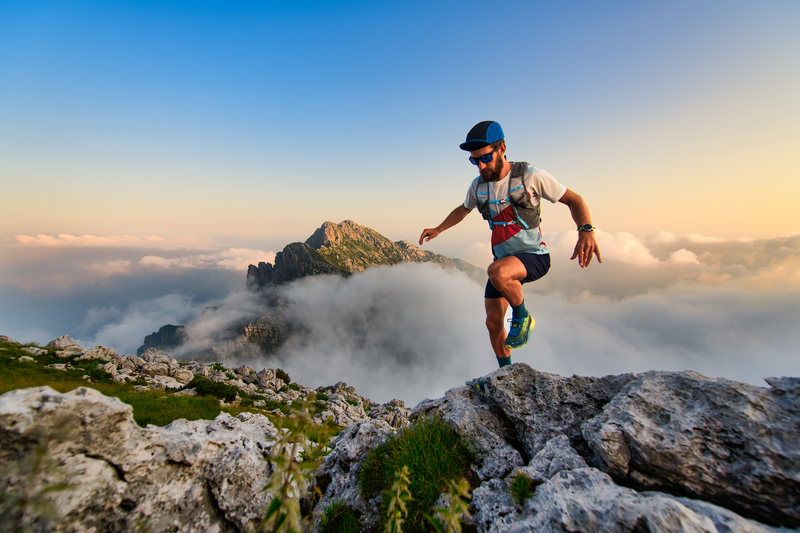
94% of researchers rate our articles as excellent or good
Learn more about the work of our research integrity team to safeguard the quality of each article we publish.
Find out more
REVIEW article
Front. Psychol. , 18 July 2011
Sec. Auditory Cognitive Neuroscience
volume 2 - 2011 | https://doi.org/10.3389/fpsyg.2011.00167
This article is part of the Research Topic The relationship between music and language View all 23 articles
Adults and infants can use the statistical properties of syllable sequences to extract words from continuous speech. Here we present a review of a series of electrophysiological studies investigating (1) Speech segmentation resulting from exposure to spoken and sung sequences (2) The extraction of linguistic versus musical information from a sung sequence (3) Differences between musicians and non-musicians in both linguistic and musical dimensions. The results show that segmentation is better after exposure to sung compared to spoken material and moreover, that linguistic structure is better learned than the musical structure when using sung material. In addition, musical expertise facilitates the learning of both linguistic and musical structures. Finally, an electrophysiological approach, which directly measures brain activity, appears to be more sensitive than a behavioral one.
Language and music are both highly complex and articulated systems; both involve the combination of a small number of elements according to rules that allow the generation of unlimited numbers of utterances or musical phrases. Despite the complexity in language input, most infants acquire their mother tongue with astonishing ease and without formal instruction. This ability to learn new languages continues, though to a lesser extent, during adulthood. Musical skills, such as tapping along to a musical beat and singing, are also acquired without effort in early life. This similarity between speech and music acquisition, along with the extensive periods of musical training undergone by musicians allow us make several predictions. Firstly, that the learning of linguistic and musical structures may be similar. Secondly, that the learning of linguistic structures may be influenced by musical structures, and vice versa. And thirdly, that musical expertise may transfer to language learning.
In this paper we will focus on one of the first major difficulties in speech perception encountered by infants and second language learners: the ability to segment speech into separate units (words). Since the speech stream does not provide consistent acoustic cues to mark word boundaries, such as pauses or stresses, speech is more likely to be perceived as a continuous stream of sounds. The challenge for the learner, therefore, is to be able to segment this continuous speech stream into separate units, words, that will then be mapped onto conceptual representations.
In addition to prosodic cues the statistical structure of language is also an important cue for implicit speech segmentation (Saffran et al., 1996b; Kuhl, 2004; Rodríguez-Fornells et al., 2009). In general, “syllables that are part of the same word tend to follow one another predictably, whereas syllables that span word boundaries do not” (Saffran et al., 2001). The design typically used in this kind of investigation begins with a passive listening phase lasting few minutes. During this phase, participants listen to a synthetic and artificial language built in such a way that the only cues to segment words are conditional probabilities between syllable pairs (high within words and lower across word boundaries). A two-alternative forced choice test follows in which participants must choose between two items based on which sounds the most familiar: one item is a word from the language while the other is not.
Eight-month-old infants can learn to segment words in a simple artificial language in 2 min (Saffran et al., 1996a). Similar results have also been reported for newborns and adults (Aslin et al., 1998; Gervain et al., 2008; Teinonen et al., 2009), indicating a certain degree of independence from the developmental stage. Statistical learning has also been described in non-human primates and rats (Toro and Trobalón, 2005; Saffran et al., 2008), suggesting that it is not unique to humans. Statistical learning also seems to be « modality independent » insofar as several results have shown efficient learning using visual stimuli (Fiser and Aslin, 2005), but also visual movement or shape sequences (Fiser and Aslin, 2002) as well as sequences of tactile stimulations (Conway and Christiansen, 2005). Finally, learning takes also place when syllables are replaced with tones (Saffran et al., 1999). On a similar vein, Tillmann and McAdams (2004) replicated previous findings using complex non-verbal auditory material (sounds of different musical instruments). They showed that listeners are sensitive to statistical regularities despite acoustical characteristics (timber) in the material that are supposed to affect grouping. Thus, although certain processing steps such as harmonic processing might be domain dependent (Peretz and Coltheart, 2003), these results reveal that this type of learning (i.e., statistical learning) is not specific to speech (and thus is not modular), but seems to be a more general-domain process.
When comparing music and language processing, it is difficult not to acknowledge the similarity between the hierarchy of musical structures and the one of prosodic structures in speech (Nespor and Vogel, 1983). Prosody refers to the patterns of rhythm, stress, and intonation in speech. Prosodic variations are crucial cues for communicating emotions, disambiguating syntax, and also at a segmental level. Metrical cues play an important role in word segmentation: for instance, lexical stress typically implies increased duration, pitch, and loudness of specific syllables in a word, thus creating different perceptual rhythmic patterns (Abercrombie, 1967) which affect segmentation. These metrical cues constrain the ordering of stressed and unstressed syllables and are at the origin of the perception of strong and weak points in speech. A similar perception of strong and weak beats occurs in music. When prosodic cues are inserted, even subliminally, within the speech stream, performance on a speech segmentation task improves (Peña et al., 2002; Cunillera et al., 2006, 2008; De Diego Balaguer et al., 2007). An interesting example of how prosody can ease speech segmentation is the specific and exaggerated intonation that is used when talking to infants (infant-directed speech, ID speech). Compared to adult directed (AD) speech, ID speech is characterized by a slower rate, larger pitch and contour variations, and longer pauses (Fernald, 1992) and is easier to segment (Thiessen et al., 2005). Thus, if ID speech can be said to possess more exaggerated and more melodic prosodic features, a further step might be to consider how similar exaggerated and melodic features of singing might also ease speech segmentation.
Most of the studies investigating the relationship between music and language have focused on one domain or the other separately and this is also the case for statistical learning investigations. This is somewhat problematic insofar as a comparison of results issued from different tasks, subjects, and types of analyses is not straightforward. Therefore, singing is particularly well-suited to the study of the relation between language and music, the advantage being that both linguistic and musical information are merged into one acoustic signal with two salient dimensions, allowing for a direct comparison within the same experimental material. Moreover, this allows asking subjects to perform a task while manipulating the relation between linguistic and musical dimensions, thus studying the potential interferences from one dimension to the other. Of course, care must be taken because, in song, the phonological and metrical structures of language are strongly influenced by the type of melody that is sung. For instance, vowels sung in a very high register have the tendency to be less recognizable (Scotto di Carlo, 1994). We will review here three studies using a sung language. The design we used was very similar to that used by Saffran et al. (1999) Participants listened to a continuous stream of sung syllables lasting a few minutes. The stream was built by a pseudo-random concatenation of 6 (Experiments 1 and 2) or 5 (Experiment 3) trisyllabic words. Each syllable was sung at a constant pitch. This learning phase was followed by a two-alternative forced choice test wherein one item was a word from the language while the other was not (i.e., a part-word built by merging the end of a word with the beginning of another). Participants had to choose the most familiar of the two presented items.
In Experiment 1 (Schön et al., 2008), we compared segmentation of a spoken language versus segmentation of a sung language (see Figure 1). Two groups of participants listened to either 7 min of a flat contour artificial language or to 7 min of an artificial language with the same statistical structure wherein each syllable was sung on a specific pitch. The test, a two-alternative forced choice test, was identical for both groups and used spoken words only (flat contour).
Figure 1. Illustration of the experimental design used in our three experiments: a learning phase is administered to participants and is followed by one (Schön et al., 2008) or two tests (Francois and Schön, 2010, 2011) depending on the experiment. The linguistic test uses spoken items while the musical test uses piano tones.
While 7 min of listening were not enough to segment a language when it was spoken, they were sufficient to learn the same language when it was sung. These results suggest that redundancy in statistical musical and linguistic structures benefits the learning process, even if more general factors linked to motivation and arousal can not be excluded. Indeed, the sung version might well have been more « arousing » than the spoken version. To gain a finer understanding of the mechanisms responsible for these differences between spoken and sung languages we ran a further experiment using a sung language, wherein linguistic and musical statistical structures mismatched. More precisely, musical boundaries were shifted of one step to the right (i.e., one syllable later). Thus, while the statistical of linguistic and musical structures were preserved, word and pitch boundaries did not occur at the same time anymore. Performances laid in between the spoken version and the original sung version obtained in the two first experiments. These results point to a beneficial effect of both structural (“statistical”) and motivational properties of music in the very first steps of language acquisition (Thiessen et al., 2005; Schön et al., 2008).
The test used in Experiment 1 only contained spoken items. Thus, we could not know whether learning of the musical structure took place. At this aim and in order to better understand how musical information facilitated speech segmentation, we designed a similar experiment that tested both learning of musical and linguistic structures contained in the sung language, and also recorded event-related potentials during the tests (ERPs). Thus, two tests followed the learning phase, one using spoken items at a fixed pitch, and another using piano tone sequences. First, we ran a study with a random sample (Francois and Schön, 2010, henceforth Experiment 2), then we compared a group of musicians and non-musicians (Francois and Schön, 2011, henceforth Experiment 3). At the behavioral level, results showed that linguistic structures were better learned than musical ones (though significance was reached only when pooling the data across the two studies). Comparison of performance with chance level (0.5) showed that while the participants’ level of performance was above chance in the test using spoken items, it was not in the test using tones. Indeed, as can be seen on Figure 2, the distribution of the performances in the musical test were highly heterogeneous across participants and only half of the subjects exhibited performances higher than chance level. Several non-exclusive explanations can account for this difference.
Figure 2. Percentage of correct responses: box plot of performances in the linguistic (left) and musical tests (right). Data from Experiments 2 and 3 (Francois and Schön, 2010, 2011), are pooled together leading to N = 50 subjects (dashed line = 50%, chance level).
First, linguistic storage and/or retrieval of “lexical” items might have been more efficient or less sensitive to interferences caused by part-words/tone-part-words. Schendel and Palmer (2007) recently showed that, overall, verbal items were better recalled than musical items. Moreover, they found a larger interference effect on musical recall than on verbal recall. Researches investigating memory representations of song have also shown an advantage for words over melodies (Serafine et al., 1984; Crowder et al., 1990; Morrongiello and Roes, 1990; Hébert and Peretz, 2001; Peretz et al., 2004). Altogether, these results seem to suggest that linguistic information may be more resistant to interferences than musical information.
The second explanation is more stimulus related. Indeed, while the statistical structure of melodic and phonologic information was the same in our material, other important factors may come into play that make melodic segmentation more difficult than word segmentation. For instance, phoneme discrimination is mostly influenced by transient information in the specter (e.g., “py” versus “gy”), while pitch discrimination is influenced by more stationary information (e.g., fundamental frequency). Moreover, phoneme transitions in our language were probably sensitive to the implicit phonotactic knowledge humans have in their mother tongue, dictating which sound sequences are allowed to occur within the words of a language (Friederici and Wessels, 1993). This is hardly transposable to music, wherein the implicit knowledge affecting music perception concerns tonal relationships between notes (Tillmann et al., 2000). Overall, it is very difficult to control and balance the perceptual saliency of the linguistic and musical structures and care must be taken in comparing and interpreting results across dimensions.
Nonetheless, when pooling the data across the two studies (Experiments 2 and 3), we observe a significant positive correlation between performances in music and linguistic tests: the higher the performance in the linguistic test, the higher in the music test (Figure 3). This fits well with results showing a correlation between linguistic and musical abilities. For instance, several studies report a positive correlation between phonological awareness and music perception (Butzlaff, 2000; Anvari et al., 2002; Foxton et al., 2003), suggesting that these competences may share some of the same auditory mechanisms. Interestingly, phonological awareness requires the ability to segment speech into its component sounds and to extract the phonological invariants. Similarly, the perception of music also requires the listener to be able to segment the stream of tones into relevant units and to be able to recognize these units when played with different timbers tempos, keys, and styles.
Figure 3. Correlation between performances in the linguistic and musical tests for 50 participants (Francois and Schön, 2010, 2011). The correlation is significant (p = 0.05), that is the better participants performance in the musical test, the better are performances in the linguistic test.
Overall, behavioral data alone could not reveal whether participants could or could not segment the musical structure (Experiments 2 and 3). Indeed, the lack of significance might simply be related to a lack in sensitivity of the testing procedure and of the dependent variable. ERPs seem to be more sensitive to the subtle mechanisms underlying implicit learning. Indeed, McLaughlin et al. (2004), in a study focusing on second language learning, showed that ERPs can be more sensitive in reflecting implicit learning than do explicit categorical judgments and that behavioral assessment might underestimate the extent to which learning has taken place. While behavior reflects the combined effects of several processing stages, ERPs, thanks to their continuous time resolution, can (sometimes) show differences in brain activity even when this is not backed by an overt behavioral response. Several examples of ERP-behavior dissociations have been reported pointing the greater ERP sensitivity, for instance in the field of subliminal perception (Sergent et al., 2005) and learning (Tremblay et al., 1998).
In our studies (Experiments 2 and 3), ERPs were also more sensitive than behavior in that they revealed a significant difference between familiar and unfamiliar items in both linguistic and musical test. Indeed, even subjects with a performance that could be hardly interpreted as higher than chance (e.g., subjects with 55% of correct responses), did show different ERPs to words and part-words. These results seem to point to a greater sensitivity of the ERP data compared to the behavioral data in implicit learning designs.
Electrophysiological data of the linguistic test showed a negative component at fronto-central sites in the 450- to 800-ms latency band which was larger for part-words than for words (see Figure 4). In order to interpret this ERP difference it is important to clarify the respective “linguistic” status of the two conditions. What researchers in field of statistical learning typically call “words” are in fact nonsense words that are repeated in a random order for N minutes during the learning phase. Therefore, “words” do not have an entry in the lexicon. However, because they are repeated so many times (typically >100 each), they “pop out” of the continuous stream of syllables, due to statistical learning or associative mechanisms. Thus, to a certain extent, “words” become familiar, and as such they can be distinguished from “part-words” acquiring a kind of proto-lexical status (Fernandes et al., 2009; Rodríguez-Fornells et al., 2009). Indeed, in an experiment using X words, if, during the learning phase, “words” are heard N times each, “part-words” are heard N/(X − 1) times (e.g., 216 and 44 times, respectively). This is because “part-words” are built by merging the end of a word with the beginning of another (and each word can be followed by X − 1 possible words). Therefore, the linguistic status of the items used in the test is that of pseudo-words which are more or less familiar (words and part-words, respectively).
Figure 4. Grand average across two experiments (Francois and Schön, 2010, 2011; N = 50), for the linguistic (left), and musical test (right) at Cz electrode. Solid lines illustrate ERPs to familiar words/tone-words; dashed lines illustrate ERPs to unfamiliar part-words/tone-part-words. Current Source Density Maps illustrate the topographic distribution of the familiarity effect in the significant time windows.
The most common use of pseudo-words in the ERP literature is found in studies concerned with lexicality. These studies most often compare a pseudo-word to a word condition. For instance, O’Rourke and Holcomb (2002) used a lexical decision task and found a larger negativity for pseudo-words than for words, most prominent at anterior sites. Moreover, the negative component to pseudo-words peaked around 600 ms, 200 ms later than the classical N400 effect described for words. The authors claimed that pseudo-word processing may undergo an additional time consuming step, consisting of a top-down checking process. Interestingly, in our studies, the negative component to both familiar and unfamiliar items was also peaking around 600 ms.
Turning to familiarity effects, previous ERP studies have shown that the amplitude of the N400 is sensitive to familiarity. Indeed, low frequency words elicited a larger negativity peaking around 400 ms compared to high frequency words (Van Petten and Kutas, 1990; Young and Rugg, 1992). Thus, the N400 amplitude seems to be sensitive to the ease of accessing the information from long-term memory (Federmeier and Kutas, 2000). In statistical learning experiments, during the passive listening phase, only sufficiently reinforced items may “survive” concurrent interference and become stored in a long lasting manner (Perruchet and Vinter, 2002). Therefore, the observed differences between words (familiar) and pseudo-words (unfamiliar) in the negative component, might reflect the difficulty of accessing and/or retrieving item representations.
Electrophysiological data of the musical test revealed a similar ERP component whose amplitude was larger for unfamiliar than for familiar items: a fronto-central negative component peaking around 900 ms after stimulus onset (Experiments 2 and 3).
In the studies described, insofar as the sung language was built by concatenating trisyllabic words, participants were (implicitly) familiarized with the three-pitch melodies corresponding to the melodic contour of the trisyllabic words. Therefore the same issues on lexical representation and familiarity described for linguistic items also apply when testing with musical sequences. Interestingly, in both Experiments 2 and 3, we found a fronto-central negative component larger for unfamiliar than familiar musical items, with a topographic distribution quite similar to the one observed in the linguistic test for the same contrast (unfamiliar – familiar, see Figure 4). However, although sensitive to the same factor (familiarity) and with a similar topography, this negative component peaked at around 600 ms to linguistic items and at 900 ms to musical items, possibly due to the fact that participants found the musical test harder than the linguistic test. One may interpret these differences in latencies in terms of functional differences, that is, different underlying processes. We favor the interpretation of these differences in terms of difficulty in item retrieval: participants probably needed more time to “search” whether an item was familiar or not in the musical test than in the linguistic test. Although less commonly described in the literature, late negative components have been observed in response to musical stimuli following for instance, unexpected harmonies (Steinbeis and Koelsch, 2008) but also following musical excerpts that do not match the concept of a previous verbal context (Daltrozzo and Schön, 2009) or at the familiarity emergence point of a melody (Daltrozzo et al., 2010).
Comparing experts to non-experts in a given domain is an elegant way to study the effect of extensive training on brain plasticity. The musicians’ brain has long been considered a model of plasticity (Münte et al., 2002). Thus, comparing musicians to non musicians allows the effects of extensive audio-motor training on the functional and structural organization of the brain to be studied (even if causation is only showed using a longitudinal design). At a perceptual level, it has been shown that musicians outperform non-musicians on a variety of music related task: musicians have a lower frequency threshold (Spiegel and Watson, 1984; Kishon-Rabin et al., 2001; Micheyl et al., 2006) and exhibit finer rhythmic processing than non-musicians (Yee et al., 1994; Jones et al., 1995). Compared to non-musicians, musicians also show an enhanced cortical attentive and pre-attentive processing of linguistic and musical features as reflected by larger amplitude and/or shorter latencies of many ERP components such as the N1, P2, MMN, P300 (Pantev et al., 1998; Koelsch et al., 1999; Shahin et al., 2003; Van Zuijen et al., 2005). In our study on statistical learning (Experiment 3) the N1 component was larger for musicians than non-musicians in both the linguistic and the musical tasks. Interestingly, recent results support the view that increased auditory evoked potentials in musicians (N1–P2) reflect an enlarged neuronal representation for specific sound features of these tones rather than selective attention biases (Baumann et al., 2008). Even at the sub-cortical level, musicians show more robust encoding of linguistic and musical features as reflected by earlier and larger brainstem responses compared to non-musicians (Kraus and Chandrasekaran, 2010). Overall, these differences can be interpreted as reflecting a greater efficiency of musicians’ auditory system in processing sound features and can be accompanied by morphological differences, showing that musicians have a larger gray matter concentration in the auditory cortex (Bermudez and Zatorre, 2005), an increased gray matter density and volume in the left inferior frontal gyrus, Broca’s area (Sluming et al., 2002) and a larger planum temporale (Schlaug et al., 1995; Keenan et al., 2001).
The fact that musicians perceive some sound features more accurately than non-musicians is not so surprising. After all, they spent hours and hours of their life focusing on sounds and the way they are generated, paying close attention to pitch, timber, duration, and timing. It would be rather surprising and even deceiving if this did not affect the way they hear, and as a consequence their brain functioning. What seems to us less evident is that such an intensive musical practice also seems to affect non-musical abilities. For example, both adult and child musicians perform better than matched controls when asked to detect fine contour modifications in the prosody of an utterance (Schön et al., 2004; Magne et al., 2006). Adult musicians also have better performances and larger ERP components to metric incongruities at the end of an utterance (Marie et al., 2011). There is also evidence for a possible correlation between musical and linguistic aptitudes in both children (Anvari et al., 2002; Milovanov et al., 2008, 2009) and adults (Foxton et al., 2003; Slevc and Miyake, 2006) as well as a benefit of music training on linguistic skills (Butzlaff, 2000; Overy, 2003; Gaab et al., 2005; Tallal and Gaab, 2006; Forgeard et al., 2008; Moreno et al., 2009; Parbery-Clark et al., 2009).
When using a sung language in artificial language learning, one needs to choose a series of pitches that are mapped onto syllables. The choice of pitches is an important one as it determines the tonal or atonal character of the stream. Although one may guess that a tonal structure will benefit language learning more than an atonal one, further work is needed to understand how and to what extent the tonality of the musical structure influences learning of the phonological one. In the experiments described here we used a musical structure with a rather strong tonal center (10 notes out of 11 were in C major). Thus, it is possible that participants keep in memory a representation of a tonal center during the post-learning test, which influences the processing of the musical items rather early on. In fact, once a tonal center is established, each individual pitch can be processed and categorized relative to that tonal center, even in the absence of absolute pitch abilities. Indeed, musicians, in the musical test, showed a significant effect of familiarity 200 ms after the first tone onset and before the beginning of the second tone on the P2 component (larger to unfamiliar that familiar items), possibly due to a more difficult categorization of the unfamiliar than familiar items (Liebenthal et al., 2010). Thus, musicians were sensitive to the tonal structure of the language, which in turn influenced their perception of the first tone of the items in the test, despite poor behavioral performance.
This benefit of musical expertise in segmenting the musical structure was also evident in later ERP components. For instance, compared to familiar items, unfamiliar items yielded a larger mismatch negativity (MMN) in response to the second tone of the melody (Experiment 3). Overall, both familiarity effects on the P2 and MMN-like components suggest that musicians did indeed learn the musical structure better than non-musicians and this in turn affected the way they processed the musical items presented during the test. This is in line with previous findings showing that implicit learning of 12-tone music is influenced by expertise. Indeed, only participants with routine exposure to atonal music do (implicitly) perceive the distinction between different types of transforms (Dienes and Longuet-Higgins, 2004).
The most striking finding of the series of experiments reviewed here is that compared to non-musicians, musicians seem to have more “robust” representations of both musical and linguistic structures that have been shaped during the listening phase. Indeed, musicians showed a larger familiarity N400-like effect than non-musicians, in both dimensions (Experiment 3).
We interpret these findings as evidence that musical expertise facilitates regularity extractions and sequence learning in general (Janata and Grafton, 2003). For instance, it is known that musicians can organize a sound sequence according to number regularity, implicitly distinguishing segments containing four tones from the segments containing five tones. By contrast, such a perceptual organization of sound in terms of number is less relevant for non-musicians (Van Zuijen et al., 2005). Moreover, recent findings showed that deaf children with cochlear implants are impaired in visual sequence learning, suggesting that a period of auditory deprivation may have a major impact on cognitive processes that are not specific to the auditory modality (Conway et al., 2010). Therefore, sound seems to provide a cognitive scaffolding for the development of serial-order behavior: whether sound processing is impaired or whether it is extensively practiced have opposite effects. Interestingly, Sluming et al. (2002) reported an increased gray matter density and volume in the left inferior frontal gyrus, Broca’s area. Moreover, neuroplastic development throughout a musicians’ life seems to promote the retention of cortical tissue (Sluming et al., 2002). Broca’s area is known to be involved in on-line speech and tone stream segmentation as well as in music harmonic perception (Tillmann et al., 2003, 2006; McNealy et al., 2006; Abla and Okanoya, 2008; Cunillera et al., 2009). Overall our results support an “auditory scaffolding hypothesis” (Conway et al., 2009) as we presently show that increased exposure to sounds leads to a benefit for implicit learning, putatively via anatomical and/or functional modifications going beyond the auditory regions.
Based on the similarity between music and speech acquisition we made several predictions. Firstly, we predicted that the learning of linguistic and musical structures may be similar. We found that ERP responses to linguistic and musical test-items present a similar (although not identical) morphology and topography (Experiments 2 and 3). While this cannot be interpreted in terms of a similar learning process, it seems that the linguistic and musical representations that are a consequence of the learning process are similar. This further supports previous findings showing that statistical learning can apply to non-linguistic stimuli. Secondly, we predicted that speech segmentation may be influenced by musical structure. Results of Experiment 1 directly address and confirm this hypothesis. By clarifying the structure of the language and by increasing the motivation/arousal of participants, music seems to facilitate speech segmentation. Finally, we predicted that musical expertise may transfer to language learning. Experiment 3 addressed and confirmed this prediction, by showing that musicians have more robust representations of both musical and linguistic structures, possibly via a more efficient brain network involving both auditory and more integrative processing.
Most studies on word segmentation and language learning rely on behavioral measures. These are typically obtained using the head-turning procedure in infants and a familiarity two-alternative forced choice procedure in adults. These procedures are used after the listening phase, in which learning takes place. Thus, these procedures test the result of learning rather than the learning process itself. The data presented here (Experiments 2 and 3) suffer from the same limitation. Researchers in the field of implicit learning have more recently realized the importance of better defining the status of the representations derived from the learning processes and promote the combined use of both subjective behavioral measures (guessing criterion and zero correlation criterion) and EEG or fMRI measures (Seth et al., 2008). In this sense, studies measuring EEG during learning are promising, pointing to several possible electrophysiological indices, ERPs, or oscillations that may reflect how learning takes place over time (Rodríguez-Fornells et al., 2009; De Diego Balaguer et al., 2011). The challenge for the coming years is to access to a temporally detailed learning curve. Ideally, one would want to know how each word presented during the learning has been processed. As some studies seem to point out, learning curves may differ across individuals (Abla et al., 2008). This might be crucial in studying pathology, since similar behavioral results (null effects) may rely upon different learning curves.
The literature comparing music and language processing (and also in studies on language learning) is mostly focused on the perceptual side, with production having received little attention. While the statistical learning framework is a brilliant way of studying language learning in a laboratory, it lacks by construction the rich context and complex behaviors that are typically found in language acquisition. For instance the natural context typically implies a word to world mapping: once a unit/word is identified, it is mapped onto one or several concepts. Moreover, learning acquisition outside the laboratory relies on coupling both listening and imitation. Thus, further efforts needs to be done to elaborate new experimental paradigms comparing language and music using a richer learning context as well as linguistic and musical unit production. Also, while the direct comparison between music and language is certainly a privileged one, it should also be extended to other domains and modalities (e.g., visual sequence learning, number sequences) in order to better understand to what extent underlying mechanisms are domain-general and to provide support to the auditory scaffolding hypothesis (Conway et al., 2009).
The study of the effects of music training on language learning also poses a challenge in terms of brain plasticity. Language learning probably relies on a distributed network involving the superior temporal gyrus (phonological representation), the dorsal stream and premotor cortex for mapping sounds to movement (phonological–articulatory trace) and the medial temporal lobe (lexical trace, Hickok and Poeppel, 2007; Rodríguez-Fornells et al., 2009). Thus, changes due to music training may take place at each of these different processing steps. Moreover, it is highly likely that music training influences the connectivity between these regions (Oechslin et al., 2010). Thus, the future challenge is to track to what extent music training influences the temporal evolution of the brain dynamics of this complicated network as learning takes place.
The authors declare that the research was conducted in the absence of any commercial or financial relationships that could be construed as a potential conflict of interest.
This study has been supported by the French National Research Agency (ANR BLAN: Does Music Boost the Brain) to D. Schön.
Abla, D., Katahira, K., and Abla, O. K. (2008). On-line assessment of statistical learning by event-related potentials. J. Cogn. Neurosci. 20, 952–964.
Abla, D., and Okanoya, K. (2008). Statistical segmentation of tone sequences activates the left inferior frontal cortex: a near-infrared spectroscopy study. Neuropsycholgia 46, 2787–2795.
Anvari, S. H., Trainor, L. J., Woodside, J., and Levy, B. A. (2002). Relations among musical skills, phonological processing, and early reading ability in preschool children. J. Exp. Child. Psychol. 83, 111–130.
Aslin, R. N., Saffran, J. R., and Newport, E. L. (1998). Computation of conditional probability statistics by 8-month-old infants. Psychol. Sci. 9, 321–324.
Baumann, S., Meyer, M., and Jäncke, L. (2008). Enhancement of auditory-evoked potentials in musicians reflects an influence of expertise but not selective attention. J. Cogn. Neurosci. 20, 2238–2249.
Bermudez, P., and Zatorre, R. J. (2005). Differences in gray matter between musicians and nonmusicians. Ann. N. Y. Acad. Sci. 1060, 395–399.
Conway, C. M., and Christiansen, M. H. (2005). Modality-constrained statistical learning of tactile, visual, and auditory sequences. J. Exp. Psychol. Learn. Mem. Cogn. 31, 24–39.
Conway, C. M., Pisoni, D. B., Anaya, E. M., Karpicke, J., and Henning, S. C. (2010). Implicit sequence learning in deaf children with cochlear implants. Dev. Sci. 11, 69–82.
Conway, C. M., Pisoni, D. B., and Kronenberg, W. G. (2009). The importance of sound for cognitive sequencing abilities: the auditory scaffolding hypothesis. Curr. Dir. Psychol. Sci. 18, 275–279.
Crowder, R. G., Serafine, M. L., and Repp, B. (1990). Physical interaction and association by contiguity in memory for the words and melodies of songs. Mem. Cognit. 18, 469–476.
Cunillera, T., Càmara, E., Toro, J. M., Marco-Pallares, J., Sebastián-Galles, N., Ortiz, H., Pujol, J., and Rodríguez-Fornells, A. (2009). Time course and functional neuroanatomy of speech segmentation in adults. Neuroimage 48, 541–553.
Cunillera, T., Gomila, A., and Rodríguez-Fornells, A. (2008). Beneficial effects of word final stress in segmenting a new language: evidence from ERPs. BMC Neurosci. 18, 9.
Cunillera, T., Toro, J. M., Sebastián-Gallés, N., and Rodríguez-Fornells, A. (2006). The effects of stress and statistical cues on continuous speech segmentation: an event-related brain potential study. Brain Res. 1123, 168–178.
Daltrozzo, J., and Schön, D. (2009). Conceptual processing in music as revealed by N400 effects on words and musical targets. J. Cogn. Neurosci. 21, 1882–1892.
Daltrozzo, J., Tillmann, B., Platel, H., and Schön, D. (2010). Temporal aspects of the feeling of familiarity for music and the emergence of conceptual processing. J. Cogn. Neurosci. 22, 1754–1769.
De Diego Balaguer, R., Fuentemilla, L., and Rodriguez-Fornells, A. (2011). Brain dynamics sustaining rapid rule extraction from speech. J. Cogn. Neurosci. [Epub ahead of print].
De Diego Balaguer, R., Toro, J. M., Rodriguez-Fornells, A., and Bachoud-Lévi, A. C. (2007). Different neurophysiological mechanisms underlying word and rule extraction from speech. PLoS ONE 2, e1175.
Dienes, Z., and Longuet-Higgins, C. (2004). Can musical transofrmations be implicitly learned? Cogn. Sci. 28, 531–558.
Fernald, A. (1992). “Human maternal vocalizations to infants as biologically relevant signals,” in The Adapted Mind: Evolutionary Psychology and the Generation of Culture, eds J. Barkow, L. Cosmides and J. Tooby (Oxford: Oxford University Press), 391–428.
Fernandes, T., Kolinsky, R., and Ventura, P. (2009). The metamorphosis of the statistical segmentation output: Lexicalization during artificial language learning. Cognition 112, 349–366.
Fiser, J., and Aslin, R. N. (2002). Statistical learning of higher-order temporal structure from visual shape-sequences. J. Exp. Psychol. Learn. Mem. Cogn. 28, 458–467.
Fiser, J., and Aslin, R. N. (2005). Encoding multielement scenes: statistical learning of visual feature hierarchies. J. Exp. Psychol. Gen. 134, 521–537.
Forgeard, M., Winner, E., Norton, A., and Schlaug, G. (2008). Practicing a musical instrument in childhood is associated with enhanced verbal ability and nonverbal reasoning. PLoS ONE 3, e3566.
Foxton, J. M., Talcott, J. B., Witton, C., Brace, H., McIntyre, F., and Griffiths, T. D. (2003). Reading skills are related to global, but not local, acoustic pattern perception. Nat. Neurosci. 6, 343–344.
Francois, C., and Schön, D. (2010). Learning of musical and linguistic structures: comparing event-related potentials and behavior. Neuroreport 21, 928–932.
Francois, C., and Schön, D. (2011). Musical expertise boosts implicit learning of both musical and linguistic structures. Cereb. Cortex. [Epub ahead of print].
Friederici, A. D., and Wessels, J. M. (1993). Phonotactic knowledge of word boundaries and its use in infant speech perception. Percept. Psychophys. 54, 287–295.
Gaab, N., Tallal, P., and Kim, H. (2005). Neural correlates of rapid spectrotemporal processing in musicians and nonmusicians. Ann. N. Y. Acad. Sci. 1060, 82–88.
Gervain, J., Macagno, F., Cogoi, S., Peña, M., and Mehler, J. (2008). The neonate brain detects speech structure. Proc. Natl. Acad. Sci. U.S.A. 105, 14222–14227.
Hébert, S., and Peretz, I. (2001). Are text and tune of familiar songs separable by brain damage? Brain Cogn. 46, 169–175.
Hickok, G., and Poeppel, D. (2007). The cortical organization of speech processing. Nat. Rev. Neurosci. 8, 393–402.
Janata, P., and Grafton, S. T. (2003). Swinging in the brain: shared neural substrates for behaviors related to sequencing and music. Nat. Neurosci. 6, 682–687.
Jones, M. R., Jagacinski, R. J., Yee, W., Floyd, R. L., and Klapp, S. T. (1995). Tests of attentional flexibility in listening to polyrhythmic patterns. J. Exp. Psychol. Hum. Percept. Perform. 21, 293–307.
Keenan, J. P., Thangaraj, V., Halpern, A. R., and Schlaug, G. (2001). Absolute pitch and planum temporale. Neuroimage 14, 1402–1408.
Kishon-Rabin, L., Amir, O., Vexler, Y., and Zaltz, Y. (2001). Pitch discrimination: are professional musicians better than non-musicians? J. Basic Clin. Physiol. Pharmacol. 12(2 Suppl.), 125–143.
Koelsch, S., Schröger, E., and Tervaniemi, M. (1999). Superior pre-attentive auditory processing in musicians. Neuroreport 10, 1309–1313.
Kraus, N., and Chandrasekaran, B. (2010). Music training for the development of auditory skills. Nat. Rev. Neurosci. 11, 599–605.
Kuhl, P. K. (2004). Early language acquisition: cracking the speech code. Nat. Rev. Neurosci. 5, 831–843.
Liebenthal, E., Desai, R., Ellingson, M. M., Ramachandran, B., Desai, A., and Binder, J. R. (2010). Specialization along the left superior temporal sulcus for auditory categorization. Cereb. Cortex 20, 2958–2970.
Magne, C., Schön, D., and Besson, M. (2006). Musician children detect pitch violations in both music and language better than non musician children. J. Cogn. Neurosci. 18, 199–211.
Marie, C., Magne, C., and Besson, M. (2011). Musicians and the metric structure of words. J. Cogn. Neurosci. 23, 294–305.
McLaughlin, J., Osterhout, L., and Kim, A. (2004). Neural correlates of second – language word learning: minimal instruction produces rapid change. Nat. Neurosci. 7, 703–704.
McNealy, K., Mazziota, J. C., and Dapretto, M. (2006). Cracking the language code: neural mechanisms underlying speech parsing. J. Neurosci. 26, 7629–7639.
Micheyl, C., Delhommeau, K., Perrot, X., and Oxenham, A. J. (2006). Influence of musical and psychoacoustical training on pitch discrimination. Hear. Res. 219, 36–47.
Milovanov, R., Huotilainen, M., Esquef, P. A., Alku, P., Välimäki, V., and Tervaniemi, M. (2009). The role of musical aptitude and language skills in preattentive duration processing in school-aged children. Neurosci. Lett. 460, 161–165.
Milovanov, R., Huotilainen, M., Välimäki, V., Esquef, P. A., and Tervaniemi, M. (2008). Musical aptitude and second language pronunciation skills in school-aged children: neural and behavioral evidence. Brain Res. 1194, 81–89.
Moreno, S., Marques, C., Santos, A., Santos, M., Castro, S. L., and Besson, M. (2009). Musical training influences linguistic abilities in 8-year-old children: more evidence for brain plasticity. Cereb. Cortex 19, 712–723.
Morrongiello, B. A., and Roes, C. L. (1990). Children’s memory for new songs: integration or independent storage of words and tunes? J. Exp. Child Psychol. 50, 25–38.
Münte, T. F., Altenmüller, E., and Jäncke, L. (2002). The musician’s brain as a model of neuroplasticity. Nat. Rev. Neurosci. 3, 473–478.
Nespor, M., and Vogel, I. (1983). “Prosodic structure above the word,” in Prosody: Models and Measurements, eds A. Cutler and D. R. Ladd (Berlin: Springer-Verlag), 123–140.
Oechslin, M. S., Imfeld, A., Loenneker, T., Meyer, M., and Jäncke, L. (2010). The plasticity of the superior longitudinal fasciculus as a function of musical expertise: a diffusion tensor imaging study. Front. Hum. Neurosci. 8, 3–76.
O’Rourke, T. B., and Holcomb, P. J. (2002). Electrophysiological evidence for the efficiency of spoken word processing. Biol. Psychol. 60, 121–150.
Overy, K. (2003). Dyslexia and music: From timing deficits to musical intervention. Ann. N. Y. Acad. Sci. 999, 497–505.
Pantev, C., Oostenveld, R., Engelien, A., Ross, B., Roberts, L. E., and Hoke, M. (1998). Increased auditory cortical representation in musicians. Nature 392, 811–814.
Parbery-Clark, A., Skoe, E., and Kraus, N. (2009). Musical experience limits the degradative effects of background noise on the neural processing of sound. J. Neurosci. 29, 14100–14107.
Peña, M., Bonatti, L., Nespor, M., and Mehler, J. (2002). Signal-driven computations in speech processing. Science 298, 604–607.
Peretz, I., Radeau, M., and Arguin, M. (2004). Two-way interactions between music and language: evidence from priming recognition of tune and lyrics in familiar songs. Mem. Cognit. 32, 142–152.
Perruchet, P., and Vinter, A. (2002). The self-organizing consciousness. Behav. Brain Sci. 25, 297–300.
Rodríguez-Fornells, A., Cunillera, T., Mestres-Missé, A., and De Diego-Balaguer, R. (2009). Neurophysiological mechanisms involved in language learning in adults. Philos. Trans. R. Soc. B. Biol. Sci. 364, 3711–3735.
Saffran, J., Johnson, E., Aslin, R., and Newport, E. (1999). Statistical learning of tone sequences by human infants and adults. Cognition 70, 27–52.
Saffran, J. R., Hauser, M., Seibel, R. L., Kapfhamer, J., Tsao, F., and Cushman, F. (2008). Grammatical pattern learning by infants and cotton-top tamarin monkeys. Cognition 107, 479–500.
Saffran, J. R., Newport, E. L., and Aslin, R. N. (1996a). Word segmentation: the role of distributional cues. J. Mem. Lang. 35, 606–621.
Saffran, J. R., Aslin, R. N., and Newport, E. L. (1996b). Statistical learning by 8-month old infants. Science 274, 1926–1928.
Saffran, J. R., Senghas, A., and Trueswell, J. C. (2001). The acquisition of language by children. Proc. Natl. Acad. Sci. U.S.A. 98, 12874–12875.
Schendel, Z. A., and Palmer, C. (2007). Suppression effects on musical and verbal memory. Mem. Cognit. 35, 640–650.
Schlaug, G., Jäncke, L., Huang, Y., Staiger, J. F., and Steinmetz, H. (1995). Increased corpus callosum size in musicians. Neuropsychologia 33, 1047–1055.
Schön, D., Boyer, M., Moreno, S., Besson, M., Peretz, I., and Kolinsky, R. (2008). Song as an aid for language acquisition. Cognition 106, 975–983.
Schön, D., Magne, C., and Besson, M. (2004). The music of speech: music training facilitates pitch processing in both music and language. Psychophysiology 41, 341–349.
Serafine, M. L., Crowder, R. G., and Repp, B. H. (1984). Integration of melody and text in memory for songs. Cognition 16, 285–303.
Sergent, C., Baillet, S., and Dehaene, S. (2005). Timing of the brain events underlying access to consciousness during the attentional blink. Nat. Neurosci. 8, 1391–1400.
Seth, A. K., Dienes, Z., Cleeremans, A., Overgaard, M., and Pessoa, L. (2008). Measuring consciousness: relating behavioural and neurophysiological approaches. Trends Cogn. Sci. 12, 314–321.
Shahin, A., Bosnyak, D. J., Trainor, L. J., and Roberts, L. E. (2003). Enhancement of neuroplastic P2 and N1c auditory evoked potentials in musicians. J. Neurosci. 23, 5545–5552.
Slevc, L. R., and Miyake, A. (2006). Individual differences in second language proficiency: does musical ability matter? Psychol. Sci. 17, 675–681.
Sluming, V., Barrick, T., Howard, M., Cezayirli, E., Mayes, A., and Roberts, N. (2002). Voxel-based morphometry reveals increased gray matter density in Broca’s area in male symphony orchestra musicians. Neuroimage 17, 1613–1622.
Spiegel, M. F., and Watson, C. S. (1984). Performance on frequency discrimination tasks by musicians and nonmusicians. J. Acoust. Soc. Am. 76, 1690–1695.
Steinbeis, N., and Koelsch, S. (2008). Comparing the processing of music and language meaning using EEG and FMRI provides evidence for similar and distinct neural representations. PLoS ONE 3, e2226.
Tallal, P., and Gaab, N. (2006). Dynamic auditory processing, musical experience and language development. Trends Neurosci. 29, 382–390.
Teinonen, T., Fellman, V., Näätänen, R., Alku, P., and Huotilainen, M. (2009). Statistical language learning in neonates revealed by event-related brain potentials. BMC Neurosci. 13, 10–21.
Thiessen, E. D., Hill, E., and Saffran, J. R. (2005). Infant-directed speech facilitates word segmentation. Infancy 7, 49–67.
Tillmann, B., Bharucha, J. J., and Bigand, E. (2000). Implicit learning of tonality: a self-organizing approach. Psychol. Rev. 107, 885–913.
Tillmann, B., Janata, P., and Bharucha, J. J. (2003). Activation of the inferior frontal cortex in musical priming. Cogn. Brain Res. 16, 145–161.
Tillmann, B., Koelsch, S., Escoffier, N., Bigand, E., Lalitte, P., Friederici, A. D., and Von Cramon, D. Y. (2006). Cognitive priming in sung and instrumental music: activation of inferior frontal cortex. Neuroimage 31, 1771–1782.
Tillmann, B., and McAdams, S. (2004). Implicit learning of musical timbre sequences: statistical regularities confronted with acoustical (dis)similarities. J. Exp. Psychol. Learn. Mem. Cogn. 30, 1131–1142.
Toro, J. M., and Trobalón, J. B. (2005). Statistical computations over a speech stream in a rodent. Percept. Psychophys. 67, 867–875.
Tremblay, K., Kraus, N., and McGee, T. (1998). The time course of auditory perceptual learning: neurophysiological changes during speech-sound training. Neuroreport 9, 3557–3560.
Van Petten, C., and Kutas, M. (1990). Interactions between sentence context and word frequency in event-related brain potentials. Mem. Cognit. 18, 380–393.
Van Zuijen, T. L., Sussman, E., Winkler, I., Näätänen, R., and Tervaniemi, M. (2005). Auditory organization of sound sequences by a temporal or numerical regularity: a mismatch negativity study comparing musicians and non-musicians. Brain Res. Cogn. Brain Res. 23, 270–276.
Yee, W., Holleran, S., and Jones, M. R. (1994). Sensitivity to event timing in regular and irregular sequences – influences of musical skill. Percept. Psychophys. 56, 461–471.
Keywords: statistical learning, word segmentation, musical expertise, music and language processing
Citation: Schön D and François C (2011) Musical expertise and statistical learning of musical and linguistic structures. Front. Psychology 2:167. doi: 10.3389/fpsyg.2011.00167
Received: 02 March 2011; Accepted: 04 July 2011;
Published online: 18 July 2011.
Edited by:
Lutz Jäncke, University of Zurich, SwitzerlandReviewed by:
Christo Pantev, University of Muenster, GermanyCopyright: © 2011 Schön and François. This is an open-access article subject to a non-exclusive license between the authors and Frontiers Media SA, which permits use, distribution and reproduction in other forums, provided the original authors and source are credited and other Frontiers conditions are complied with.
*Correspondence: Daniele Schön, Institut de Neurosciences Cognitives de la Méditerranée, 31 chemin J. Aiguier, 13402 Marseille, Cedex 20, France. e-mail:c2Nob25AaW5jbS5jbnJzLW1ycy5mcg==
Disclaimer: All claims expressed in this article are solely those of the authors and do not necessarily represent those of their affiliated organizations, or those of the publisher, the editors and the reviewers. Any product that may be evaluated in this article or claim that may be made by its manufacturer is not guaranteed or endorsed by the publisher.
Research integrity at Frontiers
Learn more about the work of our research integrity team to safeguard the quality of each article we publish.