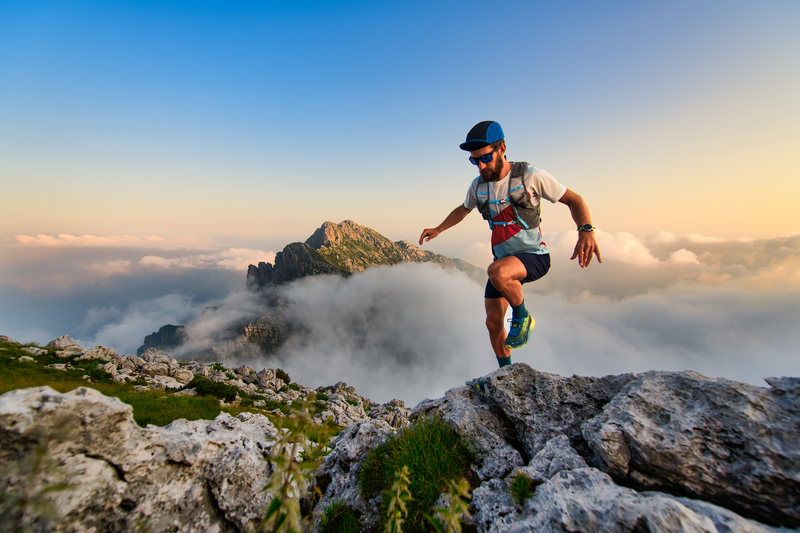
94% of researchers rate our articles as excellent or good
Learn more about the work of our research integrity team to safeguard the quality of each article we publish.
Find out more
ORIGINAL RESEARCH article
Front. Psychol. , 10 May 2011
Sec. Perception Science
volume 2 - 2011 | https://doi.org/10.3389/fpsyg.2011.00082
This article is part of the Research Topic Single-trial analyses of behavioural and neuroimaging data in perception and decision-making View all 11 articles
A commentary has been posted on this article:
Neural Correlates of the Time Course of the Waxing and Waning of Attention
Parieto-occipital electroencephalogram (EEG) alpha power and subjective reports of attentional state are both associated with visual attention and awareness, but little is currently known about the relationship between these two measures. Here, we bring together these two literatures to explore the relationship between alpha activity and participants’ introspective judgments of attentional state as each varied from trial-to-trial during performance of a visual detection task. We collected participants’ subjective ratings of perceptual decision confidence and attentional state on continuous scales on each trial of a rapid serial visual presentation detection task while recording EEG. We found that confidence and attentional state ratings were largely uncorrelated with each other, but both were strongly associated with task performance and post-stimulus decision-related EEG activity. Crucially, attentional state ratings were also negatively associated with prestimulus EEG alpha power. Attesting to the robustness of this association, we were able to classify attentional state ratings via prestimulus alpha power on a single-trial basis. Moreover, when we repeated these analyses after smoothing the time series of attentional state ratings and alpha power with increasingly large sliding windows, both the correlations and classification performance improved considerably, with the peaks occurring at a sliding window size of approximately 7 min worth of trials. Our results therefore suggest that slow fluctuations in attentional state in the order of minutes are reflected in spontaneous alpha power. Since these subjective attentional state ratings were associated with objective measures of both behavior and neural activity, we suggest that they provide a simple and effective estimate of task engagement that could prove useful in operational settings that require human operators to maintain a sustained focus of visual attention.
Attention waxes and wanes during the undertaking of a task, as our minds wander and subsequently refocus and as our levels of vigilance vary (e.g., Robertson et al., 1997; Gilden, 2001; Wagenmakers et al., 2004; Monto et al., 2008), particularly during relatively straightforward tasks for which processing becomes automated after initial orientation (Smallwood and Schooler, 2006). We refer to such fluctuations in task engagement as “attentional state.” Here, we assess two very different measures of attentional state: participants’ own introspective judgments, and spontaneous prestimulus electroencephalogram (EEG) alpha activity. On a trial-by-trial basis, we investigate the relationship between these disparate measures of attentional state, the timescales over which they fluctuate, and their interplay with performance in a rapid serial visual presentation (RSVP) detection task.
Alpha is EEG oscillatory activity between approximately 8 and 12 cycles per second that can occur over the entire scalp but is typically highest in amplitude in parieto-occipital areas. It increases in amplitude when the eyes are closed and is attenuated by visual stimulation (Berger, 1929); it has long been considered to reflect general arousal in that low alpha is associated with a state of alertness and high alpha is associated with relaxation or drowsiness (Pollen and Trachtenberg, 1972; Ray and Cole, 1985).
Over the last decade a wealth of research into alpha activity has shown that it is intimately linked to attention and it is now considered by many to reflect cortical excitability, with low alpha indicating active neuronal processing and high alpha denoting inhibition or disengagement of brain areas uninvolved in task performance (e.g., Klimesch et al., 1998; Worden et al., 2000; Pfurtscheller, 2001; Sauseng et al., 2005b; Kelly et al., 2006; Romei et al., 2008a,b; Snyder and Foxe, 2010; for a review see Klimesch et al., 2007). It has frequently been reported that when participants are spatially cued to anticipate a visual stimulus appearing on one side of space, alpha decreases contralaterally and increases ipsilaterally (Worden et al., 2000; Yamagishi et al., 2003; Sauseng et al., 2005b; Kelly et al., 2006, 2009; Thut et al., 2006; Rihs et al., 2007; Wyart and Tallon-Baudry, 2009). A similar finding has been reported for the allocation of attentional focus in the upper and lower visual fields (Worden et al., 2000; Rihs et al., 2007). Furthermore, some studies have reported this effect in tandem with an association of alpha power and task performance: Faster response latencies (Thut et al., 2006; Kelly et al., 2009) and increased accuracy of detection or discrimination (Kelly et al., 2009; Wyart and Tallon-Baudry, 2009) have been shown to coincide with lower alpha power contralaterally and higher alpha power ipsilaterally.
Recently, the causal nature of alpha oscillations in selective attention has been demonstrated: Romei et al. (2010) have shown that alpha frequency (10 Hz) repetitive transcranial magnetic stimulation (r-TMS) enhances visual detection when applied to ipsilateral visual cortex and impairs visual detection when applied to contralateral visual cortex. Doesburg et al. (2009) have reported that the phase-locking of alpha activity between low-level visual cortex and parietal cortex during the interval between an informative spatial cue and target stimulus onset increases contralaterally and decreases ipsilaterally. In a similar vein, Capotosto et al. (2009) have demonstrated that r-TMS to the right intra-parietal sulcus and right frontal eye fields disrupts attentional modulation of alpha power in visual cortex and impairs identification accuracy and response latency to a target stimulus presented approximately 2 s later. Collectively, these results implicate the parietal cortex in the control of attention by alpha suppression and enhancement in visual cortex.
There is a good deal of evidence, therefore, that relative alpha power within occipital cortex reflects the spatial focus of visual attention. In addition, the overall level of alpha power in visual cortex has been shown to be indicative of disengagement with external visual input and a focus on other senses or internal thoughts. For example, focusing attention on auditory (Foxe et al., 1998; Fu et al., 2001) or somatosensory input (Linkenkaer-Hansen et al., 2004) results in increased alpha power in parieto-occipital cortex. Alpha power in parieto-occipital cortex is also greater during internal cognitive tasks such as mental arithmetic (Ray and Cole, 1985; Palva et al., 2005) and imagery (Ray and Cole, 1985; Hari et al., 1997; Cooper et al., 2006, 2003), and during short-term and working memory retention (Jensen et al., 2002; Busch and Herrmann, 2003; Sauseng et al., 2005a). In a recent study, alpha power was found to be greater while participants were focused on an internal counting task (Braboszcz and Delorme, 2011). Conversely, when attention is redirected to visual input, alpha power is attenuated. For example, alpha power decreases in response to a warning cue that the appearance of a task-related stimulus is imminent (Klimesch et al., 1998), and is reduced following errors relative to correct trials in the Stroop task (Carp and Compton, 2009) and in digit discrimination tasks (Mazaheri et al., 2009). Since many models of cognitive control propose that detection of an error is a sign that task approach needs to be improved (Holroyd and Coles, 2002; Ridderinkhof et al., 2004; Yeung et al., 2004), this finding implies that after a lapse in attention, participants refocus on the task at hand.
These observations of elevated levels of alpha activity in visual cortex in association with disengagement from visual input have recently led researchers to investigate whether visual awareness is negatively correlated with spontaneous alpha power. However, whereas there have been many demonstrations of alpha power changes due to directed attention in a variety of paradigms, as discussed above, effects of alpha power on visual awareness are seemingly more difficult to obtain. Some authors have reported such an association in simple threshold contrast detection tasks with precisely controlled stimulus conditions, with greater prestimulus alpha preceding trials in which a target was not detected than trials in which a target was detected (Ergenoglu et al., 2004; van Dijk et al., 2008; Busch et al., 2009); during a visual stimulus duration monitoring task (O’Connell et al., 2009), alpha power was found to steadily increase starting up to 20 s before a task error occurred. Others, however, with similar paradigms have found no such association (e.g., Thut et al., 2006). Therefore it seems that whereas the link between alpha power in parieto-occipital cortex and directed attention is strong, subsequent effects on visual awareness are less consistently observed.
To summarize, prestimulus alpha power is a good candidate for use as an index of attentional state on a trial-by-trial basis since it has repeatedly been demonstrated to reflect the spatial locus of visual attention and the extent to which attention is focused on visual input. In this study, we sought to investigate the relationship between alpha power and participants’ subjective ratings of their attentional state.
There is a growing literature demonstrating that participants’ introspective judgments of attentional state are reliable and meaningful. Typically, such judgments are recorded via direct questioning at random intervals. The type of measure used has varied from a simple binary response in which participants indicate whether they were focused on the task or not immediately preceding the question (e.g., Smallwood et al., 2004, 2008; Mason et al., 2007; Christoff et al., 2009; Forster and Lavie, 2009; Kam et al., 2011), to asking participants to comment on what they were thinking about just prior to the question (for a review see Smallwood and Schooler, 2006). It has been proposed that during periods in which participants report that they are not focused on the task, attention has switched to thoughts unrelated to the task, i.e., mind-wandering (Smallwood and Schooler, 2006). This interpretation is supported by studies that asked participants to report what they were thinking about rather than simply report whether they were focused on the task or not (Teasdale et al., 1993, 1995).
During periods of reportedly low attentional state, sensory awareness of the external world may be reduced (Smallwood and Schooler, 2006). For example, in a task in which a response must be withheld upon presentation of a target digit, errors are more likely preceding reports of being unfocused on the task, assessed via probes occurring at random intervals (Smallwood et al., 2004, 2008; McVay and Kane, 2009). Furthermore, in EEG studies, early event-related potential (ERP) components related to perception (the P1 for visual stimuli and the N1 for auditory stimuli) have been shown to be attenuated in trials preceding reports of being unfocused on the task (Kam et al., 2011), as have later decision-related components, such as the P300, in response to targets (Smallwood et al., 2008). Episodes of low attentional state have also been associated with increases in neural activity in the default mode network as measured with fMRI (Mason et al., 2007; Christoff et al., 2009), and such neural changes in this network have in turn been associated with task errors (Eichele et al., 2008; Christoff et al., 2009).
One aspect of attentional state that has not yet been investigated is its temporal properties, i.e., how frequently do fluctuations in attentional state occur? Typically, attentional state probes are interspersed at random intervals of 30–90 s and all trials occurring during the 15 s prior to each mind probe are assigned with the subsequent response (e.g., Smallwood et al., 2008), as a compromise between the temporal resolution of reported attentional state and trial economy. Given that studies of this kind have found an association between subjective reports of attentional state and task performance (Smallwood et al., 2004, 2008; McVay and Kane, 2009), we might assume that fluctuations of attentional state occur with cycle durations in the order of at least tens of seconds. In support of this notion, a recent study (Monto et al., 2008) has demonstrated that somatosensory detection performance is correlated with the phase of infraslow fluctuations (0.1–0.01 Hz, i.e., 10–100 s cycles) of EEG. Furthermore, power in all frequency bands was similarly correlated, suggesting that the infraslow fluctuations reflect the excitability dynamics of cortical networks. Both of these findings could be interpreted as reflecting slow variations in attentional state.
Here, we attempt to bring together the research into EEG alpha activity and attention, and studies of subjective attentional state, by exploring the robustness of the relationship between spontaneous prestimulus EEG alpha activity and participants’ introspective ratings of attentional state on a continuous scale, with a focus on trial-by-trial variability. If these measures both relate to attentional state, they should be negatively correlated. However, it is an open question as to whether they would be associated in this way. On the one hand they operate on disparate levels: Parieto-occipital alpha power has been suggested to reflect the excitability of visual cortex (e.g., Klimesch et al., 2007), implying that it reflects relatively low-level physiological factors, whereas reporting on one’s own mind-state is clearly a high-level process. On the other hand, both have been shown to be associated with hallmark attentional effects, as reviewed above. We also anticipated that high attentional state ratings and low prestimulus alpha power would be associated with better detection performance – as the results of previous studies’ that we have reviewed above would suggest. To test these predictions, and to explore the timescales over which these measures co-varied during the course of the experiment, we correlated the time series of attentional state ratings, prestimulus alpha power, and task performance after smoothing them with increasingly large sliding windows. Finally, we considered that attentional state ratings might be positively associated with the amplitude of ERPs, for example, early perceptual components (e.g., the N1 and P1) in response to stimulus onset warning cues, steady-state visually evoked potentials (SSVEPs) generated by the RSVP stream, and post-stimulus P300 amplitude in response to detected targets.
We also asked participants to rate the confidence of their perceptual decision. We predicted that, like attentional state ratings, this measure would correlate positively with detection performance and perhaps P300 amplitude, but we did not expect it to be associated with prestimulus alpha power. We also anticipated that confidence ratings could potentially be somewhat positively correlated with attentional state ratings, because a high attentional state could improve perceptual representation of the target leading to higher decision confidence, and conversely, that decision confidence might retroactively bias judgments of attentional state.
This study is part of a wider project looking at the use of EEG to improve image triage efficiency in an operational context (e.g., Gerson et al., 2006; Mathan et al., 2006, 2008; Mathan, 2008; Parra et al., 2008; Poolman et al., 2008). As such, the task we employ here is intended to be an experimental analog of the work performed by intelligence analysts searching satellite imagery. Previous work on this project has shown that efficiency is improved if images are subdivided into smaller images and presented in an RSVP stream (Gerson et al., 2006; Mathan et al., 2006). Hence, our task was a simple detection task with complex stimuli: An RSVP stream of noise images lasting 1 s during which a target geometric pattern was sometimes presented, embedded in the noise of one of the images (see Figure 1 for a schematic illustration of the trial procedure). The stimuli we used were synthetic to allow precise control over stimulus visibility, but were designed to mimic satellite imagery used by other groups in the project. At the end of each trial participants reported whether they had seen the target or not, and rated both their confidence of this decision and their attentional state with respect to the task during that trial. To minimize the time taken to collect responses, and to provide participants with an intuitive response method, all three of these judgments were reported via a single click of a mouse within a large square (see Figure 1 for an illustration). The vertical axis of the square reflected their attentional state, and the horizontal axis reflected both their target present or absent response and their confidence of this decision: Clicks within the right half of the square indicated target present and clicks within the left half indicated target absent; distance from the vertical midline to either edge of the square indicated confidence. Participants’ EEG in 32 channels was recorded throughout the experiment.
Figure 1. Trial procedure and stimuli. (A) A schematic illustration of the sequence of events in each trial. (B) Example target image. (C) Example non-target image.
Twelve participants were recruited at the University of Oxford and were paid for their participation. One participant was excluded and replaced due to consistent reporting of target present responses as high attentional state and target absent responses as low attentional state with very little other variability. The age range of those included was 18–29 years (M = 22.33 years, SD = 4.36 years), and there were five males. All of the participants had normal or corrected-to-normal vision. The research was conducted in accordance with the American Psychological Association’s standards for ethical treatment of participants and with the approval of the University of Oxford’s institutional review board.
The experiments were created and run with the Psychophysics Toolbox version 3 (Brainard, 1997) in Matlab 2009b (The Mathworks, Inc., 2009) on a Windows PC attached to a 20′′ monitor at a resolution of 1024 × 768 and a refresh rate of 60 Hz. The participants’ task was to monitor an RSVP stream of images for the presence of a target image. The sequence of events on each trial is illustrated schematically in Figure 1.
In each trial a set of 10 images was presented serially over the course of 1 s (i.e., at 10 Hz). Each image was centered at fixation, subtended 18.5° by 18.5° of visual angle at a viewing distance of 57 cm, and was presented for 50 ms, followed by a blank gray screen for another 50 ms. The images were gray-scale white noise patterns that were randomly selected from a pre-generated set of 60 used for all participants. In target images, a set of six concentric circle patterns (each subtending 0.4° radius and consisting of two concentric circles) arranged in a randomly oriented hexagon of 3.3° radius, was embedded in the background noise. Targets were presented in 50% of trials, and their position in the RSVP stream was counterbalanced, although they were never presented in the first or last two positions. The contrast of the target pattern was determined for each participant during a brief pre-experimental session consisting of three blocks of 24 trials, in which a staircase procedure (QUEST from the Psychophysics Toolbox 3, Brainard, 1997) was used to titrate detection rate at 75%. This pre-experimental session also served as a practice session for the participants. Feedback was provided at the end of each trial during the first practice block only. The experimental session consisted of three blocks of 312 trials each.
The RSVP image stream was preceded by a red fixation point of 0.1° radius at the center of the gray screen for a period of 2 s that was interrupted after 1 s by the presentation of the words “Get Ready!” in black, centered at fixation, for 0.3 s. The letters of the words subtended 0.6° by 0.7°. After the offset of these words the screen remained blank, except for the red fixation point, for 700 ms before the RSVP stream began. It was followed by a response screen (see Figure 1) consisting of a white square (8.0° by 8.0°) with a black border subdivided into four quadrants by a faint gray line, with the response questions, “Did you see the target?”, “How confident are you of that?”, and “How focused were you?” in black at the top of the screen, the number of the current trial and block, as well as the number of trials per block and total number of blocks, in blue at the bottom of the screen. The words “Sure Present” appeared on the right hand side of the square, “Sure Absent” on the left, “More Focused” above, and “Less Focused” below, in black. Participants were instructed to click once with a mouse within the square to indicate their response to all three task questions. The vertical axis of the square reflected their attentional state, whereas the horizontal axis reflected both their target present or absent response and their confidence of this decision: Clicks within the right half of the square indicated target present and clicks within the left half indicated target absent; distance from the vertical midline to either edge of the square indicated confidence. The square was 201 by 201 pixels so attentional state ratings were measured on a 201-point scale and confidence was measured on a 101-point scale (since confidence increased from 0 up to 100 for target present responses and decreased from 0 to −100 for target absent responses). Participants were asked to rate their attentional state with respect to the current trial only in terms of the extent to which they were focused on performing the detection task. They were asked to incorporate their levels of vigilance and distraction (whether from internal sources, i.e., mind-wandering, or external sources, e.g., sounds outside the testing room) into this one response.
A Neuroscan Synamps2 system (10 GΩ input impedance; 29.8 nV resolution) was used to record EEG data from 32 Ag/AgCl electrodes mounted in an elastic cap at locations FP1, FPZ, FP2, F7, F3, FZ, F4, F8, FT7, FC3, FCZ, FC4, FT8, T7, C3, CZ, C4, T8, TP7, CP3, CPZ, CP4, TP8, P7, P3, PZ, P4, P8, POZ, O1, OZ, and O2. An additional six external electrodes were attached to the left and right mastoids, of which the left acted as a reference, the outer canthi of the left and right eyes, and above and below the right eye to measure electro-oculograms (EOGs). All electrode impedances were kept below 50 kΩ. EEG data were recorded at a sampling rate of 1000 Hz and were high-pass filtered online above 0.1 Hz. Data were downsampled off-line at 100 Hz, then low-pass filtered at 48 Hz, and subsequently epoched from 1.5 s before RSVP onset to 1 s after offset. For the alpha power analyses, the continuous data were additionally high-pass filtered at 0.5 Hz to reduce noise before the epochs were extracted. EEG epochs were baseline-corrected by subtracting the average of the data points between 1.1 and 1 s before RSVP onset. Eye blink correction was conducted using an independent components analysis approach via the EEGLab toolbox for Matlab (Delorme and Makeig, 2004). All EEG processing was conducted with custom-written scripts using native Matlab commands and commands from the EEGLab toolbox. Filtering was conducted with the “eegfilt” command from the EEGLab toolbox, which utilizes a two-way least-squares FIR filter. The order of the filter was equal to the sampling rate (100 Hz) divided by the lower edge of the band, rounded down and multiplied by three, with a minimum value of 15.
Our analysis was intended to uncover neural correlates of detection performance and continuous ratings of subjective attentional state and decision confidence. We therefore compared ERPs and prestimulus alpha power as a function of each type of rating, and correlated increasingly smoothed time series of attentional state ratings, alpha power, and task performance to investigate the timescales over which they co-varied. Finally, we used single-trial classification to appraise the robustness of the association between attentional state ratings and prestimulus alpha power. Details of these analyses are given in the following sections.
To quantify the P300 for each trial, we re-epoched the EEG data time-locked to target image onset, and baseline-corrected by subtracting the average of data points between 0.1 s before and after target image onset. We then low-pass filtered the EEG epochs below 8 Hz to remove the strong 10 Hz SSVEP signal generated by the RSVP stream, averaged them across central, parietal, and occipital midline electrodes CZ, CPZ, PZ, POZ, and OZ, and took the maximum voltage between 350 and 450 ms post-target image onset as the amplitude of the P300 for each trial.
To quantify prestimulus alpha power for each trial, we first band-pass filtered the EEG epochs from parieto-occipital channels P7, P3, PZ, P4, P8, POZ, O1, OZ, and O2, using a 4-Hz band centered on each participant’s modal alpha frequency (typically 10 Hz, Klimesch et al., 2007). We subsequently computed the envelope of the amplitude-modulated signal via the Hilbert transform (“hilbert” function in Matlab), which discards phase information and reveals oscillatory power fluctuations over time, and averaged this signal within the 1-s time period between the onset of the words “Get Ready!” and the onset of the RSVP stream. Hence, data from the first 0.5 s and last 2 s of the epochs were discarded, avoiding contamination from edge effects after filtering. We then divided by the average Fourier spectrum power for all frequencies except those in the alpha band, from the same time interval, to normalize alpha power to that of the rest of the EEG frequency spectrum. We performed this step because we were concerned that a simple measure of alpha power might be unduly affected by changes in broadband EEG power (i.e., recording noise) during the session, which might mask the activity of interest. By dividing alpha power by the average broadband power from the same time interval, we eliminate this source of extraneous variance in our alpha quantifications. Without this step the results were slightly less clear in some participants, but the overall pattern was not materially affected. Therefore while the normalization procedure does have an effect, it only served to reduce noise.
The aim of the timescales analyses was to investigate the pairwise associations between prestimulus alpha power, attentional state ratings and task performance (trial accuracy), at different timescales within the experimental session. Our novel approach was to hold the trial-by-trial time series of one variable constant and increasingly smooth the corresponding time series of the other variable, calculating Pearson’s Product Moment Correlation Coefficient after each increase in the sliding window size. Prior to smoothing, we high-pass filtered the time series of each variable at two cycles per experimental session and subsequently discarded the first and last 72 trials to avoid contamination from edge effects. The purpose of this pre-processing step was to remove the gradual downward drift that dominated the time series of attentional state ratings of 4 of the 12 participants, while having minimal effects for the other eight participants. Hence, the analysis focused on fluctuations within the experimental session. Our smoothing method was to average over trials within a sliding window. At the beginnings and ends of the time series, the number of available trials was used instead of the specified sliding window size. Since smoothing also resulted in edge effects, we discarded an additional 36 trials at the start and end of each time series, leaving 720 trials. We then repeated the smooth and correlate procedure, leaving the previously smoothed time series unsmoothed and smoothing the other time series.
Due to the novelty of our approach, we repeated the analysis on simulated time series data to verify that it could reveal correlated fluctuations in pairs of time series and would not generate spurious correlations. Thus, the simulated time series could be uncorrelated or could be correlated over fast timescales, slow timescales, or both fast and slow timescales. Of interest was the effectiveness with which the smoothing analysis, applied exactly as it was to our empirical EEG data, would identify the presence or absence of these correlations in the time series data. Specifically, we generated four sets of simulated time series: The first set contained no oscillations; the second consisted of sine waves of 1–9 cycles per experiment (i.e., slow oscillations); the third, sine waves of 46–90 cycles per experiment (i.e., fast oscillations); the fourth, sine waves of 1–9 and 46–90 cycles per experiment (i.e., both slow and fast oscillations). We subsequently inverted the values of each signal to form a complementary signal that was perfectly negatively correlated with the first. We then added independently generated white noise to each simulated time series, and finally performed our smoothed time series correlation analysis on 12 time series pairs (to match the number of participants).
We subsequently used a single-trial classification analysis to appraise the robustness of the association between attentional state ratings and prestimulus alpha power. Specifically, we classified upper vs. lower attentional state rating quartiles on the basis of prestimulus alpha power. We used a logistic regression classifier (Parra et al., 2002) that identifies the spatial distribution of scalp EEG activity in a given time window that maximally distinguishes two conditions to deliver a scalar estimate of component amplitude on each trial. The derived estimates are robust (i.e., have high signal-to-noise) because the discriminating components act as a spatial filter that estimates component amplitude as a spatially weighted average across electrodes for each trial, in much the same way that conventional ERP analysis averages across trials to reduce noise (Parra et al., 2002).
For the classification analyses, alpha amplitude was quantified as Fourier spectral power in a 4-Hz band centered on each participant’s modal alpha frequency (typically 10 Hz). We used the 1 s of EEG data between the onset of the words “Get Ready!” and the onset of the of the RSVP stream to compute a Fourier spectrum for each trial. Since we had downsampled the data to 100 Hz, an array of five values – one for each integer frequency within the 4-Hz band – was obtained per electrode, per trial. The classifier identified an optimal weighting of electrodes for each of these five frequencies as a predictor of upper vs. lower attentional state quartile. We averaged the classifier output for the five frequencies to obtain a scalar value for each trial from 0 to 1, which can be conceptualized as the estimated probability that attentional state rating was in the upper quartile on that trial. By comparing these values with the objective truth label of the trial (i.e., 0 = lower attentional state rating quartile and 1 = upper), we computed the Az score (the area under the receiver operating characteristic curve, Stanislaw and Todorov, 1999) for each participant.
We employed a 2-fold cross validation approach, such that we trained the classifier on half of the data and tested it on the other half, then repeated this procedure after switching training and validation data. We employed a random-fold assignment procedure, in which data samples are randomly assigned to training and validation sets, rather than a sequential-fold assignment procedure, in which data from each class are split into training and validation sets based on their temporal order. Whereas sequential folds provide a better indication of cross-session generalization, the advantage of random folds is that non-stationary aspects of the EEG signal are factored out and the invariant correlates of the conditions being classified become more prominent. Another advantage of a random-fold assignment procedure is that it introduces a source of variability into the computation, such that classifications can be repeated a number of times and Az score confidence intervals can be computed. We repeated all classifications 1000 times and give the mean and 95% confidence interval for individual participants’ Az scores.
We first present analyses of the continuous trial-by-trial ratings of attentional state and perceptual decision confidence, and their relation to task performance. We then relate these measures to key EEG indices of attention and task performance: ERPs and ongoing prestimulus alpha power as a function of quantile-split attentional state and confidence ratings. Having observed an overall relationship between these measures, our next analyses focus on the timescales of variation of attentional state ratings, prestimulus EEG alpha power, and task performance. Finally, to assess the robustness of EEG alpha power as a measure of attentional state, we investigate whether this measure can be used to predict participants’ subjective attentional state ratings as they vary from trial-to-trial using multivariate classification.
Target contrast corresponding to a detection rate of 75% was determined for each participant with a staircase procedure during a pre-experimental session. The mean contrast value was 0.15 (SD = 0.02). The mean detection rate across participants was 71% (SD = 8%) and the mean false alarm rate (target present responses when no target was presented) was 9% (SD = 5%). Mean d′ was 1.98 (SD = 0.49).
Figure 2A shows the locations within the response square of all clicks by all participants for target present trials (in black) and target absent trials (in red), and the grand average distributions of attentional state and confidence ratings for target present and absent trials separately (also in black and red). Since clicks within the right half of the square denoted target present responses and clicks within the left half denoted target absent responses, black dots on the right indicate trials in which a target was presented and detected (hits), whereas those on the left indicate trials in which a target was presented but not detected (misses). Correspondingly, red dots on the right indicate trials in which no target was presented but one was reported (false alarms), and those on the left indicate trials in which it was correctly reported that no target had been presented (correct rejections). An association between attentional state and confidence rating can be observed for both target present and absent responses: A dense diagonal cloud of dots is evident from the center to the top right corner for target present responses (black dots) and from the center to the top left corner for target absent responses (red dots), indicating that participants were more confident about their judgments of both target presence and target absence on trials in which they felt they had paid greater attention to the task.
Figure 2. Behavioral results from the visual detection task. (A) The locations of all responses (mouse clicks) from all participants. Responses in target trials are shown as black dots; responses in non-target trials are shown as red dots. The frequency distributions of attentional state ratings for target trials (black line) and non-target trials (red line) are shown on the left hand side, and the frequency distributions of decision confidence ratings are shown below. (B,C) Grand average percentages of hits and false alarms as a function of attentional state rating decile and confidence rating decile, respectively. Error bars show the SE of the mean across participants. (D) The normalized time series of attentional state ratings (red line) and detection task accuracy (black line) for one participant. (E) The normalized time series of attentional state ratings (red line) and detection task accuracy (black line) for one participant after smoothing each time series with a sliding window size of 101 trials.
The grand average distribution of confidence ratings (lower panel of Figure 2A) for target present trials (black line) shows that confidence ratings on hit trials were bimodally distributed with a peak at the maximum confidence rating (100), and another close to the minimum confidence rating (0). Confidence ratings on miss trials were more distributed, with no peak at the maximum (−100), but a small one at the minimum confidence rating (0). Hence, when confidence was low, participants tended to opt for target present rather than absent. In addition, these low confidence target present responses were very frequently correct since there is no corresponding peak in the distribution of confidence ratings for target absent trials (red line). Instead, this distribution is biased toward higher confidence ratings, so there seems to have been a tendency to rate correct rejections as high confidence. False alarms on the other hand were very rarely rated as high confidence and tend to cluster close to 0, suggesting that they were guesses.
The grand average distributions of attentional state ratings (Figure 2A, left panel) are negatively skewed for both target present and absent trials (black and red lines, respectively), indicating that participants tended to rate their attentional state as high rather than low, with modes of 150 (out of 200) for target present trials, and 118 (out of 200) for target absent trials. The mean attentional state rating for target present trials was 130.71 (SD = 20.46) and for target absent trials was 126.36 (SD = 19.95), a small but significant reduction, F(1, 11) = 17.01, MSE = 113.75, p = 0.002, This result reveals a slight but consistent bias in attentional state ratings, since whether a target was presented in a trial or not was randomly determined and could not be related to fluctuations in attentional state. We interpret this effect as a small retroactive bias of attentional state ratings in hit trials: Noticing a target may lead to increased alertness and hence a higher attentional state rating, or might lead participants to assume their attention must have been high given that they detected a target.
To investigate the relationship between attentional state ratings and task performance, we binned trials by attentional state rating and subsequently performed one-way ANOVAs on hit rate, false alarm rate and d′ (Stanislaw and Todorov, 1999) by bin. Higher attentional state ratings were associated with better performance in terms of hit rate, F(9, 99) = 8.65, MSE = 709.47, p < 0.001, and d′, F(9, 99) = 6.84, MSE = 1.19, p < 0.001,
but not false alarm rate, F < 1. Grand average hit rate and false alarm rate as a function of attentional state rating bin are shown in Figure 2B. A clear linear trend is evident with hit rate increasing as attentional state rating increases, F(1, 99) = 63.43, MSE = 5203.05, p < 0.001,
There was also a significant linear trend for d′, F(1, 99) = 53.42, MSE = 9.29, p < 0.001,
As noted above, we observed a retroactive bias to rate attentional state as higher in target present trials. To rule out the possibility that this bias produced an artifactual association between attentional state ratings and task performance, we repeated the analysis using the rating from the following trial, thus eliminating any bias arising from the presence or absence of a target on the current trial. The association persisted in hit rates, F(9, 99) = 2.21, MSE = 152.65, p = 0.027, as did the linear trend, F(1, 99) = 9.53, MSE = 657.92, p = 0.003,
Additionally, we repeated the analysis after excluding any participant for whom the distributions of attentional state ratings for target present and absent trials did not overlap (N = 7). Again, the association remained for hit rates, F(9, 36) = 4.74, MSE = 471.80, p < 0.001,
as did the linear trend, F(1, 36) = 31.52, MSE = 3138.03, p < 0.001,
Effect sizes for both the ANOVA and the test of trend increased rather than decreased after removing participants who exhibited a retroactive bias, so if anything the association between attentional state ratings and task performance was weakened by these participants rather than enhanced.
These analyses demonstrate that subjective attentional state ratings were meaningful: Detection performance was superior during periods of higher reported attentional state.
To investigate the association between confidence ratings and task performance, we performed a corresponding analysis to that above: We binned trials on the basis of confidence rating decile, then performed one-way ANOVAs on hit rate, false alarm rate and d′ by bin. Note that our confidence scale increases from 0 up to 100 for target present responses and decreases from 0 to −100 for target absent responses, hence we took the absolute value of confidence ratings for this analysis. Grand average hit rate and false alarm rate as a function of confidence rating bin are shown in Figure 2C. Higher confidence ratings were associated with better performance in terms of false alarm rate, F(9, 99) = 34.99, MSE = 3409.16, p < 0.001, and d′, F(9, 99) = 14.76, MSE = 4.69, p < 0.001,
but not hit rate, F(9, 99) = 1.76, MSE = 532.91, p = 0.086. A clear linear trend is evident with false alarm rate decreasing as confidence rating increases and a test of linear trend revealed this to be significant, F(1, 99) = 189.19, MSE = 18,435.18, p < 0.001,
There was also a significant linear trend for d′, F(1, 99) = 104.70, MSE = 33.24, p < 0.001,
Hence, higher confidence ratings were associated with better detection performance, as expected; however, it is surprising that the effect was not found for hit rate. This finding seems to be related to the bimodality seen in the distribution of hit trial confidence ratings, in that a large proportion of hits were rated as very low confidence. It could reflect a strong tendency to guess target present rather than absent, or alternatively indicates implicit target detection.
One of the main aims of this study was to look at fluctuations of attentional state over the course of the experiment. As an example, Figure 2D presents the normalized time series of attentional state ratings and detection task accuracy for one participant, and Figure 2E presents the same time series after smoothing by averaging with a sliding window size of 101 trials. There is a positive correlation between attentional state rating and detection accuracy for this participant. We will return to this issue later with a timescale analysis for all participants on attentional state rating, prestimulus alpha power, and detection performance.
Figure 3A presents grand averaged ERPs at central and posterior midline scalp electrodes as a function of attentional state rating quartile. Figure 3B presents grand averaged ERPs as a function of confidence rating quartile for hit trials only, with miss trials represented by an additional line. The waveforms are time-locked to the onset of the words “Get Ready!” that signified that the RSVP stream would begin in 1 s.
Figure 3. Event-related potentials during preparation and stimulus presentation. (A,B) Grand average ERPs time-locked to the onset of the words “Get Ready!” by attentional state rating quartile and confidence rating quartile, respectively. (C,D) Grand average ERPs time-locked to target onset by attentional state rating quartile and confidence rating quartile, respectively. In (B–D), miss trials are shown separately as a blue line. For (C,D) EEG epochs were low-pass filtered at 8 Hz. Scalp plot in (A) shows the locations of the electrodes included in the analyses in (A–D).
A clear P1–N1 complex followed by an N400 is visible in response to the presentation of the words “Get Ready!”, and a SSVEP is generated by the RSVP stream (onset at 1 s). We might have expected these responses to be of greater amplitude in higher rated attentional state trials; however, this was not the case – ERP and SSVEP amplitudes varied little, if at all, as a function of attentional state rating. The P300 in response to targets is evident in the latter half of the SSVEP for the two upper quartiles of attentional state and confidence ratings. For a clearer view, Figure 3 presents grand averaged ERPs time-locked to target onset, for hit trials only as a function of attentional state rating quartile (Figure 3C), and confidence rating quartile (Figure 3D), with miss trials presented in a separate line. P300 amplitude in hit trials was significantly larger in trials with higher attentional state ratings, F(3, 33) = 7.82, MSE = 24.02, p < 0.001, and in trials with higher confidence ratings, F(3, 33) = 25.95, MSE = 43.64, p < 0.001,
Both effects followed a linear trend: For attentional state ratings, F(1, 33) = 20.57, MSE = 63.20, p < 0.001,
and for confidence ratings, F(1, 33) = 75.83, MSE = 127.53, p < 0.001,
These effects were not driven by differential hit rates because only hit trials were included in the analyses.
To assess changes in alpha power as a function of attentional state and confidence rating, we band-pass filtered EEG epochs at alpha frequencies (see Materials and Methods for details), and subsequently computed the envelope of the amplitude-modulated signal, thereby discarding phase information and revealing fluctuations in oscillatory power over time. Figure 4 shows the grand average amplitude-modulated signal as a function of attentional state rating quartile (Figure 4A) and confidence rating quartile (Figure 4B), time-locked to the onset of the words “Get Ready!”. Alpha power can be seen to steadily increase after the initial response to the words, and peaks at the start of the SSVEP in response to the RSVP stream. Subsequently, alpha power declines sharply up to 0.4 s after RSVP offset.
Figure 4. Alpha power during preparation and stimulus presentation. (A,B) Grand average amplitude-modulated EEG signal after band-pass filtering at alpha frequencies, time-locked to the onset of the words “Get Ready!”, by attentional state rating quartile and confidence rating quartile, respectively. In (B), miss trials are shown separately as a blue line. (C,D) Grand average normalized prestimulus alpha power as a function of attentional state rating decile and confidence rating decile, respectively. Error bars show the SE of the mean across participants. Scalp plot in (A) shows the locations of the electrodes included in the analyses in (A–D).
Inspection of Figure 4A reveals that alpha power was lower throughout the epoch in trials rated as high attentional state, as predicted. There was no association of alpha power and confidence rating, except during the second half of the RSVP stream and beyond, perhaps as a result of greater P300 amplitudes in trials with decisions rated as higher confidence. The relationships between prestimulus alpha power and attentional state and confidence ratings can be clearly seen in Figures 4C,D, respectively, in which grand average normalized prestimulus alpha power is plotted as a function of attentional state and confidence rating decile. Higher prestimulus alpha power was associated with lower attentional state ratings, F(9, 99) = 4.27, MSE = 0.000549, p < 0.001, with a significant linear trend, F(1, 99) = 32.57, MSE = 0.00419, p < 0.001,
but was not reliably associated with confidence rating, F(9, 99) = 1.25, MSE = 0.000103, p = 0.273.
This basic analysis illustrates clearly the relationship between attentional state ratings and prestimulus alpha power that we anticipated: Trials in which attentional state was rated as high featured lower prestimulus alpha power. Combined with the finding that attentional state rating was associated with detection performance, this result suggests that both measures reflect attentional state.
The above analyses reveal a strong relationship between attentional state rating and prestimulus alpha power. To assess whether the strength of this association varies across different timescales, we performed a series of correlations between individual participants’ time series of attentional state ratings and normalized prestimulus alpha power, after smoothing the time series of attentional state ratings with increasingly large sliding windows. We then repeated this procedure, leaving the time series of attentional state ratings unsmoothed and instead smoothing the time series of prestimulus alpha power. This analysis is illustrated in a flow chart in Figure 5A. For comparison, we performed a corresponding analysis of the relationship between prestimulus alpha power and task performance (detection task accuracy). The grand average power spectra of the time series of attentional state ratings, prestimulus alpha power, and task accuracy are shown in Figures 5B–D, respectively, for reference.
Figure 5. Correlations between attentional state ratings, prestimulus EEG alpha power, and task performance (detection accuracy). (A) Flow chart illustrating the smoothing analysis. (B–D) Grand average Fourier power spectra of the trial-by-trial time series of attentional state ratings, prestimulus EEG alpha power, and detection task accuracy, respectively. The SE of the mean is shown in gray. (E,F) Grand average correlations (r) as a function of the degree of smoothing (sliding window size). The correlations between the time series of attentional state ratings and prestimulus EEG alpha power are shown in black. The correlations between the time series of detection task accuracy and prestimulus EEG alpha power are shown in blue. Significant correlations are highlighted in red. Thin lines show the SE of the mean. In (E), smoothing is applied to the time series of attentional state ratings and detection task accuracy; in (F), smoothing is applied to the time series of prestimulus EEG alpha power.
Figures 5E,F present the resulting grand average correlations between alpha power and attentional state ratings (black lines), and between alpha power and detection task accuracy (blue lines). The time series of attentional state ratings and alpha power were negatively correlated, replicating the basic relationship described above. Crucially, this association increased in strength when we smoothed either the time series of attentional state ratings (Figure 5E) or alpha power (Figure 5F), with the peak correlation occurring at a sliding window size of 75 trials (approximately 7 min) in both analyses. The correlations between alpha power and task performance were much weaker.
We tested the correlations for significance with a series of two-tailed paired-samples t-tests between individual participants’ r values and zero, one for each sliding window size, controlling for multiple comparisons via the Benjamini and Hochberg (1995) procedure, which optimally balances statistical power and the control of false discovery rate for ERP analyses (Lage-Castellanos et al., 2010). Significant correlations are shown in Figure 5 in red. Whereas none of the correlations between task performance and alpha power were significant, the correlations between attentional state rating and alpha power were significantly different from zero between sliding window sizes of 3 and 133 trials, inclusive, in both analyses.
At first glance, the results of this analysis indicate that attentional state ratings and alpha power co-vary maximally over periods of minutes. However, increasing smoothing window size may be confounded with increasing signal-to-noise ratio (because trial-varying noise should increasingly average to zero with increasing smoothing). To investigate the extent of this issue, and to illustrate further the properties of our novel smoothing analysis, we repeated the analysis on four sets of simulated data. We simulated time series of attentional state ratings and alpha power that were negatively correlated at short timescales, long timescales, both short and long timescales, or at neither timescale. The results, shown in Figure 6, indicate that the analysis was effective in identifying whichever correlations were present in the simulated time series, with peaks apparent at corresponding smoothing window sizes, and did not create artifactual correlations where none were present. These simple simulations demonstrate that our smoothed time series correlation analysis was effective in revealing the timescales of covariation between attentional state ratings and prestimulus alpha power. This point is further supported by the fact that there were no significant correlations in the alpha power and task performance analysis. It should be noted, however, that it is still theoretically possible that the associations at short timescales were stronger than our analysis suggests, if there had been increased noise at these timescales.
Figure 6. Smoothed time series correlation analysis performed on simulated time series. (A) Uncorrelated time series. (B–D) Time series negatively correlated at long timescales, short timescales, and both long and short timescales, respectively. Thin lines show the SE of the mean across the 12 pairs of simulated time series.
The peak negative correlation between the time series of attentional state ratings and alpha power occurred at a sliding window size of 75 trials (approximately 7 min). Figure 7 plots the time series of attentional state ratings and alpha power both smoothed with a sliding window size of 75 trials for each participant individually, together with the correlation coefficient (r) between these time series. Strong negative correlations are evident for all participants except one, with fluctuations in attentional state ratings (red lines) varying in the opposite direction to fluctuations in alpha power (black lines).
Figure 7. Individual participants’ smoothed time series of attentional state ratings and prestimulus EEG alpha power. Normalized time series smoothed with a sliding window size of 75 trials are shown for each participant individually (P1–P12). The correlation coefficient (r) between the two time series for each participant is shown at the top of each plot. Significant correlations are indicated by a star. Triple starred correlations are significant at p < 0.001.
Overall, this analysis of the timescales of covariation between attentional state ratings and prestimulus alpha power reveals that the association between the two measures was even stronger at longer timescales: The negative correlation between the two measures peaked when we smoothed either of the time series with a sliding window size of approximately 7 min worth of trials.
To explore the reliability of the association between attentional state ratings and prestimulus EEG alpha power on single trials, we conducted single-trial logistic regression classification of upper vs. lower subjective attentional state rating quartile on the basis of prestimulus EEG alpha power for each participant individually. Fourier spectral power values within a 4-Hz band centered on each participant’s modal alpha frequency (typically 10 Hz), across 32 electrodes, were used as the input features with which the classifier estimated attentional state rating quartile.
To explore classification performance at longer timescales, we smoothed the time series of attentional state ratings with increasingly large sliding windows, and repeated our classification procedure after each increase in sliding window size. Figure 8A presents grand average classification performance (Az score) as a function of the sliding window size. The performance of the classifier improved dramatically as we increased the extent of smoothing. The point of inflection on the curve occurs at a sliding window size of approximately 80 trials, indicating where further smoothing ceases to improve classification performance.
Figure 8. Single-trial classification of attentional state rating on the basis of prestimulus EEG alpha power. (A) Grand average (thick line) and SE (thin lines) Az score as a function of the degree of smoothing (sliding window size) to the time series of attentional state ratings. (B) Average Az scores and 95% confidence intervals over 1000 repetitions for each participant, for attentional state ratings unsmoothed (in black), smoothed with a sliding window size of 75 trials (in red), and with shuffled attentional state rating trial labels (in gray).
Figure 8B presents the average Az score and 95% confidence interval over 1000 repetitions with unsmoothed attentional state ratings (in black) and with attentional state ratings smoothed with a sliding window size of 75 trials (in red), for each participant, ordered from the left by descending classification performance with smoothed data. As a bootstrap test of significance, we repeated the classification procedure a further 1000 times for each participant, shuffling attentional state rating trial labels each time. The resulting average Az score and 95% confidence intervals are shown in Figure 8B (in gray). Eight of the 12 participants’ average Az scores for unsmoothed attentional state ratings were greater than their bootstrap significance values (the upper end of the 95% confidence interval for Az scores from classification with shuffled attentional state rating trial labels), meaning that classification performance without smoothing was significantly better than chance for those participants. For the smoothed attentional state ratings, all 12 participants’ average Az scores were greater than their bootstrap significance values, and nine of them had average Az scores greater than the upper end of the 95% confidence interval for Az scores from classification with unsmoothed attentional state ratings. The three participants whose classification performance did not improve with smoothing, however, may have already been at ceiling – their average Az scores with unsmoothed attentional state ratings were already above 0.85. Smoothing attentional state ratings therefore improved classification performance significantly for all participants with average Az scores below 0.85.
In summary, trial-by-trial variations in prestimulus EEG alpha power reflected subjective attentional state for most participants, and smoothing attentional state ratings with a sliding window size of approximately 7 min improved classification performance considerably.
In this study, participants’ subjective ratings of attentional state and perceptual decision confidence were collected on continuous scales at the end of each trial of an RSVP target detection task while recording EEG. We found that attentional state and confidence ratings were both strongly positively associated with task performance and P300 amplitude, and that attentional state ratings were also associated negatively with spontaneous prestimulus EEG alpha activity. Furthermore, we could reliably classify single trials as upper or lower quartile reported attentional state on the basis of prestimulus EEG alpha power for most participants. We also investigated at what timescale these associations were strongest, and found that smoothing with a sliding window size of around 7 min worth of trials yielded the maximum correlation values. In addition, classification performance improved when we smoothed attentional state ratings to the same extent beforehand. Our results suggest that participants’ subjective ratings of their attentional state are informative in behavioral terms and reflect the excitability of visual cortex.
Higher attentional state ratings were associated with better task performance. This association persisted – in fact, became stronger – when participants with a bias for reporting attentional state as higher on target present trials were excluded from the analysis, and persisted when we repeated the analysis using the attentional state rating from the next trial. This finding supports the proposal that perceptual awareness of external events is attenuated during periods of low attentional state (Smallwood and Schooler, 2006).
Previous studies have demonstrated that perceptual task errors are more likely preceding reports of being unfocused on the task, in paradigms in which subjective attentional state was assessed on a discrete scale via probes occurring at random intervals (e.g., Smallwood et al., 2004, 2008; McVay and Kane, 2009). We queried participants’ attentional state on a continuous scale and after every trial, and yet ratings were strongly associated with performance, hence our study demonstrates that attentional state can be indexed efficiently on a continuous scale and in individual trials. These aspects of our design are advantageous because they capture more of the variability in attentional state, both of the construct itself and of its temporal characteristics.
One disadvantage of our design, however, is that in the interests of speed, we did not ask participants to report what they were thinking about when they rated their attentional state, as some previous studies have done (Teasdale et al., 1993, 1995). We therefore do not know whether reports of low attentional state were accompanied by task-unrelated thoughts (i.e., mind-wandering) or would be better characterized as periods of low vigilance. This issue could be addressed in a future study.
Studies of subjective attentional state have typically employed tasks that are optimized to find an association between task errors and reports of low attentional state, such as response inhibition tasks, in which a response that is required for most trials must be withheld on infrequently occurring target trials (Smallwood et al., 2004, 2008; McVay and Kane, 2009). Since our task required a response to both targets and non-targets, and targets were presented frequently (in 50% of trials), we extend previous demonstrations that subjective judgments of attentional state are associated with task performance (Smallwood et al., 2004, 2008; McVay and Kane, 2009) to a standard type of perceptual decision task. Hence, our results lend wider support to the proposition that sensory awareness of the external world is reduced during periods of low attentional state (Smallwood and Schooler, 2006).
From the proposal that sensory perception is attenuated during periods of low attentional state (Smallwood and Schooler, 2006), we might have expected to find that EEG responses related to the perception of visual events in our task – such as the ERP in response to the words “Get Ready!”, or the SSVEP generated by the RSVP stream – would be reduced in trials in which attentional state had been rated as low. Previous studies have produced discrepant results in this regard. Kam et al. (2011) reported a smaller P1 in response to visual stimuli in trials preceding reports of low task focus, whereas Smallwood et al. (2008) found no such effects in a similar paradigm, and O’Connell et al. (2009) also reported no such effects preceding stimulus duration judgment errors in a study showing steady increases in parieto-occipital alpha power in the 20 s leading up to errors. Kam et al. (2011) suggest that these two studies, both of which featured visual task stimuli presented foveally, failed to find variations in visually evoked EEG responses because the effect is limited to peripherally presented stimuli and does not occur, or is far weaker, for foveally presented stimuli, as has been suggested to be generally true of attentional effects on foveated stimuli (e.g., Handy and Khoe, 2005). Our RSVP stream images, although larger in retinal representation than the fovea, were centered at fixation, and target patterns were not much larger than foveal area, which might explain our lack of such an effect.
P300 amplitude in hit trials was strongly associated with both perceptual decision confidence rating and attentional state rating. Binary subjective judgments of attentional state recorded at random intervals have previously been shown to be associated with P300 amplitude (Smallwood et al., 2008), as has the allocation of spatial attention (e.g., Duncan-Johnson and Donchin, 1977; Johnson, 1993), and stimulus intensity (Roth et al., 1984; Covington and Polich, 1996). The effects of attentional state and spatial attention might therefore operate via a common mechanism: The enhancement of perceptual representations of task stimuli.
P300 amplitude was also predicted by confidence rating. If we assume that confidence ratings are primarily determined by target visibility (in our task images the background noise generated variance in target visibility), then this result could reflect the influence of stimulus intensity on P300 amplitude as has been reported previously (Roth et al., 1984; Covington and Polich, 1996). On the other hand, since neural representations are themselves noisy, it could alternatively suggest that P300 amplitude reflects the perceptual evidence available for a decision, with variability in attention and confidence reflecting the variability in perceptual representation of the target.
In combination with the fact that we found no differences in perceptual EEG responses as a function of attentional state rating, these strong associations of perceptual confidence and attentional state ratings with P300 amplitude suggest that the effects of attentional state occur at the decision level rather than the perceptual level, and hence further along the neural processing stream.
We propose that participants’ subjective attentional state ratings reflect both vigilance and the balance of attentional focus between external task-relevant information (in our case visual information) and internal task-unrelated thoughts (i.e., mind-wandering). This assertion is supported firstly in behavioral terms by the finding that attentional state ratings were positively associated with task performance, and secondly in neurophysiological terms by the finding that attentional state ratings were negatively associated on a trial-by-trial basis with spontaneous prestimulus parieto-occipital EEG alpha power. It has been widely reported that alpha power in these regions of cortex tracks the spatial focus of visual attention (e.g., Worden et al., 2000; Sauseng et al., 2005b), and there is some evidence that overall parieto-occipital alpha power is indicative of disengagement with external visual input, since it increases when attention is focused on other modalities (Foxe et al., 1998; Fu et al., 2001; Linkenkaer-Hansen et al., 2004), or during internal cognitive tasks (e.g., Ray and Cole, 1985; Cooper et al., 2003, 2006; Sauseng et al., 2005a), and decreases when attention is redirected toward visual input (Klimesch et al., 1998) or is refocused following visual task errors (Carp and Compton, 2009; Mazaheri et al., 2009). We have now demonstrated that parieto-occipital alpha power reflects attentional state. Importantly, our results imply that alpha power changes related to attentional state are accessible to consciousness.
We found that the association between attentional state ratings and prestimulus EEG alpha power increased in strength when we smoothed either or both of them in time, with the peak negative correlation occurring at a sliding window size of approximately 7 min worth of trials. In addition, the performance of our classifier reached an asymptote when attentional state ratings were smoothed to the same extent. Although participants did report fluctuations in attentional state at shorter timescales than this – indeed, with significant variability from trial to trial – these faster fluctuations were not as strongly associated with prestimulus alpha power in our analysis. This finding does not definitively indicate that fluctuations at shorter timescales were more weakly associated, however, because these faster fluctuations could have been subject to increased noise, thereby curtailing the ability of our analysis to reveal them. Nevertheless, smoothing did more than merely boost the signal-to-noise characteristics of the data: The correlation between attentional state ratings and alpha power did not continue to improve with larger sliding windows, in fact, it returned to baseline well before the maximum sliding window size was reached. Furthermore, we did not see any significant correlations in the timescale analysis of alpha power and task performance.
Previous EEG alpha and attention studies, as well as investigations of subjective attentional state, have not systematically analyzed the timescales of variability, but previous research has shown that behavioral performance (e.g., Verplanck et al., 1952; Gilden et al., 1995, see Gilden, 2001, for a review) and neural activity (e.g., Linkenkaer-Hansen et al., 2001; Leopold et al., 2003; Nikulin and Brismar, 2005) both exhibit 1/f frequency spectra. This type of distribution implies that the largest fluctuations in behavioral and neural responses over extended periods are at the longer timescales, i.e., minutes rather than seconds. Hence, our finding that attentional state ratings and prestimulus alpha power co-vary strongly when smoothed with a sliding window size of several minutes’ worth of trials is in agreement with this literature. In addition, many studies investigating periodicities in reaction times and error rates in detection tasks have reported cycle times in the range of 2–10 min (e.g., Wertheimer, 1953; Elliott, 1960; Makeig and Inlow, 1993; Conte et al., 1995; Smith et al., 2003; Arruda et al., 2009; Aue et al., 2009). In particular, Monto et al. (2008) have demonstrated that somatosensory detection performance and power in all frequency bands are correlated with the phase of infraslow fluctuations (0.1–0.01 Hz, i.e., 10–100 s cycles) of EEG, a finding that could be interpreted as reflecting slow variations in attentional state. Collectively, these findings suggest that changes in attentional state over time are not solely characterized by simple monotonic declines, since fluctuations occur over periods of minutes. Our study provides a link between this literature and research into subjective attentional state, spontaneous EEG alpha, and attention. Our findings are also informative with respect to online monitoring of user attention in contexts in which the users’ attentional state could be a critical determinant of task performance. In particular, our results suggest that measures of attentional state might be optimally assessed with a frequency in the region of minutes, and that periods of low attentional state can be relatively long lasting.
Previous studies featuring simple visual detection tasks have demonstrated that prestimulus alpha power is greater preceding failures to detect the target than successes (Ergenoglu et al., 2004; van Dijk et al., 2008; Busch et al., 2009). We found no such association in our data, which is surprising given the pairwise associations we found between prestimulus alpha power and attentional state ratings, and between attentional state ratings and detection accuracy. However, previous studies featured paradigms that were optimized for detecting associations between alpha power and visual awareness by precisely controlling stimulus conditions, such that target stimuli were identical from trial-to-trial. In contrast, our design was motivated by the desire to enable the extrapolation of our results to real-world tasks, for example, the detection of landmarks in satellite photographs (e.g., Poolman et al., 2008). In particular, our target stimuli were intended to vary in visibility considerably due to being embedded in white noise. Hence, an effect of prestimulus EEG alpha power on detection accuracy may have simply been lost in the inherent noise of EEG recordings coupled with the noise in the visibility of our target stimuli. Indeed, several other previous studies featuring much more controlled stimuli, akin to those aforementioned, have also failed to find an association between prestimulus alpha power and detection accuracy (e.g., Thut et al., 2006).
One other feature of our paradigm is potentially relevant in this context: Targets were never presented in the first two images of the RSVP stream, so there was always a delay of at least 200 ms between the start of the RSVP stream and target presentation. It is possible that this delay gave participants time to re-engage with the task following prestimulus periods of high alpha power, thereby attenuating any effect of alpha power on task performance. However, because attentional state ratings were associated with detection accuracy as well as prestimulus alpha power, this explanation implies that detection accuracy and prestimulus alpha power are associated with distinct portions of trial-to-trial variance in attentional state ratings. This idea could be addressed in a future study using a visual detection paradigm optimized to find an association between prestimulus alpha power and detection accuracy (e.g., Busch et al., 2009), in which attentional state ratings are collected on every trial, as we did. Such a study might find that attentional state ratings correlate with detection performance at shorter timescales than they do with alpha power, supporting the notion that attentional state is multi-faceted, featuring dissociable relationships with behavioral and neural measures.
We have demonstrated a strong association between subjective ratings of attentional state and prestimulus EEG alpha power. This association implies that the level of alpha activity in parieto-occipital cortex not only reflects cortical excitability (e.g., Klimesch et al., 2007), but is also indicative of attentional state with respect to a visual task. Furthermore, it suggests that fluctuations of alpha activity are accessible to consciousness. We propose that participants’ subjective attentional state ratings reflect both vigilance and the balance of attentional focus between external task-relevant information and internal task-unrelated thoughts (i.e., mind-wandering). Because these ratings were associated with objective measures of both behavior and neural activity, we suggest that they are a simple and effective means of estimating task engagement. As such, they could provide valuable information in operational settings in which monitoring users’ attentional state might permit the optimization of task parameters such as stimulus presentation rate or the weight assigned to their decisions as their attentional state waxes and wanes.
The authors declare that the research was conducted in the absence of any commercial or financial relationships that could be construed as a potential conflict of interest.
We thank Misha Pavel at Oregon Health and Science University for the images used in the experiment, Patricia Ververs at Honeywell Laboratories for useful discussions and feedback, and Sam Rogers at the University of Oxford for help with data collection. This work was supported by the Defense Advanced Research Projects Agency (DARPA) under contract N10PC20048. The views, opinions, and/or findings contained in this article/presentation are those of the authors and should not be interpreted as representing the official views or policies, either expressed or implied, of DARPA or the United States Department of Defense.
Arruda, J. E., Zhang, H., Amoss, R. T., Coburn, K. L., and Aue, W. R. (2009). Rhythmic oscillations in quantitative EEG measured during a continuous performance task. Appl. Psychophysiol. Biofeedback 34, 7–16.
Aue, W. R., Arruda, J. E., Kass, S. J., and Stanny, C. J. (2009). Cyclic variations in sustained human performance. Brain Cogn. 71, 336–344.
Benjamini, Y., and Hochberg, Y. (1995). Controlling the false discovery rate: a practical and powerful approach to multiple testing. J. R. Stat. Soc. Series B Stat. Methodol. 57, 289–300.
Berger, H. (1929). Uber das elektrenkephalogramm des menschen. Arch. Psychiatr. Nervenkr. 87, 527–570.
Braboszcz, C., and Delorme, A. (2011). Lost in thoughts: neural markers of low alertness during mind wandering. Neuroimage 54, 3040–3047.
Busch, N. A., Dubois, J., and VanRullen, R. (2009). The phase of ongoing EEG oscillations predicts visual perception. J. Neurosci. 29, 7869–7876.
Busch, N. A., and Herrmann, C. S. (2003). Object-load and feature-load modulate EEG in a short-term memory task. Neuroreport 14, 1721–1724.
Capotosto, P., Babiloni, C., Romani, G. L., and Corbetta, M. (2009). Frontoparietal cortex controls spatial attention through modulation of anticipatory alpha rhythms. J. Neurosci. 29, 5863–5872.
Carp, J., and Compton, R. J. (2009). Alpha power is influenced by performance errors. Psychophysiology 46, 336–343.
Christoff, K., Gordon, A. M., Smallwood, J., Smith, R., and Schooler, J. W. (2009). Experience sampling during fMRI reveals default network and executive system contributions to mind wandering. Proc. Natl. Acad. Sci. U.S.A. 106, 8719–8724.
Conte, S., Ferlazzo, F., and Renzi, P. (1995). Ultradian rhythms of reaction times in performance in vigilance tasks. Biol. Psychol. 39, 159–172.
Cooper, N. R., Burgess, A. P., Croft, R. J., and Gruzelier, J. H. (2006). Investigating evoked and induced electroencephalogram activity in task-related alpha power increases during an internally directed attention task. Neuroreport 17, 205–208.
Cooper, N. R., Croft, R. J., Dominey, S. J. J., Burgess, A. P., and Gruzelier, J. H. (2003). Paradox lost? Exploring the role of alpha oscillations during externally vs. internally directed attention and the implications for idling and inhibition hypotheses. Int. J. Psychophysiol. 47, 65–74.
Covington, J. W., and Polich, J. (1996). P300, stimulus intensity, and modality. Electroencephalogr. Clin. Neurophysiol. 100, 579–584.
Delorme, A., and Makeig, S. (2004). EEGLAB: an open source toolbox for analysis of single-trial EEG dynamics including independent component analysis. J. Neurosci. Methods 134, 9–21.
Doesburg, S. M., Green, J. J., McDonald, J. J., and Ward, L. M. (2009). From local inhibition to long-range integration: a functional dissociation of alpha-band synchronization across cortical scales in visuospatial attention. Brain Res. 1303, 97–110.
Duncan-Johnson, C. C., and Donchin, E. (1977). On quantifying surprise: the variation of event-related potentials with subjective probability. Psychophysiology 14, 456–467.
Eichele, T., Debener, S., Calhoun, V. D., Specht, K., Engel, A. K., Hugdahl, K., von Cramon, D. Y., and Ullsperger, M. (2008). Prediction of human errors by maladaptive changes in event-related brain networks. Proc. Natl. Acad. Sci. U.S.A. 105, 6173–6178.
Ergenoglu, T., Demiralp, T., Bayraktaroglu, Z., Ergen, M., Beydagi, H., and Uresin, Y. (2004). Alpha rhythm of the EEG modulates visual detection performance in humans. Brain Res. Cogn. Brain Res. 20, 376–383.
Forster, S., and Lavie, N. (2009). Harnessing the wandering mind: the role of perceptual load. Cognition 111, 345–355.
Foxe, J. J., Simpson, G. V., and Ahlfors, S. P. (1998). Parieto-occipital approximately 10 Hz activity reflects anticipatory state of visual attention mechanisms. Neuroreport 9, 3929–3933.
Fu, K. M., Foxe, J. J., Murray, M. M., Higgins, B. A., Javitt, D. C., and Schroeder, C. E. (2001). Attention-dependent suppression of distracter visual input can be cross-modally cued as indexed by anticipatory parieto-occipital alpha-band oscillations. Brain Res. Cogn. Brain Res. 12, 145–152.
Gerson, A. D., Parra, L. C., and Sajda, P. (2006). Cortically coupled computer vision for rapid image search. IEEE Trans. Neural Syst. Rehabil. Eng. 14, 174–179.
Gilden, D. L., Thornton, T., and Mallon, M. W. (1995). 1/f noise in human cognition. Science 267, 1837–1839.
Handy, T. C., and Khoe, W. (2005). Attention and sensory gain control: a peripheral visual process? J. Cogn. Neurosci. 17, 1936–1949.
Hari, R., Salmelin, R., Mäkelä, J. P., Salenius, S., and Helle, M. (1997). Magnetoencephalographic cortical rhythms. Int. J. Psychophysiol. 26, 51–62.
Holroyd, C. B., and Coles, M. G. H. (2002). The neural basis of human error processing: reinforcement learning, dopamine, and the error-related negativity. Psychol. Rev. 109, 679–709.
Jensen, O., Gelfand, J., Kounios, J., and Lisman, J. E. (2002). Oscillations in the alpha band (9-12 Hz) increase with memory load during retention in a short-term memory task. Cereb. Cortex 12, 877–882.
Johnson, R. (1993). On the neural generators of the P300 component of the event-related potential. Psychophysiology 30, 90–97.
Kam, J. W. Y., Dao, E., Farley, J., Fitzpatrick, K., Smallwood, J., Schooler, J. W., and Handy, T. C. (2011). Slow fluctuations in attentional control of sensory cortex. J. Cogn. Neurosci. 23, 460–470.
Kelly, S. P., Gomez-Ramirez, M., and Foxe, J. J. (2009). The strength of anticipatory spatial biasing predicts target discrimination at attended locations: a high-density EEG study. Eur. J. Neurosci. 30, 2224–2234.
Kelly, S. P., Lalor, E. C., Reilly, R. B., and Foxe, J. J. (2006). Increases in alpha oscillatory power reflect an active retinotopic mechanism for distracter suppression during sustained visuospatial attention. J. Neurophysiol. 95, 3844–3851.
Klimesch, W., Doppelmayr, M., Russegger, H., Pachinger, T., and Schwaiger, J. (1998). Induced alpha band power changes in the human EEG and attention. Neurosci. Lett. 244, 73–76.
Klimesch, W., Sauseng, P., and Hanslmayr, S. (2007). EEG alpha oscillations: the inhibition-timing hypothesis. Brain Res. Rev. 53, 63–88.
Lage-Castellanos, A., Martínez-Montes, E., Hernández-Cabrera, J. A., and Galán, L. (2010). False discovery rate and permutation test: an evaluation in ERP data analysis. Stat. Med. 29, 63–74.
Leopold, D. A., Murayama, Y., and Logothetis, N. K. (2003). Very slow activity fluctuations in monkey visual cortex: implications for functional brain imaging. Cereb. Cortex 13, 422–433.
Linkenkaer-Hansen, K., Nikouline, V. V., Palva, J. M., and Ilmoniemi, R. J. (2001). Long-range temporal correlations and scaling behavior in human brain oscillations. J. Neurosci. 21, 1370–1377.
Linkenkaer-Hansen, K., Nikulin, V. V., Palva, S., Ilmoniemi, R. J., and Palva, J. M. (2004). Prestimulus oscillations enhance psychophysical performance in humans. J. Neurosci. 24, 10186–10190.
Makeig, S., and Inlow, M. (1993). Lapses in alertness: coherence of fluctuations in performance and EEG spectrum. Electroencephalogr. Clin. Neurophysiol. 86, 23–35.
Mason, M. F., Norton, M. I., Van Horn, J. D., Wegner, D. M., Grafton, S. T., and Macrae, C. N. (2007). Wandering minds: the default network and stimulus-independent thought. Science 315, 393–395.
Mathan, S., Erdogmus, D., Huang, Y., Pavel, M., Ververs, P., Carciofini, J., Dorneich, M., and Whitlow, S. (2008). “Rapid image analysis using neural signals,” in CHI ’08 Extended Abstracts on Human Factors in Computing Systems, CHI EA ’08 (New York, NY: ACM), 3309–3314.
Mathan, S., Whitlow, S., Erdogmus, D., Pavel, M., Ververs, P., and Dorneich, M. (2006). “Neurophysiologically driven image triage: a pilot study,” in CHI ’06 Extended Abstracts on Human Factors in Computing Systems, CHI EA ’06 (New York, NY: ACM), 1085–1090.
Mazaheri, A., Nieuwenhuis, I. L. C., van Dijk, H., and Jensen, O. (2009). Prestimulus alpha and mu activity predicts failure to inhibit motor responses. Hum. Brain Mapp. 30, 1791–1800.
McVay, J. C., and Kane, M. J. (2009). Conducting the train of thought: working memory capacity, goal neglect, and mind wandering in an executive-control task. J. Exp. Psychol. Learn. Mem. Cogn. 35, 196–204.
Monto, S., Palva, S., Voipio, J., and Palva, J. M. (2008). Very slow EEG fluctuations predict the dynamics of stimulus detection and oscillation amplitudes in humans. J. Neurosci. 28, 8268–8272.
Nikulin, V. V., and Brismar, T. (2005). Long-range temporal correlations in electroencephalographic oscillations: relation to topography, frequency band, age and gender. Neuroscience 130, 549–558.
O’Connell, R. G., Dockree, P. M., Robertson, I. H., Bellgrove, M. A., Foxe, J. J., and Kelly, S. P. (2009). Uncovering the neural signature of lapsing attention: electrophysiological signals predict errors up to 20 s before they occur. J. Neurosci. 29, 8604–8611.
Palva, J. M., Palva, S., and Kaila, K. (2005). Phase synchrony among neuronal oscillations in the human cortex. J. Neurosci. 25, 3962–3972.
Parra, L. C., Alvino, C., Tang, A., Pearlmutter, B., Yeung, N., Osman, A., and Sajda, P. (2002). Linear spatial integration for single-trial detection in encephalography. Neuroimage 17, 223–230.
Parra, L. C., Christoforou, C., Gerson, A. D., Dyrholm, M., Luo, A., Wagner, M., Philiastides, M. G., and Sajda, P. (2008). Spatiotemporal linear decoding of brain state: application to performance augmentation in high-throughput tasks. IEEE Signal Process. Mag. 25, 95–115.
Pollen, D. A., and Trachtenberg, M. C. (1972). Some problems of occipital alpha block in man. Brain Res. 41, 303–314.
Poolman, P., Frank, R. M., Luu, P., Pederson, S. M., and Tucker, D. M. (2008). A single-trial analytic framework for EEG analysis and its application to target detection and classification. Neuroimage 42, 787–798.
Ray, W. J., and Cole, H. W. (1985). EEG alpha activity reflects attentional demands, and beta activity reflects emotional and cognitive processes. Science 228, 750–752.
Ridderinkhof, K. R., Ullsperger, M., Crone, E. A., and Nieuwenhuis, S. (2004). The role of the medial frontal cortex in cognitive control. Science 306, 443–447.
Rihs, T. A., Michel, C. M., and Thut, G. (2007). Mechanisms of selective inhibition in visual spatial attention are indexed by alpha-band EEG synchronization. Eur. J. Neurosci. 25, 603–610.
Robertson, I. H., Manly, T., Beschin, N., Daini, R., Haeske-Dewick, H., Hömberg, V., Jehkonen, M., Pizzamiglio, G., Shiel, A., and Weber, E. (1997). Auditory sustained attention is a marker of unilateral spatial neglect. Neuropsychologia 35, 1527–1532.
Romei, V., Brodbeck, V., Michel, C. M., Amedi, A., Pascual-Leone, A., and Thut, G. (2008a). Spontaneous fluctuations in posterior alpha-band EEG activity reflect variability in excitability of human visual areas. Cereb. Cortex 18, 2010–2018.
Romei, V., Rihs, T. A., Brodbeck, V., and Thut, G. (2008b). Resting electroencephalogram alpha-power over posterior sites indexes baseline visual cortex excitability. Neuroreport 19, 203–208.
Romei, V., Gross, J., and Thut, G. (2010). On the role of prestimulus alpha rhythms over occipito-parietal areas in visual input regulation: correlation or causation? J. Neurosci. 30, 8692–8697.
Roth, W. T., Dorato, K. H., and Kopell, B. S. (1984). Intensity and task effects on evoked physiological responses to noise bursts. Psychophysiology 21, 466–481.
Sauseng, P., Klimesch, W., Doppelmayr, M., Pecherstorfer, T., Freunberger, R., and Hanslmayr, S. (2005a). EEG alpha synchronization and functional coupling during top-down processing in a working memory task. Hum. Brain Mapp. 26, 148–155.
Sauseng, P., Klimesch, W., Stadler, W., Schabus, M., Doppelmayr, M., Hanslmayr, S., Gruber, W. R., and Birbaumer, N. (2005b). A shift of visual spatial attention is selectively associated with human EEG alpha activity. Eur. J. Neurosci. 22, 2917–2926.
Smallwood, J., Beach, E., Schooler, J. W., and Handy, T. C. (2008). Going AWOL in the brain: mind wandering reduces cortical analysis of external events. J. Cogn. Neurosci. 20, 458–469.
Smallwood, J., Davies, J. B., Heim, D., Finnigan, F., Sudberry, M., O’Connor, R., and Obonsawin, M. (2004). Subjective experience and the attentional lapse: task engagement and disengagement during sustained attention. Conscious. Cogn. 13, 657–690.
Smith, K. J., Valentino, D. A., and Arruda, J. E. (2003). Rhythmic oscillations in the performance of a sustained attention task. J. Clin. Exp. Neuropsychol. 25, 561–570.
Snyder, A. C., and Foxe, J. J. (2010). Anticipatory attentional suppression of visual features indexed by oscillatory alpha-band power increases: a high-density electrical mapping study. J. Neurosci. 30, 4024–4032.
Stanislaw, H., and Todorov, N. (1999). Calculation of signal detection theory measures. Behav. Res. Methods Instrum. Comput. 31, 137–149.
Teasdale, J. D., Dritschel, B. H., Taylor, M. J., Proctor, L., Lloyd, C. A., Nimmo-Smith, I., and Baddeley, A. D. (1995). Stimulus-independent thought depends on central executive resources. Mem. Cognit. 23, 551–559.
Teasdale, J. D., Proctor, L., Lloyd, C. A., and Baddeley, A. D. (1993). Working memory and stimulus-independent thought: effects of memory load and presentation rate. Eur. J. Cogn. Psychol. 5, 417–433.
Thut, G., Nietzel, A., Brandt, S. A., and Pascual-Leone, A. (2006). Alpha-band electroencephalographic activity over occipital cortex indexes visuospatial attention bias and predicts visual target detection. J. Neurosci. 26, 9494–9502.
van Dijk, H., Schoffelen, J.-M., Oostenveld, R., and Jensen, O. (2008). Prestimulus oscillatory activity in the alpha band predicts visual discrimination ability. J. Neurosci. 28, 1816–1823.
Verplanck, W. S., Collier, G. H., and Cotton, J. W. (1952). Nonindependence of successive responses in measurements of the visual threshold. J. Exp. Psychol. 44, 273–282.
Wagenmakers, E.-J., Farrell, S., and Ratcliff, R. (2004). Estimation and interpretation of 1/falpha noise in human cognition. Psychon. Bull. Rev. 11, 579–615.
Wertheimer, M. (1953). An investigation of the randomness of threshold measurements. J. Exp. Psychol. 45, 294–303.
Worden, M. S., Foxe, J. J., Wang, N., and Simpson, G. V. (2000). Anticipatory biasing of visuospatial attention indexed by retinotopically specific alpha-band electroencephalography increases over occipital cortex. J. Neurosci. 20, RC63.
Wyart, V., and Tallon-Baudry, C. (2009). How ongoing fluctuations in human visual cortex predict perceptual awareness: baseline shift versus decision bias. J. Neurosci. 29, 8715–8725.
Yamagishi, N., Callan, D. E., Goda, N., Anderson, S. J., Yoshida, Y., and Kawato, M. (2003). Attentional modulation of oscillatory activity in human visual cortex. Neuroimage 20, 98–113.
Keywords: attention, alpha, mind-wandering, vigilance, detection, confidence, P300
Citation: Macdonald JSP, Mathan S and Yeung N (2011) Trial-by-trial variations in subjective attentional state are reflected in ongoing prestimulus EEG alpha oscillations. Front. Psychology 2:82. doi: 10.3389/fpsyg.2011.00082
Received: 11 January 2011; Accepted: 16 April 2011;
Published online: 10 May 2011.
Edited by:
Paul Sajda, Columbia University, USAReviewed by:
Tom Eichele, University of Bergen, Norway;Copyright: © 2011 Macdonald, Mathan and Yeung. This is an open-access article subject to a non-exclusive license between the authors and Frontiers Media SA, which permits use, distribution and reproduction in other forums, provided the original authors and source are credited and other Frontiers conditions are complied with.
*Correspondence: James S. P. Macdonald, Department of Experimental Psychology, University of Oxford, South Parks Road, Oxford OX1 3UD, UK. e-mail:amFtZXMubWFjZG9uYWxkQHBzeS5veC5hYy51aw==
Disclaimer: All claims expressed in this article are solely those of the authors and do not necessarily represent those of their affiliated organizations, or those of the publisher, the editors and the reviewers. Any product that may be evaluated in this article or claim that may be made by its manufacturer is not guaranteed or endorsed by the publisher.
Research integrity at Frontiers
Learn more about the work of our research integrity team to safeguard the quality of each article we publish.