- Sheffield Autism Research Lab, Department of Psychology, The University of Sheffield, Sheffield, UK
Intra-participant variability in clinical conditions such as autistic spectrum disorder (ASD) is an important indicator of pathophysiological processing. The data reported here illustrate that trial-by-trial variability can be reliably measured from EEG, and that intra-participant EEG variability is significantly greater in those with ASD than in neuro-typical matched controls. EEG recorded at the scalp is a linear mixture of activity arising from muscle artifacts and numerous concurrent brain processes. To minimize these additional sources of variability, EEG data were subjected to two different methods of spatial filtering. (i) The data were decomposed using infomax independent component analysis, a method of blind source separation which un-mixes the EEG signal into components with maximally independent time-courses, and (ii) a surface Laplacian transform was performed (current source density interpolation) in order to reduce the effects of volume conduction. Data are presented from 13 high functioning adolescents with ASD without co-morbid ADHD, and 12 neuro-typical age-, IQ-, and gender-matched controls. Comparison of variability between the ASD and neuro-typical groups indicated that intra-participant variability of P1 latency and P1 amplitude was greater in the participants with ASD, and inter-trial α-band phase coherence was lower in the participants with ASD. These data support the suggestion that individuals with ASD are less able to synchronize the activity of stimulus-related cell assemblies than neuro-typical individuals, and provide empirical evidence in support of theories of increased neural noise in ASD.
Introduction
Autism spectrum disorder (ASD) is a complex neurodevelopmental disorder that has been estimated to occur in 1.16% of children in the UK (Baird et al., 2006). It is characterized by substantial difficulties in social cognition, interaction, and communication (APA, 1994). In addition to these core deficits, ASD is associated with a wide range of more general impairments in many cognitive domains including, executive function (Hill, 2004), memory (Bennetto et al., 1996), attention (Allen and Courchesne, 2001), and perception (Simmons et al., 2009). An underlying etiology that links impairments across such an array of domains has not yet been identified. The literature on perceptual function is particularly puzzling, as while those with ASD show impaired performance of some tasks, e.g., detecting coherent motion within local motion noise (Milne et al., 2002), they show superior performance on tasks that involve detecting a target within a static array (Plaisted et al., 1998). Furthermore, enhanced and diminished perceptual sensitivity appear to co-occur, as Bertone et al. (2005) have demonstrated enhanced first-order contrast perception and decreased second-order contrast perception within the same group of participants.
A persistent difficulty in identifying the etiology of ASD arises from a high level of inter-participant variability. This is evidenced by the number of studies that discuss participant sub-groups within the ASD sample, and the number of studies in which estimates of variation around the mean/median are larger in the ASD group than in the control group. In addition, although many cognitive functions appear to be impaired in those with ASD, the literature is littered with examples of non-replication, suggesting that there is no specific cognitive impairment that is consistent and universal in ASD (see also Happé et al., 2006). The data on impairments in cognitive task performance in ASD may therefore be better interpreted as arising from a pervasive and generalized impairment rather than a collection of several, different, modality, or task specific impairments. A promising candidate for such a generalized impairment is increased levels of intra-participant variability, as this would lead to increased variability between participants, and represents a parsimonious explanation for the many areas of cognition that appear to be impaired in those with ASD. Furthermore, increased intra-participant variability would lead to reduced test re-test reliability and could therefore explain the high level of inconsistency within the literature.
Variability and fluctuation in behavior and task performance are commonly observed in individuals with developmental disorders (Castellanos et al., 2005). Although most typically associated with ADHD, recent empirical work has demonstrated that individuals with ASD show significantly greater intra-individual response time variability during a simple 2AFC task compared not only to a group of typically developing (TD) matched control participants, but also compared to a group of matched participants with ADHD (Geurts et al., 2008). Intra-individual variability appears to be an important indicator of pathophysiological processing therefore, and its potential to explain a number of task-related behaviors in those with ASD should not be over-looked. However, there are many potential routes to response time variability, including: variability in higher-order cognitive functions such as initiating or generating motor responses (Deutsch and Newell, 2005); transient lapses of attention; and/or variability in early sensory encoding (e.g., Croner et al., 1993; Arieli et al., 1996). The source of intra-participant variability in ASD is therefore unclear.
A number of authors have suggested that neural noise may be increased in individuals with ASD or that increased levels of noise may contribute to reduced cognitive task performance in those with ASD (e.g., Rubenstein and Merzenich, 2003; Baron-Cohen and Belmonte, 2005; Dakin and Frith, 2005; Simmons et al., 2009). In particular some have suggested cortical hyper-excitation especially in primary sensory cortices, which would lead to increased cortical noise in ASD (Rubenstein and Merzenich, 2003). However, as yet, there is no direct evidence for greater neural noise in those with ASD. Increased neural noise would be evidenced by increased variability across individual trials of EEG, therefore the aim of this study is to compare variability in single-trial EEG in a group of children / adolescents with ASD with a group of TD matched controls.
Unfortunately, single-trial analysis of EEG is seldom performed. The main reason for this is that EEG recorded at the scalp constitutes a mixture of a number of sources signals, therefore, activity associated with a single process, being mixed with signals arising from other processes as well as on-going “background” oscillations, is difficult to identify within each trial. Here, EEG data are decomposed with independent component analysis (ICA), which, as described below, un-mixes the different source signals recorded at the scalp, enabling activity from independent processes to be identified in single-trials (Makeig et al., 1997, 2004; Onton et al., 2006) and variability within individuals to be measured.
Whole-brain dynamic processes are underpinned by the formation of cell assemblies, i.e., groups of cells that oscillate in synchrony, or precisely timed succession, for transient periods (Nunez and Srinivasan, 2006). As numerous cell assemblies may be active at any given time, oscillation synchronicity within specific frequency bands is thought to be the mechanism by which the output of single units is identified as being part of a coherent network (Singer et al., 1997). Performing even a simple experimental task will excite a number of different cell assemblies which will be active alongside numerous other task-unrelated assemblies. A difficulty faced by EEG researchers is the fact that electrical activity generated by these separate assemblies becomes mixed, and, via volume conduction, smeared, across the scalp. That is, each EEG electrode records a mixture of signals arising from multiple cognitive processes and from on-going “background” oscillatory activity. Furthermore, scalp electrodes also record activity from non-brain sources including muscle (eye-movements, blinking, heartbeat) and in some cases from non-physiological electrical sources (e.g., line-noise). Filtering and artifact rejection reduces the influence of some of these unwanted contributions to EEG, however, the spatial mixing of numerous brain-based processes means that the signal of interest, i.e., the signal associated with the cognitive task, is mixed with signals from task-unrelated processes and is therefore difficult to observe and measure on a trial-by-trial basis.
This spatial mixing of EEG has shaped the way that EEG data are analyzed, most notably by leading to a dominance in single-trial averaging to calculate the event-related potential (ERP). The theory behind the ERP technique is that by calculating an average of several time-locked trials, event unrelated activity, being phase- and time-random with respect to the time-locking event, cancels to near zero amplitude, whereas the part of the EEG that is time-locked to the relevant event remains visible in the signal. Single-trial analysis is therefore rejected in favor of “average” event-related analyses. However, given the value of understanding variability across single-trials, just as the SD provides vital information regarding the distribution of values around a mean response time, a number of alternate methods have been put forward for facilitating single-trial analysis of EEG data. These include complex filtering (Salajegheh et al., 2004), maximum likelihood estimation (Jaskowski and Verleger, 1999), parametric modeling (von Spreckelsen and Bromm, 1988), multivariate matching pursuit algorithms (Sieluzycki et al., 2009), general linear model analyses (Pernet et al., 2011), and decomposing data using ICA (Jung et al., 2001). ICA provides an elegant solution to the problems associated with spatial mixing of EEG, and facilitates analysis of single-trials by decomposing EEG data into separate informational components of brain dynamics that closely reflect activity associated with specific cognitive or sensory processes, thus removing the need for time-locked averaging (e.g., see Jung et al., 2001).
Independent component analysis is a method of blind source separation that separates N linear mixtures into N independent informational components (Makeig et al., 1997). It is based on the assumption that source signals are statistically independent whereas signal mixtures are not. Maximizing the joint entropy of the extracted signals gives rise to the “un-mixing matrix” W that, when multiplied by EEG data X, produces the original source signals U, i.e., U = WX. The columns of the un-mixing matrix, W, hold coefficients of spatial filters that pass the activity of only one independent source process and suppress all the others. Each IC is represented by the time-course of activation (given by each row of U), and the weights with which the component projects to the electrodes which are given in the inverse of the un-mixing matrix W−1. Plotting these weights onto a schematic head model allows one to visualize the scalp topography of each independent component.
A number of papers have demonstrated the usefulness of decomposing EEG data into ICs, not only for isolating artifactual contributions to scalp EEG, but also for studying on-going oscillatory activity, and event-related activity that contribute to ERP deflections recorded at the scalp (for examples see Debener et al., 2005; Onton et al., 2005; Eichele et al., 2010). Here, ICA is used to identify independent components that represent early (<200 ms) activity evoked by presentation of a simple visual stimulus, in order to compare single-trial EEG variability in those with and without ASD. In addition to decomposing the data with ICA, the EEG epochs were converted to current source density (CSD) models (e.g., Kayser and Tenke, 2006). CSD transforms compute the second spatial derivative of voltage between nearby electrode sites, which enhances local electrical activity while attenuating distal activity. By emphasizing local contributions to the surface map, some of the variability associated with spatial smearing via volume conduction may be reduced. Comparing measures of variability obtained from the raw channel EEG with measures of variability obtained from two different methods of spatially filtering will illustrate which method produces the least variable data, thus ensuring that the most appropriate source of data is used when comparing variability between the participants with and without ASD.
The result in this paper present a re-analysis of data collected for a previous study (Milne et al., 2009), in which the visual evoked response in children/adolescents with and without ASD was investigated. Previously, we compared amplitude and latency indices of the visual evoked potential (VEP, e.g., the C1 and P1 deflections), and changes in α- and γ-band power associated with presentation of Gabor patches at a range of different spatial frequencies. We found that the time at which spectral power increase following stimulus onset was reduced in the participants with ASD (see also Isler et al., 2010), and that the extent to which the spatial frequency content of the stimuli modulated α- and γ-band power was less in the participants with ASD (see also Jemel et al., 2010). Here, I now investigate intra-participant EEG variability: single-trial variability across the time-course is analyzed by comparing point-by-point amplitude variability across trials, and also by computing the degree of inter-trial phase consistency across the time-course. In addition, variability of P1 amplitude, i.e., the consistency of P1 magnitude, and variability of P1 latency, i.e., the consistency of the time at which the peak occurs will be investigated. The P1 deflection is a positive going deflection within the VEP. It peaks between 100 and 160 ms after stimulus onset, is maximal over posterior leads and is generated within the extra-striate cortex. The P1 was selected for analysis as it is time-locked and phase-locked to stimulus onset. Therefore a “P1-like” increase in amplitude should be visible across single-trials, enabling variability between the two groups to be compared1.
Materials and Method
Participants
Data were collected from 20 children/adolescents with ASD. Data from two participants were not analyzed as these participants had co-morbid diagnoses of ADHD. Data from another participant were excluded as despite having a clinical diagnosis of ASD, she failed to reach cut-off for ASD on the two screening measures that we used to ensure the homogeneity of our sample (further details are given below). From the remaining 17 participants, data from four participants were excluded as these participants did not show an independent component in their data decomposition that fulfilled the criteria imposed for IC selection (further details are given below). Data are therefore presented from a total of 13 participants with ASD.
Participants in the TD control group were selected from a sample of 37 participants (19 male) who performed this task either as part of another study investigating developmental change of the VEP, or as part of the previously published study (Milne et al., 2009). Data from 12 of these participants is presented here; their inclusion was based on being a suitable match to one of the participants with ASD in terms of gender, age, and IQ and generating data from which an independent component that fulfilled the selection criteria (see below) was identified. The two samples of data were therefore well matched both in terms of participant characteristics and data quality. Participant details are provided in Table 1.
The participants with ASD were recruited from a local clinic for children with pervasive developmental disorders. Only participants with a clinically defined diagnosis of autism (N = 5), Asperger’s syndrome (N = 5), or ASD (N = 3) were recruited2, no participant had a co-morbid diagnosis, or a known specific neurological or genetic condition (e.g., Fragile X, Rett Syndrome) that could account for their diagnosis of ASD. The TD children were recruited from an e-mail list of volunteers and were screened for any history of developmental, neurological, or genetic disorder. No participant had taken medication within 24 h of participation. Written informed consent was obtained from the parents of all participants and verbal assent was obtained by the participants prior to testing. The procedures followed were in accordance with the ethical standards of the South Sheffield NHS ethics committee and the Declaration of Helsinki.
Psychometric Assessment
Degree of ASD symptoms were assessed in all participants by way of an observational measure (the Childhood Autism Rating Scale, CARS, Schopler et al., 1988) and a parental questionnaire (the Social Communication Questionnaire, SCQ – lifetime version Berument et al., 1999). The CARS requires the experimenter to rate the participant from a scale of 1–4 on 15 item behavioral rating scale, for example “emotional response” and “fear and nervousness.” Scores range from 15 to 60 and the cut-off for ASD is 30. The SCQ consists of 40 “Yes/No” questions asking parents if their child currently displays specific autism-related behaviors or whether those behaviors were present between the ages of 4–5 years. Scores range from 0 to 40 and the cut-off score for ASD is 15. Intellectual ability was measured using the Wechsler Abbreviated Scale of Intelligence (WASI, Wechsler, 1999). This is comprised of four standardized sub-tests that assess expressive language, perceptual organization, abstract verbal reasoning, and non-verbal fluid reasoning abilities. The four sub-tests when considered together yield a “full scale IQ” that provides a composite measure of the participant’s general intelligence. All participants had normal, or corrected-to-normal, visual acuity.
Stimuli and Procedure
Gabor patches were created using Matlab (The Mathworks, Inc.) and the psychophysics toolbox (Brainard, 1997). They were presented on a 17-inch monitor, which refreshed at 75 Hz. Stimuli were centrally presented on a gray background (average luminance = 14.4 cd/m2). The space-average luminance of each grating was 16.3 cd/m2, and the Michelson contrast, defined by (Lmax − Lmin)/(Lmax + Lmin) was 68%. The slight difference between the average luminance of the background and the stimuli was not visibly apparent and did not lead to any visible edges around the stimuli. At a viewing distance of 114 cm the patches subtended 6.78° by 6.78° of visual angle. All patches were presented in diagonal (45°), orientation, had a gaussian envelope with SD of 0.68°, and with spatial frequency modulation of 0.5, 1, 4, or 8 cycles/degree. An additional stimulus, a gray-scaled image of a zebra was presented. Participants were instructed to respond by pressing a response button with the index finger of their dominant hand as quickly as possible whenever they saw the zebra.
Each of the four Gabor patches was shown 72 times, the zebra was shown 36 times. The order of stimulus presentation was randomized. Each stimulus remained on screen for 39 refresh cycles (507 ms), with an additional variable inter-stimulus interval of either 24 (312 ms), 39 (507 ms), or 54 (702 ms) refresh cycles. A white fixation cross measuring 0.2° by 0.2° remained in the center of the screen for the duration of the task. Participants were asked to maintain fixation and to limit their blink frequency during the experiment.
Data Recording
EEG was continuously recorded using a high-density array of 128 Ag/AgCl electrodes (Electrical Geodesics Inc., Tucker, 1993). Impedance was kept below 50 kΩ. The signal was amplified (×1000), filtered on line with a band-pass of 0.01–80 Hz, then digitized at a sampling rate of 1 kHz. The electro-oculogram (EOG) was recorded from bipolar electrode pairs located at the outer canthi and above and below the left and right eyes. Data were referenced to the vertex electrode, and were stored on the hard drive of a G4 Macintosh power PC.
Data Pre-Processing
Data were analyzed off-line using EEGLAB (Delorme and Makeig, 2004, http://www.sccn.ucsd.edu/eeglab), and the CSD toolbox (Kayser and Tenke, 2006; Kayser, 2009) running under Matlab 7.4 (The Mathworks, Inc.). A number of pre-processing steps were performed on the data before applying either ICA or CSD interpolation. First, the data were high-pass filtered (1 Hz) to minimize drift. Then the number of channels was pruned from 128 to 64. Pruning was necessary in order to improve the quality of ICA decomposition, given the relatively small amount of data recorded (∼5 min). Initially channels that showed noise artifacts due to poor connection to the scalp were deleted, then channels were removed if they showed high kurtosis, finally, additional channels that showed the smallest inter-electrode distance were removed until 64 relatively evenly spaced electrodes remained. In some cases a small number of additional channels were deleted by the experimenter if any noise artifacts on any particular channel were still visible. (Given the small amount of data recorded there was a bias toward rejecting channels rather than portions of data in order to facilitate ICA.)
After channel pruning, noisy segments of data, i.e., segments that contained gross artifacts such as muscle twitching or swallowing were removed from the data. At this stage, the data were (i) decomposed by extended infomax ICA using the function runica, as implemented in EEGLAB, and then segmented into epochs associated with presentation of the high spatial frequency Gabor patch (8 cycles/degree); epochs were 800 ms long (−100 ms pre-stimulus), and baseline corrected by subtracting the mean of the 100 ms pre-stimulus interval, and (ii) segmented into epochs as described above and then transformed into CSD estimates (measured in μV/cm2) using a spherical spline surface Laplacian (Perrin et al., 1989) as implemented by Kayser and Tenke (2006), Kayser (2009), with a spline-smoothing coefficient (λ) of 1.0−5. Both sets of data were then low-pass filtered (<30 Hz).
Independent component source locations were estimated by creating an equivalent current dipole model for each component using the dipfit function from EEGLAB, this function estimates dipole location by applying inverse source modeling methods to a standard boundary element head model. The coordinate system used to specify electrode locations of both sets of data is publicly available as a look-up table [“10-5-System Mastoids EGI129.csd”] via the CSD toolbox. Three types of data were analyzed: (i) the back-projected independent time-course of the selected component (see below), (ii) the time-course of the CSD interpolated data from the selected channel (see below), and (iii) the time-course of the raw, spatially un-filtered data from the selected channel.
Data Selection
IC selection
Only components whose scalp maps had <15% residual variance from the best-fitting forward model scalp projection were considered for further analysis. Any remaining components that reflected muscle activity, electrocardiogram, or eye-movements, on the basis of their dipole location, spectra, and scalp maps, were considered artifacts and excluded from further analysis. From the remaining components, selection of the component that represented activity associated with visual perception was based on two criteria: (i) the back-projected time-course was required to show time-locked increase in amplitude between 100 and 170 ms after stimulus onset; and (ii) the estimated equivalent current dipole was required to be located in posterior cortex. In all cases the selected component accounted for a larger percentage of the variance of the total EEG than any other component between 100 and 170 ms. The mean percentage variance accounted for by the selected components in this time-window was 70%, indicating that the selected components contributed substantially to the VEP. The mean residual variance of the dipole fit for the selected independent components was reasonably low (6.5%), suggesting that the selected ICs reflect the activity of a compact region in the cortex. The estimated Talairach coordinates of the equivalent current dipole of each component suggested that the neural generators of the component activations were located in extra-striate cortex.
Channel selection
Channel selection was based on an optimized electrode approach (e.g., Foxe and Simpson, 2002), whereby the channel that showed the highest P1 amplitude, from the CSD interpolated data, was selected for analysis. The CSD scalp maps at the latency at which the P1 peak was maximal, with the selected electrode marked by a black dot are presented in Figure 1 (left column); scalp maps from the IC selected for each participant are presented in Figure 1 (right column). Despite not being used in the IC selection criteria, the similarity between the weight with which the ICs project to the scalp electrodes and the topography of the CSD maps at the time-point when P1 was maximal, confirms that the selected component represent activity associated with the P1 deflection.
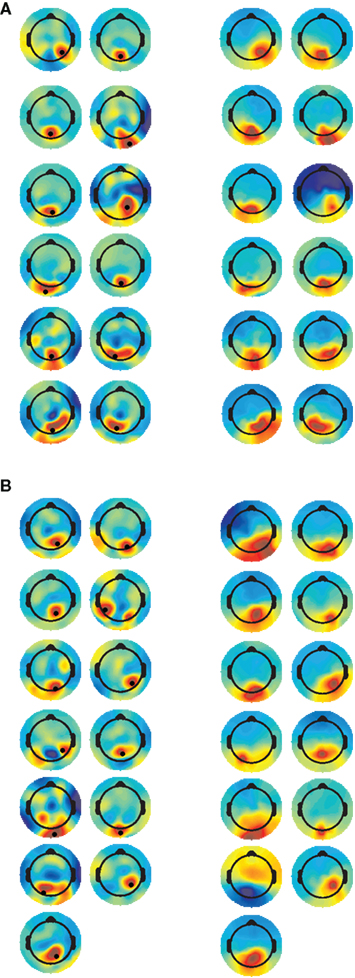
Figure 1. Current source density (CSD) and IC scalp maps. CSD maps (left panel) are plotted at the time when P1 amplitude was highest. The electrode selected for analysis is indicated by the black dot. IC scalp maps (right panel) are stationary, and therefore constant across the time-course. [(A) = TD, (B) = ASD].
Defining the Variables of Interest
P1 amplitude and latency
A number of variables were calculated from the three sources of data. In line with conventional ERP analyses, P1 amplitude was given by the highest (peak) amplitude between 100 and 170 ms; P1 latency was given by the time of this peak from stimulus onset. For the single-trial analyses, the highest amplitude between 100 and 170 ms was identified in each trial; P1 amplitude was calculated as the median amplitude value, P1 latency was calculated as the median time at which these single-trial peaks occurred.
Variability
Variability in the expression of the P1 amplitude was estimated by calculating the median absolute deviation (MAD) of the P1 amplitude and latency values, normalized by dividing by the median. In addition, across-trial amplitude variability was calculated at each time-point in the epoch. Due to the inherent difficulty of calculating co-efficients of variation when the central tendency is close to 0, amplitude values were normalized prior to computing the MAD estimate by converting all of the data to a z-score.
The degree to which each participant showed phase coherence over trials was calculated by computing an inter-trial phase coherence (ITPC) measure. ITPC is a frequency domain measure that indicates the degree to which EEG activity is phase-locked, across trials, to specific experimental events – in this case presentation of the 8 cycles/degree Gabor patch. The power spectrum of each trial was calculated using a hanning tapered fast Fourier transform with a window size of 128 ms. α-Band power was dominant in the signal following stimulus presentation, therefore inter-trial α phase coherence, specifically at 11.7 Hz, was calculated using the newtimef function in EEGLAB. The highest ITPC value within the 100–170 ms post stimulus onset time-window was extracted for each participant to give an indication of the maximum level of ITPC, a high level (up to 1) indicates strong phase coherence, i.e., low variability; a low level (down to 0) indicates weak phase coherence, i.e., high variability. In addition, ITPC was calculated across the time-series.
In sum, three single variables were extracted for analysis – variability of P1 amplitude, variability of P1 latency and maximum ITPC. The amplitude and ITPC time-courses were also compared on a point-by-point basis.
Results
A positive going deflection was seen in the single-trials from all three sources of data. This is illustrated in Figure 2, which shows the single-trial amplitude across the epoch from a TD participant and a participant with ASD as measured from each source of data.
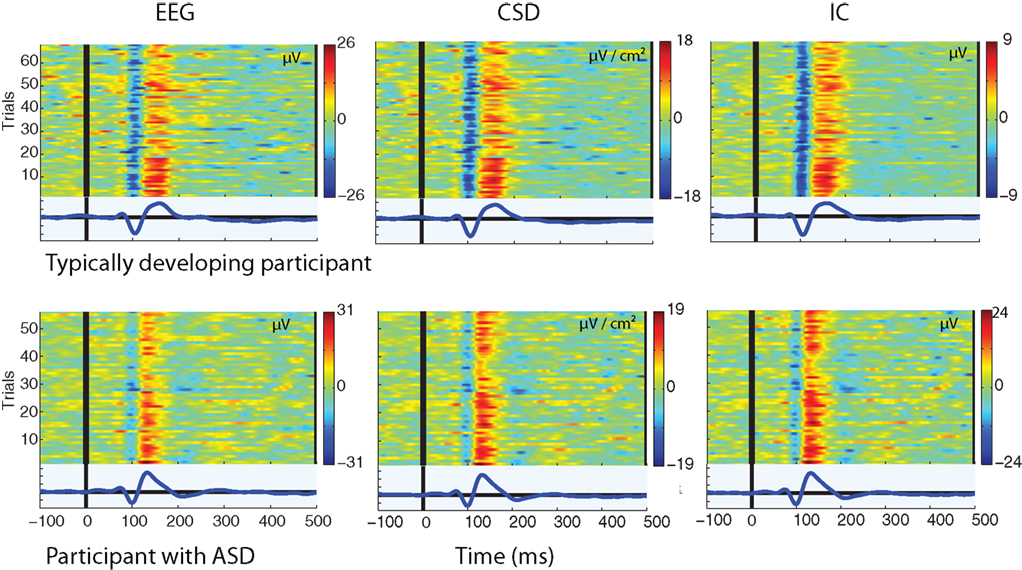
Figure 2. Single-trial amplitude from the raw EEG, CSD interpolated data, and back-projected IC data. Each colored trace on the y-axis indicates amplitude across the time-course of a single-trial (red = +ve, blue = −ve as shown on colormap). The blue trace below each figure is the average of the single-trials.
Which Source of Data Shows the Highest Variability?
The three measures of peak variability (see above), from all subjects, were entered into separate one-way ANOVAs with a within-subject factor of data source (raw EEG, CSD interpolated data, and back-projected IC). All three ANOVAs showed a significant effect of data source (all F > 3.4, all p < 0.05) and were therefore followed-up with paired-samples t-tests. These analyses indicated that there was no difference in variability when measured from the CSD interpolated data and the back-projected IC data (all t < 1, all p > 0.1), but the variables computed from the raw channels were significantly more variable than the variables computed from the spatially filtered sources. The data are presented in Table 2. Based on these results, the following analyses of group differences in variability were performed using the spatially filtered data rather than the raw EEG.
Group Comparison: Intra-Participant Variability
The extent to which the participants with ASD showed increased variability when compared with the neuro-typical participants was investigated with three 2 × 2 repeated measures ANOVAs with a within-subject factor of data source (CSD or ICA) and a between subjects factor of group (ASD or TD). The results of these analyses are shown in Table 3, and the data are plotted in Figure 3A. The participants with ASD showed, on average, greater variance than the neuro-typical participants. This was the case for both measures of P1 variability: amplitude variability, ASD mean = 0.38 [0.33 0.43], TD mean = 0.30 [0.25 0.35]; latency variability, ASD mean = 0.07 [0.05 0.08], TD mean = 0.04 [0.03 0.06]; and also for the maximum α-band ITPC, ASD mean = 0.74 [0.66 0.83], TD mean = 0.87 [0.78 0.96].

Table 3. Results of statistical tests comparing measures of variability in the two groups of participants.
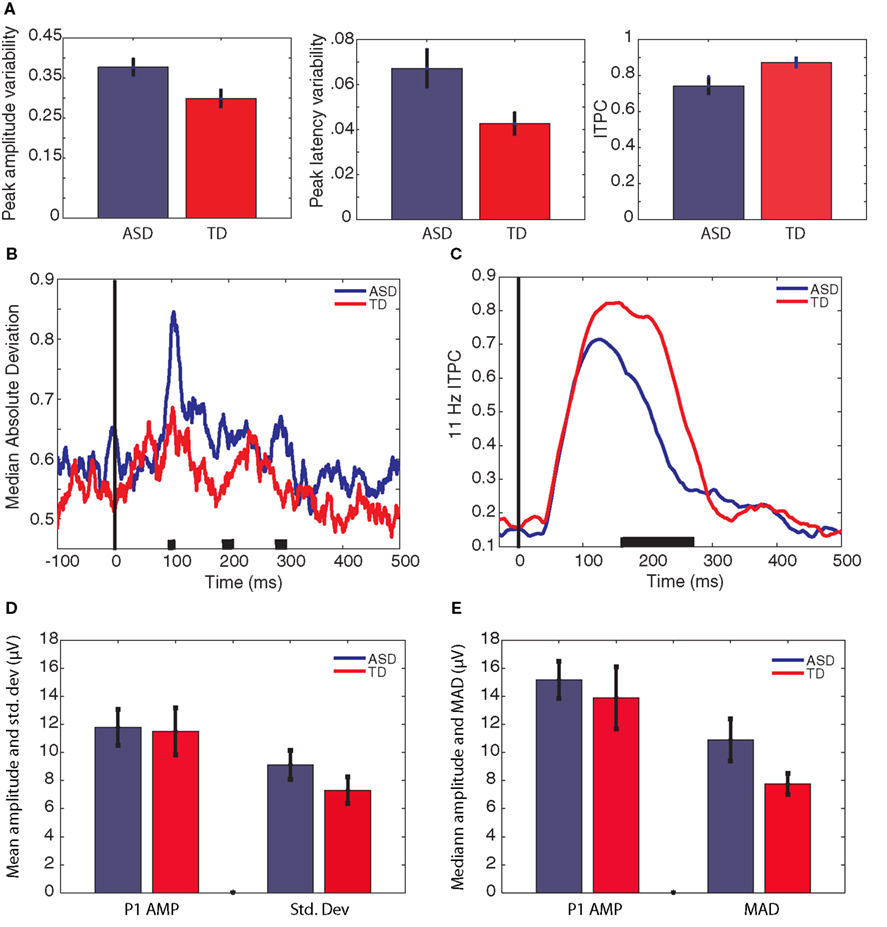
Figure 3. Estimates of variability averaged across group. [(A) (normalized)] measures of peak variability. The left graph shows mean variation in the amplitude of the P1 peak, the middle graph shows mean variation in the latency of the P1 peak, and the right graph shows the mean maximum ITPC between 100 and 170 ms. [(B) (normalized)] median absolute deviation in amplitude across trials at each time-point, averaged across participant groups. (C) ITPC at each time-point, averaged across participant groups. The black lines on the x-axis of plots (B) and (C) indicate time-points of group difference (p < 0.05). P1 amplitude is shown in plots (D) and (E). (D) P1 amplitude calculated from the ERP peak, and the SD of the single-trial P1 peaks. (E) P1 amplitude calculated as the median of the single-trial P1 peaks, and the median absolute deviation of the single-trial P1 peaks. Bars represent ±1 SE.
Figure 3B illustrates amplitude variability in the two groups of participants at each time-point. Figure 3C illustrates α-band ITPC in the two groups of participants at each time-point. In order to compare these group time-courses, independent-samples t-tests were computed at each time-point. ITPC was significantly reduced (p < 0.05) in the participants with ASD between 160 and 271 ms after stimulus onset. Amplitude variability was significantly greater (p < 0.05) in the participants with ASD between 97 and 114 ms, between 185 and 194 ms, and between 283 and 300 ms after stimulus onset.
Group Comparison – P1 Amplitude and Latency
Although not the main area of interest in this article, P1 latency and amplitude were compared between groups as this is the first study to report P1 amplitude calculated from single-trials rather than the average ERP in individuals with ASD. It has been suggested that individuals with ASD may show hyper-sensitivity in posterior cortical areas associated with early visual perception (Mottron et al., 2006). As such, significantly greater P1 amplitude could be predicted3. Given that individuals with ASD show increased latency jitter compared to TD participants (see above), the amplitude of the ERP could be artificially reduced in this population, masking any such effect. By contrast, computing P1 from the single-trials creates a variable that is immune to the effects of latency jitter. However, there was no group difference in P1 amplitude from any data source when calculated from either the ERP peak or the median of single-trials (all t < 1. all p > 0.4). Figures 3D,E illustrate P1 amplitude when calculated as the peak of the ERP (Figure 3D) and the median amplitude of the single-trial peaks (Figure 3E). In addition these figures show variability as the SD of the single-trial peaks (3D) and the MAD of the single-trial peaks (3E). For comparison with previously published data, data from the raw EEG is presented in order to illustrate the difference in amplitude when calculated from the ERP and from the median of the single-trials.
Latency to peak was compared in the two groups using a repeated measures ANOVA with three levels: data source (EEG, CSD, or ICA), data type (ERP or median of single-trials) and group (ASD or TD). The only significant effect was the main effect of group, F(1,23) = 9.4, p < 0.01 which reflected the fact that latency to peak was faster, on average 18 ms faster, in the ASD group than the TD group, regardless of the data source or the data type. Table 4 presents P1 amplitude and latency values in each group as calculated from the different sources of data. The table illustrates that, as expected, when calculated from the median of single-trials rather than the peak of the ERP, P1 amplitude is larger, whereas the latency of the peak does not differ.
Under some circumstances, ERP amplitude has been shown to decrease over multiple trials (e.g., Maurer et al., 2008). Therefore, in order to evaluate whether increased variability in the participants with ASD may reflect a larger habituation-effect, linear regressions of trial number against (IC) peak amplitude were computed for each participant. Mean slope size did not differ between groups however, indicating that this was not the case (TD mean β = −0.04 [−0.13 0.05], ASD mean β = −0.06 [−0.15.03], t(23) < 1, p > 0.1.
Data Integrity
Differences in data integrity between the two groups could conflate estimates of variability, therefore a number of analyses were carried out to ensure that data from both groups were of similar quality. The mean number of epochs analyzed did not differ between the two groups, TD = 58.6 [52 65], ASD = 53.3 [47 60], t(23) = −1.2, p = 0.241, suggesting that any differences in variability between the two groups are unlikely to arise from unequal number of epochs being submitted to analysis. There was no significant difference in the percentage variance accounted for by the selected ICs within the P1 time-window, TD = 70.7% [59.8 81.6], ASD group = 69% [58.6 79.5], t(23) < 1, p = 0.822. Furthermore, there was no significant group difference in residual variability of the dipole fit, TD = 7.1% [5.7 8.6], ASD = 5.9% [4.5 7.3], t(23) = −1.28, p = 0.213), suggesting that the quality of the ICA decomposition was similar between the two groups.
Correlation with Reaction Time
The experiment from which these data are taken required participants to press a response key whenever they saw an oddball stimulus – a zebra. The groups did not differ in the number of responses made, TD = 34.3 [33.0 36.9], ASD = 34.5 [32.2 36.3], t(20) < 1, p = 0.657, in mean reaction time TD = 393.3 [363 413], ASD = 412.4 [378 431], t(20) = 1.37, p = 0.307, nor in the mean MAD estimate of reaction time variability, TD = 45.1 [34.4 52.5], ASD = 38.2 [28.2 48.1], t(20) < 1, p = 0.423. However, there was a significant relationship between reaction time variability and peak amplitude variability, rs(22) = 0.479, p = 0.024. (Behavioral data from three participants with ASD was unavailable due to a technical fault.)
Discussion
The aim of this work was to establish whether those with ASD show greater variability across single-trial evoked EEG compared with neuro-typical individuals. A second aim was to compare single-trial EEG variability when extracted from spatially filtered data and from raw-scalp EEG data in order to select the most appropriate variables for group comparison. All three measures of peak variability – P1 amplitude, P1 latency, and maximum α-band phase coherence – were smaller when analyzed from the spatially filtered data than from the scalp EEG data, highlighting the benefits of applying spatial filtering techniques to EEG. Having validated the use of CSD and ICA in this study, measures of single-trial variability were compared between the participants with and without ASD, with the finding that intra-participant variability was significantly greater in the participants with ASD than in the control group.
These data suggest that previous reports of increased response time variability in those with ASD (Geurts et al., 2008) may be underpinned by variability within cortical dynamics associated with the ability to synchronize the activity of stimulus-related cell assembly(ies) consistently across trials. The experimental paradigm used here did not elicit a significant difference between response time variability in those with and without ASD. This may be because only a small number of trials (36) were available to ascertain variability, in contrast to Geurts et al. (2008) who used nearly twice as many (64 trials), or it may be because of the small group sizes and consequently reduced power of the analyses performed here. Nevertheless, there was a significant relationship between response time variability and P1 amplitude variability. Note that P1 and the behavioral data were extracted from separate trials (the P1 was extracted from trials in which the eliciting stimulus was a Gabor patch with a spatial frequency content of 8 cycles/degree and the behavioral data were derived from trials in which the eliciting stimulus was a zebra), therefore it is not the case that specific trial-by-trial variations in ERP amplitude are driving the variability in response time, rather it appears as though a common mechanism may underpin both behavioral variability and ERP amplitude variability.
As described in the Introduction, neurocortical dynamics result from the activation of partially distinct and interacting cell assemblies; the mechanism of communication within these cell assemblies is synchronous oscillations. A number of authors have suggested that ASD may be characterized by reduced neural synchrony, especially of high-frequency (γ-band) oscillations (e.g., see Brock et al., 2002), although evidence to support this position is mixed. While some studies have shown lower levels of evoked γ-band power in those with ASD (Wilson et al., 2007), more recent data indicates that while evoked γ-band power may be reduced in those with ASD, induced γ-band power is increased, and inter-trial γ-band phase coherence (ITPC) is reduced (Rojas et al., 2008). The concept of “evoked” or “induced” EEG is defined by whether or not single-trial activity is time- and phase-locked to a stimulus (evoked activity),or whether it is perturbed by the stimulus, but neither time- nor phase-locked to it (induced activity). However, as the data presented above, and numerous other estimations of ITPC (e.g., Tallon-Baudry and Bertrand, 1999) illustrate, complete phase-locking across trials (i.e., ITPC = 1) is physiologically unrealistic. Therefore the boundary for defining whether stimulus-related activity should be considered to be evoked or induced is unclear. Rojas et al. (2008) point out that their data fit a model in which total (evoked + induced) stimulus-related γ-band power is equivalent in the participants with and without ASD, and that reduced inter-trial phase consistency, computationally, leads to a reduction of what is classed as evoked activity and an increase in what is classed as induced activity. Thus these authors conclude that the production of γ-band oscillations in response to external stimulation is no different in those with and without ASD, rather their data point toward dysfunction in the timing of γ-band oscillations in the participants with ASD.
The data reported here provide evidence of reduced ITPC in the α-band in ASD. Together with the result of Rojas et al. (2008), these data indicate widespread dysfunction of neural timing in ASD, rather than a specific deficit of high-frequency γ-band oscillations as some authors have predicted. Reduced ITPC in ASD indicates that those with ASD are less able to synchronize the activity of stimulus-related cell assembly(ies) consistently across trials, and provide evidence for temporal disruptions in the organization and recruitment of cell assemblies. It is not clear whether this temporal disruption underpins, is caused by, or is unrelated to, postulated neural de-synchrony in ASD.
A number of possible etiologies of atypical neural oscillations in ASD have been suggested, including: a surfeit in local connectivity – especially in primary sensory areas (Belmonte et al., 2004); smaller and more dispersed cortical mini-columns leading to a reduction in inhibitory inter-neuronal activity (Casanova et al., 2002); an imbalance of cortical excitation and inhibition due to increased glutamergic/reduced GABAergic signaling (Rubenstein and Merzenich, 2003); and impairment in the inferior olive – a structure that that mediates electrical synapses and that drives neural synchrony, and has been found to be structurally atypical in some individuals with ASD (Welsh et al., 2005). No theory has yet linked any of these putative impairments with increased intra-participant variability in those with ASD. However within the literature on ADHD, intra-participant variability has been theoretically linked with inconsistent and inefficient neuronal transmission, which may arise from impairment in astrocytes, a type of glial cell that plays a critical role in fueling neuronal oscillations (Russell et al., 2006). Astrocyte impairment in ASD could therefore account for a range of features of ASD including neural de-synchrony, EEG single-trial variability, and behavioral (response time) variability. Given the important role of glia in synapse formation and maintenance (Bolton and Eroglu, 2009) the suggestion that astrocyte impairment may be a critical factor in ASD is a tantalizing one. It is important to note however that, in addition to the theoretically formulated suggestions described above, a variety of neuronal characteristics (e.g., synaptic transmission, channel gating, fluctuation in transmitter release, postsynaptic receptor activation, ion concentrations, membrane conductance) may contribute to variability of evoked EEG response (Sannita, 2006), therefore it is not currently possible to identify the precise source(s) of EEG variability.
Regardless of the precise source, increased EEG variability in those with ASD is evidence of increased intrinsic neural “noise” (Sannita, 2006). Increased neural noise in ASD has been predicted by a number of authors (see Simmons et al., 2009), however, the data reported here represent the first empirical demonstration of increased neural noise in ASD. Increased neural noise has the potential to influence behavior in a variety of ways, and its impact on different levels of function, e.g., perception, cognition, and behavior, may not be consistent. Whilst an increased noise-to-signal ratio leads to reduced perceptual sensitivity in many cases, one type of noise – stochastic resonance – can amplify a signal, leading to increased sensitivity. Increased levels of neural noise have therefore been discussed in relation to atypical perception in ASD, and offered as a parsimonious explanation of data in which those with ASD show both hyper- and hypo-reactivity to perceptual stimuli and enhanced and impaired perceptual sensitivity measured with psychophysical tasks (Simmons et al., 2009).
Increased neural noise is less likely to have an advantageous effect on cognitive task performance however, as it may lead to a number of sub-optimal outcomes including a general decrease in response times and greater response time variability, more errors in tasks with more than one possible response, and the need for increased repetitions of a task to achieve the same outcome as those with lower levels of noise. Furthermore, noise-related reduction in task performance would be evidenced by impairments across many domains and tasks, rather than in isolated tasks, and it would also lead to increased inter-participant variability. This description of data is very similar to that represented by the literature on cognitive function in ASD. Increased neural noise is therefore a plausible, and parsimonious, explanation both for the array of cognitive tasks in which participants with ASD have been shown to perform more poorly than those without ASD, and for the significant inter-individual variability present in those with ASD. In support of this position are two demonstrations where reduced task performance can be accounted for by what may be termed “noise.” For example, thresholds for detecting coherent motion can be artificially inflated by transient lapses of attention (McAnally et al., 2001), and intra-individual response variability is a strong predictor of success in the Go No-Go task (Bellgrove et al., 2004), suggesting that lower sensitivity to coherent motion and failure to inhibit prepotent responses, both of which have been reported in those with ASD (see Ozonoff et al., 1994; Milne et al., 2002 respectively), may arise due to increased neural noise rather than reflecting a specific impairment in either motion perception or in response inhibition, as is the current interpretation of these data (see also Baron-Cohen and Belmonte, 2005 for a similar argument).
Some authors have suggested that cortical hyper-excitability in ASD may be restricted to/more pronounced in, primary sensory areas (e.g., Rubenstein and Merzenich, 2003; Mottron et al., 2006). Therefore in order to evaluate these results in light of current theories it is necessary to consider where the neural generators of the P1 deflection analyzed here might be. The location of the electrodes selected from the CSD data, and the estimated location of the equivalent current dipole of the ICs suggests that the P1 analyzed here is generated in extra-striate cortex. This is commensurate with a number of papers that have localized the neural generators of the P1 deflection to the extra-striate cortex (e.g., Di Russo et al., 2001; Ales et al., 2010). Therefore, these data provide evidence for variability in extra-striate cortex rather than primary visual cortex. As noted in the Introduction the earlier C1 deflection would be a more appropriate deflection with which to investigate variability in primary visual cortex, however this was not analyzed here as a number of participants with ASD failed to show a clear C1 deflection, either in the (averaged) ERP or in the single-trials. The specific reason for this is unclear, but is being addressed by on-going studies by our research group. Therefore, although the P1 deflection reported here does not tap the earliest stage visual processing, it was the most robust early deflection in the data, making it the best available candidate to investigate increased noise in the visual cortex in ASD. Future studies are required to establish whether areas of primary sensory cortex, generating earlier ERP deflections, show similar, or possibly greater, levels of variability in participants with ASD.
In addition to increased variability, the P1 peak in the participants with ASD occurred significantly sooner than in the control group. This finding, from these data, was reported previously (Milne et al., 2009), so will not be dwelt on here. Nevertheless, reduced latency to peak is commensurate with the suggestion of local hyper-connectivity in ASD which has been predicted by some to lead to increased cortical noise (see for example, Belmonte et al., 2004).
There are a number of implications from this work for existing EEG studies in those with ASD. Consistent with previous reports, these data provide no evidence for difference in P1 amplitude in participants with ASD. Although there is one report of reduced P1 amplitude in children/adolescents with pervasive developmental disorder, including ASD (Hoeksma et al., 2004), this may have been due to latency jitter, as ERP amplitude (when calculated from the peak of the averaged single-trials) is intrinsically related to latency variability. Conversely, the suggestion that individuals with ASD may have hyper-responsive visual cortices would predict increased P1 amplitude in those with ASD, and increased latency jitter may mask this potential outcome. However, the data did not support this prediction, as when P1 amplitude was calculated as the median of the single-trial peaks, there was still no group difference in P1 amplitude. The data reported here indicate that establishing degree of latency jitter within each participant is possible, and should be an essential part of ERP analysis if conclusions are to be drawn to regarding the origin of observed group differences. Before leaving this point, it is important to point out that a number of physiological factors contribute to ERP amplitude. Although ICA was able to isolate the signal associated with perceptual encoding from the total EEG, and therefore facilitate comparison of within-participant variables such as variability, it cannot address the potentially confounding factors of individual differences that may lead to differences in ERP amplitude between groups including differences in cortical convolution, position of the calcarine sulcus, and/or conductivity of underlying tissue, etc. Given that there is some evidence of cortical folding abnormalities in children with ASD (Nordahl et al., 2007), direct comparison of EEG amplitude or EEG power between experimental groups, without first normalizing the data, may not be a reliable technique.
When analyzing the data presented here, significant attempts were made to minimize potential confounds that could artificially inflate the estimates of variability in one group or another. Note that the within-subject estimates of variability reported here are normalized, thus validating group comparisons; note also that the two groups of participants were well matched as regards to age, IQ, and gender, and that the data from the two groups was matched in terms of quality. It is therefore unlikely that methodological factors contributed to the group differences reported here. However, as is outlined in the methodology, data is not reported from all participants who participated in the study. This was driven primarily by the goal of ensuring well matched samples, but was also a consequence of the fact that not all participants generated an IC that was considered reliable enough to be analyzed. The most likely reason for this is that only a small amount of data (approximately 51/2 min of data per participant) were recorded and available for ICA decomposition. The experiment was necessarily short given the age of the participants, but the quality of ICA decomposition would be greatly improved with longer recordings, therefore future work should aim to replicate the findings reported here with larger groups of participants and with longer data recordings.
Behavioral variability is not unique to those with ASD (Castellanos et al., 2005), therefore future research is required to establish the universality of increased EEG variability in ASD and in other developmental disorders (such as ADHD), and to establish whether increased variability is a general characteristic of brain pathology, or whether distinctive features of variability occur in different developmental disorders. Furthermore it is necessary to establish the extent to which increased EEG variability is an enduring endophenotype of ASD, or whether it related either to external factors such as context or particular task requirements, or to internal factors such as cognitive state (e.g., awake, asleep, tired, alert). The presence of a significant correlation between EEG variability and response time variability provides preliminary evidence that response time variability and EEG variability are related, albeit in a small sample of participants. This relationship should be tested more rigorously in future studies in which larger groups of participants are tested and different types of behavioral response tasks (such as simple reaction time, choice reaction time, response inhibition, etc.) are performed. In addition, more detailed single-trial analyses should be performed in order to examine the temporally dynamic patterns of EEG fluctuations, and the relationship between EEG variability and cognitive task performance and both inter- and intra-participant variability needs to be clarified.
Note that the mean P1 peak amplitude variability, measured as the MAD estimate of the P1 peak amplitude from the raw EEG data in the TD group, was 0.37. This broadly concurs with existing data in which the coefficient of variation of VEP amplitude recorded in 100 healthy adults from electrodes positioned above the occipital cortex was reported to be 0.41 (Klistorner and Graham, 2001). However, given that the MAD estimator is less influenced by outlying data points than the coefficient of variation, estimates of variability from this statistic tend to be lower than from the coefficient of variation, so a direct comparison between these two statistics cannot be made. For comparison, the co-efficient of variation of P1 amplitude in the TD group, based on the SD of these data was 0.58, i.e., higher than that reported by Klistorner and Graham (2001) – possibly reflecting developmental change in amplitude variability.
Conclusion
In conclusion, these data illustrate that analysis of single-trial EEG activity is less variable when the data are spatially filtered, either using ICA or CSD, prior to analysis. Therefore, when comparing data between different groups of participants, more accurate results are likely to be obtained if indices obtained from ICs of CSD interpolated EEG rather than raw channel indices are compared. Further to this, EEG variability across single-trials was significantly greater in the participants with ASD as compared to the TD control group. These data provide the first empirical demonstration of increased neural noise in those with ASD. Increased variability in neural activity may result in a number of negative consequences for individuals with ASD and may contribute to the substantial inter-individual variability that characterizes the literature on cognitive function in those with ASD.
Conflict of Interest Statement
The author declares that the research was conducted in the absence of any commercial or financial relationships that could be construed as a potential conflict of interest.
Acknowledgments
I would like to thank all the participants and their families, who generously gave up their time to participate in this research. I am indebted to Scott Makeig, Arnaud Delorme, and others at SCCN for numerous, and invaluable, discussions about ICA. I would also like to thank Alison Scope for collecting the data presented here, Suzanna Laycock for stimulating discussions about neural noise and developmental disorders, and Mike X. Cohen for introducing me to CSD interpolation.
Footnotes
- ^Note, that an index of earlier cortical response to visual stimuli is expressed by the C1 component, which peaks at around 100 ms and is generated in the striate cortex (Di Russo et al., 2001). However, C1 was not analyzed here as not all participants with ASD demonstrated a clear C1 deflection.
- ^In the rest of this manuscript this sample will be collectively referred to as “participants with ASD.”
- ^Although, as discussed in the introduction, the late P1 complex analyzed here most likely reflects activity in extra-striate cortex rather than the primary visual cortex.
References
Ales, J. M., Yates, J. L., and Norcia, A. M. (2010). V1 is not uniquely identified by polarity reversals of responses to upper and lower visual field stimuli. Neuroimage 52, 1401–1409.
Allen, G., and Courchesne, E. (2001). Attention function and dysfunction in autism. Front. Biosci. 16, D105–D119.
APA. (1994). Diagnostic and Statistical Manual of Mental Disorders. Washington, DC: American Psychiatric Press.
Arieli, A., Sterkin, A., Grinvald, A., and Aertsen, A. (1996). Dynamics of ongoing activity: explanation of the large variability in evoked cortical responses. Science 273, 1868–1871.
Baird, G., Simonoff, E., Pickles, A., Chandler, S., Loucas, T., Meldrum, D., and Charman, T. (2006). Prevalence of disorders of the autism spectrum in a population cohort of children in South Thames: the special needs and autism project (SNAP). Lancet 368, 210–215.
Baron-Cohen, S., and Belmonte, M. K. (2005). Autism: a window onto the development of the social and the analytic brain. Annu. Rev. Neurosci. 28, 109–126.
Bellgrove, M. A., Hester, R., and Garavan, H. (2004). The functional neuroanatomical correlates of response variability: evidence from a response inhibition task. Neuropsychologia 42, 1910–1916.
Belmonte, M. K., Cook, E. H., Anderson, G. M., Rubenstein, J. L., Greenough, W. T, Beckel-Mitchener, A., Courchesne, E., Boulanger, L. M., Powell, S. B., Levitt, P. R., Perry, E. K., Jiang, Y. H., DeLorey, T. M., and Tierney, E. (2004). Autism as a disorder of neural information processing: directions for research and targets for therapy. Mol. Psychiatry 9, 646–663.
Bennetto, L., Pennington, B. F., and Rogers, S. J. (1996). Intact and impaired memory functions in autism. Child Dev. 67, 1816–1835.
Bertone, A., Mottron, L., Jelenic, P., and Faubert, J. (2005). Enhanced and diminished visuo-spatial information processing in autism depends on stimulus complexity. Brain 128, 2430–2441.
Berument, S. K., Rutter, M., Lord, C., Pickles, A., and Bailey, A. (1999). Autism screening questionnaire: diagnostic validity. Br. J. Psychiatry 175, 444–451.
Bolton, M. M., and Eroglu, C. (2009). Look who is weaving the neural web: glial control of synapse formation. Curr. Opin. Neurobiol. 19, 491–497.
Brock, J., Brown, C., Boucher, J., and Rippon, G. (2002). The temporal binding deficit hypothesis of autism. Dev. Psychopathol. 14, 209–224.
Casanova, M. F., Buxhoeveden, D. P., Switala, A. E., and Roy, E. (2002). Minicolumnar pathology in autism. Neurology 58, 428–432.
Castellanos, F. X., Sonuga-Barke, E. J. S., Scheres, A., Di Martino, A., Hyde, C., and Walters, J. R. (2005). Varieties of attention-deficit/hyperactivity disorder-related intra-individual variability. Biol. Psychiatry 57, 1416–1423.
Croner, L. J., Purpura, K., and Kaplan, E. (1993). Response variability in retinal ganglion-cells of primates. Proc. Natl. Acad. Sci. U.S.A. 90, 8128–8130.
Debener, S., Makeig, S., Delorme, A., and Engel, A. K. (2005). What is novel in the novelty oddball paradigm? Functional significance of the novelty P3 event-related potential as revealed by independent component analysis. Cogn. Brain Res. 22, 309–321.
Delorme, A., and Makeig, S. (2004). EEGLAB: an open source toolbox for analysis of single-trial EEG dynamics including independent component analysis. J. Neurosci. Methods 134, 9–21.
Deutsch, K. M., and Newell, K. M. (2005). Noise, variability, and the development of children’s perceptual-motor skills. Dev. Rev. 25, 155–180.
Di Russo, F., Martinez, A., Sereno, M. I., Pitzalis, S., and Hillyard, S. A. (2001). Cortical sources of the early components of the visual evoked potential. Hum. Brain Mapp. 15, 95–111.
Eichele, H., Juvodden, H. T., Ullsperger, M., and Eichele, T. (2010). Mal-adaptation of event-related EEG responses preceding performance errors. Front. Hum. Neurosci. 4:65. doi: 10.3389/fnhum.2010.00065
Foxe, J. J., and Simpson, G. V. (2002). Flow of activation from V1 to frontal cortex in humans. Exp. Brain Res. 142, 139–150.
Geurts, H. M., Grasman, R. P. P. P., Verté, S., Oosterlaan, J., Roeyers, H., van Kammen, S. M., and Sergeant, J. A.. (2008). Intra-individual variability in ADHD, autism spectrum disorders and Tourette’s syndrome. Neuropsychologia 46, 3030–3041.
Happé, F., Ronald, A., and Plomin, R. (2006). Time to give up on a single explanation for autism. Nat. Neurosci. 9, 1218–1220.
Hill, E. L. (2004). Evaluating the theory of executive dysfunction in autism. Dev. Rev. 24, 189–233.
Hoeksma, M. R., Kemner, C., Verbaten, M. N., and van Engeland, H. (2004). Processing capacity in children and adolescents with pervasive developmental disorders. J. Autism Dev. Disord. 34, 341–354.
Isler, J. R., Martien, K. M., Grieve, P. G., Stark, R. I., and Herbert, M. R. (2010). Reduced functional connectivity in visual evoked potentials in children with autism spectrum disorder. Clin. Neurophysiol. 121, 2035–2043.
Jaskowski, P., and Verleger, R. (1999). Amplitudes and latencies of single-trial ERPs estimated by a maximum-likelihood method. IEEE Trans. Biomed. Eng. 46, 987–993.
Jemel, B., Mimeault, D., Saint-Amour, D., Hosein, A., and Mottron, L. (2010). VEP contrast sensitivity responses reveal reduced functional segregation of mid and high filters of visual channels in autism. J. Vis. 10, 13.
Jung, T. P., Makeig, S., Westerfield, M., Townsend, J., Courchesne, E., and Sejnowski, T. J. (2001). Analysis and visualization of single-trial event-related potentials. Hum. Brain Mapp. 14, 166–185.
Kayser, J. (2009). Current Source Density (CSD) Interpolation Using Spherical Splines – CSD Toolbox (version 1.1). Available at: http://psychophysiology.cpmc.columbia.edu/Software/CSDtoolbox
Kayser, J. R., and Tenke, C. E. (2006). Principal components analysis of Laplacian waveforms as a generic method for identifying ERP generator patterns: I. Evaluation with auditory oddball tasks. Clin. Neurophysiol. 117, 348–368.
Klistorner, A. I., and Graham, S. L. (2001). Electroencephalogram-based scaling of multifocal visual evoked potentials: effect on intersubject amplitude variability. Invest. Ophthalmol. Vis. Sci. 42, 2145–2152.
Makeig, S., Debener, S., Onton, J., and Delorme, A. (2004). Mining event-related brain dynamics. Trends Cogn. Sci. 8, 204–210.
Makeig, S., Jung, T.-P., Bell, A. J., Ghahremani, D., and Sejnowski, T. J. (1997). Blind separation of auditory event-related brain responses into independent components. Proc. Natl. Acad. Sci. U.S.A. 94, 10979–10984.
Maurer, U., Rossion, B., and McCandliss, B. D. (2008). Category specificity in early perception: face and word N170 responses differ in both lateralization and habituation properties. Front. Hum. Neurosci. 2:18. doi: 10.3389/neuro.09.018.2008
McAnally, K. I., Stuart, G., and Castles, A. (2001). Can contrast sensitivity functions in dyslexics be explained by inattention rather than a magnocellular deficit? Vision Res. 24, 3205–3211.
Milne, E., Scope, A., Pascalis, O., Buckley, D., and Makeig, S. (2009). Independent component analysis reveals atypical EEG activity during visual perception in individuals with autism. Biol. Psychiatry 65, 22–30.
Milne, E., Swettenham, J., Hansen, P., Campbell, R., Jeffries, H., and Plaisted, K. (2002). High motion coherence thresholds in children with autism. J. Child Psychol. Psychiatry 43, 255–263.
Mottron, L., Dawson, M., Soulieres, I., Hubert, B., and Burack, J. A. (2006). Enhanced perceptual functioning in autism: an update and eight principles of autistic perception. J. Autism Dev. Disord. 36, 27–43.
Nordahl, C. W., Dierker, D., Mostafavi, I., Schumann, C. M., Rivera, S. M., Amaral, D. G., and Van Essen, D. C. (2007). Cortical folding abnormalities in autism revealed by surface-based morphometry. J. Neurosci. 27, 11725–11735.
Nunez, P. L., and Srinivasan, R. (2006). Electric Fields of the Brain. Oxford: Oxford University Press.
Onton, J., Delorme, A., and Makeig, S. (2005). Frontal midline EEG dynamics during working memory. Neuroimage 27, 341–356.
Onton, J., Westerfield, M., Townsend, J., and Makeig, S. (2006). Imaging human EEG dynamics using independent component analysis. Neurosci. Biobehav. Rev. 30, 808–822.
Ozonoff, S., Strayer, D. L., McMahon, W. M., and Filloux, F. (1994). Executive function abilities in autism and Tourette syndrome: an information processing approach. J. Child Psychol. Psychiatry 35, 1015–1032.
Pernet, C. R., Chauveau, N., Gaspar, C., and Rousselet, G. A. (2011). Limo EEG: a toolbox for hierarchical linear modeling of electroencephalographic data. Comput. Intell. Neurosci. doi: 10.1155/2011/831409
Perrin, F., Pernier, J., Bertrand, O., and Echallier, J. F. (1989). Spherical splines for scalp potential and current denisty mapping. Electroencephalogr. Clin. Neurosci. 72, 184–187.
Plaisted, K., O’Riordan, M., and Baron-Cohen, S. (1998). Enhanced visual search for a conjunctive target in autism: a research note. J. Child Psychol. Psychiatry 39, 777–783.
Rojas, D. C., Maharajh, K., Teale, P., and Rogers, S. J. (2008). Reduced neural synchronization of gamma-band meg oscillations in first-degree relatives of children with autism. BMC Psychiatry 8, 66. doi: 10.1186/1471-244X-8-66
Rubenstein, J. L. R., and Merzenich, M. M. (2003). Model of autism: increased ratio of excitation/inhibition in key neural systems. Genes Brain Behav. 2, 255–267.
Russell, V. A., Oades, R. D., Tannock, R., Killeen, P. R., Auerbach, J. G., Johansen, E. B., and Sagvolden, T. (2006). Response variability in attention-deficit/hyperactivity disorder: a neuronal and glial energetics hypothesis. Behav. Brain Funct. 2, 30.
Salajegheh, A., Link, A., Elster, C., Burghoff, M., Sander, T., Trahmsb L., and Poeppeland, D. (2004). Systematic latency variation of the auditory evoked m100: from average to single-trial data. Neuroimage 23, 288–295.
Sannita, W. G. (2006). Individual variability, end-point effects and possible biases in electrophysiological research. Clin. Neurophysiol. 117, 2569–2583.
Schopler, E., Reichler, R. J., and Renner, B. R. (1988). The Childhood Autism Rating Scale. Los Angeles: Western Psychological Services.
Sieluzycki, C., Konig, R., Matysiak, A., Kus, R., Ircha, D., and Durka, P. J. (2009). Single-trial evoked brain responses modeled by multivariate matching pursuit. IEEE Trans. Biomed. Eng. 56, 74–82.
Simmons, D. R., Robertson, A. E., McKay, L. S., Toal, E., McAleer, P., and Pollick, F. E. (2009). Vision in autism spectrum disorders. Vision Res. 49, 2705–2739.
Singer, W., Engel, A. K., Kreiter, A. K., Munk, M. H. J., Neuenschwander, S., and Roelfsema, P. R. (1997). Neuronal assemblies: necessity, signature and detectability. Trends Cogn. Sci. 1, 252–260.
Tallon-Baudry, C., and Bertrand, O. (1999). Oscillatory gamma activity in humans and its role in object representation. Trends Cogn. Sci. 3, 151–162.
Tucker, D. (1993). Spatial sampling of head electrical fields: the geodesic sensor net. Electroencephalogr. Clin. Neurophysiol. 87, 154–163.
von Spreckelsen, M., and Bromm, B. (1988). Estimation of single-evoked cerebral potentials by means of parametric modeling and kalman filtering. IEEE Trans. Biomed. Eng. 35, 691–700.
Wechsler, D. (1999). Wechsler Abbreviated Scale of Intelligence. San Antonio, TX: The Psychological Corporation.
Welsh, J. P., Ahn, E. S., and Placantonakis, D. G. (2005). Is autism due to brain desynchronization? Int. J. Dev. Neurosci. 23, 253–263.
Keywords: autism, EEG, variability, perception, noise, synchrony, alpha, phase-locking
Citation: Milne E (2011) Increased intra-participant variability in children with autistic spectrum disorders: evidence from single-trial analysis of evoked EEG. Front. Psychology 2:51. doi: 10.3389/fpsyg.2011.00051
Received: 28 December 2010;
Accepted: 19 March 2011;
Published online: 30 March 2011.
Edited by:
Guillaume A. Rousselet, University of Glasgow, UKReviewed by:
David R. Simmons, University of Glasgow, UKCyril R. Pernet, University of Edinburgh, UK
Copyright: © 2011 Milne. This is an open-access article subject to a non-exclusive license between the authors and Frontiers Media SA, which permits use, distribution and reproduction in other forums, provided the original authors and source are credited and other Frontiers conditions are complied with.
*Correspondence: Elizabeth Milne, Sheffield Autism Research Lab, Department of Psychology, The University of Sheffield, Western Bank, Sheffield, South Yorkshire S10 2TN, UK. e-mail: e.milne@sheffield.ac.uk