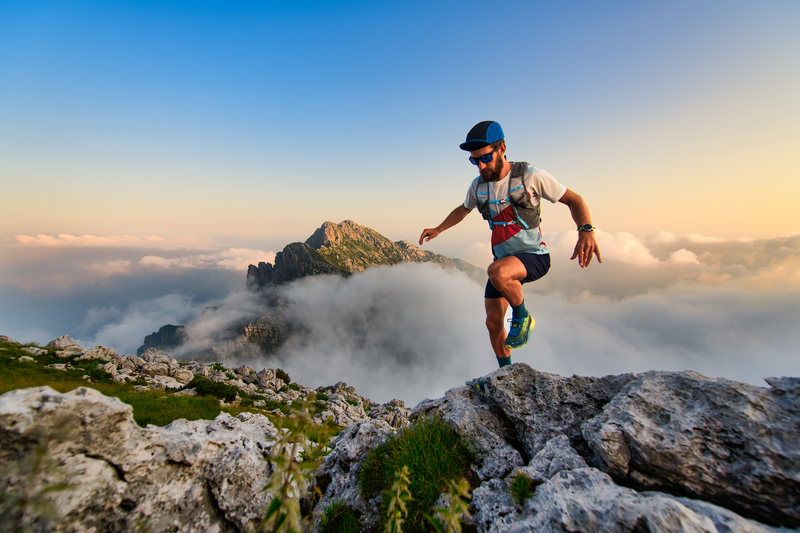
95% of researchers rate our articles as excellent or good
Learn more about the work of our research integrity team to safeguard the quality of each article we publish.
Find out more
ORIGINAL RESEARCH article
Front. Psychol. , 13 June 2011
Sec. Auditory Cognitive Neuroscience
volume 2 - 2011 | https://doi.org/10.3389/fpsyg.2011.00113
This article is part of the Research Topic The relationship between music and language View all 23 articles
Even in the quietest of rooms, our senses are perpetually inundated by a barrage of sounds, requiring the auditory system to adapt to a variety of listening conditions in order to extract signals of interest (e.g., one speaker’s voice amidst others). Brain networks that promote selective attention are thought to sharpen the neural encoding of a target signal, suppressing competing sounds and enhancing perceptual performance. Here, we ask: does musical training benefit cortical mechanisms that underlie selective attention to speech? To answer this question, we assessed the impact of selective auditory attention on cortical auditory-evoked response variability in musicians and non-musicians. Outcomes indicate strengthened brain networks for selective auditory attention in musicians in that musicians but not non-musicians demonstrate decreased prefrontal response variability with auditory attention. Results are interpreted in the context of previous work documenting perceptual and subcortical advantages in musicians for the hearing and neural encoding of speech in background noise. Musicians’ neural proficiency for selectively engaging and sustaining auditory attention to language indicates a potential benefit of music for auditory training. Given the importance of auditory attention for the development and maintenance of language-related skills, musical training may aid in the prevention, habilitation, and remediation of individuals with a wide range of attention-based language, listening and learning impairments.
“Attention is the holy grail. Everything that you’re conscious of, everything you let in, everything you remember and you forget, depends on it.”
D. Strayer1
The human nervous system is constantly faced with an astounding amount of sensory input. Despite the fact that our brains house over 10 billion neurons with more than 10 trillion synapses, accurate encoding of a complete environmental scene is a functional impossibility. Fortunately, the brain has evolved in ways that permit the modulation of neural activity according to environmental and systemic demands, permitting the selection, efficient encoding, and appropriate behavioral response to the stimuli of greatest biological interest. Selective attention makes this modulation possible, directing the allocation of neural resources to selectively encode one aspect of the environment while excluding competing aspects. Selective attention resolves the competition imposed by a mass of incoming signals through the activation of executive control regions (e.g., prefrontal cortex) to promote increased spiking in neurons that represent the attended object (Desimone and Duncan, 1995). Variability in the activation of brain networks that underlie selective attention can bring about significant behavioral disadvantages, such as attention lapses (Weissman et al., 2006) and symptoms of an attention impairment (Depue et al., 2010).
Recently, scientific pursuits concerning attention have invoked a hotbed of discussion. With the dawn of attention-fracturing devices such as portable music players, texting, and the internet, the act of sustaining attention on a single task may be rapidly fading into oblivion. More than ever, scientists need to determine the neural mechanisms that underlie attention, their behavioral outcomes, and how we might strengthen them with training and life experience. Here, we emphasize the discussion of auditory attention given its importance for language processing and the development and maintenance of language-related skills, such as hearing speech in background noise.
Event-related potentials (ERPs) have provided striking insights into the neuronal underpinnings of selective auditory attention (Hillyard et al., 1973; Woldorff et al., 1993; Coch et al., 2005), especially as it relates to everyday auditory function in noise. Standard experimental procedures imitate listening requirements in noisy environments by presenting separate sound streams to the right and left ears, asking participants to pay attention to one side while ignoring the other. When ERPs to the attended relative to the ignored sounds are compared, the negative deflection occurring at ~100 ms post-stimulus (i.e., the N100) is deeper in amplitude (i.e., more negative). Although more information is needed to precisely define the neuronal mechanisms that drive such outcomes and their malleability with training, development, and life experiences, these findings reveal that attention has the power to modulate early sensory processing.
The brains of musicians may provide insight into neural attention mechanisms and their potential for experience-dependent plasticity (Kraus and Chandrasekaran, 2010). Musical practice and performance require sustained attentional control for the delicate online manipulation of sound and, for ensemble players, to permit coordination with other instrumentalists. Given that musicians traditionally initiate training during early developmental years, attention to sound is regularly practiced during pivotal periods of brain development. Recent evidence from our laboratory indicates enhanced auditory but not visual attention ability in musicians relative to non-musicians, with musicians demonstrating faster reaction times to a target sound than non-musicians, but not to the task’s visual analog (Figure 1A; Strait et al., 2010; see Materials and Methods for task details). This finding may reflect decreased variability in musicians’ sustained auditory attention task performance. Surprisingly little is known about the impact of musical training on the neural correlates of attention. We do know, however, that cortical networks that promote attention to music share considerable overlap with those that underlie general attention in other auditory domains, such as language. In addition to the primary auditory cortex, these sites include the fronto-parietal attention and working memory networks that comprise the prefrontal cortex, the intraparietal sulcus, the supplementary and presupplementary motor areas, and the precentral gyrus (Janata et al., 2002; Kane and Engle, 2002). This functional overlap between attention to language and music corroborates previous results suggesting that a combination of modality-specific and general attention and working memory mechanisms (e.g., the fronto-parietal attention network) contribute to sustained auditory attention (Zatorre et al., 1999; Petkov et al., 2004). The prefrontal cortex has been particularly emphasized for its role in sustaining attention by providing access to recently presented stimuli and directs sensory processing according to behavioral goals – especially in challenging perceptual environments (Kane and Engle, 2002). Although we lack direct evidence for how musical training shapes brain mechanisms that underlie auditory attention performance, that musical training tunes the brain’s executive control network for auditory processing beyond the music domain – particularly for sustaining attention with minimal variability – would not be surprising.
Figure 1. Musicians, auditory attention, and processing speech in noise. We assessed auditory attention, speech-in-noise perception and auditory brainstem function in musicians and non-musicians (Parbery-Clark et al., 2009; Strait et al., 2010). Musicians demonstrated enhanced auditory attention as measured by reaction time (A) and were better able to accurately repeat sentences presented in noise at poorer signal-to-noise ratios than non-musicians (B). Auditory attention performance correlated with speech-in-noise perception across all subjects, with individuals having faster reaction times during a sustained attention task demonstrating better hearing in noise (C). Although both musicians and non-musicians demonstrated robust neural responses to a speech sound when presented in a quiet background, non-musicians’ responses were particularly degraded with the addition of a six-talker babble noise to the background. In both groups, the brainstem response waveform is positively correlated with the acoustic waveform of the stimulus. However, when the stimulus is presented in the presence of background noise musicians’ brainstem responses represent the stimulus more faithfully than non-musicians’ (D). *p < 0.05; **p < 0.01.
While little is known about the neural correlates of attention ability in musicians, it is well established that musical training strengthens cortical and subcortical mechanisms for auditory processing. Despite the fact that neural specializations for music and speech have been established (Zatorre et al., 2002; Brown et al., 2006; Abrams et al., 2010; Rogalsky et al., 2011), there is no doubt that the human brain also recruits shared mechanisms for processing sound in both domains (Koelsch et al., 2002; Patel, 2003; Zatorre and Gandour, 2008; Fedorenko et al., 2009; Slevc et al., 2009). Such shared mechanisms may account, at least in part, for musicians’ structural (Schmithorst and Wilke, 2002; Schneider et al., 2002; Gaser and Schlaug, 2003; Hutchinson et al., 2003; Schlaug et al., 2009) and functional enhancements for general auditory processing that are not constrained to the domain of music but that extend to language (Schon et al., 2004; Marques et al., 2007; Musacchia et al., 2007; Wong et al., 2007; Moreno et al., 2009) and emotional communication sounds (Strait et al., 2009). Neural enhancements are particularly evident in musicians in the context of challenging listening environments, such as in the presence of background noise (Parbery-Clark et al., 2009a) and reverberation (Bidelman and Krishnan, 2010), with musically trained adults demonstrating less noise-induced degradation in the subcortical encoding of speech than non-musicians (Figure 1D). The degree of noise-induced subcortical response degradation is functionally correlated with speech-in-noise perceptual ability in that individuals with increased subcortical resilience to background noise demonstrating better speech-in-noise perception (Parbery-Clark et al., 2009a). These findings imply that musicians’ nervous systems are fine-tuned for the extraction of meaning from a complex soundscape, being shaped through their extensive and consistent interactions with organized sound to better exclude competing noise and more accurately encode signals of interest.
The precise neurobiological mechanisms that bring about musical training-induced neuronal enhancements remain undetermined, although strengthened cognitive control over auditory processing, as would be directed by attention, provides a plausible agency (Strait and Kraus, in press; Strait et al., 2010). Increasing evidence has accrued to indicate that musicians more heavily recruit extra-sensory cortical areas associated with attention and working memory, such as the prefrontal, superior parietal, and inferior frontal cortices, during challenging auditory tasks that demand discriminatory alertness (e.g., when subjects are instructed to listen for certain auditory targets) compared to non-musicians (Stewart et al., 2003; Haslinger et al., 2005; Baumann et al., 2008). The prefrontal cortex has been attributed particular importance, being associated with goal-directed behavior and the top-down guiding of sensory processing according to internal states or intentions (Miller and Cohen, 2001). Whereas musically trained and non-trained adults demonstrate equivalent auditory cortex activation for the completion of pitch discrimination and sound recall tasks, musicians more extensively activate parietal and prefrontal extra-sensory networks – indicating more extensive involvement of attention and working memory networks that could facilitate heightened control over sensory processing (Gaab and Schlaug, 2003; Pallesen et al., 2010). Musicians’ recruitment of extra-sensory networks involved in attention and working memory may account for their enhanced performance on auditory tasks such as pitch discrimination (Kishon-Rabin et al., 2001; Parbery-Clark et al., 2009a; Strait et al., 2010), sound recall and hearing speech in noise (Figure 1; Parbery-Clark et al., 2009a, 2011).
That strengthened cognitive control mechanisms guide general auditory processing enhancements in musicians in a top-down manner is particularly viable given recent observations to this effect in animal models, in which auditory training leads to modifications in spectrotemporal tuning curves in the primary auditory cortex that appear to be facilitated by functional connections with the prefrontal cortex (cf. Bajo and King, 2010; Fritz et al., 2010). Even with regard to subcortical auditory plasticity, a primary role has been established for the reciprocal corticocollicular pathway, with training-induced changes in inferior collicular response properties being ablated with the targeted cooling of the cortex (Bajo et al., 2010). This is not surprising given the noted strength of cortical descending pathways in modulating subcortical (i.e., collicular) neuronal response properties (Suga et al., 2002). The resiliency of musicians’ nervous systems for encoding signals of interest in the presence of background noise (Figure 1D) may indicate increased executive control over auditory function, or, in other words, strengthened top-down attentional mechanisms within the primary auditory cortex that guide the resolution of competition imposed by a mass of incoming signals.
As noted, when multiple auditory streams are present in a scene, they compete for cortical representation. Selective auditory attention provides a mechanism for determining which sounds will be most thoroughly processed and brought to awareness, to the exclusion of others. It is unlikely, however, that the human brain is able to invariably maintain attention on a specific sound stream of interest over a sustained period of time. Consistently sustaining attention – with minimal attention lapses – is particularly difficult in input-rich sensory environments, such as when tracking a single individual’s voice amidst other conversations. Accordingly, Weissman and colleagues have demonstrated that brain regions associated with attention routinely demonstrate performance variability during the execution of sustained attention-demanding tasks, decreasing in activity while other brain regions increase in activity. Specifically, variability in the activation of the attention network during task performance has been linked to momentary lapses in attention, with continued activation of prefrontal and parietal regions underlying successful sustained attention performance (Weissman et al., 2006). Variability in the activation of prefrontal control regions is interpreted as the failure to accomplish attention’s goal, being to maximally and consistently enhance the sensory processing of behaviorally relevant stimuli. Decreases in the fronto-parietal network’s activation reduce its suppression of a default – or “daydreaming” – network, which corresponds with poorer attention task performance.
Here, we aimed to define the impact of musical training on neural networks underlying selective auditory attention performance in a natural language-listening environment. In light of the functional importance of sustained fronto-parietal attention network activation combined with musicians’ enhanced reliance on this network compared to non-musicians for the execution of auditory tasks, we asked two questions. First, we asked whether the act of sustaining auditory attention on a target speech stream leads to decreases in auditory-evoked response variability across all participants, especially within the primary auditory cortex and fronto-parietal attention areas. Second, we asked whether this decrease is larger in musicians. We hypothesized that musicians demonstrate less variability in neural responses to speech with auditory attention compared to non-musicians, particularly in prefrontal and parietal cortices, and that this decrease in variability correlates with musicians’ training backgrounds.
All experimental procedures were approved by the Northwestern University Institutional Review Board. Twenty-three normal hearing adults (≤20 dB pure tone thresholds at octave frequencies from 125 to 8000 Hz) between the ages of 18–35 participated in this study, for which they provided informed consent. All participants completed an extensive questionnaire addressing family history, musical experience and educational history. Musicians (Mus, N = 11) were self-categorized, began instrumental musical training at < age 7 and had consistently practiced for ≥11 years (consistently defined as practicing at least 3 days weekly; M = 16.5, SD = 5.8). Non-musicians (NonMus, N = 12) were self-categorized, had <5 years of formal musical experience throughout their lifespans (M = 1.2, SD = 1.8), began musical training after age 11 and had not played a musical instrument in the 5 years leading up to the experiment. Nine of the 12 NonMus participants had no degree of musical experience. Mus and NonMus groups did not differ according to age (F(1,22) = 0.20, p = 0.66), sex (χ2 = 0.35, p = 0.68), non-verbal I.Q. as measured by the Wechsler abbreviated matrix reasoning subtest (F(1,22) = 0.37, p = 0.55; Harcourt Assessment, San Antonio, TX, USA), or performance on the attention task (as measured by quiz scores, described below) (F(1,22) = 0.32, p = 0.58).
In order to clarify the relationship between speech-in-noise perception and auditory attention, we assessed these skills in 22 18- to 35-year-old musician and non-musician participants (N = 14; Mus = 8) using data collected for two separate experiments, the isolated results of which have since been published and are discussed above (Parbery-Clark et al., 2009a,b; Strait et al., 2010). Five of these participants also participated in the electrophysiological paradigm, described below. Speech-in-noise (SIN) perception was measured using the Hearing in Noise Test (Nilsson et al., 1994), in which participants are asked to repeat short sentences presented in speech-shaped background noise using a speaker placed one meter directly ahead. The noise presentation level was fixed at 65 dB SPL and the program adjusted perceptual difficulty by increasing or decreasing the intensity level of the target sentences until the threshold signal-to-noise ratio was determined. Perceptual SIN thresholds were defined as the level difference (in dB) between the speech and the noise presentation levels at which 50% of sentences are correctly repeated.
Auditory attention was assessed using the IHR Multicentre Battery of Auditory Processing’s Auditory Attention subtest (Barry et al., 2010), which measures phasic alertness via reaction times induced by the presence or absence of a cue that occurred with a variable delay (0.5–1.0 s) before a target stimulus. We have previously reported between-group differences using this measure in musicians and non-musicians, with musicians demonstrating enhanced performance compared to non-musicians (Figure 1A; Strait et al., 2010). Participants were instructed to listen for a “beep” (presented at 80 dB SPL) and to press a button on a response box as soon as they heard it. Participants were cued by a second sound (a “siren,” presented at 70 dB SPL) on some trials and were asked not to respond to that cue. Reaction time was measured in milliseconds. Results reported here (Figure 1C) reflect subjects’ average reaction time to the cued stimulus.
We employed a paradigm designed by Helen Neville and colleagues that has proven enormously successful for studying neural mechanisms of selective auditory attention in children and adults (Coch et al., 2005).
The evoking stimulus was a six-formant, 170 ms speech syllable synthesized in Klatt (1980) with a 5 ms voice onset time and a level fundamental frequency (100 Hz). The first, second and third formants were dynamic over the first 50 ms (F1, 400–720; F2, 1700–1240; F3, 2580–2500 Hz) and then maintained frequency for the rest of the duration. The fourth, fifth and sixth formants were constant throughout the entire duration of the stimulus (F4, 3300; F5, 3750; F6, 4900 Hz). The stimulus was presented using NeuroScan Stim2 (Compumedics, Charlotte, NC, USA).
Auditory-evoked potentials were recorded to the speech sound /da/ using a 31-channel tin-electrode cap (Electrocap International, Eaton, OH, USA) in NeuroScan Aquire 4.3 (Compumedics) while participants were seated in a sound-attenuated booth. Single electrodes were placed on the earlobes and on the superior and outer canthi of the left eye, thereby acting as reference and eye-blink monitors, respectively. Contact impedance for all electrodes was under <5 kΩ with less than 3 kΩ difference across channels. Neural recordings were on-line filtered from 0.05 to 100 Hz and digitally sampled at a rate of 500 Hz.
The evoking stimulus was presented in the context of short story recitations through two wall-mounted speakers located 1 m to the left and right of the participant. Participants were asked to attend to one of the two simultaneously presented stories, which differed in direction (left/right speaker), presentation voice (male/female), and story content. Instructions described both the direction of the attended story and its speaker’s sex (listen to the story on your right/left, which will be told by a male/female, and ignore the story presented from the other side by a speaker of the opposite sex). The initial direction of the attended voice was randomized across participants to control for potential advantages or disadvantages of attending to one voice over the other. The evoking stimulus was presented randomly to the left or right (i.e., attended or ignored) sides of the head with a randomized inter-stimulus interval (ISI) that was either 600, 900, or 1200 ms. The stories and the evoking stimulus were presented with a 10 dB difference between the stories (65 dB SPL) and the stimulus (75 dB SPL). The recording was paused every 8 min, during which participants were given one minute to complete a five-question multiple choice quiz regarding the attended story content and one minute to stretch. An average score of ≥4/5 correct answers was required for study inclusion. After each break, the attended story changed directions and participants were asked to change their attended side (left/right) in order to continue with the same voice. The entire recording session lasted 40 min and yielded 600 simultaneously recorded responses in both attended and ignored conditions.
Continuous neural data for attended and ignored conditions were baseline corrected and the removal of eye-blink artifacts was accomplished using the spatial filtering algorithm in NeuroScan Edit 4.3 (Compumedics). Response variability was computed through generation of a variability index (VI) for each subject in each condition, following a procedure described in Smith and Goffman (1998), who applied it to assess variability in speech movements. Continuous files were epoched from −100 to 500 ms, referenced to the presentation of the stimulus (0 ms); epochs demonstrating amplitudes beyond ±100 μV were rejected as muscular artifact and the first 500 artifact-free responses from each participant were subjected to analysis. Epochs were grouped into twenty subsets of 25 individual responses; these 25 individual responses in each subset were then averaged, resulting in 20 averaged waveforms (i.e., subaverages). The VI was determined through calculation of amplitude variances across these subaverages. Specifically, amplitudes were determined for each of the 300 points that made up the evoked response subaverages. Rather than comparing amplitudes across subaverages on a point-by-point basis, we averaged point-by-point amplitudes across 50 equally spaced increments (comprised of six points each), computed the variances in these increments across the subaverages and summed them. This generated a single VI for each subject in both attended and ignored conditions. Although evoked response variability has been previously assessed in humans (Anderson et al., 1991), our method is unique in that it enables the assessment of variance over the entire evoked response, including early evoked potentials that are not observable in individual evoked responses (P1/N1). Because these early components are small, we performed our analysis on small subaverages. All data processing was executed with scripts generated in Matlab 7.5.0 (The Mathworks, Natick, MA, USA).
Differences in response variability between attend and ignore conditions were compared for all of the 31 electrode sites using a Repeated Measures ANOVA. Effects at individual electrode sites (Figure 2) were subsequently explored using post-hoc paired and independent samples t-tests for all electrode sites except for F7, F8, O1, O2, and OZ, which did not demonstrate clear responses characteristic of cortical auditory-evoked activity (i.e., the P1–N1–P2–N2 complex; all other electrode sites demonstrated clear responses characteristic of cortical auditory-evoked activity). Relationships among musical practice histories (i.e., age of onset of musical practice, years of musical practice) and cortical variability were examined with Pearson’s correlations (SPSS Inc., Chicago, IL, USA). All results reported herein reflect two-tailed values and normality for all data was confirmed using the Kolmogorov–Smirnov test for equality.
Figure 2. Impact of attention on cortical auditory-evoked response variability at individual electrode sites. Electrode sites demonstrating a significant decrease in response variability in the attend relative to the ignore condition are in bold black font. Gray italics denote sites that were not subjected to individual analysis. Auditory-evoked activity recorded from PF1/PF2 demonstrated a decrease in variability with attention in musicians only (see Figure 3). ~p < 0.10; *p < 0.05; **p < 0.01; ***p < 0.001.
Within the subset of participants who had both measures (as described in Materials and Methods), auditory attention performance correlated with speech-in-noise perceptual ability, with better auditory attention relating to the ability to accurately perceive speech in higher levels of background noise (i.e., at lower signal-to-noise ratios; Figure 1C).
All participants demonstrated less cortical auditory-evoked response variability over a majority of electrode sites in responses to the stimulus when it was presented in the attended compared to the ignored story (Figure 2). Only musicians, however, demonstrated decreased cortical response variability with auditory attention over the prefrontal cortex (Figure 3), a region of particular importance for sustaining attention in challenging perceptual environments (Kane and Engle, 2002). The degree to which attention decreased prefrontal response variability correlated with musical practice histories and is interpreted in the context of musical training’s impact on cortical mechanisms of selective auditory attention.
Figure 3. Cortical auditory-evoked response variability in musicians and non-musicians. (A) 31-Channel headplots for musicians (left) and non-musicians (right) demonstrate the difference in cortical auditory-evoked response variability between ignore and attention conditions, plotting variability across the scalp as a function of attention. Because the difference was calculated by subtracting attend from ignore variability, positive values (red) indicate a decrease in response variability in the attend relative to the ignore condition. Negative values (blue) indicate an increase in response variability in the attend relative to the ignore condition. (B) Musicians demonstrate an increased impact of attention on prefrontal response variability compared to non-musicians. Whereas musicians demonstrate a decrease in prefrontal response variability in the attend relative to the ignore condition, non-musicians do not. **p < 0.01.
A 2 × 31 × 2 RMANOVA with condition (attend/ignore) and electrode site as within subject variables and group (Mus/NonMus) as between subject variable revealed a main effect of condition on response variability, indicating that cortical response variability varied as a function of attention (F(1,22) = 10.49, p < 0.005). We also observed an interaction between condition electrode site, indicating that attention impacted response variability differentially across the scalp (F(1,22) = 17.08, p < 0.0001), and an anticipated main effect of electrode site (F(1,22) = 17.49, p < 0.0001). Post hoc paired t-tests demonstrated that, across all participants, response variability decreased to stimuli in the attended compared to the ignored story at all analyzed electrode sites except for seven (for these seven sites, all t(22) < 1.4, all p ≥ 0.2; Figure 2). An overall effect of attention across all participants was not observed for the three prefrontal sites (F(1,22) = 0.31, p = 0.40).
Musicians and non-musicians did not differ based on overall response variability at any electrode site. That is, neither group was more or less variable in auditory-evoked responses to the ignored or to the attended stories individually, indicating no group difference in general auditory-evoked variability. Rather, differences between musicians and non-musicians were observed with regard to the extent to which attention decreased auditory-evoked response variability at prefrontal electrode sites. Specifically, a 2 × 2 RMANOVA with condition (attend/ignore) as within subject variable and group (Mus/NonMus) as between subject variable revealed a significant condition–group interaction at prefrontal electrode sites FP1 and FP2 (F(1,22) = 10.21, p < 0.005). Post hoc within-group paired t-tests demonstrated that whereas musicians demonstrated decreased response variability over the prefrontal cortex with auditory attention (t(10) = 3.0, p < 0.01), non-musicians did not (t(11) = 1.6, p = 0.2; Figure 3B). Differences in response variability with attention between musicians and non-musicians were not observed for any of the other electrode sites (all t < 1.4, all p > 0.12).
Across all participants with some degree of musical training (N = 14; Mus = 11, NonMus = 3), the age of onset of musical training correlated with the extent to which response variability decreased in responses to the attended relative to the ignored story (r = −0.54, p < 0.05).
Here, we substantiate a relationship between auditory attention performance and speech-in-noise perception (Figure 1C) and reveal a novel neural index for selective auditory attention in musician and non-musician adults, consisting of decreased auditory-evoked response variability in attended relative to ignored speech. Across all participants, attention decreased cortical auditory-evoked response variability at central, temporal, and parietal sites (Figure 2), and this effect was equivalent in musicians and non-musicians. Only musicians, however, demonstrated an impact of selective auditory attention on prefrontal evoked activity (Figure 3). These results provide evidence for the power of musical training to shape prefrontal neural activity involved in sustaining auditory attention and may contribute to the definition of a biological mechanism that would facilitate musicians’ advantages in auditory tasks (Kraus and Chandrasekaran, 2010; Strait et al., 2010).
The goal of sustaining attention on a specific task is to reduce moment-to-moment variability in one’s performance. Sustaining attention becomes particularly difficult in the presence of competing stimuli, such as when tracking a single voice amidst a noisy background. In this situation it is the listener’s goal to absorb the entirety of the attended speaker’s content in order to adequately respond, and lapses in attention result in comprehension gaps that can lead to conversational confusion. Variability in attention performance (i.e., lapses in attention) can also have more drastic consequences, being responsible for accidents while operating mechanical equipment (e.g., cars) and, in educational scenarios, has the potential to diminish the quality of learning that takes place in young brains (Vaurio et al., 2009).
Moment-to-moment behavioral variability has been directly linked with variability in the brain’s extra-sensory evoked activity during task performance (i.e., prefrontal, frontal, and parietal cortices; Carmena et al., 2005; Fox et al., 2005; Weissman et al., 2006). As tasks become more difficult, cortical response variability increases, concurrent with poorer task performance (Vogels et al., 1989). Given that the most frequent analysis technique for electrophysiologic data involves averaging, scientists might regularly overlook a crucially informative neural index for attention and human behavior. This is because evoked potentials traditionally necessitate the averaging of many individual responses to a repeated stimulus in order to maximize that which is consistent across trials (i.e., the average evoked response), effectively minimizing that which is inconsistent and discarding it as noise. This disregarded “noise,” or response variability, is often as large or even larger than the average response itself (Figure 4; Vogels et al., 1989; Softky and Koch, 1993; Arieli et al., 1996). Arieli et al. (1996) encouraged the revision of what we regard as noise in the nervous system, proposing that in doing so we may discover that “response variability… provide[s] the neuronal substrate for the dependence of sensory information processing on behavioral and conscious states”. The data we present here corroborate Arieli’s suggestion by demonstrating a functional relevance for variability in cortical evoked potentials in humans, serving as an index for selective auditory attention. Further work comparing cortical response variability with more commonly employed techniques for analyzing electrophysiologic recordings is likely to reveal relationships between response variability and both spontaneous and averaged evoked activity, such as average peak amplitudes/latencies and oscillatory activity within different frequency bands.
Figure 4. Variability in evoked neural activity from intracranial recordings in the cat visual cortex (areas 17 and 18). The local field potential (LFP) and spike discharges of two isolated neurons were simultaneously recorded from a microelectrode in response to repetitive visual stimulation that occurred every 3.5 ms (see Arieli et al., 1996 for further information). Variability in neuronal activity can be seen within (A) trial-by-trial LFPs as well as (B) within the spike trains of individual neurons contributing to the LFP.
Although it is possible that cortical evoked response variability stems, at least in part, from stochastic noise (Faisal et al., 2008), evoked response variability can be predicted by deterministic interactions of sensory responses with ongoing spontaneous activity (Arieli et al., 1996; Curto et al., 2009) that can be modulated by an individual’s brain state (Steriade et al., 2001) and cognitive capacity (Benasich et al., 2008). The decreased evoked response variability with selective auditory attention demonstrated here may indicate general changes in ongoing spontaneous activity between attended and ignored states, revealing a novel neural metric for selective auditory attention in behaving humans. Furthermore, group differences as a function of musical training reflect more consistent prefrontal activity in musicians with auditory attention, which may translate into increased control over the sensory competition imposed by competing auditory signals. This implication is particularly relevant given the role of the prefrontal cortex in directing goal-oriented behavior and the top-down shaping of sensory processing according to internal states or intentions (Miller and Cohen, 2001). Further studies coupling behavioral and neural indices of selective auditory attention are needed in order to better define the functional advantage of decreased prefrontal response variability in musicians. Furthermore, simultaneous recording of cortical and subcortical evoked activity may shed light on relationships between prefrontal response variability and subcortical response properties, such as to speech in background noise (Figure 1D).
Our results demonstrate a selective impact of musical training on response variability with attention at prefrontal electrode sites. This outcome contributes to a growing literature suggesting that musical training shapes auditory function by training the brain to more extensively recruit extra-sensory mechanisms affiliated with cognitive control, such as working memory and attention, for the completion of general auditory tasks (Gaab and Schlaug, 2003; Stewart et al., 2003; Haslinger et al., 2005; Baumann et al., 2008; Pallesen et al., 2010). Previous experiments, however, have not explicitly investigated neural mechanisms of auditory attention in musicians but, rather, studied musicians’ brain function during the execution of psychophysical auditory discrimination and memory tasks. Our data provide the first direct evidence for differential brain activation in musicians and non-musicians during selective auditory attention to speech. That these data are observed in an ecologically valid language-listening environment strengthens arguments for musical training’s impact on functional brain networks that underlie language processing.
Our findings may indicate that musicians demonstrate more consistent ongoing (i.e., spontaneous) prefrontal activity during selective auditory attention, compared to non-musicians. As described above, the dynamics of ongoing neural activity convincingly predict variability in cortical evoked responses. Specifically, evoked activity is low when spontaneous activity is low and evoked activity is high when spontaneous activity is high, with spontaneous and evoked activity positively correlating at an impressive r = 0.9 and p < 10−12 (Arieli et al., 1996). Decreased variability in musicians’ responses would imply increased consistency in ongoing prefrontal activity and, given the importance of consistency for sustaining attention (Weissman et al., 2006), provides a biological mechanism that could account for our previously reported advantage for sustained attention task performance in musicians (Figure 1A; Strait et al., 2010).
Distinctive neural activity during selective auditory attention in musicians and non-musicians may be attributed to the musicians’ rehearsal of auditory cognitive mechanisms required for focused musical practice and performance, strengthening top-down contributors to auditory processing (Tervaniemi et al., 2009; Kraus and Chandrasekaran, 2010; Strait et al., 2010). Although the argument can be made for a genetic contributor to structural and functional neural differences between musicians and non-musicians, repeated evidence substantiates that these differences can be modulated, at least in part, by one’s method of musical practice (Seppanen et al., 2007) or instrument of specialization (Pantev et al., 2001; Shahin et al., 2008; Margulis et al., 2009; Strait et al., 2011). Furthermore, data consistently reveal correlations between the extent of neural enhancement observed in musicians and their years of musical practice or age of practice onset (Gaser and Schlaug, 2003; Hutchinson et al., 2003; Wong et al., 2007; Parbery-Clark et al., 2009a,b; Strait et al., 2009). These data, together with the correlation reported here between prefrontal response variability and age of onset of musical practice, suggest a contribution of experience-induced neuroplasticity to musicians’ auditory processing characteristics.
That musical training has the power to shape neural mechanisms underlying selective attention to speech carries substantial implications for educators and clinicians involved in the remediation of attention-based listening and learning impairments. The ability to attend to a target signal and suppress competing noise is a primary concern for child educators and clinicians given its primacy in everyday learning and communication. It is also of concern to those involved in the treatment of aging-induced listening impairment, which may be prevented through the strengthening of auditory cognitive abilities, such as attention (Parbery-Clark et al., 2011). Accordingly, interest in learning to attend has increased in recent years (Tang and Posner, 2009); within the visual domain, outcomes reveal that task-specific training can improve the temporal allocation of attention (Makovski et al., 2008) and, as required by our paradigm here, increases the neural capacity to filter out competing irrelevant input (Dixon et al., 2009; Kelley and Yantis, 2009). Musical training may provide a naturalistic and entertaining means for strengthening auditory cognitive processing through increasing the consistency of prefrontal control over auditory function.
Although improving attention and the ability to tune in to a signal of interest would benefit the general population, the topic of behavioral and neural variability during selective attention has particular relevance for attention deficit/hyperactivity disorder (ADHD). This is because ADHD is characterized by moment-to-moment variability in behavioral performance (Mullins et al., 2005; Vaurio et al., 2009) and neuronal activity (Depue et al., 2010). Furthermore, structural and functional prefrontal anomalies have been associated with the disorder (Hynd et al., 1990; Casey et al., 1997; Filipek et al., 1997; Gilliam et al., 2011) and are reflected in decreased prefrontal activity during attention task performance (Bush et al., 1999; Rubia et al., 1999). Children with ADHD are particularly noted for an inability to suppress the neural processing of competing sensory input (Suskauer et al., 2008), contributing to frequent distraction. Our association between musical training and decreased prefrontal variability in a neuronal mechanism that underlies selective attention to language during the simultaneous suppression of a competing sound stream may suggest musical training as a viable remediation strategy in children with attention impairment. Still, more work should be done to test the efficacy of music as a remedial approach for ADHD. Population studies investigating the prevalence of ADHD in children and adults with musical training, particularly those with a family history of ADHD, could yield interesting insights.
Increasing effort is being expended to define activities that strengthen what might be considered the cornerstone of human perception: attention. While musical training is known to bolster auditory-specific cognitive skills, such as auditory short-term memory, and the ability to pull out speech signals from competing background noise, little is known about how musical training strengthens attention; even less is known about how music shapes the neural mechanisms that underlie it. Here, we present the first biological evidence for musical training’s impact on neural mechanisms of selective auditory attention within a language context. Given the high prevalence of developmental attention disorders and their detrimental impacts on educational performance, musical training’s power to shape neural mechanisms that underlie selective attention to speech may be of interest to individuals involved in the habilitation and remediation of attention and attention-based learning impairment.
The authors declare that the research was conducted in the absence of any commercial or financial relationships that could be construed as a potential conflict of interest.
The authors wish to thank V. Abecassis for his assistance with data collection, T. Nicol for his contributions to the variability analyses and T. Nicol, A. Parbery-Clark, and K. Chan for their constructive comments on a previous version of this manuscript. This work was supported by NIH F31DC011457-01, NSF 0921275, and a Grammy Foundation Research Grant.
Abrams, D. A., Bhatara, A., Ryali, S., Balaban, E., Levitin, D. J., and Menon, V. (2010). Decoding temporal structure in music and speech relies on shared brain resources but elicits different fine-scale spatial patterns. Cereb. Cortex. doi: 10.1093/cercor/bhq198. [Epub ahead of print].
Anderson, J., Rennie, C., Gordon, E., Howson, A., and Meares, R. (1991). Measurement of maximum variability within event related potentials in schizophrenia. Psychiatry Res. 39, 33–44.
Arieli, A., Sterkin, A., Grinvald, A., and Aertsen, A. (1996). Dynamics of ongoing activity: explanation of the large variability in evoked cortical responses. Science 273, 1868–1871.
Bajo, V. M., Nodal, F. R., Moore, D. R., and King, A. J. (2010). The descending corticocollicular pathway mediates learning-induced auditory plasticity. Nat. Neurosci. 13, 253–260.
Barry, J. G., Ferguson, M. A., and Moore, D. R. (2010). Making sense of listening: the IMAP test battery. J. Vis. Exp. 44.
Baumann, S., Meyer, M., and Jancke, L. (2008). Enhancement of auditory-evoked potentials in musicians reflects an influence of expertise but not selective attention. J. Cogn. Neurosci. 20, 2238–2249.
Benasich, A. A., Gou, Z., Choudhury, N., and Harris, K. D. (2008). Early cognitive and language skills are linked to resting frontal gamma power across the first 3 years. Behav. Brain Res. 195, 215–222.
Bidelman, G. M., and Krishnan, A. (2010). Effects of reverberation on brainstem representation of speech in musicians and non-musicians. Brain Res. 1355, 112–125.
Brown, S., Martinez, M. J., and Parsons, L. M. (2006). Music and language side by side in the brain: a PET study of the generation of melodies and sentences. Eur. J. Neurosci. 23(10), 2791–2803.
Bush, G., Frazier, J. A., Rauch, S. L., Seidman, L. J., Whalen, P. J., Jenike, M. A., Rosen, B. R., and Biederman, J. (1999). Anterior cingulate cortex dysfunction in attention-deficit/hyperactivity disorder revealed by fMRI and the counting Stroop. Biol. Psychiatr. 45, 1542–1552.
Carmena, J. M., Lebedev, M. A., Henriquez, C. S., and Nicolelis, M. A. (2005). Stable ensemble performance with single-neuron variability during reaching movements in primates. J. Neurosci. 25, 10712–10716.
Casey, B. J., Castellanos, F. X., Giedd, J. N., Marsh, W. L., Hamburger, S. D., Schubert, A. B., Vaituzis, A. C., Dickstein, D. P., Sarfatti, S. E., and Rapoport, J. L. (1997). Implication of right frontostriatal circuitry in response inhibition and attention-deficit/hyperactivity disorder. J. Am. Acad. Child Adolesc. Psychiatr. 36, 374–383.
Coch, D., Sanders, L. D., and Neville, H. J. (2005). An event-related potential study of selective auditory attention in children and adults. J. Cogn. Neurosci. 17, 605–622.
Curto, C., Sakata, S., Marguet, S., Itskov, V., and Harris, K. D. (2009). A simple model of cortical dynamics explains variability and state dependence of sensory responses in urethane-anesthetized auditory cortex. J. Neurosci. 29, 10600–10612.
Depue, B. E., Burgess, G. C., Willcutt, E. G., Bidwell, L. C., Ruzic, L., and Banich, M. T. (2010). Symptom-correlated brain regions in young adults with combined-type ADHD: their organization, variability, and relation to behavioral performance. Psychiatry Res. 182, 96–102.
Desimone, R., and Duncan, J. (1995). Neural mechanisms of selective visual attention. Annu. Rev. Neurosci. 18, 193–222.
Dixon, M. L., Ruppel, J., Pratt, J., and De Rosa, E. (2009). Learning to ignore: acquisition of sustained attentional suppression. Psychon. Bull. Rev. 16, 418–423.
Faisal, A. A., Selen, L. P., and Wolpert, D. M. (2008). Noise in the nervous system. Nat. Rev. Neurosci. 9, 292–303.
Fedorenko, E., Patel, A., Casasanto, D., Winawer, J., and Gibson, E. (2009). Structural integration in language and music: evidence for a shared system. Mem. Cognit. 37, 1–9.
Filipek, P. A., Semrud-Clikeman, M., Steingard, R. J., Renshaw, P. F., Kennedy, D. N., and Biederman, J. (1997). Volumetric MRI analysis comparing subjects having attention-deficit hyperactivity disorder with normal controls. Neurology 48, 589–601.
Fox, M. D., Snyder, A. Z., Vincent, J. L., Corbetta, M., Van Essen, D. C., and Raichle, M. E. (2005). The human brain is intrinsically organized into dynamic, anticorrelated functional networks. Proc. Natl. Acad. Sci. U.S.A. 102, 9673–9678.
Fritz, J. B., David, S. V., Radtke-Schuller, S., Yin, P., and Shamma, S. A. (2010). Adaptive, behaviorally gated, persistent encoding of task-relevant auditory information in ferret frontal cortex. Nat. Neurosci. 13, 1011–1019.
Gaab, N., and Schlaug, G. (2003). The effect of musicianship on pitch memory in performance matched groups. Neuroreport 14, 2291–2295.
Gaser, C., and Schlaug, G. (2003). Brain structures differ between musicians and non-musicians. J. Neurosci. 23, 9240–9245.
Gilliam, M., Stockman, M., Malek, M., Sharp, W., Greenstein, D., Lalonde, F., Clasen, L., Giedd, J., Rapoport, J., and Shaw, P. (2011). Developmental trajectories of the corpus callosum in attention-deficit/hyperactivity disorder. Biol. Psychiatry. 69, 839–846.
Haslinger, B., Erhard, P., Altenmuller, E., Schroeder, U., Boecker, H., and Ceballos-Baumann, A. O. (2005). Transmodal sensorimotor networks during action observation in professional pianists. J. Cogn. Neurosci. 17, 282–293.
Hillyard, S. A., Hink, R. F., Schwent, V. L., and Picton, T. W. (1973). Electrical signs of selective attention in the human brain. Science 182, 177–180.
Hutchinson, S., Lee, L. H., Gaab, N., and Schlaug, G. (2003). Cerebellar volume of musicians. Cereb. Cortex 13, 943–949.
Hynd, G. W., Semrud-Clikeman, M., Lorys, A. R., Novey, E. S., and Eliopulos, D. (1990). Brain morphology in developmental dyslexia and attention deficit disorder/hyperactivity. Arch. Neurol. 47, 919–926.
Janata, P., Tillmann, B., and Bharucha, J. J. (2002). Listening to polyphonic music recruits domain-general attention and working memory circuits. Cogn. Affect. Behav. Neurosci. 2, 121–140.
Kane, M. J., and Engle, R. W. (2002). The role of prefrontal cortex in working-memory capacity, executive attention, and general fluid intelligence: an individual-differences perspective. Psychon. Bull. Rev. 9, 637–671.
Kelley, T. A., and Yantis, S. (2009). Learning to attend: effects of practice on information selection. J. Vis. 9, 16.
Kishon-Rabin, L., Amir, O., Vexler, Y., and Zaltz, Y. (2001). Pitch discrimination: are professional musicians better than non-musicians? J. Basic Clin. Physiol. Pharmacol. 12(2 Suppl.), 125–143.
Klatt, D. (1980). Software for a cascade/parallel formant synthesizer. J. Acoust. Soc. Amer. 67, 13–33.
Koelsch, S., Gunter, T. C., Cramon, D. Y., Zysset, S., Lohmann, G., and Friederici, A. D. (2002). Bach speaks: a cortical “language-network” serves the processing of music. Neuroimage 17, 956–966.
Kraus, N., and Chandrasekaran, B. (2010). Music training for the development of auditory skills. Nat. Rev. Neurosci. 11, 599–605.
Makovski, T., Vázquez, G. A., and Jiang, Y. V. (2008). Visual learning in multiple-object tracking. PLoS ONE 3, e2228. doi: 10.1371/journal.pone.0002228
Margulis, E. M., Mlsna, L. M., Uppunda, A. K., Parrish, T. B., and Wong, P. C. M. (2009). Selective neurophysiologic responses to music in instrumentalists with different listening biographies. Hum Brain Mapp 30, 267–275.
Marques, C., Moreno, S., Castro, S. L., and Besson, M. (2007). Musicians detect pitch violation in a foreign language better than nonmusicians: behavioral and electrophysiological evidence. J. Cogn. Neurosci. 19, 1453–1463.
Miller, E. K., and Cohen, J. D. (2001). An integrative theory of prefrontal cortex function. Annu. Rev. Neurosci. 24, 167–202.
Moreno, S., Marques, C., Santos, A., Santos, M., Castro, S. L., and Besson, M. (2009). Musical training influences linguistic abilities in 8-year-old children: more evidence for brain plasticity. Cereb. Cortex 19, 712–723.
Mullins, C., Bellgrove, M. A., Gill, M., and Robertson, I. H. (2005). Variability in time reproduction: difference in ADHD combined and inattentive subtypes. J. Am. Acad. Child Adolesc. Psychiatry 44, 169–176.
Musacchia, G., Sams, M., Skoe, E., and Kraus, N. (2007). Musicians have enhanced subcortical auditory and audiovisual processing of speech and music. Proc. Natl. Acad. Sci. U.S.A. 104, 15894–15898.
Nilsson, M., Soli, S., and Sullivan, J. (1994). Development of the hearing in noise test for the measurement of speech reception thresholds in quiet and in noise. J. Acoust. Soc. Am. 95, 1085–1099.
Pallesen, K. J., Brattico, E., Bailey, C. J., Korvenoja, A., Koivisto, J., Gjedde, A., and Carlson, S. (2010). Cognitive control in auditory working memory is enhanced in musicians. PLoS ONE 5, e11120. doi: 10.1371/journal.pone.0011120
Pantev, C., Roberts, L. E., Schulz, M., Engelien, A., and Ross, B. (2007). Timbre-specific enhancement of auditory cortical representations in musicians. Neurorep 12, 169–174.
Parbery-Clark, A., Skoe, E., and Kraus, N. (2009a). Musical experience limits the degradative effects of background noise on the neural processing of sound. J. Neurosci. 29, 14100–14107.
Parbery-Clark, A., Skoe, E., Lam, C., and Kraus, N. (2009b). Musician enhancement for speech-in-noise. Ear Hear. 30, 653–661.
Parbery-Clark, A., Strait, D. L., Anderson, S., Hittner, E., and Kraus, N. (2011). Musical experience and the aging auditory system: implications for cognitive abilities and hearing speech in noise. PLoS ONE 6, e18082. doi: 10.1371/journal.pone.0018082
Petkov, C. I., Kang, X., Alho, K., Bertrand, O., Yund, E. W., and Woods, D. L. (2004). Attentional modulation of human auditory cortex. Nat. Neurosci. 7, 658–663.
Rogalsky, C., Rong, F., Saberi, K., and Hickok, G. (2011). Functional anatomy of language and music perception: temporal and structural factors investigated using functional magnetic resonance imaging. J. Neurosci. 31, 3843–3852.
Rubia, K., Overmeyer, S., Taylor, E., Brammer, M., Williams, S. C., Simmons, A., and Bullmore, E. T. (1999). Hypofrontality in attention deficit hyperactivity disorder during higher-order motor control: a study with functional MRI. Am. J. Psychiatry 156, 891–896.
Schlaug, G., Forgeard, M., Zhu, L., Norton, A., and Winner, E. (2009). Training-induced neuroplasticity in young children. Ann. N. Y. Acad. Sci. 1169, 205–208.
Schmithorst, V. J., and Wilke, M. (2002). Differences in white matter architecture between musicians and non-musicians: a diffusion tensor imaging study. Neurosci. Lett. 321, 57–60.
Schneider, P., Scherg, M., Dosch, H. G., Specht, H. J., Gutschalk, A., and Rupp, A. (2002). Morphology of Heschl’s gyrus reflects enhanced activation in the auditory cortex of musicians. Nat. Neurosci. 5, 688–694.
Schon, D., Magne, C., and Besson, M. (2004). The music of speech: music training facilitates pitch processing in both music and language. Psychophysiology 41, 341–349.
Seppanen, M., Brattico, E., and Tervaniemi, M. (2007). Practice strategies of musicians modulate neural processing and the learning of sound-patterns. Neurobiol. Learn. Mem. 87, 236–247.
Shahin, A. J., Roberts, L. E., Chau, W., Trainor, L. J., and Miller, L. M. (2008). Music training leads to the development of timbre-specific gamma band activity. NeuroImage 41, 113–132.
Slevc, L. R., Rosenberg, J. C., and Patel, A. D. (2009). Making psycholinguistics musical: self-paced reading time evidence for shared processing of linguistic and musical syntax. Psychon. Bull. Rev. 16, 374–381.
Smith, A., and Goffman, L. (1998). Stability and patterning of speech movement sequences in children and adults. J. Speech Lang. Hear. Res. 41, 18–30.
Softky, W. R., and Koch, C. (1993). The highly irregular firing of cortical cells is inconsistent with temporal integration of random EPSPs. J. Neurosci. 13, 334–350.
Steriade, M., Timofeev, I., and Grenier, F. (2001). Natural waking and sleep states: a view from inside neocortical neurons. J. Neurophysiol. 85, 1969–1985.
Stewart, L., Henson, R., Kampe, K., Walsh, V., Turner, R., and Frith, U. (2003). Brain changes after learning to read and play music. Neuroimage 20, 71–83.
Strait, D. L., Chan, K., Ashley, R., and Kraus, N. (2011). Specialization among the specialized: auditory brainstem function is tuned in to timbre. Cortex. doi: 10.1016/j.cortex.2011.03.015. [Epub ahead of print].
Strait, D. L., and Kraus, N. (in press). Playing music for a smarter ear: cognitive, perceptual and neurobiological evidence. Music Percept.
Strait, D. L., Kraus, N., Parbery-Clark, A., and Ashley, R. (2010). Musical experience shapes top-down auditory mechanisms: evidence from masking and auditory attention performance. Hear Res. 261, 22–29.
Strait, D. L., Kraus, N., Skoe, E., and Ashley, R. (2009). Musical experience and neural efficiency: effects of training on subcortical processing of vocal expressions of emotion. Eur. J. Neurosci. 29, 661–668.
Suga, N., Xiao, Z., Ma, X., and Ji, W. (2002). Plasticity and corticofugal modulation for hearing in adult animals. Neuron 36, 9–18.
Suskauer, S. J., Simmonds, D. J., Fotedar, S., Blankner, J. G., Pekar, J. J., Denckla, M. B., and Mostofsky, S. H. (2008). Functional magnetic resonance imaging evidence for abnormalities in response selection in attention deficit hyperactivity disorder: differences in activation associated with response inhibition but not habitual motor response. J. Cogn. Neurosci. 20, 478–493.
Tang, Y. Y., and Posner, M. I. (2009). Attention training and attention state training. Trends Cogn. Sci. 13, 222–227.
Tervaniemi, M., Kruck, S., De Baene, W., Schroger, E., Alter, K., and Friederici, A. D. (2009). Top-down modulation of auditory processing: effects of sound context, musical expertise and attentional focus. Eur. J. Neurosci. 30, 1636–1642.
Vaurio, R. G., Simmonds, D. J., and Mostofsky, S. H. (2009). Increased intra-individual reaction time variability in attention-deficit/hyperactivity disorder across response inhibition tasks with different cognitive demands. Neuropsychologia 47, 2389–2396.
Vogels, R., Spileers, W., and Orban, G. A. (1989). The response variability of striate cortical neurons in the behaving monkey. Exp. Brain Res. 77, 432–436.
Weissman, D. H., Roberts, K. C., Visscher, K. M., and Woldorff, M. G. (2006). The neural bases of momentary lapses in attention. Nat. Neurosci. 9, 971–978.
Woldorff, M. G., Gallen, C. C., Hampson, S. A., Hillyard, S. A., Pantev, C., Sobel, D., and Bloom, F. E. (1993). Modulation of early sensory processing in human auditory cortex during auditory selective attention. Proc. Natl. Acad. Sci. U.S.A. 90, 8722–8726.
Wong, P. C., Skoe, E., Russo, N. M., Dees, T., and Kraus, N. (2007). Musical experience shapes human brainstem encoding of linguistic pitch patterns. Nat. Neurosci. 10, 420–422.
Zatorre, R. J., Belin, P., and Penhune, V. B. (2002). Structure and function of auditory cortex: music and speech. Trends Cogn. Sci. 6, 37–46.
Zatorre, R. J., and Gandour, J. T. (2008). Neural specializations for speech and pitch: moving beyond the dichotomies. Philos. Trans. R. Soc. Lond. B Biol. Sci. 363, 1087–1104.
Keywords: attention, cortical variability, auditory-evoked potentials, music, musicians, speech in noise, prefrontal cortex, language
Citation: Strait DL and Kraus N (2011) Can you hear me now? Musical training shapes functional brain networks for selective auditory attention and hearing speech in noise. Front. Psychology 2:113. doi: 10.3389/fpsyg.2011.00113
Received: 15 February 2011;
Accepted: 14 May 2011;
Published online: 13 June 2011.
Edited by:
Lutz Jäncke, University of Zurich, SwitzerlandReviewed by:
Mireille Besson, Centre National de la Recherche Scientifique, FranceCopyright: © 2011 Strait and Kraus. This is an open-access article subject to a non-exclusive license between the authors and Frontiers Media SA, which permits use, distribution and reproduction in other forums, provided the original authors and source are credited and other Frontiers conditions are complied with.
*Correspondence: Nina Kraus, Auditory Neuroscience Laboratory, Northwestern University, 2240 Campus Drive, Evanston, IL 60208, USA. e-mail:bmtyYXVzQG5vcnRod2VzdGVybi5lZHU=http://www.brainvolts.northwestern.edu
Disclaimer: All claims expressed in this article are solely those of the authors and do not necessarily represent those of their affiliated organizations, or those of the publisher, the editors and the reviewers. Any product that may be evaluated in this article or claim that may be made by its manufacturer is not guaranteed or endorsed by the publisher.
Research integrity at Frontiers
Learn more about the work of our research integrity team to safeguard the quality of each article we publish.