Bharucha, J. J., and Stoeckig, K. (1987). Priming of chords: spreading activation or overlapping frequency spectra? Percept. Psychophys. 41, 519–524.
Bigand, E., and Pineau, M. (1997). Global context effects on musical expectancy. Percept. Psychophys. 59, 1098–1107.
Bigand, E., and Poulin-Charronnat, B. (2006). Are we “experienced listeners”? A review of the musical capacities that do not depend on formal musical training. Cognition 100, 100–130.
Bigand, E., Vieillard, S., Madurell, F., Marozeau, J., and Dacquet, A. (2005). Multidimensional scaling of emotional responses to music: the effect of musical expertise and of the duration of the excerpts. Cogn. Emot. 19, 1113–1139.
Bookheimer, S. (2002). Functional MRI of language: new approaches to understanding the cortical organization of semantic processing. Annu. Rev. Neurosci. 25, 151–188.
Bornstein, R. F. (1989). Exposure and affect: overview and meta-analysis of research, 1968–1987. Psychol. Bull. 106, 265–289.
Brochard, R., Abecasis, D., Potter, D., Ragot, R., and Drake, C. (2003). The “ticktock” of our internal clock: direct brain evidence of subjective accents in isochronous sequences. Psychol. Sci. 14, 362–366.
Conway, C. M., Bauernschmidt, A., Huang, S. S., and Pisoni, D. B. (2010). Implicit statistical learning in language processing: word predictability is the key. Cognition 114, 356–371.
Curran, T. (1997). Higher-order associative learning in amnesia: evidence from the serial reaction time task. J. Cogn. Neurosci. 9, 522–533.
De Diego-Balaguer, R., Couette, M., Dolbeau, G., Durr, A., Youssov, K., and Bachoud-Levi, A. C. (2008). Striatal degeneration impairs language learning: evidence from Huntington’s disease. Brain 131, 2870–2881.
de Vries, M. H., Barth, A. C. R., Maiworm, S., Knecht, S., Zwitserlood, P., and Flöel, A. (2010a). Electrical stimulation of Broca’s area enhances implicit learning of an artificial grammar. J. Cogn. Neurosci. 22, 2427–2436.
de Vries, M. H., Ulte, C., Zwitserlood, P., Szymanski, B., and Knecht, S. (2010b). Increasing dopamine levels in the brain improves feedback-based procedural learning in healthy participants: an artificial-grammar-learning experiment. Neuropsychologia 48, 3193–3197.
DeKeyser, R., and Larson-Hall, J. (2005). “What does the critical period really mean?” in Handbook of Bilingualism: Psycholinguistic Approaches, eds. J. F. Kroll, and A. M. B. De Groot (New York, NY: Oxford University Press), 88–108.
Dell, G. S., Reed, K. D., Adams, D. R., and Meyer, A. S. (2000). Speech errors, phonotactic constraints, and implicit learning: a study of the role of experience in language production. J. Exp. Psychol. Learn. Mem. Cogn. 26, 1355–1367.
Desain, P., and Honing, H. (1999). Computational models of beat induction: the rule-based approach. J. New Music Res. 28, 29–42.
Digiulio, D. V., Seidenberg, M., O’Leary, D. S., and Raz, N. (1994). Procedural and declarative memory: a developmental study. Brain Cogn. 25, 79–91.
Doyon, J., Gaudreau, D., Laforce, R. Jr., Castonguay, M., Bedard, P. J., Bedard, F., and Bouchard, J. P. (1997). Role of the striatum, cerebellum, and frontal lobes in the learning of a visuomotor sequence. Brain Cogn. 34, 218–245.
Doyon, J., Laforce, R. Jr., Bouchard, G., Gaudreau, D., Roy, J., Poirier, M., Bedard, P. J., Bedard, F., and Bouchard, J. P. (1998). Role of the striatum, cerebellum and frontal lobes in the automatization of a repeated visuomotor sequence of movements. Neuropsychologia 36, 625–641.
Eichenbaum, H., and Cohen, N. J. (2001). From Conditioning to Conscious Recollection: Memory Systems of the Brain. Oxford: Oxford University Press.
Embick, D., Marantz, A., Miyashita, Y., O’Neil, W., and Sakai, K. L. (2000). A syntactic specialization for Broca’s area. Proc. Natl. Acad. Sci. U.S.A. 97, 6150–6154.
Ettlinger, M., Bradlow, A. R., and Wong, P. C. M. (in press). “The persistence and obliteration of opaque interactions,” in Proceedings of the 45th Annual Meeting of the Chicago Linguistics Society, ed. R. Bochnak Chicago, IL: Chicago Linguistics Society.
Evans, J. L., Saffran, J. R., and Robe-Torres, K. (2009). Statistical learning in children with specific language impairment. J. Speech Lang. Hear. Res. 52, 321–335.
Exner, C., Koschack, J., and Irle, E. (2002). The differential role of premotor frontal cortex and basal ganglia in motor sequence learning: evidence from focal basal ganglia lesions. Learn. Mem. 9, 376–386.
Fiser, J., and Aslin, R. N. (2001). Unsupervised statistical learning of higher-order spatial structures from visual scenes. Psychol. Sci. 12, 499–504.
Fodor, J. A. (1983). The Modularity of Mind: An Essay on Faculty Psychology. Cambridge, MA: MIT Press.
Forkstam, C., Hagoort, P., Fernandez, G., Ingvar, M., and Petersson, K. M. (2006). Neural correlates of artificial syntactic structure classification. Neuroimage 32, 956–967.
Friederici, A. D., Steinhauer, K., and Pfeifer, E. (2002). Brain signatures of artificial language processing: evidence challenging the critical period hypothesis. Proc. Natl. Acad. Sci. U.S.A. 99, 529–534.
Gabrieli, J. D., Stebbins, G. T., Singh, J., Willingham, D. B., and Goetz, C. G. (1997). Intact mirror-tracing and impaired rotary-pursuit skill learning in patients with Huntington’s disease: evidence for dissociable memory systems in skill learning. Neuropsychology 11, 272–281.
Graybiel, A. M. (2005). The basal ganglia: learning new tricks and loving it. Curr. Opin. Neurobiol. 15, 638–644.
Grill-Spector, K., Henson, R., and Martin, A. (2006). Repetition and the brain: neural models of stimulus-specific effects. Trends Cogn. Sci. (Regul. Ed.) 10, 14–23.
Grodzinsky, Y. (2000). The neurology of syntax: language use without Broca’s area. Behav. Brain Sci. 23, 1–21; discussion 21–71.
Grodzinsky, Y., and Santi, A. (2008). The battle for Broca’s region. Trends Cogn. Sci. (Regul. Ed.) 12, 474–480.
Hagoort, P. (2005). On Broca, brain, and binding: a new framework. Trends Cogn. Sci. (Regul. Ed.) 9, 416–423.
Halpern, A. R., and Mullensiefen, D. (2008). Effects of timbre and tempo change on memory for music. Q. J. Exp. Psychol. (Colchester) 61, 1371–1384.
Halpern, A. R., and O’Connor, M. G. (2000). Implicit memory for music in Alzheimer’s disease. Neuropsychology 14, 391–397.
Harris, K. C., Dubno, J. R., Keren N. I. (2009). Speech recognition in younger and older adults: a dependency on low-level auditory cortex. J. Neurosci. 29, 6078–6087.
Huron, D. (2006). Sweet Anticipation: Music and The Psychology of Expectation. Cambridge, MA: MIT Press.
Huron, D., and Margulis, E. H. (2010). “Musical expectancy and thrills,” in Handbook of Music and Emotion, eds. P. N. Juslin, and J. Sloboda (Oxford: Oxford University Press), 575–604.
Hutchins, S., and Palmer, C. (2008). Repetition priming in music. J. Exp. Psychol. Hum. Percept. Perform. 34, 693–707.
Jackson, G. M., Jackson, S. R., Harrison, J., Henderson, L., and Kennard, C. (1995). Serial reaction time learning and Parkinson’s disease: evidence for a procedural learning deficit. Neuropsychologia 33, 577–593.
James, C. E., Britz, J., Vuilleumier, P., Hauert, C. A., and Michel, C. M. (2008). Early neuronal responses in right limbic structures mediate harmony incongruity processing in musical experts. Neuroimage 42, 1597–1608.
Kamide, Y. (2008). Anticipatory processes in sentence processing. Lang. Linguist. Compass 2, 647–670.
Kamide, Y., Scheepers, C., and Altmann, G. T. (2003). Integration of syntactic and semantic information in predictive processing: cross-linguistic evidence from German and English. J. Psycholinguist. Res. 32, 37–55.
Kaufman, S. B., Deyoung, C. G., Gray, J. R., Jimenez, L., Brown, J., and Mackintosh, N. (2010). Implicit learning as an ability. Cognition 116, 321–340.
Kiehl, K. A., Laurens, K. R., and Liddle, P. F. (2002). Reading anomalous sentences: an event-related fMRI study of semantic processing. Neuroimage 17, 842–850.
Klein, T. A., Neuman, J., Reuter, M., Hennig, J., Cramon, D., and Ullsperger, M. (2007a). Genetically determined differences in learning from errors. Science 318, 1642–1645.
Klein, T. A., Neumann, J., Reuter, M., Hennig, J., Von Cramon, D. Y., and Ullsperger, M. (2007b). Genetically determined differences in learning from errors. Science 318, 1642–1645.
Knowlton, B. J., Mangels, J. A., and Squire, L. R. (1996). A neostriatal habit learning system in humans. Science 273, 1399–1402.
Knowlton, B. J., and Squire, L. R. (1996). Artificial grammar learning depends on implicit acquisition of both abstract and exemplar-specific information. J. Exp. Psychol. Learn. Mem. Cogn. 22, 169–181.
Koechlin, E., Danek, A., Burnod, Y., and Grafman, J. (2002). Medial prefrontal and subcortical mechanisms underlying the acquisition of motor and cognitive action sequences in humans. Neuron 35, 371–381.
Koelsch, S. (2006). Significance of Broca’s area and ventral premotor cortex for music-syntactic processing. Cortex 42, 518–520.
Koelsch, S. (2010). “Unconscious memory representations underlying music-syntactic processing and processing of auditory oddballs,” in Unconscious Memory Representations in Perception: Processes and Mechanisms in The Brain, eds. I. Cziglar, and I. Winkler (Herndon, VA: John Benjamins Publishing Co.), 209–244.
Koelsch, S., Gunter, T., Friederici, A. D., and Schroger, E. (2000). Brain indices of music processing: “nonmusicians” are musical. J. Cogn. Neurosci. 12, 520–541.
Koelsch, S., and Jentschke, S. (2008). Short-term effects of processing musical syntax: an ERP study. Brain Res. 1212, 55–62.
Kraus, N., and Chandrasekaran, B. (2010). Music training for the development of auditory skills. Nat. Rev. Neurosci. 11, 599–605.
Levitin, D. J. (1994). Absolute memory for musical pitch: evidence from the production of learned melodies. Percept. Psychophys. 56, 414–423.
Lieberman, M. D., Chang, G. Y., Chiao, J., Bookheiner, S. Y., and Knowlton, B. J. (2004). An event-related fMRI study of artificial grammar learning in a balanced chunk strength design. J. Cogn. Neurosci. 16, 427–438.
Maess, B., Koelsch, S., Gunter, T. C., and Friederici, A. D. (2001). Musical syntax is processed in Broca’s area: an MEG study. Nat. Neurosci. 4, 540–545.
Margulis, E. H., and Levine, W. (2006). Timbre priming effects and expectation in melody. J. New Music Res. 35, 175–182.
McNab, F., Varrone, A., Farde, L., Jucaite, A., Bystritsky, P., Forssberg, H., and Klingberg, T. (2009). Changes in cortical dopamine D1 receptor binding associated with cognitive training. Science 323, 800–802.
McNealy, K., Mazziotta, J. C., and Dapretto, M. (2006). Cracking the language code: neural mechanisms underlying speech parsing. J. Neurosci. 26, 7629–7639.
Mestres-Misse, A., Camara, E., Rodriguez-Fornells, A., Rotte, M., and Munte, T. F. (2008). Functional neuroanatomy of meaning acquisition from context. J. Cogn. Neurosci. 20, 2153–2166.
Misyak, J. B., Christiansen, M. H., and Tomblin, J. B. (2010). Sequential expectations: the role of prediction-based learning in language. Top. Cogn. Sci. 2, 138–153.
Moro, A., Tettamanti, M., Perani, D., Donati, C., Cappa, S. F., and Fazio, F. (2001). Syntax and the brain: disentangling grammar by selective anomalies. Neuroimage 13, 110–118.
Musso, M., Moro, A., Glauche, V., Rijntjes, M., Reichenbach, J., Buchel, C., and Weiller, C. (2003). Broca’s area and the language instinct. Nat. Neurosci. 6, 774–781.
Nissen, M. J., and Bullemer, P. (1987). Attentional requirements of learning: evidence from performance measures. Cogn. Psychol. 19, 1–32.
Onishi, K. H., Chambers, K. E., and Fisher, C. (2002). Learning phonotactic constraints from brief auditory experience. Cognition 83, B13–B23.
Packard, M. G., and Knowlton, B. J. (2002). Learning and memory functions of the basal ganglia. Annu. Rev. Neurosci. 25, 563–593.
Paradis, M. (1994). “Neurolinguistic aspects of implicit and explicit memory: implications for bilingualism,” in Implicit and Explicit Learning of Second Languages, ed. N. Ellis (London: Academic Press), 393–419.
Patel, A. D., Gibson, E., Ratner, J., Besson, M., and Holcomb, P. J. (1998a). Processing syntactic relations in language and music: an event-related potential study. J. Cogn. Neurosci. 10, 717–733.
Patel, A. D., Peretz, I., Tramo, M., and Labreque, R. (1998b). Processing prosodic and musical patterns: a neuropsychological investigation. Brain Lang. 61, 123–144.
Peach, R. K., and Wong, P. C. M. (2004). Integrating the message level into treatment for agrammatism using story retelling. Aphasiology 18, 429–441.
Peigneux, P., Maquet, P., Van Der Linden, M., Meulemans, T., Degueldre, C., Delfiore, G., Luxen, A., Cleeremans, A., and Franck, G. (1999a). Left inferior frontal cortex is involved in probabilistic serial reaction time learning. Brain Cogn. 40, 215–219.
Peigneux, P., Meulemans, T., Van Der Linden, M., Salmon, E., and Petit, H. (1999b). Exploration of implicit artificial grammar learning in Parkinson’s disease. Acta Neurol. Belg. 99, 107–117.
Peretz, I., Gaudreau, D., and Bonnel, A. M. (1998). Exposure effects on music preference and recognition. Mem. Cognit. 26, 884–902.
Petersson, K. M., Folia, V., and Hagoort, P. (in press). What artificial grammar learning reveals about the neurobiology of syntax. Brain Lang. 10.1016/j.bandl.2010.08.003. [Epub ahead of print].
Petersson, K. M., Forkstam, C., and Ingvar, M. (2004). Artificial syntactic violations activate Broca’s region. Cogn. Sci. 28, 383–407.
Phillips, L. H., Wynn, V., Gilhooly, K. J., Della Sala, S., and Logie, R. H. (1999). The role of memory in the Tower of London task. Memory 7, 209–231.
Pinker, S., and Ullman, M. T. (2002). The past and future of the past tense. Trends Cogn. Sci. (Regul. Ed.) 6, 456–463.
Ranganath, C., and Rainer, G. (2003). Neural mechanisms for detecting and remembering novel events. Nat. Rev. Neurosci. 4, 193–202.
Rauch, S. L., Whalen, P. J., Savage, C. R., Curran, T., Kendrick, A., Brown, H. D., Bush, G., Breiter, H. C., and Rosen, B. R. (1997). Striatal recruitment during an implicit sequence learning task as measured by functional magnetic resonance imaging. Hum. Brain Mapp. 5, 124–132.
Reber, P. J., Martinez, L. A., and Weintraub, S. (2003). Artificial grammar learning in Alzheimer’s disease. Cogn. Affect. Behav. Neurosci. 3, 145–153.
Reber, P. J., and Squire, L. R. (1994). Parallel brain systems for learning with and without awareness. Learn. Mem. 1, 217–229.
Redington, M., and Chater, N. (1997). Probabilistic and distributional approaches to language acquisition. Trends Cogn. Sci. (Regul. Ed.) 1, 273–281.
Rodriguez-Fornells, A., Cunillera, T., Mestres-Misse, A., and De Diego-Balaguer, R. (2009). Neurophysiological mechanisms involved in language learning in adults. Philos. Trans. R. Soc. Lond. B Biol. Sci. 364, 3711–3735.
Saffran, J. R., Aslin, R. N., and Newport, E. L. (1996a). Statistical learning by 8-month-old infants. Science 274, 1926–1928.
Saffran, J. R., Newport, E. L., and Aslin, R. N. (1996b). Word segmentation: the role of distributional cues. J. Mem. Lang. 35, 606–621.
Saffran, J. R., Johnson, E. K., Aslin, R. N., and Newport, E. L. (1999). Statistical learning of tone sequences by human infants and adults. Cognition 70, 27–52.
Sahin, N. T., Pinker, S., Cash, S. S., Schomer, D., and Halgren, E. (2009). Sequential processing of lexical, grammatical, and phonological information within Broca’s area. Science 326, 445–449.
Sammler, D., Koelsch, S., and Friederici, A. D. (2011). Are left fronto-temporal brain areas a prerequisite for normal music-syntactic processing? Cortex 47, 659–673.
Samson, S., and Peretz, I. (2005). Effects of prior exposure on music liking and recognition in patients with temporal lobe lesions. Ann. N. Y. Acad. Sci. 1060, 419–428.
Schacter, D. L. (1987). Implicit memory: history and current status. J. Exp. Psychol. Learn. Mem. Cogn. 13, 501–518.
Schacter, D. L., and Graf, P. (1986). Preserved learning in amnesic patients: perspectives from research on direct priming. J. Clin. Exp. Neuropsychol. 8, 727–743.
Schellenberg, E. G., Bigand, E., Poulin-Charronnat, B., Garnier, C., and Stevens, C. (2005). Children’s implicit knowledge of harmony in Western music. Dev. Sci. 8, 551–566.
Schön, D., Boyer, M., Moreno, S., Besson, M., Peretz, I., and Kolinsky, R. (2008). Songs as an aid for language acquisition. Cognition 106, 975–983.
Seamans, J., and Yang, C. R. (2004). The principal features and mechanisms of dopamine modulations in the prefrontal cortex. Prog. Neurobiol. 74, 1–57.
Seitz, A. R., and Watanabe, T. (2003). Psychophysics: is subliminal learning really passive? Nature 422, 36.
Shallice, T. (1982). Specific impairments of planning. Philos. Trans. R. Soc. Lond. B Biol. Sci. 298, 199–209.
Shohamy, D., and Adcock, R. A. (2010). Dopamine and adaptive memory. Trends Cogn. Sci. (Regul. Ed.) 14.
Shohamy, D., Myers, C. E., Hopkins, R. O., Sage, J., and Gluck, M. A. (2009). Distinct hippocampal and basal ganglia contributions to probabilistic learning and reversal. J. Cogn. Neurosci. 21, 1821–1833.
Slevc, L. R., and Miyake, A. (2006). Individual differences in second-language proficiency: does musical ability matter? Psychol. Sci. 17, 675–681.
Slevc, L. R., Rosenberg, J. C., and Patel, A. D. (2009). Making psycholinguistics musical: self-paced reading time evidence for shared processing of linguistic and musical syntax. Psychon. Bull. Rev. 16, 374–381.
Squire, L. R., and Knowlton, B. J. (2000). “The medial temporal lobe, the hippocampus, and the memory systems of the brain,” in The New Cognitive Neurosciences, ed. M. S. Gazzaniga (Cambridge, MA: MIT Press), 765–780.
Szpunar, K. K., Schellenberg, E. G., and Pliner, P. (2004). Liking and memory for musical stimuli as a function of exposure. J. Exp. Psychol. Learn. Mem. Cogn. 30, 370–381.
Tillmann, B., Bharucha, J. J., and Bigand, E. (2000). Implicit learning of tonality: a self-organizing approach. Psychol. Rev. 107, 885–913.
Tillmann, B., Janata, P., and Bharucha, J. J. (2003). Activation of the inferior frontal cortex in musical priming. Brain Res. Cogn. Brain Res. 16, 145–161.
Tillmann, B., and Poulin-Charronnat, B. (2010). Auditory expectations for newly acquired structures. Q. J. Exp. Psychol. (Colchester) 63, 1646–1664.
Tulving, E., Schacter, D. L., and Stark, H. A. (1982). Priming effects in word-fragment completion are independent of recognition memory. J. Exp. Psychol. Learn. Mem. Cogn. 8, 336–342.
Ullman, M. T. (2006). Is Broca’s area part of a basal ganglia thalamocortical circuit? Cortex 42, 480–485.
Vakil, E., Kahan, S., Huberman, M., and Osimani, A. (2000). Motor and non-motor sequence learning in patients with basal ganglia lesions: the case of serial reaction time (SRT). Neuropsychologia 38, 1–10.
Van Berkum, J. (2008). Understanding sentences in context: what brain waves can tell us. Curr. Dir. Psychol. Sci. 17, 376–380.
Wade, T., and Holt, L. L. (2005). Incidental categorization of spectrally complex non-invariant auditory stimuli in a computer game task. J. Acoust. Soc. Am. 118, 2618–2633.
Witt, K., Nuhsman, A., and Deuschl, G. (2002). Intact artificial grammar learning in patients with cerebellar degeneration and advanced Parkinson’s disease. Neuropsychologia 40, 1534–1540.
Wong, P. C. M., Chan, A. H. D., Roy, A., and Margulis, E. H. (in press). The bimusical brain is not two monomusical brains in one: evidence from musical affective processing. J. Cogn. Neurosci. 10.1162/jocn_a_00105. [Epub ahead of print].
Wong, P. C. M., Ettlinger, M., Sheppard, J., Gunasekaran, G., and Dhar, S. (2010). Neuroanatomical characteristics and speech perception in noise in older adults. Ear Hear. 31, 471–479.
Wong, P. C. M., Jin, J. X., Gunasekera, G. M., Abel, R., Lee, E. R., Dhar. S. (2009a). Aging and cortical mechanisms of speech perception in noise. Neuropsychologia 47, 693–703.
Wong, P. C. M., Roy, A. K., and Margulis, E. H. (2009b). Bimusicalism: the implicit dual enculturation of cognitive and affective systems. Music Percept. 27, 81–88.
Wong, P. C. M., Skoe, E., Russo, N. M., Dees, T., and Kraus, N. (2007). Musical experience shapes human brainstem encoding of linguistic pitch patterns. Nat. Neurosci. 10, 420–422.
Wong, P. C. M., Uppunda, A. K., Parrish, T. B., and Dhar, S. (2008). Cortical mechanisms of speech perception in noise. J. Speech Lang. Hear. Res. 51, 1026–1041.
Keywords: language, music, implicit memory, artificial grammar learning
Citation: Ettlinger M, Margulis EH and Wong PC (2011) Implicit memory in music and language. Front. Psychology 2:211. doi: 10.3389/fpsyg.2011.00211
Received: 16 February 2011; Accepted: 15 August 2011;
Published online: 09 September 2011.
Edited by:
Lutz Jäncke, University of Zurich, SwitzerlandReviewed by:
Mathias S. Oechslin, University of Geneva, SwitzerlandStefan Elmer, University of Zurich, Switzerland
Copyright: © 2011 Ettlinger, Margulis and Wong. This is an open-access article subject to a non-exclusive license between the authors and Frontiers Media SA, which permits use, distribution and reproduction in other forums, provided the original authors and source are credited and other Frontiers conditions are complied with.
*Correspondence: Patrick C. M. Wong, The Roxelyn and Richard Pepper Department of Communication Sciences and Disorders, Communication Neural Systems Research Group, Northwestern University, 2240 Campus Drive, Evanston, IL 60208, USA. e-mail: pwong@northwestern.edu