- 1 Department of Psychology, University of Potsdam, Potsdam, Germany
- 2 Beijing Key Laboratory of Learning and Cognition and Department of Psychology, Capital Normal University, Beijing, China
- 3 Center for Brain and Cognitive Sciences and Department of Psychology, Peking University, Beijing, China
- 4 Key Laboratory of Machine Perception (Ministry of Education), Peking University, Beijing, China
Linear mixed models (LMMs) provide a still underused methodological perspective on combining experimental and individual-differences research. Here we illustrate this approach with two-rectangle cueing in visual attention (Egly et al., 1994). We replicated previous experimental cue-validity effects relating to a spatial shift of attention within an object (spatial effect), to attention switch between objects (object effect), and to the attraction of attention toward the display centroid (attraction effect), also taking into account the design-inherent imbalance of valid and other trials. We simultaneously estimated variance/covariance components of subject-related random effects for these spatial, object, and attraction effects in addition to their mean reaction times (RTs). The spatial effect showed a strong positive correlation with mean RT and a strong negative correlation with the attraction effect. The analysis of individual differences suggests that slow subjects engage attention more strongly at the cued location than fast subjects. We compare this joint LMM analysis of experimental effects and associated subject-related variances and correlations with two frequently used alternative statistical procedures.
Introduction
Nobody doubts that there are individual differences in effects of experimental manipulations. Can reliable individual differences help us constrain and advance cognitive theories with a distinct experimental psychological flavor? In two citation classics, Cronbach (1957, 1975) assessed options of cooperation between the “experimental and the correlation streams of research,” which have since then been called the “the two sciences of psychology.” In the first article, Cronbach was quite optimistic that there would be convergence with mutual benefits. In his re-assessment 18 years later, optimism had given way to skepticism, in light of little evidence of any significant progress. He thought that the best one can hope for is that the two disciplines delineate largely independent territories within which both of them appear to do quite well – a view recently re-evaluated with a slightly more optimistic outlook for the fields of social (i.e., experimental) and personality (i.e., correlational) psychology (Tracy et al., 2009). Here we propose that linear mixed models (LMMs) offer a new hope for a productive convergence between the two streams of research. We illustrate this approach with data from a classic experiment on the dissociation of spatial and object-based shifts of visual attention.
A New Hope: Convergence with Linear Mixed Models
We re-evaluate Cronbach’s proposal with a classic experiment in visual attention and demonstrate that analyses of experiments with LMMs may forge a promising interface of mutual benefit between the two psychological sciences. In this section we briefly describe three advantages of LMMs that have already led to much acceptance of this approach in psycholinguistic research during the last years (see citations of Baayen et al., 2008). Then, we focus on three additional advantages of LMMs that are of relevance for experimental psychological research in general. In this article, we illustrate these advantages for an experiment in attention research and briefly describe their statistical foundation.
Our presentation of LMMs is necessarily selective. For broader context we refer to Baayen (2008), Faraway (2006), Gelman and Hill (2008), Snijders and Bosker (1999), and Zuur et al. (2009). All these books contain chapters that offer introductions to LMMs that are accessible for experimental psychologists; they also describe generalized LMMs (GLMMs) required for binary responses (e.g., the accuracy) or response variables with a small set of alternatives (e.g., count data). Bates (2010) and Pinheiro and Bates (2000) are fully devoted to LMMs, GLMMs, and also non-LMMs (e.g., for psychometric or dose–response functions) and provide much in-depth mathematical statistics background.
Linear mixed models can substitute for mixed-model analyses of variance (ANOVAs) used in traditional experiments, but for a perfectly balanced design with one random factor (usually subjects), the two analyses yield identical inferential statistics for main effects and interactions (i.e., fixed effects) associated with experimental and quasi-experimental manipulations. LMMs offer much additional information about variance and covariance components associated with random factors of the design, such as subjects or items. The (co-)variance components are estimates of reliable differences between subjects or items, both with respect to the differences in overall mean performance and with respect to the differences in within-subject or within-item (quasi-)experimental effects. We highlight three advantages of LMMs over ANOVAs that have led to their broad acceptance in psycholinguistic research (e.g., Quené and van den Bergh, 2004, 2008; Baayen et al., 2008; Kliegl et al., 2010).
The primary advantage of LMMs over ANOVAs is the option to specify crossed random factors such as subjects and items (e.g., words/sentences in a psycholinguistic experiment). In this context, a single LMM replaces the F1-ANOVA (using subjects as the random factor and averaging over items in the cells of the design) and F2-ANOVA (using items as the random factor and averaging over subjects), following a proposal by Forster and Dickinson (1979; see also Clark, 1973; Raaijmakers et al., 1999). Often a factor is varied within subjects (e.g., a manipulation of low and high frequency words), but must be specified as between items (e.g., each word is either of low or high frequency), leading to differences in statistical power for F1- and F2-ANOVAs and interpretational ambiguity. In contrast, such designs pose no problems for an LMM with crossed random factors. All responses (i.e., number of subjects × number of items) constitute the units of analysis rather than two types of aggregated scores.
A second advantage of LMMs over mixed-model ANOVAs is that they are not restricted to factors with a fixed set of categorical levels (e.g., low, medium, and high frequency words), but that they also allow tests of effects of continuous variables (called covariates; yielding, for example, fixed effects of linear and quadratic trends of printed word frequency) and their interactions with categorical factors – usually with a substantial gain in statistical power. Note that a covariate such as word frequency usually enters the model as a within-subject covariate because each subject is exposed to words varying widely in frequency. Traditionally, tests of within-subject covariate effects have been analyzed with repeated-measures multiple regression analyses (rmMRA; Lorch and Myers, 1990). Simulations in Baayen (2008) show that rmMRA may lead to anti-conservative estimates.
The third advantage of LMMs over mixed-model ANOVAs is that the former suffer less severe loss of statistical power if an experimental design loses balance due to missing data (see Pinheiro and Bates, 2000; Quené and van den Bergh, 2004, 2008 for simulations). Loss of data is often very high and close to inevitable in psycholinguistic eye-movement research where subjects’ blinks or loss of tracking due to instable calibration are largely beyond experimental control. If subjects do not contribute a sufficient number of responses to a design cell, typically all their data are excluded from analysis, compounding the negative effect on statistical power.
Baayen et al. (2008), Kliegl et al. (2010), and Quené and van den Bergh (2008) illustrated these three advantages with concrete examples from psycholinguistic research. With the present research we focus on additional LMM advantages that are of relevance for standard experimental psychological research, that is (a) only subjects are included as a random factor, (b) all the other factors represent genuine experimental manipulations with a small number of discrete levels, and (c) loss of data is minimal (e.g., only a small percentage of incorrect responses is excluded in an analysis of reaction times [RTs]). We describe three advantages with an experiment on cueing of visual attention.
Firstly and most importantly, we use LMMs to estimate not only effects and interactions of experimental manipulations (i.e., fixed effects parameters), but to estimate simultaneously parameters of the variance and covariance components of random effects due to subjects. Random effects are subjects’ deviations from the grand mean RT and subjects’ deviations from the fixed-effect parameters. They are assumed to be independently and normally distributed with a mean of 0. It is important to recognize that these random effects are not parameters of the LMM – only their variances and covariances are. This LMM feature encapsulates the legacy of Cronbach (1957, 1975).
Secondly, LMMs have much more statistical power than ANOVAs in unbalanced designs. Here we are not referring to lack of balance due to missing data, but due to experimental design. Most notable in this respect are experimental manipulations of cue validity in attention research where trials in which a cue validly indicates the location of a target outnumber those with invalid cue conditions by a factor of 3 or 4. Obviously, performance is assessed much more reliably in valid than invalid cue conditions. In an ANOVA this imbalance in design and subsequent difference in reliability is ignored by averaging the response times to a single value per cue condition.
Thirdly, we illustrate how LMM parameters in combination with subjects’ data can be used to generate “predictions” (conditional modes) of random effects for each subject. These predictions are corrected for the unreliability inherent in within-subject estimates of experimental effects. Usually these random effects are treated as nuisance parameters; here they serve as an important heuristic for identifying reliable between-subject variances and covariances that may guide the design of the next experiment.
In the next section, we introduce the theoretical background for the visual-attention experiment; then we provide some mathematical background regarding the LMM used for the analysis of this experiment.
Space-Based, Object-Based, and Attraction-Based Effects of Visual Attention
We carried out a classical experiment of visual attention: the two-rectangle cueing paradigm (Egly et al., 1994). Attentional selection of visual information has been conceptualized as space-based, as object-based, and as being due to an attraction back to the original fixation location. Our experiment replicates these effects with RT differences between four experimental conditions of the two-rectangle cueing paradigm.
Space-based selection assumes that the focus of attention is moved in analogy to either a spotlight (Posner, 1980) or a zoom lens (Eriksen and Yeh, 1985; Eriksen and St. James, 1986) to a particular location in the visual space. Object-based attention assumes that, once an object is selected, a spatial movement of attention within the object is much faster than one of the same distance between objects. Support of such object-based attentional selection comes from a variety of paradigms, among which the two-rectangle cuing technique (Egly et al., 1994) stands at a prominent position. Egly et al. (1994) presented two parallel rectangles to participants and asked them to detect as quickly as possible a target flash, which appeared at one end of a rectangle (see Figure 1 for an illustration). Before the target was presented, however, there was an informative cue presented at one of the four ends of the rectangles. Most of the times, the cue validly indicated the location of the subsequent target. Some of the times, the cue was presented at the opposite end of the same rectangle where the target appeared, or at the equidistant end of the alternative rectangle. Here we extend the paradigm by including also targets at the fourth location, diagonally across from the cue.
In this new format the two-rectangle cueing paradigm yields three experimental effects based on differences in RTs. First, RTs to the target are shorter at the validly cued location than when the target is presented at the opposite end of the same rectangle. We call this RT difference the spatial effect. The spatial effect is most closely related to the cue-validity effect, that is, the classic indicator of spatial attentional selection, because it measures the time needed for a pure shift of spatial attention (albeit in our case within an object). Second, RTs to targets at the invalid location within the cued object are shorter than the RTs to targets in the uncued equidistant object. We refer to this same-object advantage, or the different-object disadvantage, as the object effect. It suggests that the allocation of attention is not only constrained by the relative spatial positions of the cue and the target, but also by the perceived object structure of the display. Objects in the visual field guide the definition and selection of a region of space (Arrington et al., 2000).
A new issue in our experiment is the third effect based on the difference in RT between the two targets on the invalidly cued object. Vecera (1994) reported that responses to the uncued target at the alternative rectangle are faster when this rectangle is closer to the cued rectangle than when this rectangle is further away from the cued rectangle. Thus, the object effect can be influenced by the manipulation of the attention shift over space. In other words, the object effect is reduced when the spatial distance is shortened. From this perspective, given a longer physical distance, RTs to the target diagonally across from the cue location should be slower than those to targets horizontally or vertically across from the cue location.
There is, however, an alternative perspective on this effect. Zhou et al. (2006), using a measure of the effect of inhibition of return in non-predictive spatial cueing (Posner and Cohen, 1984; Klein, 2000), demonstrated that the centroid of a group of spatially laid-out objects has a special ability in attracting attention, producing small but significant effect in facilitating target detection (see also Kravitz and Behrmann, 2008, for the crucial role of the center of the object).
In the two-rectangle cueing paradigm, the initial fixation position is at the centroid. Attention tends to return to the fixation location after it is released from capture by a salient peripheral stimulus and there is evidence that this could accelerate movement of attention along this path (Pratt et al., 1999). A target diagonally across at the other end of the uncued rectangle forces disengagement from the cued location and attentional movement to the target will pass through the fixation location. Thus, if centroid and fixation location, which are identical in this experiment, do have a special ability to attract attention and accelerate the attentional movement along the path, RTs to the target at the diagonal location should be faster (or at least not slower) than responses to the target at the other end of the same uncued rectangle. We shall refer to this combined effect of initial fixation location and centroid as the attraction effect.
Individual Differences in Mean RT and Experimental Effects
Intercept-effect correlation
The main purpose of this article is to demonstrate that with an LMM we can estimate correlations between spatial, object, and attraction effects simultaneously with the genuine experimental effects. We expected one such correlation from prior research. Lamy and Egeth (2002) manipulated the SOA between cue and target and found that the spatial effect increased with an increase of SOA from 100 to 300 ms whereas the object effect did not vary as a function of SOA. If we assume that individual differences in mean RT act like quasi-experimental manipulations of SOA, we predict a positive correlation between mean RT and spatial effect but no (or a much weaker) correlation between mean RT and object effect.
Effect–effect-correlation
We also predict a negative correlation between spatial and attraction effect on the assumption that subjects differ in the degree to which they can disengage attention from the cued location, implying that attention gravitates back to the center of fixation more quickly for the “disengagers.” If this “gravitational pull” facilitates a move of attention quickly across the diagonal (centroid), “disengagers” should exhibit a smaller spatial effect.
Operationalization of Effects
We used an experimental design similar to Egly et al. (1994), except that the target could also appear at the location diagonal to the cued location. According to its relative position to the cue, the target could appear at one of the four locations. If the target was presented at the cued location, this trial was “valid.” If the target was presented at the other end of the cued rectangle, this trial was called same-object condition (SOD; “same object, different location”). If the target was presented at one end of the uncued rectangle and this end was equidistant as the SOD location from the cued location, then this trial belonged to the different-object condition (DOS; “different object, same distance”). Finally, if the target was presented at the other end of the uncued rectangle, this trial was called diagonally different-object condition, or for short, diagonal condition (DOD; “different object, diagonal location”). The four experimental conditions yield three contrasts (in addition to an estimate of the grand mean RT based on the four condition means):



We specify these three contrasts as planned comparisons. In addition, we are interested in the correlation of these effects. As described above, on the admittedly speculative assumption that slow RTs translate into the equivalent of a long SOA, spatial effects should be associated with long mean RTs. Assuming individual differences in the degree to which attention gravitates back to the display centroid, we expect a negative correlation between the spatial and the attraction effects.
Mathematical Representation of LMM
Linear mixed models extend the linear model with the inclusion of random effects, in our case due to differences between subjects. Following Pinheiro and Bates (2000), we use Laird and Ware’s (1982) formulation that expresses the ni-dimensional response vector yi for the ith of M subjects as:

where β is the p-dimensional vector of fixed effect parameters, bi is the q-dimensional vector of random effects assumed to be normally distributed with a mean of 0 and a variance–covariance matrix Ψ, and εi is the ni-dimensional within-subject error vector also conforming to a normal distribution. The random effects bi and the within-subject errors εi are assumed to be independent for different subjects and to be independent of each other for the same subject.
Xi with dimensions ni × p is the familiar design matrix of the general linear model; Xiβ is the overall or fixed component of the model. Zi with dimensions ni × q is the design matrix for subject i; Zibi represents the random effects due to subject i. Thus, the columns of Z map correspond to the random factor of the experimental design. In a model including only a random intercept, there is one column per subject; in a model including a random intercept and random slopes, each random slope adds another column for each subject. Overall, an LMM contains q × M random effects.
Counter to their frequent treatment as mere nuisance parameters, the vector of random effects bi and its associated variance–covariance matrix Ψ are the focus of the present article. The elements of Ψ are the random-effects parameters of the LMM; they are of central concern for the attempt to link experimental and individual-differences research. The covariance estimates in Ψ imply correlations between the random effects within each subject. It is easy to show that they have an effect on the structure of the covariance matrix Vi of the response vector yi. Specifically,

Thus, random effects will induce a correlation structure between responses of a given subject. A pure random-intercept model with subjects as the random factor yields estimates of the between-subject variance Ψ2 and of the within-subject (residual) variance σ2; the intraclass coefficient Ψ2/(Ψ2 + σ2) represents the correlation between values of two randomly drawn responses in the same, randomly drawn subject (Snijders and Bosker, 1999).
Application to Visual-Attention Experiment
For the visual-attention experiment we estimate four fixed-effect parameters (p = 4; i.e., intercept and three effects from four experimental conditions). For each of these four parameters we assume reliable differences between the subjects (q = 4; M = 61; i.e., 4 × 61 = 244 random effects). The random effects are parameterized with ten variance/covariance components, that is, with four variances – between-subject variability of mean RT (i.e., random intercept) and between-subject variability of three effects (i.e., random slopes) – and with six correlations of the subject-specific differences in mean RT and three experimental effects. Thus, we estimate a total of 14 model parameters plus the variance of the residual error for the full LMM. Note that the number of parameters grows quadratically with the number of random effects if the full variance–covariance matrix Ψ is estimated. Frequently, one encounters practical limits, primarily related to the amount of information that can be extracted reliably from the data of a psychological experiment. Therefore, the correlation parameters are often forced to 0 or only a theoretically motivated subset of fixed effects β is parameterized in the variance–covariance matrix Ψ.
For tests of hypotheses relating to individual differences in experimental effects there are at least three procedures. In the first procedure, groups are defined post hoc on median splits on one of the effects or on mean RT; this group factor is included as a between-subject factor in the ANOVA. Then, predictions of correlations map onto group × effect or effect × effect interactions. For example, a positive correlation between mean RT and spatial effect may correspond to a larger post hoc group difference on the SOD than the VALID cue condition. The problem with this procedure is that it does not use information about individual differences within each of the post hoc groups and typically the dependent variable (i.e., RT) is used to define an independent variable (i.e., it requires a post hoc specification of an experimental design factor).
In the second strategy, mean RTs and experimental effects are estimated separately for each subject, for example, with ordinary least-squares regression (i.e., a within-subject analysis of the experimental contrasts). Subsequent correlations between these regression coefficients represent the desired effect correlations. The problem with this procedure is that per-subject regressions accumulate a considerable degree of overfitting (Baayen, 2008). It is also well known, of course, that such within-subject difference scores are notoriously unreliable. With few exceptions, the low reliability of difference scores derived from experimental conditions may have greatly limited the use of experimental effects in individual-differences research, for example their adoption for diagnostic purposes.
There is a third procedure that optimally uses all information and therefore affords the best statistical inferences about experimental effects and individual-difference predictions: LMM. Here the estimation of experimental effects and the estimation of their correlations are carried out simultaneously as described above. Rather than computing the mean RT and the three difference scores for each subject separately, the variances and covariances (correlations) are estimated along with the respective fixed-effect parameters. The LMM estimates are statistically superior to within-subject based estimates because they are corrected for the inherent unreliability of difference scores (e.g., due to scores from subjects with extreme slow or fast RT and, consequently, with much variance).
When model estimates are used to generate prediction intervals for subjects’ mean RT and the three experimental effects, the estimates of extreme scores (i.e., scores based on highly variable item RTs) are “shrunk” more strongly toward the estimate of the population effect than those from the normal range and with low variability. It is said that the model “borrows strength” from the population estimate for the prediction of unreliable scores (Gelman and Hill, 2008; Bates, 2010). These so-called best linear unbiased predictions (BLUPs; Henderson, 1953) of the random effects are more appropriately called the conditional modes of the distribution of the random effects (i.e., the point of maximum density), given the observed data and evaluated at the parameter estimates. In an LMM the conditional modes are also the “conditional means,” but this term is ambiguous for other reasons (Bates, 2010). Therefore, we adhere to Bates’s terminological recommendation. We report inferential statistics for the LMM, but as an illustration compare the results with those from a traditional within-subject analysis. We also show that effect correlations map onto post hoc group × effect interactions.
Materials and Methods
Subjects
Sixty-one right-handed subjects were tested for this experiment after giving their informed consent. They were undergraduate students from Peking University and were paid for their participation. All of the participants had normal or corrected-to-normal vision.
Stimuli
The two rectangles were presented either horizontally or vertically. Each rectangle subtended 0.9° of visual angle in width and 8° in length. The two rectangles were symmetrically presented in parallel, with the middle point of the rectangle 4° from fixation. Thus the distance between the cued location and SOD location and the distance between the cued location and DOS location was equal, and the cue (or the target) was 5.7° away from fixation. The fixation point was a plus sign (+), which subtended 0.1° × 0.1° of visual angle. The distance between the center of the screen (i.e., the fixation sign) and eyes was kept at 65 cm.
There were 600 trials in total, with 480 critical trials and 120 catch trials. Among the critical trials, 336 (70%) were valid trials, with the target appearing at the cued location. The remaining invalid trials were divided equally for the SOD, DOS, and DOD conditions, with 48 (10%) per condition. Half of the trials in each condition were with horizontally placed parallel rectangles and half with vertically presented rectangles.
Procedure
Presentation of stimuli and recording of response times and error rates were controlled by Presentation software (http://nbs.neuro-bs.com/). Stimuli were presented in dim white on a dark gray background. Each trial began with the presentation of a fixation sign at the center of the screen and the two parallel rectangles appeared for 1000 ms (see Figure 1). Then the outlines of one end of a rectangle thickened and changed from gray to white for 100 ms. The thickening and brightening cued the likely location of the subsequent target. After the presentation of the cue, the cued position returned to its original gray color, and the fixation display was presented for another 200 ms. A target, at one end of a rectangle, was then presented until the participant made the detection response. Thus the SOA between the cue and the target was 300 ms. The target was a filled square presented within one end of a rectangle. The fixation sign and the outlines of the rectangles were all light gray whereas the cue and the target were white. In catch trials, the target was not presented after the presentation of the cue. The fixation sign was presented throughout a trial and the participant was asked to fixate on it all the time before a response was made. Participants were asked to press the left button of the computer mouse as accurately and as quickly as possible once they saw the target appeared at any end of the two rectangles and to withhold response on the occasional catch trials in which no target was presented.
Each participant began with a practice session of 60 trials. The formal test was divided into three blocks, with a break of about 2 min between blocks. Each block comprised 200 trials, with different types of rectangles, cue locations, target locations, and catch trials equally distributed over the three blocks. Unlike Egly et al. (1994), trials with horizontal and vertical rectangles were randomly mixed over trials, rather than blocked.
Linear Mixed Modeling with lmer
We used the lmer program of the lme4 package (Bates and Maechler, 2010) for estimating fixed and random coefficients. This package is supplied in the R system for statistical computing (version 2.12.0; R Development Core Team, 2010) under the GNU General Public License (Version 2, June 1991). Scripts and data are available as a supplement and at http://read.psych.uni-potsdam.de/PMR2/. Here we describe model specification and evaluation assuming a data set with 28,710 RTs, collected from 61 subjects.
Reaction times were recorded under four experimental conditions (“VAL,” “SOD,” “DOS,” “DOD”). Spatial, object, and attraction contrasts between neighboring levels of this factor as specified in Eqs 1–3 are assigned to the factor with the contr.sdif() function of the MASS package (Venables and Ripley, 2002). For model comparisons, an alternative specification with three vectors coding the three contrasts c1, c2, and c3
is convenient. We specified three models of increasing complexity with respect to the parameterization of the subject-related variance/covariance matrix. The simple model is a random-intercept model, allowing only for between-subject variance in the mean RT. The lmer specification for this model is:

Reaction time is modeled as a function of fixed spatial (c1
), object (c2
), and attraction effects (c3
). In addition, the (1|id
) term requests the variance for the intercept over subjects. LMM estimates of fixed effects correspond to the grand mean RT (based on the four condition means) and to the three planned comparisons for spatial, object, and attraction effects. Effects larger than twice their standard errors are interpreted as significant at the 5% level (i.e., given the large number of subjects and the large number of observations for each subject, the t-statistic [i.e., M/SE] effectively corresponds to the z-statistic). As the contrasts are not orthogonal, an adjustment of the p-value (e.g., Bonferroni) may be advised (i.e., two-tailed t = 2.4).
The second model is a random-intercept-and-slopes model; it includes also terms for estimating variance components for each of the three contrasts:

Listing the terms separately specifies them as independent of each other (i.e., with zero covariance); the “0” in each contrast term suppresses the default estimation of the intercept and its covariance with the contrast. We will also estimate the significance of each variance component by checking the decrease in goodness of fit due to its exclusion from model m1.
The final model requests a fully parameterized variance–covariance matrix for the subject factor id
. This model estimates parameters for the six covariances (correlations) for the four variance components (i.e., in addition to the four variance components of model m2
).

The models were fit by restricted maximum likelihood (REML). As the models are nested and differ only in the random effects part, the REML statistic also serves as the basis for assessing their relative goodness of fit. For assessment of relative differences in goodness of fit, the lmer program provides the Akaike Information Criterion (AIC; decreases with goodness of fit), the Bayesian Information Criterion (BIC; decreases with goodness of fit), the log-likelihood (logLik; increases with goodness of fit), and, in the case of model comparisons, the χ2-distributed likelihood ratio and its associated p-value. The AIC (= −2 logLik + 2 nparam) and BIC (= −2 logLik + nparamlog Nobs) values correct the log-likelihood statistic for the number of estimated parameters and, in the case of BIC, also for the number of observations. That is, we use them as a guide against overfitting during the process of model selection. Nested models can be compared in R with the command anova (m0, m1, m2)
. A few additional specific tests will be described as part of the Results.
Results
Description of Raw Data
Incorrect responses and responses faster than 150 ms (anticipations) were excluded from the following analysis; they amounted to 570 of 29280 trials (2%). Ignoring the subject factor, means (standard deviations, number of trials) for the correctly answered items were 358 ms (SD = 83 ms, N = 20141), 391 ms (SD = 93 ms, N = 2863), 405 ms (SD = 93 ms, N = 2843), and 402 ms (SD = 95 ms, N = 2863) for VALID, SOD, DOS, and DOD conditions, respectively. This corresponds to a spatial effect of 33 ms, an object effect of 14 ms, and an attraction effect of 3 ms (i.e., unweighted means).
LMM Fixed-Effects Parameters
The LMM fixed-effect parameter statistics are taken from model m2 (see Eq. 8) with the fully parameterized variance–covariance matrix (see below for justification). Two of the three contrasts were significant and in the expected direction: (1) RTs were shorter for valid than invalid cues in the same object [spatial effect: M = 34 ms; SE = 3.3 ms; t = 10.2]. (2) RTs were shorter for invalidly cued targets in the same rectangle than for targets in the alternative rectangle of the equivalent distance [object effect: M = 14 ms; SE = 2.3 ms; t = 6.0]. (3) Detection of the target at the location diagonal to the cue was numerically faster (3 ms) than detection of the alternative target in the same rectangle cue location [attraction effect: 3 ms; SE = 2.2 ms; t = 1.2]. The statistics are also listed in the left part of Table 1. The effect magnitudes are in good agreement with the unweighted effects described above.
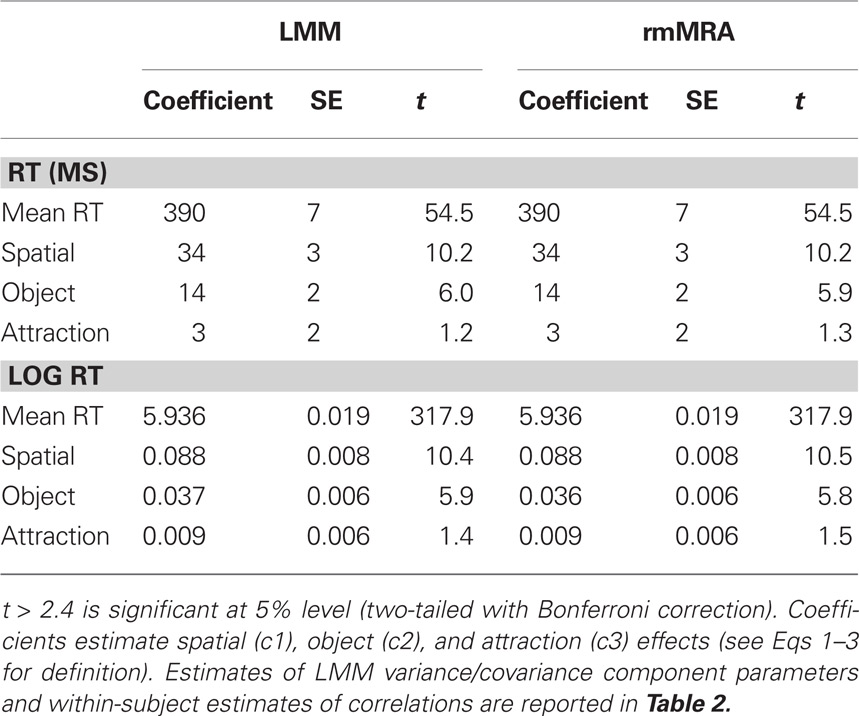
Table 1. Fixed effects estimated with fully parameterized linear mixed model (LMM) and with repeated-measures multiple regression analysis (rmMRA) for RT (top) and log RT (bottom).
The attraction effect was not significant but given that the target was as far from the cue location than the other two invalidly cued targets and given that there were intervening stimuli between the cue and the target (e.g., lines for object boundaries; see Cave and Bichot, 1999), a net advantage of the diagonally opposite invalid cue may be inferred as due to the compensating influence of the attraction effect. As we show below, for present purposes the significance of the associated variance component and the correlation with the spatial effect is more important than the significance of the fixed effect. Thus, the absence of a significant fixed effect does not necessarily indicate the absence of reliable information associated with this manipulation.
Analyses of LMM residuals suggested that log-transformed RTs were in better agreement with distributional assumptions of the model. The violations, however, were mild and the LMM with log-transformed RTs led to the same conclusions as the LMM with untransformed RTs (see bottom left of Table 1). There were, however, some numeric differences for estimates of variance/covariance components1.
Repeated-Measures Multiple Regression Analysis
Analyses of variance-equivalent planned comparisons for the assessment of within-subject spatial, object, and attraction effects are achieved with a rmMRA using the contrast predictors c1, c2, and c3
. For this analysis, coefficients were first estimated for each subject independently and then means and standard errors computed on the basis of the 61 regression coefficients. Results are shown in the right part of Table 1. The estimates are virtually identical to those from the LMM, both for RTs and log-transformed RTs. Thus, for a large number of subjects and observations per subject, LMMs clearly do not lead to different results as far as fixed-parameter estimates are concerned.
Estimates of Variance/Covariance Parameters
Estimates of variance/covariance parameters are reported as standard deviations (i.e., the square root of the estimate of the variance) and as correlations (see top left panel of Table 2; corresponding estimates for log-transformed RTs are listed in the bottom panels). The main result of this experiment is a strikingly strong negative correlation between the spatial and attraction effects (−0.85), as predicted by the assumptions that subjects differ in the degree to which their attention gravitates back to the initial fixation location and that fast moves across the diagonal (i.e., large attraction effects) go hand in hand with small spatial effects. Moreover, consistent with the assumption that individual differences in RT represent a quasi-experimental SOA manipulation, the spatial effect correlated strongly positively with the mean RT (+0.60). These strong correlations are complemented by a positive correlation between attraction and object effects (+0.38), possibly because both effects involve an object switch. Finally, the correlation between spatial and object effect is very close to 0.
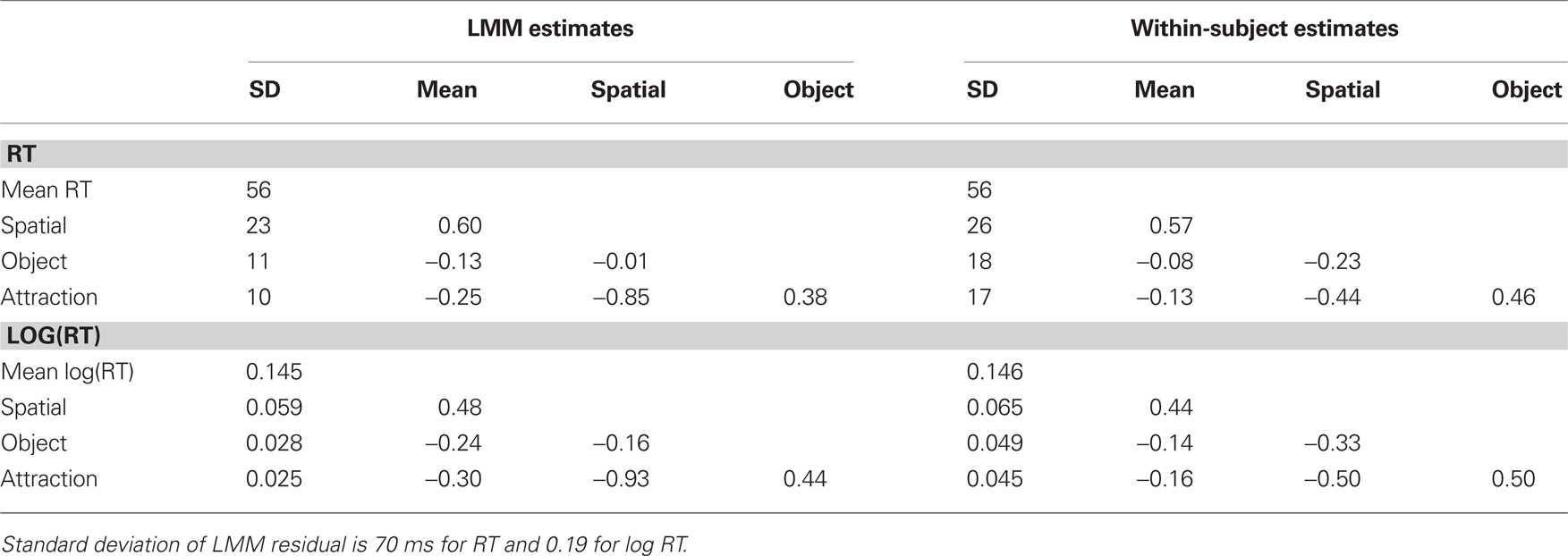
Table 2. Standard deviation/correlation parameter estimates from LMM (left) and corresponding estimates from within-subject analysis (right) for RT (top) and log RT (bottom).
As a statistical test of the significance of variance/covariance components, we started with a model containing only a parameter for the variance of mean RTs between subjects. The logLikelihood for this baseline model with six degrees of freedom was −163275. Then, we allowed for differences between subjects in spatial, object, and attraction effects. The change in logLikelihood Δχ2 (3 df) = 700, p < 2.2e − 16 represents a gigantic improvement in goodness of fit. Finally, the change in logLikelihood between this model and the model including six additional covariance components again was statistically significant; Δχ2 (6 df) = 39, p = 6.967e − 07. Thus, there are reliable individual differences associated with the three attention effects and there are also significant correlations between these effects. The logLikelihood of the final model was −162905 with 15 degrees of freedom. AIC and BIC statistics decreased, indicating increases in goodness of fit (AIC: 326561, 325867, 328540; BIC: 326611, 325941, 325964).
Visualization of Effect Predictions for Individual Subjects
On the basis of model estimates (which comprise fixed-effect parameters and parameters of the variance/covariance components for subject-related mean RT and experimental effects, not estimates at the individual level), “predictions” for individual mean RTs and the three effects can be computed. Figures 2 and 3 display these conditional modes for the 61 subjects, sorted by the size of the spatial effect, using the dotplot() and ranef() functions of the lme4 package (Bates, 2010; Bates and Maechler, 2010) and the xyplot() function of the lattice package (Sarkar, 2008). Also included are 95% prediction intervals based on the evaluation of the conditional modes and the conditional variances of the random effects given the observed data. Note that we allowed the x-scale range to vary between panels.
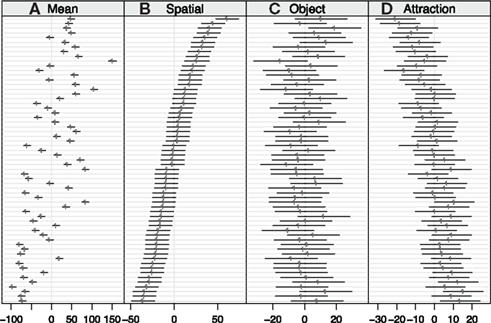
Figure 2. “Caterpillar plots” for conditional modes and 95% prediction intervals of 61 subjects for (A) mean RT, (B) spatial effect, (C) object effect, and (D) attraction effect. Subjects are ordered by spatial effect (after Bates, 2010). Note different x-scales for panels.
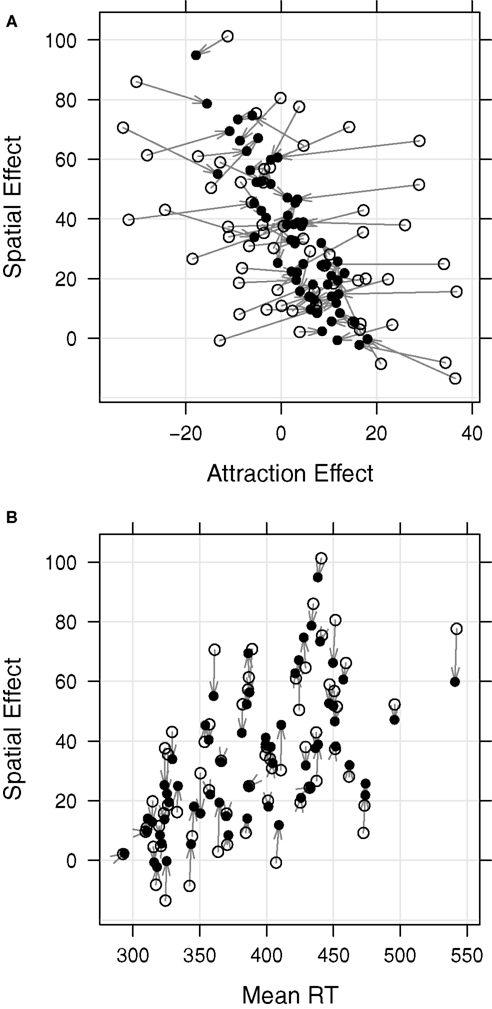
Figure 3. (A) Scatterplot for spatial and attraction effects. Filled symbols show conditional modes of the distributions of random effects, given the observations and evaluated at the parameter estimates. Open symbols show within-subject estimates. Arrows connect the two values for each subject. Shrinkage correction reveals a very strong negative correlation between spatial and attraction effects. LMM correlation estimates and within-subject correlations are reported for all effects in Table 1. (B) Analogous scatterplot for spatial effect over mean RT. Vertical arrows in this panel indicate that there is almost no shrinkage for mean RTs.
There are several important results. First, obviously individual differences are very pronounced for the mean RT and also for the spatial effect. There are subjects whose prediction intervals are completely on opposite sides of the zero line (representing the corresponding fixed-effect estimate.) Also, although not directly visible in the figure due to scale differences, prediction intervals of mean RTs are quite a bit narrower than those of the effects due to the fact that the latter are difference scores. Finally, the “implicit slopes” in Figure 2 for conditional modes across subjects reflect the positive correlation between spatial effect and mean RT.
Second, prediction intervals for the subjects’ object effects overlap very strongly, suggesting that there is not much reliable between-subject variance associated with this effect. Nevertheless, an LMM without variance/covariance components for the object effect fits marginally worse than the complete model, with a Δχ2 (4 df) = 9.5, p = 0.02, for the decrease in loglikelihood. The reported p-value is based on a parametric bootstrap (1000 samples) since the conventional χ2-reference distribution is known to be conservative for tests on variance components and no analytic expressions for the reference distribution are available (Self and Liang, 1987).
Third, although the fixed-effect parameter for attraction was not significant, it still commands reliable individual-difference variance; the drop in goodness of fit of Δχ2 (4 df) = 18.2, p = 0.001, for an LMM without variance/covariance components, is significant for this effect. Moreover, as expected from the estimated LMM correlation, the panels reveal a fairly consistent inverse ordering of subjects relative to their spatial effects. This negative correlation, estimated as −0.85 in the LMM (see Table 1), is illustrated with the scatterplot of filled symbols in the top panel of Figure 3.
Note that the LMM covariance/correlation parameter is not identical to correlations computed directly from the conditional modes. Kliegl et al. (2010) show with simulations that a “correlation” computed from two conditional modes may diverge strongly from the corresponding correlation parameter of the LMM. Conditional modes of different subjects are not independent observations, but values weighted by distance from the population mean. Therefore, statistical inference must refer to estimates of LMM parameters; it is not advised to use conditional modes for further inferential statistics (e.g., to correlate them with each other or with other subject variables such as age or intelligence).
Comparison of LMM-Based Conditional Modes and within-Subject Estimates of Effect Correlations
Figure 3 illustrates the consequences of LMM shrinkage correction for the two theoretically predicted correlations with joint displays of within-subject estimates (open symbols; i.e., experimental effects computed separately for each subject) and LMM predictions (filled symbols; i.e., conditional modes displayed in Figure 2). Arrows connect each within-subject estimates with this subject’s corresponding LMM-based conditional mode.
The top panel displays the correlation between spatial and attraction effect. The within-subject based correlation (open symbols) is only −0.44; the LMM model parameter is −0.85 (filled symbols; see Table 2). The display gives immediate meaning to the term “shrinkage.” Obviously, the more extreme a mean or effect, the stronger the shrinkage toward the estimate of the population mean, representing the correction for unreliability of extreme scores. In this case the same qualitative pattern of correlations is present for LMM parameters and within-subject estimates (see Table 2 for a comparison), but the LMM parameters deliver statistically sound and much stronger results.
The bottom panel displays the correlation for spatial effect and mean RT. In this case, shrinkage correction leads only to a very slight increase in the correlation from 0.57 to 0.60. The vertical orientation of arrows indicates that there basically is no shrinkage correction for mean RT; that is, within-subject based mean RTs basically do not differ from “shrinkage-corrected” mean RTs. This is to be expected given the large number of RTs entering the computation of subject mean RTs. In contrast, the length of the arrows for the spatial effects (i.e., the difference in RTs between SOD and VALID conditions; see Eq. 1) indicates the amount of shrinkage due to the unreliability of this difference score. Thus, the panel illustrates very nicely that mean RTs are more reliable (i.e., less subject to shrinkage correction) than difference scores.
Comparison between LMM Estimates of Effect Correlations and Mixed-Model Anova
Sometimes experimental psychologists assess individual differences in RTs with a post hoc grouping of subjects on the dimension of interest, for example into fast and slow responders when effects of overall speed are under consideration; occasionally, even only extreme groups are included in the analyses. For such an analysis, we must first aggregate items for each subject to four mean RTs – one for each experimental condition. In the present study, this data aggregation effectively “hides” the imbalance in the number of trials per condition that is necessarily due to the cue-validity manipulation. The post hoc grouping based on mean RT is specified as a between-subject factor in the mixed-model ANOVA. Of course, the main effect of group is of no interest, rather the focus is on the interaction between group and experimental manipulations, in our case these are the spatial, object, and attraction contrasts. With this preprocessing in place, there is a straightforward translation of the hypotheses about LMM covariance parameters into hypotheses about mixed-model ANOVA interactions. In the following we illustrate this for the LMM covariance parameters reported in Table 2. We used the ggplot2 package (Wickham, 2009) for the figures.
Speed as post hoc grouping factor
We used a median-split on subjects’ mean RTs to define groups of fast and slow responders. The theoretically interesting question concerns the interactions between speed group and experimental effects. As shown in Figure 4, slow subjects showed larger spatial effects [F(1, 59) = 17.1, MSE = 264, p < 0.01]. The two other interactions were not significant; both F-values < 1. Note that between-subject variance in mean RT was removed for this plot; this, of course, also removes the main effect of speed group. Consequently, it becomes apparent that slow subjects are relatively faster on trials with valid than invalid cue-target relations. Thus, the results are in agreement with the expectation that the spatial effect is modulated by individual differences in RT. Slow subjects engage attention more at the cued location than fast subjects. There is no significant evidence for such a modulation for object and attraction effects. The corresponding LMM correlation parameters were +0.60, −0.13, and −0.25 (see Table 2).
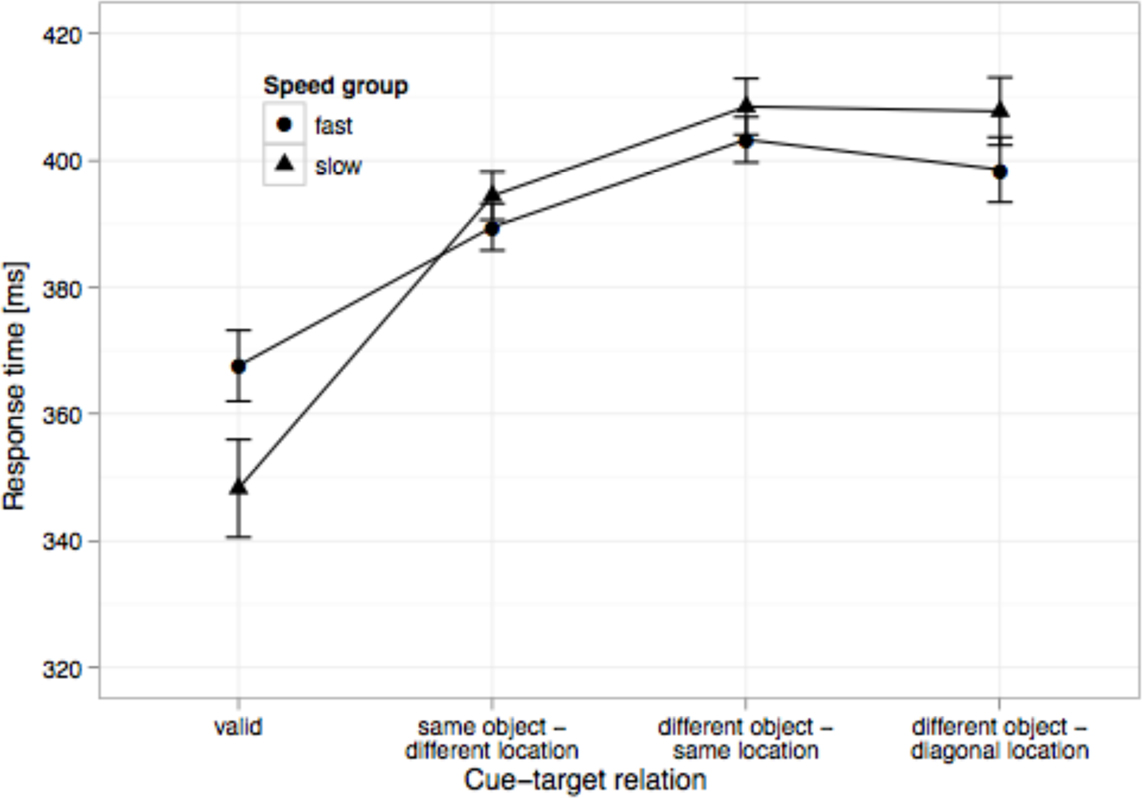
Figure 4. Mean RTs for the four experimental conditions for fast and slow subjects (median split on means of four conditions) after removal of between-subject variance in mean RT. Error bars are ±2 within-subject standard errors of means.
Attraction effect as post hoc grouping factor
We also predicted that subjects with an attraction effect will show a comparatively small spatial effect. For a post hoc ANOVA test of this hypothesis, we classified subjects according to whether or not they showed an attraction effect (DOD < DOS), despite the larger cue-target distance. Indeed, this was the case for 32 of 61 subjects. As shown in Figure 5, subjects with a positive attraction effect exhibited a significantly smaller spatial effect [F(1, 59) = 11.8, MSE = 283, p < 0.01]. Again, the group difference is linked primarily to valid trials: Subjects who presumably engage attention at the cued location and, therefore, respond faster on valid trials do not show an attraction effect. Subjects with an attraction effect also showed a significantly larger object effect [F(1, 59) = 4.8, MSE = 158, p < 0.05]. The positive correlation between attraction and object effects presumably reflects the common process of switching to a different object. The corresponding LMM correlation parameters were −0.85 and +0.38 (see Table 2).
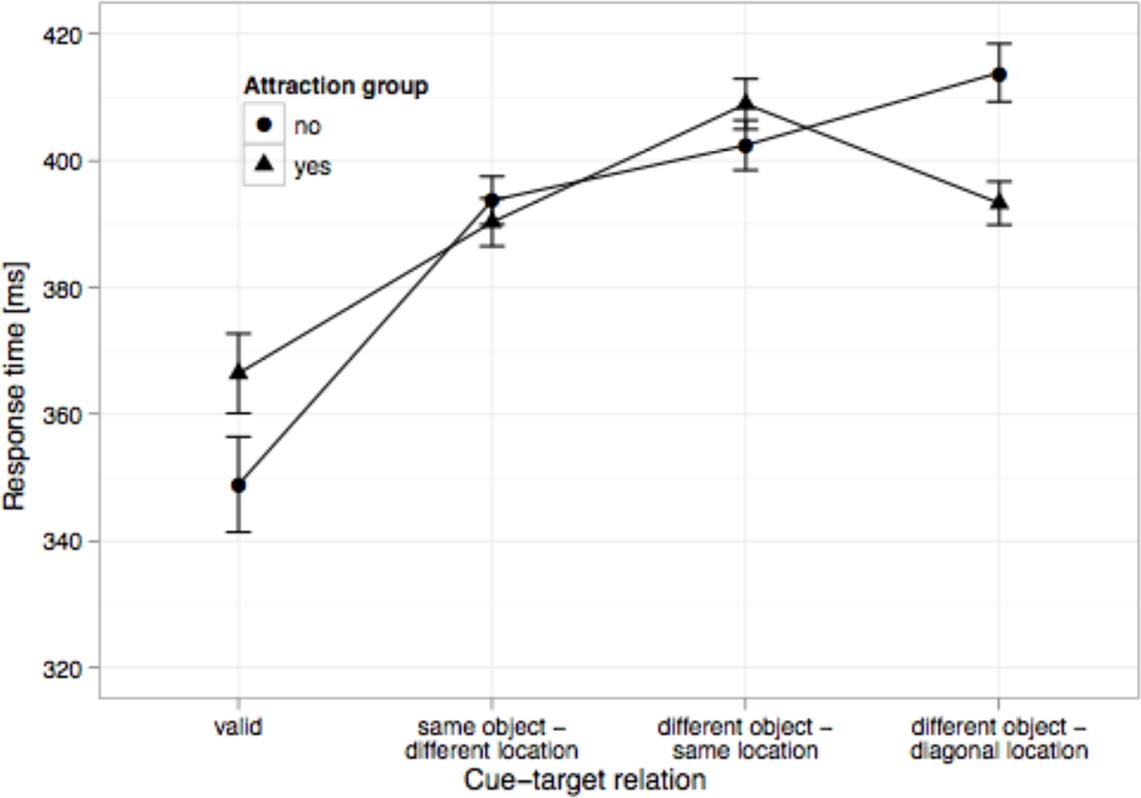
Figure 5. Mean RTs for the four experimental conditions for subjects with and without an attraction effect (DOD < DOS) after removal of between-subject variance in mean RT. Error bars are ±2 within-subject standard errors of means.
Discussion
We used an LMM for the assessment of fixed effects and variance components in a classic experiment in visual attention. The pattern of overall means replicated Egly et al. (1994) and many other studies using the two-rectangle cueing paradigm. A valid spatial cue induced the expected RT benefit at the cued location and RT cost at the uncued location on the same object. This cue-validity effect correlated strongly with the mean RT (+0.60), with the size of the effect becoming larger for slower subjects.
We also observed the well-established specific cost related to switching to an equidistant target on an object different from the cued one. This object effect, in contrast, did not correlate with subjects’ mean RTs. Furthermore, targets diagonally across from the cue were responded to as fast as targets horizontally or vertically across from the cue, indicating that the RT to detect a target on a different object did not depend on the distance from the cue. This lack of an additional RT cost for the diagonal target has been interpreted as an attraction effect exerted by the centroid of the display through which the attention shift from the cue to the diagonal target must pass (Zhou et al., 2006). Finally and importantly, we observed a negative correlation between the attraction effect and the spatial effect. In the next sections, we discuss these effects as well as the advantage of using LMM for analyzing individual differences in experimental effects.
Dissociation of Attention Effects in the Two-Rectangle Cueing Paradigm
The spatial effect, or the cue-validity effect, is consistent with previous studies on spatial cueing (e.g., Posner, 1980; see Cave and Bichot, 1999 for a review). However, unlike in classical spatial cueing, the cue in the present study draws attention not only to the cued location but also to the cued object. This object activation increases its ability to compete with neighboring representations and strengthens the sensory representation of the entire object (Martínez et al., 2006). Alternatively, it prioritizes the attentional deployment to locations within the object with its unattended parts enjoying an attentional advantage over other objects and locations in the scene (Shomstein and Yantis, 2002, 2004). The spatial effect, as operationalized in this study, takes place on top of the activated object representation. Possibly therefore, the spatial cueing effect in our study was augmented by the simultaneous activation of an object – an issue that should be addressed in future studies.
Importantly and in support of the assumption that individual differences in RT may represent a quasi-experimental SOA manipulation (Lamy and Egeth, 2002), we found that the spatial effect increases as the subject’s response speed slows down, because slow subjects respond relatively faster to validly cued targets. Just as a longer (300 ms) SOA appears to tie down attention at the cue more forcefully than a short (100 ms) SOA, making it hard to disengage attention subsequently, the additional processing time of slow subjects may lead to a stronger engagement of attention at the cued location. The negative correlation between spatial effect and attraction effect is also in agreement with this interpretation: Subjects who showed an attraction effect were relatively slow to detect validly cued targets.
There was no significant evidence for a relationship between the object effect and the subjects’ mean RT, nor did the object effect correlate with the spatial effect. This dissociation from the spatial-cueing effect suggests that the object effect may have a different underlying mechanism. We suggest, in line with others, that the spatial effect is related to an individual’s ability to deploy attention to the cued location and shift it to the uncued target location, whereas the object effect is tied to the structural properties of the object or to the general configuration of attentional shifts between objects (e.g., Arrington et al., 2000).
There was no significant attraction effect, but there were statistically reliable differences between subjects in this effect. Obviously, the absence of a significant experimental main effect does not preclude the presence of reliable individual differences in this effect. This occurs when roughly equal numbers of subjects respond reliably in an opposite manner to an experimental manipulation. The functional significance of such a differential response was validated with correlations with the other experimental effects. The attraction effect correlated strongly and inversely with the spatial effect and, to a lesser extent, positively with the object effect (see Table 2; Figure 5). The correlations of the attraction effect with the spatial and object effects suggest that the centroid enjoys a privileged status in attentional selection (Alvarez and Scholl, 2005; Kravitz and Behrmann, 2008) and in facilitating attentional shift (Zhou et al., 2006).
We readily agree that analyses and interpretations of the above effect correlations need to be followed up with experimental manipulations. For example, effects related to shifts of attention within and between hemifields need to be taken into account (e.g., Egly and Homa, 1991). Also explicit manipulations of SOA and the location of the display centroid can provide additional information about the assumption that individual differences in mean RT map onto experimental design parameters. Indeed, we consider it a reasonable general strategy to aim for the conversion of significant variance/covariance components into fixed effects across a series of experiments. We submit that LMMs will serve a very valuable heuristic purpose in such a research program.
Linear Mixed Model vs. Mixed Model Anova
Individual differences figured prominently in the present research. The results presented here were obtained in three ways: (a) with a mixed model ANOVA including a post hoc grouping of subjects based on median splits of mean RT or experimental effects, (b) with correlations based on mean RT and experimental effects estimated separately for each subject (i.e., within-subject analysis), and (c) with an LMM. We briefly discuss why the convergence of results across the three methods cannot in general be taken for granted.
Post hoc grouping of subjects comes at much cost of statistical power and may cause one to overlook effects present in the data. This data-analytic strategy can be employed profitably for exploratory and illustrative purposes. In general, however, it is quite questionable to use a dependent measure to determine the levels of the independent variable.
Correlations of effects computed within subjects are by far the most frequent approach to examine individual differences in experimental contexts. For the present set of data this analysis leads to roughly the same conclusions as the LMM-based correlation estimates, but some of these estimates of correlations are considerably stronger than the corresponding correlations computed from the within-subject analyses (e.g., −0.85 vs. −0.44 for the correlation between spatial and attraction effect; see Table 2). Thus, the shrinkage-related reduction of variance of these two experimental effects (i.e., the removal of unreliable between-subject variance in the effects) unveiled a much stronger correlation than suggested by the correlation of within-subject difference scores.
Linear mixed models offer much potential for determining correlations of experimental effects, but we emphasize that this benefit does not (or does only rarely) come without a “cost” of a larger number of subjects than we routinely recruit for experiments. In our experience so far, typical psycholinguistic and cognitive experiments with RTs as dependent variables require around 60 subjects for obtaining reliable correlations between effects. Obviously, this value may vary greatly between experimental paradigms. Given that experiments tend to be statistically “underpowered” anyway, the perspective that an experiment could deliver information about effect correlations might serve as an independent incentive to increase number of subjects.
We strongly recommend the adoption of LMM for the joint analysis of experimental effects and the correlations between them. There are quite a few additional reasons for this recommendation. Of particular relevance for the spatial cueing paradigm is that LMMs take into account the implied imbalance in number of trials with manipulations of cue validity. The difference in number of valid and invalid trials is reflected in the size of prediction intervals. There probably is no simple solution for realizing an orthogonal manipulation of cue validity, but ignoring the associated differences in statistical power between valid and invalid cue conditions, as it is conventionally done with repeated-measures ANOVA of subject-by-condition cell means, is defensible only for pragmatic reasons.
Finally, the model considered in the present article is structurally identical to the hierarchical linear model (HLM) as it has been used, for example, in social sciences (e.g., Snijders and Bosker, 1999; Raudenbush and Bryk, 2002). The classic HLM example is that students are the units of observation (analogous to RTs in our case) nested within a random group factor “class” (analogous to “subjects” in our case). We consider it a general advantage that LMMs allow for the simultaneous specification of several partially crossed random factors. In psycholinguistic experiments, words or sentences in addition to the conventional subject factor are examples of this kind. In that field LMMs already appear to be replacing traditional F1- and F2-ANOVAs with a single and statistically and computationally more efficient analysis (e.g., check citations in Baayen, et al., 2008). In perception and attention experiments, the inclusion of additional random factors (e.g., colors or shapes of stimuli) would contribute to the generalizability of results. The fact that we do not sample our stimuli more broadly is probably linked to the absence of statistical procedures that efficiently estimate the associated variability. With the recent progress in computational statistics, we should seriously reconsider design options affording much larger generalizability than current practices of experimental psychology.
Conclusion
This article demonstrates the potential of using LMMs for experimental psychologists who commonly use only mixed-model ANOVA. Specifically, we illustrate how different theoretical components in visual attention research can be linked to different components (e.g., mean, variance, and covariance) using the LMM terminology. Most noticeably, significant effect correlations may guide new research. We submit that this approach comes closest to Cronbach’s (1957) vision of the merging of the two sciences of psychology, its “experimental and the correlation streams of research.”
Conflict of Interest Statement
The authors declare that the research was conducted in the absence of any commercial or financial relationships that could be construed as a potential conflict of interest.
Acknowledgments
This study was supported by grants from Natural Science Foundation of China (30770712, 90920012), the Ministry of Science and Technology of China (2010CB833904), and Deutsche Forschungsgemeinschaft (FOR 868 / A2). Data and R scripts for all analyses and figures are available upon request and at the Potsdam Mind Research Repository, http://read.psych.uni-potsdam.de/PMR2/. We thank the reviewers as well as Sven Hohenstein, Jochen Laubrock, and Fabian Scheipl for helpful comments.
Footnote
- ^Kliegl et al. (2010) report LMM analyses for lexical decision RTs from masked repetition priming. In this study, a reciprocal transformation was called for on the basis of distributional analyses.
References
Alvarez, G., and Scholl, B. (2005). How does attention select and track spatially extended objects? New effects of attentional concentration and amplification. J. Exp. Psychol. Gen. 134, 461–476.
Arrington, C. M., Carr, T. H., Mayer, A. R., and Rao, S. M. (2000). Neural mechanisms of visual attention: object-based selection of a region in space. J. Cogn. Neurosci. 12, 106–117.
Baayen, R. H. (2008). Analyzing Linguistic Data: A Practical Introduction to Statistics Using R. Cambridge, MA: Cambridge University Press.
Baayen, R. H., Davidson, D. J., and Bates, D. M. (2008). Mixed-effects modeling with crossed random effects for subjects and items. J. Mem. Lang. 59, 390–412.
Bates, D. M. (2010). lme4: Mixed-Effects Modeling with R. New York: Springer. Prepublication version at: http://lme4.r-forge.r-project.org/book/
Bates, D. M., and Maechler, M. (2010). lme4: Linear Mixed-Effects Models Using S4 Classes. R package version 0.999375-36/r1083. http://R-Forge.R-project.org/projects/lme4
Cave, K., and Bichot, N. P. (1999). Visuospatial attention: beyond a spotlight model. Psychon. Bull. Rev. 6, 204–223.
Clark, H. H. (1973). The language-as-fixed-effect fallacy: a critique of language statistics in psychological research. J. Verbal Learn. Verbal Behav. 12, 335–259.
Cronbach, L. J. (1975). Beyond the two disciplines of scientific psychology. Am. Psychol. 30, 116–127.
Egly, R., Driver, J., and Rafal, R. D. (1994). Shifting visual attention between objects and locations: evidence from normal and parietal lesion subjects. J. Exp. Psychol. Gen. 123, 161–177.
Egly, R., and Homa, E. (1991). Reallocation of attention. J. Exp. Psychol. Hum. Percept. Perform. 17, 142–159.
Eriksen, C. W., and St. James, J. D. (1986). Visual attention within and around the field of focal attention: a zoom lens model. Percept. Psychophys. 40, 225–240.
Eriksen, C. W., and Yeh, Y. Y. (1985). Allocation of attention in the visual field. J. Exp. Psychol. Hum. Percept. Perform. 11, 583–597.
Forster, K. I., and Dickinson, R. G. (1979). More on the language-as-fixed effect: Monte-Carlo estimates of error rates for F1, F2, F’, and minF’. J. Mem. Lang. 32, 781–804.
Gelman, A., and Hill, J. (2008). Data Analysis Using Regression and Multilevel/Hierarchical Models. Cambridge, MA: Cambridge University Press.
Kliegl, R., Masson, M. E. J., and Richter, E. M. (2010). A linear mixed model analysis of masked repetition priming. Vis. Cogn. 18, 655–681.
Kravitz, D. J., and Behrmann, M. (2008). The space of an object: object attention alters the spatial gradient in the surround. J. Exp. Psychol. Hum. Percept. Perform. 34, 298–309.
Laird, N. M., and Ware, J. H. (1982). Random-effects models for longitudinal data. Biometrics 38, 963–974.
Lamy, D., and Egeth, H. (2002). Object-based selection: the role of attentional shifts. Percept. Psychophys. 64, 52–66.
Lorch, R. F., and Myers, J. L. (1990). Regression analysis of repeated measures data in cognitive research. J. Exp. Psychol. Learn. Mem. Cogn. 16, 149–157.
Martínez, A., Teder-Salejarvi, W., Vazquez, M., Molholm, S., Foxe, J. J., Javitt, D. C., Di Russo, F., Worden, M. S., and Hillyard, S. A. (2006). Objects are highlighted by spatial attention. J. Cogn. Neurosci. 18, 298–310.
Posner, M. I., and Cohen, Y. (1984). “Components of visual orienting,” in Attention and Performance X: Control of Language Processes, eds H. Bouma and D. G. Bowhuis (Hillsdale, NJ: Erlbaum), 531–556.
Pratt, J., Spalek, T. M., and Bradshaw, F. (1999). The time to detect targets at inhibited and noninhibited locations: preliminary evidence for attentional momentum. J. Exp. Psychol. Hum. Percept. Perform. 25, 730–774.
Quené, H., and van den Bergh, H. (2004). On multi-level modeling of data from repeated measures designs: a tutorial. Speech Commun. 43, 103–121.
Quené, H., and van den Bergh, H. (2008). Examples of mixed-effects modeling with crossed random effects and with binomial data. J. Mem. Lang. 59, 413–425.
Raaijmakers, J. G. W., Schrijnemakers, J. M. C., and Gremmen, F. (1999). How to deal with “the language as fixed effect fallacy”: common misconceptions and alternative solutions. J. Mem. Lang. 41, 416–426.
Raudenbush, S. W., and Bryk, A. S. (2002). Hierarchical Linear Models: Applications and Data Analysis Methods, 2nd Edn. Newbury Park, CA: Sage.
R Development Core Team. (2010). R: A Language and Environment for Statistical Computing. Vienna: R Foundation for Statistical Computing. ISBN 3-900051-07-0, URL: http://www.R-project.org/
Self, S. G., and Liang, K.-L. (1987). Asymptotic properties of maximum likelihood estimators and likelihood ratio tests under nonstandard conditions. J. Am. Stat. Assoc. 82, 605–610.
Shomstein, S., and Yantis, S. (2002). Object-based attention: sensory modulation or priority setting? Percept. Psychophys. 64, 41–56.
Shomstein, S., and Yantis, S. (2004). Configural and contextual prioritization in object-based attention. Psychon. Bull. Rev. 11, 247–253.
Tracy, J. L., Robbins, R. W., and Sherman, J. W. (2009). The practice of psychological science: searching for Cronbach’s two streams in social–personality psychology. J. Pers. Soc. Psychol. 96, 1206–1225.
Vecera, S. P. (1994). Grouped locations and object-based attention: comment on Egly, Driver, and Rafal (1994). J. Exp. Psychol. Gen. 123, 316–320.
Venables, W. N., and Ripley, B. D. (2002). Modern Applied Statistics with S, 4th Edn. New York: Springer.
Keywords: linear mixed model, individual differences, visual attention, spatial attention, object-based attention
Citation: Kliegl R, Wei P, Dambacher M, Yan M and Zhou X (2011) Experimental effects and individual differences in linear mixed models: estimating the relationship between spatial, object, and attraction effects in visual attention. Front. Psychology 1:238. doi: 10.3389/fpsyg.2010.00238
Received: 21 June 2010;
Accepted: 15 December 2010;
Published online: 05 January 2011.
Edited by:
Moon-Ho R. Ho, Nanyang Technological University, SingaporeReviewed by:
Hong Xu, Nanyang Technological University, SingaporeRung-Ching Tsai, National Taiwan Normal University, Taiwan
Hsiu-Ting Yu, McGill University, Canada
Copyright: © 2011 Kliegl, Wei, Dambacher, Yan and Zhou. This is an open-access article subject to an exclusive license agreement between the authors and the Frontiers Research Foundation, which permits unrestricted use, distribution, and reproduction in any medium, provided the original authors and source are credited.
*Correspondence: Reinhold Kliegl, Department of Psychology, University of Potsdam, Karl-Liebknecht-Str. 24-25, 14476 Potsdam, Germany. e-mail:a2xpZWdsQHVuaS1wb3RzZGFtLmRl; Xiaolin Zhou, Center for Brain and Cognitive Sciences and Department of Psychology, Peking University, Beijing 100871, China. e-mail:eHoxMDRAcGt1LmVkdS5jbg==