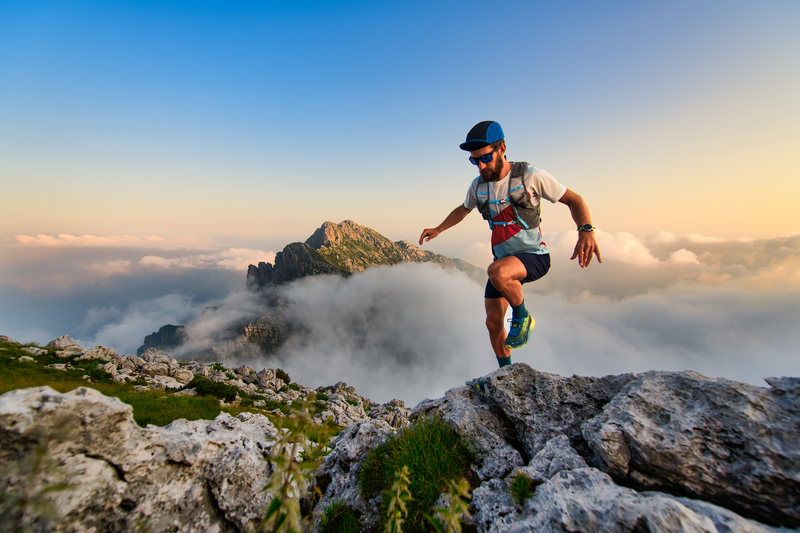
95% of researchers rate our articles as excellent or good
Learn more about the work of our research integrity team to safeguard the quality of each article we publish.
Find out more
SYSTEMATIC REVIEW article
Front. Psychiatry , 28 March 2025
Sec. Schizophrenia
Volume 16 - 2025 | https://doi.org/10.3389/fpsyt.2025.1540260
Introduction and methods: To discover biomarkers for schizophrenia (SCZ) at the metabolomics level, we registered this systematic review (CRD42024572133 (https://www.crd.york.ac.uk/PROSPERO/home)) including 56 qualified articles, and we identified the characteristics of metabolites, metabolite combinations, and metabolic pathways associated with SCZ.
Results: Our findings showed that decreased arachidonic acid, arginine, and aspartate levels, and the increased levels of glucose 6-phosphate and glycylglycine were associated with the onset of SCZ. Metabolites such as carnitine and methionine sulfoxide not only helped to identify SCZ in Miao patients, but also were different between Miao patients and Han patients. The decrease in benzoic acid and betaine and the increase in creatine were the notable metabolic characteristics of first-episode schizophrenia (FESCZ). The metabolite combination formed by metabolites such as methylamine, dimethylamine and other metabolites had the best diagnostic effect. Arginine and proline metabolism and arginine biosynthesis had a clear advantage in identifying SCZ and acute SCZ. Butanoate metabolism played an important role in identifying SCZ, toxoplasma infection and SCZ comorbidity. Biosynthesis of unsaturated fatty acids was also significantly enriched in the diagnosis and treatment of SCZ.
Discussion: This study summarizes the current progress in clinical metabolomic research related to SCZ, deepens understanding of the pathogenesis of SCZ, and lays a foundation for subsequent research on SCZ-related metabolites.
Systematic review registration: https://www.crd.york.ac.uk/PROSPERO/home, identifier CRD42024572133.
Schizophrenia (SCZ) is a clinically common severe mental illness, affecting approximately 1 in every 300 people, accounting for 1% of the global population. It is one of the top 10 leading causes of disability worldwide (1). Studies show that men are more likely to suffer from SCZ at a younger age (2). Symptoms of SCZ begin to appear between the ages of 20 and 29 and include delusions, hallucinations, and a lack of coordination between thinking and behavior (positive symptoms), and insociability, world-weariness, anorexia, and decreased energy (negative symptoms), and decreased attention and memory (cognitive dysfunction) (3). The social misunderstanding and stigmatization of patients with SCZ further aggravate their psychological burden and limit their social participation and rehabilitation opportunities. There is evidence that the life expectancy of people with SCZ is reduced by 15 to 25 years due to suicide, accidents, antipsychotic medications, lower quality of life, and various comorbidities such as cardiovascular disease, hypertension, and diabetes, inadequate medical care, and premature aging (4).
As of this writing, the diagnosis of SCZ remains overly subjective, not only because the symptom spectrum is complex and similar to other mental disorders, but also because of a lack of objective disease biomarkers (5). Moreover, the experience of clinicians is uneven, and it is difficult to make an accurate diagnosis based solely on a subjective understanding of symptoms. Thus, there is a significant clinical demand for biomarkers that can aid in the diagnosis of SCZ (6). The lack of reliable biomarkers leads to delays in diagnosis, preventing patients from receiving timely and effective treatment. Therefore, identifying biomarkers associated with SCZ is crucial for early diagnosis and treatment.
Metabolomics is an important part of system biology, and its research objects are small molecules with molecular weight less than 1,000 Da, such as sugars, organic acids, lipids, amino acids and aromatic hydrocarbons (7). Metabolomics techniques have been widely applied in basic research in recent years, such as nuclear magnetic resonance (NMR) technology, gas chromatography-mass spectrometry (GC-MS), liquid chromatography-mass spectrometry (LC-MS), and capillary electrophoresis-mass spectrometry (CE-MS) (8). Principal component analysis and partial least squares discriminant analysis provide a statistical foundation for identifying differential metabolites. According to varying research objectives, metabolomics can be classified as either non-targeted metabolomics or targeted metabolomics (9). In non-targeted metabolomics, the entire metabolome of an organism is comprehensively searched to detect any metabolic characteristics in which there are significant changes between the experimental and control groups; while in targeted metabolomics, target metabolites are studied to verify biomarkers. In metabolomics, there is a terminal effect and an amplification effect, which can reflect organisms’ disease physiological states more directly and sensitively than genomics or transcriptomics. Compared with genomics and proteomics, metabolomics studies have fewer substances and a simpler information bases, which gives metabolomics a unique advantage in disease diagnosis and biomarker discovery. The use of metabolomics to study SCZ has attracted more and more attention in recent years, and related research is on the rise. However, the quality of these studies has been mixed, and the aims and methods of these studies have been multifarious, so it is currently necessary to consider many studies together to form consensus.
To this end, we used a systematic review to summarize current research progress in SCZ at the metabolomics level, elaborate on the metabolite changes related to SCZ, reveal the pathogenesis of SCZ, and determine a metabolite combination that can identify patients with SCZ, so as to lay a foundation for a more accurate diagnosis of SCZ. Moreover, this study also explores the current challenges in metabolomics research related to SCZ, providing direction for future studies. Through this systematic review, insight and ideas for the diagnosis, prognosis, and disease detection of SCZ are provided.
This systematic review was registered with PROSPERO (CRD42024572133) in accordance with the requirements of a routine systematic review.
We searched for metabolite studies related to SCZ in PubMed, Embase, and Web of Science databases, with a literature time span from database establishment to August 2024. The search strategy was formulated using a combination of subject words and free words. The main subject terms were: “schizophrenias”, “dementia praecox”, “schizophrenic disorders”, “disorder,schizophrenic”, “disorders, schizophrenic”, “schizophrenic disorder”, “metabolomics”, “metabolome”, “metabolic flux analysis”, “metabolic profiling”, “metabolic signature”, “metabolic biomarker” and “meta-bolic profile”. The literature was searched separately by two researchers, and if there was any disagreement, it was decided by senior literature experts. The specific search strategy can be found in the Supplementary Materials. We searched for relevant literature based on the pre-established search strategy, and then imported the retrieved literature into NoteExpress (version 4.1.0).
1. Study subjects: patients with SCZ diagnosed by the Diagnostic and Statistical Manual of Mental Disorders (DSM) or International Classification of Diseases (ICD)-10.
2. Research types: case-control, randomized controlled trials, etc.
3. Research content: the diagnosis and prognosis of SCZ through metabolomics.
4. Data types: human-involved clinical studies, including clinical and metabolomic data.
5. Language type: English.
Duplicate literature or literature published with already-published data; literature involving other diseases or literature not involving metabolite studies; comments and letters; animal experiment literature; review, Mendelian randomization research, protocol, and case reports; literature with incomplete data or data that cannot be extracted; research on intervention mechanisms; literature that was unavailable in full-text format.
After determining the final included literature based on the inclusion and exclusion criteria, we began to extract relevant information from the included studies. First, we recorded the extracted author name, publication year, journal, country, research subjects, gender, group settings, research type, sample size, analysis platform, research purpose, sample type, whether it was a targeted study, any changes in related metabolites, and the pathways involved in Excel. If a study included both case-control studies and self-controlled studies before and after intervention, it was divided into two studies to extract the data.
We used QUADOMICS (Quality Assessment of Diagnostic Accuracy Studies for Omics), a quality assessment tool specifically modified for omics studies, in assessing the methodological quality of studies related to differential metabolites that can be used in the diagnosis of SCZ (10). This tool evaluates the methodological quality of the literature from 16 dimensions: patient selection, selection of diagnostic criteria, randomization methods, description of specimen types, interpretation of results, reproducibility of trial protocols, and rigor of trial execution. As for studies related to metabolites associated with SCZ prognosis, we used the QUIPS (Quality In Prognosis Studies tool for evaluation (11). This tool assesses the quality of a study from six perspectives: selection of participants, study attrition, measurement of diagnostic factors, measurement of outcomes confounding factors of the study, and statistical analysis and reporting. Documents that meet 75% and above of the items are considered high-quality documents, those that meet 50-75% are considered medium-quality documents, and those that meet less than 50% are considered low-quality documents (12).
In order to comprehensively summarize the current progress of metabolomics research in SCZ, we recorded the countries, specimen types, metabolite detection technologies, related metabolites and metabolic pathways involved in the study, and used descriptive statistics to analyze their frequencies and percentages. Then, we used visualization to display them.
We conducted a summary statistical analysis of the metabolites involved in high-quality literature, collated information on the accuracy (Area Under Curve, AUC) of metabolite combinations in the diagnosis and prognosis analysis of SCZ and completed the metabolic pathway enrichment using MetaboAnalyst 6.0 online software (http://www.metaboanalyst.ca/). This comprehensive analysis of significantly enriched pathways in high-quality literature revealed the pathological mechanisms associated with SCZ.
We used Adobe Illustrator (2024 version) to create a flowchart for literature search and screening, and mapped the study sites via Wei Sheng Xin (https://www.bioinformatics.com.cn/). Other plots were completed in Rstudio (version 4.4.2); the histogram of specimen classification and the enrichment map of metabolite pathways for the high-quality literature were conducted in the ggplot2 package (version 3.5.1). We drew the literature quality assessment chart with the dplyr package (version 1.1.4) and ggplot2 package (version 3.5.1) and prepared the high-quality literature accumulation histograms using the reshape2 package (version 1.4.4) and ggplot2 package (version 3.5.1).
Based on the pre-determined search strategy, we retrieved 215, 341, and 902 articles from the PubMed, Embase, and Web of Science databases, respectively. After importing the above 1,458 documents into NoteExpress, 306 duplicate documents were eliminated by author, publication year and title. After reading the title, abstract and full text to eliminate unqualified documents, 56 documents were ultimately included. The literature search and screening process is depicted in Figure 1.
The 56 articles included in this study involved 6,772 participants, with one study only including male patients and two studies only including female patients. Since two of the studies (13, 14) included both case-control and pre-and post-intervention self-control studies, each study was split into two studies for statistical analysis. The sample size for a single study ranged from 29 to 481. The 58 study sites were distributed in Japan (5), China (36), the United Kingdom (2), the United States (2), Brazil (3), Germany (4), and the remaining 6 were completed in Poland, Australia, the Czech Republic, Russia, Finland and Malaysia, respectively. 52 studies were designed to identify any metabolites that differed between people with SCZ and healthy controls. One study was conducted to compare the metabolites of SCZ between Chinese Han and Miao ethnic groups and the healthy population. Two studies involved first-episode schizophrenia (FESCZ). One study focused on patients with chronic schizophrenia (CSCZ). Three studies explored metabolite differences in untreated SCZ patients. What’s more, two studies explored the blood metabolites that differ in cognitively impaired and cognitively intact SCZ patients. One study explored the plasma differential metabolites of SCZ with violence tendency and SCZ without violence tendency. Another study investigated salivary metabolites in patients with clozapine-induced salivation and in patients without clozapine-induced salivation. Another explored the differential metabolites of the pituitary gland after autopsy in SCZ patients, and another explored metabolite differences in patients with SCZ caused by toxoplasma gondii infection. Two studies explored the differences in metabolites before and after intervention based on self-control before and after intervention. One study explored the metabolomic differences between SCZ with and without auditory hallucinations. 54 studies employed a case-control design, while two were cross-sectional studies. 51 studies were diagnostic, 5 were used for complication identification, and 2 involved prognostic analysis. Among the 58 metabolomics studies related to SCZ, 49 used blood as the specimen, which may be because blood sampling provides convenience for clinical research. In addition, 39 of these 58 studies performed metabolomics studies using LC-MS and 5 used NMR techniques to detect metabolites. In 37 studies, non-targeted metabolomics technology was used, and targeted technology was used in 19. Two studies did not report whether targeted technology was used. See Supplementary Table S1; Figure 2 for details.
Figure 2. Results of the basic characteristics of the included literature. (A). Distribution of research locations included in the literature. (B). Classification of specimens involved in the study. (C). Round pie chart of the metabolite detection technique. (D). Round pie chart of whether to target detection. NMR, nuclear magnetic resonance; GC-MS, gas chromatography-mass spectrometry; LC-MS, liquid chromatography-mass spectrometry; CE-MS, capillary electrophoresis-mass spectrometry; MS, mass spectrometry.
Of the 51 diagnostic studies, 39 met the scoring criteria on at least 12 dimensions and were rated as high quality, while the remaining 12 were rated as medium quality. One of the two prognostic analyses adequately controlled for confounders and considered dropout to reduce bias. Of the 7 studies that identified complications and analyzed prognosis, 5 were high-quality and 2 were intermediate-quality. See Figure 3 for specific information.
Figure 3. Quality assessment chart of included literature. (A) Results of a methodological quality assessment of studies related to differential metabolites that can be used for the diagnosis of schizophrenia. (B) Results of a methodological quality assessment of studies related to differential metabolites that can be used to predict the prognosis of schizophrenia.
One study (15) explored the metabolic differences in serum levels between patients with CSCZ and healthy individuals, and found that glutamate metabolism and the urea cycle were severely downregulated after onset. Meanwhile, another study (16) discovered significant differences in serum metabolites between Han and Miao schizophrenics and healthy controls in China. The metabolites Han and Miao patients had in common were: fatty acids and derivatives (e.g., indole-3-butyric acid, 2-oxovalic acid, eicosapentaenoic acid), amino acids (e.g., glutamate, pyroglutamic acid, proline, taurine), and other types (e.g., bilirubin, uric acid, α-tocopherol). The pathways with significant metabolic alteration in Miao patients were the arachidonic acid metabolism and α-linolenic and linoleic acid pathway. Meanwhile in Han Chinese patients, arginine and proline metabolism, arachidonic acid, alanine metabolism, the urea cycle, and glycine and serine pathways had changed significantly. Lipid and amino acid metabolism were common metabolic pathways across different ethnic groups of patients. Two studies (17, 18) compared the differences in plasma metabolites between patients with FESCZ and a normal population. They discovered that in patients with FESCZ, levels of creatine, isocitrate, succinic acid, itaconic acid, and L-2-hydroxyglutarate were all upregulated, while levels of betaine, nonanoic acid, benzoic acid, perillic acid, L-3,4-dihydroxyphenylalanine (L-dopa), dopamine 3-O-sulfate, norepinephrine sulfate, and normetanephrine were downregulated. Additionally, alterations were observed in the metabolic pathways of homocysteine metabolism, creatine kinase-emia, oxidative stress, aromatic amino acid metabolism, glutamate metabolism, nucleotide metabolism, and the tricarboxylic acid cycle. Two studies (19, 20) compared the metabolic differences between untreated SCZ patients and healthy individuals, finding that the differential metabolites were concentrated in aspartic acid, carnitine, lithocholic acid, lyso-phosphatidylcholine, lyso-phosphatidylethanolamines, phosphatidylcholine, phosphatidylethanolamine, and γ-aminobutyric acid (GABA). It was worth mentioning that 41 studies (6, 13, 21–59) reported a series of differential metabolites related to SCZ, including eicosanoids, docosahexaenoic acid, eicosapentaenoic acid, ethanamide, eicosadienoic acid, linolic acid, arginine, tryptophan and other metabolites. Two studies (60, 61) reported changes in metabolites induced by cognitive function, including sphinganine, D-glutamine, pyrrolidonecarboxylic acid, choline and creatine, lactic acid, aspartic acid, erythronic acid and 2-furoic acid. What’s more, one study (62) reported metabolites associated with violent tendencies in SCZ, including vanillylmandelic acid, glycerol, glyceraldehyde, malic acid and L-methionine. One clinical trial (63) explored the metabolite changes induced by salivation in patients with SCZ induced by clozapine such as guanosine, adrenaline, pyroglutamate, histidine, deoxycytidine, histamine, D-ornithine, isoleucyl-glutamate, hypoxanthine and kynurenine. A study from Germany (64) investigated SCZ from the perspective of pituitary metabolites and found differences in metabolites such as signal-induced proliferation-associated protein 1, protein KIAA1199, fibrinogen beta chain, prolactin, and secretagogin. Another study (65) revealed a correlation between toxoplasma infection and the onset of SCZ, involving metabolites such as alpha hydroxyglutaric acid, caprolactam, 3,30-thiopropionic acid, adenosine monophosphate, inosine, hypoxanthine and xanthine. A different study (66) analyzed the metabolites related to auditory hallucination, and found that metabolites such as phenylalanine, pyrroline hydroxyl carboxylic acid and pyruvate were related to auditory hallucination. Two studies (13, 14) reported metabolite changes in SCZ patients after antipsychotic treatment, including: tyrosine, linoleic acid, palmitic acid, oleic acid, tryptophan, uric acid, lactate, aspartate, glycine, myo-inositol, glucuronic acid, stearic acid, glycerol, lactobionic acid, lysoPC, sulfate, linoleic acid, oleic acid, palmitoleic acid, γ-linolenic acid, oxoglutaric acid, and androsterone.
Frequency analysis of the metabolites involved in high-quality research showed the commonalities of metabolomics research in different backgrounds (see Figure 4). Compared with the healthy control population, the main up-regulated metabolites of SCZ were glucose 6-phosphate and glycylglycine; the main down-regulated metabolites were arachidonic acid, arginine, aspartate, citrate, creatinine, glutamine, LPC (14:0), LPC (15:0), LPC (17:1), LysoPC (18:0), oleic acid, stearic acid and tryptophan. Metabolites with up-regulation in some studies and down-regulation in others included: cholesterol, cortisol, creatine, GABA, glucose, glycerol, L-arginine, lactic acid, lactate, leucine, LPC (16:0), myo-inositol, ornithine, proline, pyroglutamic acid. There were also differences in metabolites between Miao and Han SCZ patients. Glutamate and methionine sulfoxide were up-regulated more often in Miao SCZ patients than in Han SCZ patients, and carnitine and decanoylcarnitine were more often down-regulated in Miao SCZ patients. Within the Miao population, there were also differences in metabolites between SCZ patients and healthy people. Compared with the healthy Miao population, on the one hand, the main up-regulated metabolites in Miao SCZ patients were: phthalic acid, 3-hydroxybenzoic acid, methionine sulfoxide, N-acetyl-L-2-aminoadipa, suberic acid, 5-aminopentanamide, 9-oxo-nonanoic acid, caprylic acid, camphanic acid, perillic acid and cholic acid glucuronide, on the other hand, the main down-regulated metabolites were: cystine, aspartylphenylalanine, leucine, aminobutyric acid, decanoylcarnitine, 3-carboxy-4-methyl-5-pr, carnitine, nicotinamide riboside, pentachlorophenol, coniferyl alcohol, 3-3,4,5-trimethoxyphen and abscisic acid. Compared to healthy individuals, creatine was significantly upregulated in FESCZ patients, while betaine, nonanoic acid, benzoic acid and perillic acid were significantly downregulated. The metabolic profile of pituitary tissue in patients with SCZ was also altered. Compared to healthy individuals, SCZ patients showed significantly upregulated levels of fibrinogen beta chain, proopiomelanocortin, and myosin-9 in their pituitary tissue, while levels of prolactin, secretagogin, catenin delta-2, transglutaminase 2, apolipoprotein A2, tubulin beta chain, and alpha-2-hs-glycoprotein were significantly downregulated. Compared to healthy individuals without toxoplasma infection, SCZ patients with concurrent toxoplasma infection showed a significant increase in α-hydroxyglutaric acid and caprolactam, while inosine, hypoxanthine, and xanthine were significantly downregulated. Effective treatment can reverse metabolic changes. After treatment, patients with SCZ showed a significant increase in palmitic acid, phenylalanine, tyrosine, uric acid, and γ-tocopherol, and a significant decrease in androsterone, aspartate, glucuronic acid, glycine, myo-inositol, and stearic acid. Oleic acid and linoleic acid exhibited upregulation in some studies and downregulation in others. Comprehensive analysis revealed that tyrosine and γ-tocopherol were downregulated in patients with SCZ and significantly upregulated after effective treatment. Therefore, we speculate that tyrosine and γ-tocopherol can not only be used for the accurate identification of SCZ patients, but also for predicting treatment outcomes.
Figure 4. Accumulation histograms of metabolites associated with SCZ in high quality literature. Lyso-PC, Lyso-phosphatidylocholine; GABA, γ-aminobutyric acid.
A total of 30 studies used metabolites or metabolite combinations to identify SCZ or predict treatment prognosis, among which 8 (16, 27, 35, 44, 47–50) used multiple individual metabolites for AUC analysis, and 22 (6, 13, 14, 19–21, 26, 30, 31, 34, 36, 38, 39, 42, 43, 51–57) used metabolite combinations. At the single metabolite level, methionine sulfoxide had the highest accuracy in identifying Miao SCZ patients (test set AUC = 0.98) and c-glucys had the highest accuracy in identifying general SCZ patients (test set AUC = 0.8874). Pyruvate can be used to predict whether SCZ patients will experience auditory hallucinations (AUC=0.8394 in test). At the level of metabolite combination, the metabolite group formed by methylamine, dimethylamine, N-(1-deoxy-1-fructosyl) isoleucine, phenylalanylphenylalanine, LPA (18:1 (9Z)/0:0), and oleamide showed the best accuracy in identifying SCZ patients (training set AUC=1, test set AUC=1), thencholic acid, 4,8 dimethylnonanoyl carnitine, 3-hydroxycapric acid and prostaglandin A2 also showed good performance (training set AUC=0.9917, test set AUC=0.9945). The metabolite panel consisting of PC 32:1, Pe 34:2, PE (O-34:3), and aspartic acid performed well in identifying both untreated SCZ and medically treated SCZ patients (training set AUC = 0.936, test set AUC = 0.963). Myoinositol, uric acid, and tryptophan can serve as a metabolite combination to predict the treatment efficacy in SCZ (test set AUC=0.949). Imidazolepropionic acid, erythronic acid, homoserine, and aspartic acid can be used to predict the salivation induced by chlorpromazine treatment in SCZ (test set AUC=0.791).
We conducted metabolic pathway enrichment analysis by matching the metabolites in the high-quality literature with the MetaboAnalyst 6.0 database. This resulted in 6 major categories and 98 metabolic pathways (Figure 5). Compared with healthy controls, there were significant enrichment pathways in SCZ such as arginine and proline metabolism, arginine biosynthesis, neomycin, kanamycin and gentamicin biosynthesis, glutathione metabolism, alanine, aspartate and glutamate metabolism, valine, leucine and isoleucine biosynthesis, galactose metabolism, glyoxylate and dicarboxylate metabolism, biosynthesis of unsaturated fatty acids, starch and sucrose metabolism, pantothenate and CoA biosynthesis, beta-alanine metabolism and other pathways. In the comparison between ethnic Miao and Han SCZ patients in China, the metabolite pathway enrichment analysis identified pathways such as nitrogen metabolism, arginine biosynthesis, butanoate metabolism, and histidine metabolism. Valine, leucine and isoleucine biosynthesis, nicotine and nicotinamide metabolism, butanoate metabolism and other pathways were significantly enriched in the comparison between SCZ patients and the healthy control population within the Miao ethnic group in China. Pathways such as histidine metabolism, glycine, serine and threonine metabolism, argenine and proline metabolism, nitrogen metabolism, argenine biosynthesis, and butanolate metabolism were significantly enriched in comparison between FESCZ patients and the healthy controls. Only the purine metabolism and butanoate metabolism pathways were significantly enriched in the comparison between SCZ patients co-infected with toxoplasmos and healthy people not infected with toxoplasmosis. The biosynthesis pathways of unsaturated fatty acids, phenylalanine, tyrosine, and tryptophan, phenylalanine metabolism, ascorbate and aldarate metabolism, inositol phosphate metabolism, and linoleic acid metabolism were significantly enriched in the comparison before and after effective treatment.
Figure 5. Enrichment results of metabolites pathways in high-quality literature. The size of the circle represents the number of metabolites, and the larger the circle, the more metabolites are enriched. The color of the circle represents the significance of the enrichment analysis. The redder the color of the circle, the more significant the enrichment analysis. (A) Schizophrenia versus healthy control. (B) Miao ethnic schizophrenia versus Miao ethnic healthy control. (C) Miao ethnic schizophrenia versus Han ethnic schizophrenia. (D) First-episode schizophrenia versus healthy control. (E) Schizophrenia patients co-infected with toxoplasmos versus healthy control not infected with toxoplasmosis. (F) Schizophrenia patients at baseline versus schizophrenia patients after intervention.
In this study, different metabolites in serum, plasma, cerebrospinal fluid (CSF), saliva, prefrontal cortex and pituitary tissues were found between SCZ patients and healthy controls, such as arachidonic acid, GABA, tryptophan and proline. These metabolites are involved in the pathogenesis of SCZ through a variety of metabolic pathways, including glutathione metabolism, butanoate metabolism, histidine metabolism, linoleic acid metabolism and kynurenine pathway, involving various biological mechanisms, such as oxidative stress response, energy metabolism, purine metabolism. This study has facilitated the deepening of clinical staff’s understanding of the pathogenesis of SCZ. At the same time, it has provided clinical staff with a potential diagnostic biomarker combination and provided options for subsequent accurate diagnosis.
A systematic review of body fluid metabolomics for SCZ patients analyzed the differential metabolites and metabolic pathways between SCZ patients and healthy subjects (5), the study found that the content of polyunsaturated fatty acid (linoleic acid) and creatinine decreased in SCZ patients, which is consistent with the results of our study. In our study, in addition to the literature on serum, plasma, CSF, and peripheral blood mononuclear cells, we also selected studies involving the prefrontal cortex and pituitary gland. Thus, the specimens in this study are both more comprehensive and more convincing. Lipidomics, as a branch of metabolomics, can also be used to study the differences in metabolites and metabolic pathways between patients and healthy controls. A recent systematic review, including brain tissue (prefrontal cortex) and peripheral blood, analyzed lipidomic changes in SCZ patients from four perspectives: triglycerides, phospholipids, sphingolipids, and steroids (67). The study found that the levels of sulfates, N-acylphosphotidylserines, ethanolamine, and choline plasminogen were significantly upregulated in brain tissue, while the levels of phosphatidylethanolamine, lysophosphatidylethanolamine, phosphatidylcholine, lysophosphatidylcholine, and ethanolamine plasminogen were significantly downregulated in peripheral tissues. Moreover, the level of ethanolamine plasmalogen was significantly down-regulated in the plasma of patients with both first-episode and recurrent SCZ, which is crucial for clinical diagnosis. In our study, we also revealed that the plasma levels of phosphatidylethanolamine and lysophosphatidylcholine had decreased in women with SCZ. Moreover, we found that isocitrate, succinic acid and itaconic acid were up-regulated, and L-3,4-dihydroxyphenylalanine (L-dopa), dopamine 3-osulfate and norepinephrine sulfate were down-regulated in FESCZ patients. Another study has shown abnormal metabolism of isoform 1 of dimethylarginine dimethylaminohydrolase (DDAH1) and arginase in patients with SCZ, manifested specifically by abnormally elevated levels of asymmetric dimethylarginine (ADMA), dimethylamine, and ornithine (68), However, the study found no significant difference in arginine levels between SCZ patients and controls. Ourstudy not only revealed that ADMA and ornithine increased in SCZ patients, but also that ornithine can be used to predict whether cognitive function of SCZ patients is impaired, and L-ornithine and D-ornithine decreased in SCZ patients. In contrast to previous studies, we also found that arginine levels decreased in patients with SCZ, but the results for L-arginine levels were not consistent across studies. Several systematic reviews have indicated that omega-3 dietary supplementation can mitigate negative symptoms in FESCZ and partially alleviate the symptoms of CSCZ patients (69). Our study also indicated that the content of omega-6 polyunsaturated fatty acids (PUFA) increased in patients with SCZ. Therefore, we speculate that different types of fatty acids may play distinct roles in the pathogenesis of SCZ. More and more studies are exploring the role of the kynurenine pathway in the pathogenesis of SCZ. It is generally believed that the kynurenic acid (KYNA) content increases in the central nervous systems (CNS) of SCZ patients, and that the kynurenine pathway is over-activated in SCZ patients (70). However, in our study, we found that KYNA levels had declined in peripheral serum, which may have been due to the inability of KYNA to penetrate the blood-brain barrier. The above comparison further confirms the role of specific metabolites in the diagnosis and prognosis of SCZ.
A single study may be affected by confounding factors, making it difficult to derive strong persuasiveness. Therefore, in this systematic review, we comprehensively analyzed multiple studies and found that the changes from metabolites to pathways further revealed the pathological mechanism of SCZ at the metabolite level.
Amino acids are known for supporting neural function in two ways, directly acting as neurotransmitters to mediate neural communication and participate in signal transmission in neurons, and indirectly participating in energy supply as metabolic substrate (71). It is generally believed that abnormal arginine metabolism is involved in the pathogenesis of SCZ from multiple perspectives, such as NO regulation, immune inflammatory response, and mitochondrial dysfunction. Previous studies have found that the arginine biosynthetic pathway is a genetic risk factor for SCZ (51), and our study also found significant enrichment in SCZ versus healthy populations. Arginine can be decomposed into many metabolites under different conditions: nitric oxide (NO), citrulline, ornithine, urea and agmatine. Arginine is a precursor substance for the synthesis of NO. The gaseous signaling molecule NO plays an important role in nerve signaling and nerve cell protection, and can also regulate synaptic plasticity, neurodevelopment and cerebral blood flow. Excessive free radical NO can produce neurotoxicity and neurodegeneration (72). NO synthesis is affected in SCZ and may be related to changes in arginine metabolites. For example, the levels of ADMA and ornithine vary significantly in SCZ, and changes in these metabolites may affect the synthesis of NO and thus the functional and behavioral performance of neurons (67). Ornithine can be directed to the production of glutamic acid, glutamine, and GABA (73), and is a major precursor to polyamine putrescine, spermidine, and spermine, which are essential for maintaining normal cellular function. Immune inflammation is considered an important factor in the pathogenesis of SCZ. The levels of inflammatory factors (such as IL-6) are significant in patients with SCZ, and arginine metabolism is closely related to the inflammatory response. Arginine regulates the inflammatory response by affecting the activity of microglial cells, which may alleviate neuronal damage and the exacerbation of psychiatric symptoms (74). Mitochondrial dysfunction is closely related to the pathogenesis of SCZ. Changes in arginine metabolism may affect mitochondrial energy metabolism (75), leading to insufficient energy and oxidative stress in neurons, increasing the risk of SCZ.
Arginine metabolism can regulate tryptophan metabolism. Arginine can activate mTOR signaling pathway, and mTOR activation can regulate the activity of indoleamine-2,3-dioxygenase (IDO). IDO is a key enzyme in tryptophan metabolism, which catalyzes tryptophan metabolism along the kynurenine pathway, thus affecting the metabolism level of tryptophan (76). The tryptophan metabolic pathway has been a focus of SCZ researchers. As an essential amino acid, tryptophan is metabolized in the human body primarily through two pathways: the 5-HT pathway and the kynurenine pathway. Approximately 95% of tryptophan is metabolized via the kynurenine pathway. Specifically, tryptophan is converted into kynurenine through the catalytic action of tryptophan-2,3-dioxygenase (TDO) and IDO in the CNS (microglia and astrocytes) and the peripheral nervous system (liver and kidneys). Kynurenine then undergoes further metabolism through two branches: 1) it is converted into KYNA under the action of kynurenine transaminase; 2) it is first converted into 3-hydroxykynurenine (3-HK) under the action of kynurenine monooxygenase (KMO), and then generates 3-amino-4-hydroxybenzoic acid under the action of kynureninase, ultimately breaking it down into quinolinic acid (70). Quinolinic acid and KYNA are agonists and antagonists of N-methyl-D-aspartic acid receptor (NMDAR), respectively, and KYNA is also an α7 nicotinic acetylcholine receptor (α7nAChR) antagonist. Thus, quinolinic acid and KYNA are considered neuromodulators (77). Nicotinic acetylcholine receptors are mainly found in brain regions such as the hippocampus and the prefrontal cortex which regulate cognitive function, as well as in midbrain dopaminergic nerve cells. Additionally, NMDAR can negatively regulate the uptake and release of glutamate in the presynaptic membrane. Therefore, the abnormally high content of KYNA can dysregulate multiple neurotransmitters, such as glutamate, dopamine and acetylcholine (78–81). Research has indicated that abnormally elevated levels of KYNA are associated with cognitive function impairment, suggesting that the aforementioned neurotransmitter may mediate this pathological process (77).
Other than arginine metabolism, proline metabolism is the most significant pathway for functional enrichment in SCZ (82). Proline is a nonessential amino acid synthesized by pyrroline-5 carboxylate synthesis (P5CS) and pyrroline carboxylate reductases (PyCRS), and decomposed by proline dehydrogenase (PRODH) (83). It is involved in many important metabolic processes, such as glycolysis, the tricarboxylic acid cycle and the pentose phosphate pathway. PRODH is the first proline-degrading enzyme in the metabolic pathway, and it has been identified as a susceptibility gene for SCZ (84). Animal studies confirm that PRODH knockout mice exhibit SCZ-like behavior (85), We speculate that this may be because reduced proline degradation causes accumulation, and proline accumulation can independently lead to the onset of SCZ. This may be because proline has structural similarities with GABA and glutamate, which may affect the binding site (86).
Modern neuroimaging research has shown that the first brain area affected in patients with SCZ is the hippocampus, and specifically its CA1 area (87). The main pathophysiological mechanisms of hippocampal dysfunction are hyperactivity, atrophy, and abnormal increase in glutamate content (88). However, the level of glutamate in SCZ-related studies has historically been controversial (89). Recent research suggests that primary pyramidal dysfunction leading to decreased glutamate levels is at the forefront of SCZ studies (90). Our research reveals new insight into the changes in glutamate associated with SCZ. It suggests that the manifestation of positive symptoms in SCZ also depends on secondary disinhibition effects, which arise from downstream adaptive changes in inhibitory feedback, resulting in an increase in glutamate levels compared to previous states. Specifically, the full process of SCZ is divided into three stages: the genetic susceptibility state, the clinical high-risk state, and the diagnosed state with symptomatic manifestations. People who are genetically susceptible have lower levels of glutamate, especially in the anterior cingulate cortex. The low levels of glutamate in the population are associated with the core symptoms of SCZ, reflecting reduced excitability; people at high clinical risk will have a secondary disinhibition effect, and their levels of glutamic acid will be relatively excessive, and positive symptoms will also appear. When the deinhibition state is fully present, the patient will exhibit significant core symptoms, and glutamate levels will normalize (90). A recent clinical trial has revealed similar results for glutamate content in the anterior cingulate cortex by comparing glutamate patients with genetically high risk, clinically high risk, FESCZ (91). This theoretical interpretation profoundly explains the broad variation inglutamate content in patients, and why the linear relationship between glutamate content and core symptoms of SCZ is elusive.
The pathogenesis of SCZ is related to multiple metabolites, and some patients may also have sleep disorders. Research has shown that butanoate metabolism plays an important role in the pathogenic mechanism of sleep disorders in patients with SCZ (92). Another study revealed that butanoate metabolism was significantly downregulated in the gut microbiomes of patients after amisulpride treatment (93). Our study found that butanoate metabolism was significantly enriched in Chinese ethnic Miao patients, FESCZ, patients with SCZ and toxoplasma infection. This shows that butanoate metabolism is widely involved in the pathogenesis of SCZ, yet the current understanding of butanoate metabolism in the field of neuropsychiatry is not comprehensive. Thus, its role remains to be explored. Butanoate metabolism is significantly downregulated in patients with Parkinson’s disease (94), and is also significantly associated with prenatal depression (95). In metabolomics studies, butyric acid metabolism has been considered a form of carbohydrate metabolism. We speculate that the correlation between SCZ and butyric acid metabolism may be mainly due to the downregulation of butyric acid metabolism leading to energy supply disorders in patients with SCZ.
Our study is generally consistent with the key pathological mechanisms (oxidative stress, neuroinflammation, energy metabolism) discovered in previous studies (5). In fact, there are many metabolites closely related to the pathological mechanisms of SCZ, such as lipid metabolites: LPC (18:0), LPC (20:0), PC (18:2/18:2), PC (O-16:0/18:2), LPE (20:4), and PE (P-18:0/18:2), which collectively improve the diagnostic accuracy of SCZ (39). As a long-chain organic acid, fatty acid metabolism plays an important role in the pathogenesis of SCZ. PUFA are important fatty acids which can be classified as either ω-3 fatty acids or ω-6 fatty acids. Linoleic acid and linoleic acid are ω-3 fatty acids and ω-6 fatty acids, respectively, which affect the energy supply of SCZ (13, 14, 46, 50). Arachidonic acid is another important ω-6 fatty acid, and it has the most consistent findings in SCZ considering that the content of arachidonic acid is decreased in patients with SCZ (5, 46, 50).
In summary, the multiple metabolites we found in this study not only summarize the metabolic disorders related to SCZ, but also indicate the direction for effective treatment. Metabolites are widely involved in the pathogenesis of SCZ and are also effective indicators for judging and predicting treatment effectiveness.
There has been considerable progress in metabolomics research in both experimental design and metabolite detection techniques. However, the current research results cannot fully explain the pathological mechanism of the disease, and many challenges remain in this field. Firstly, the current trial designs primarily focus on case-control studies, exploring the metabolic changes in patient populations by comparing them with healthy individuals. This comparative approach is beneficial for uncovering the pathological mechanisms of diseases at the metabolic level. At present, the case-control design of SCZ has been well studied, and we suggest that more metabolomics studies be placed on clinical treatment to explore the mechanism of effect from the metabolomics level. Secondly, considering the convenience of sampling, most of the current research analyzes the changes of metabolites in the serum and plasma, and the changes of metabolites in the peripheral circulation system cannot completely reflect the changes of metabolites in CNS. Thus, it has been suggested that the changes of metabolites in CSF and brain tissue deserve more attention, and that the changes of metabolites in both central and peripheral tissues should be considered jointly in subsequent research. Finally, due to its genetic specificity, SCZ is the result of the interaction between genetic and environmental factors, and current research has under-emphasized impoverished regions, such as Africa. In order to fully understand the pathogenesis of SCZ in different populations, patients from impoverished regions such as Africa should also be studied.
In this study, we have summarized the current clinical metabolomics research progress related to SCZ, and thoroughly explored the metabolite changes related to onset and prognosis among different populations. This systematic review summarizes dozens of studies, deepening our understanding of the pathogenesis of SCZ and laying the foundation for future research on metabolites related to SCZ.
The original contributions presented in the study are included in the article/Supplementary Material. Further inquiries can be directed to the corresponding authors.
GY: Conceptualization, Formal analysis, Methodology, Software, Supervision, Visualization, Writing – original draft. JZ: Conceptualization, Formal analysis, Methodology, Software, Visualization, Writing – original draft. YH: Data curation, Formal analysis, Methodology, Software, Visualization, Writing – original draft. HL: Investigation, Methodology, Resources, Validation, Writing – original draft. JP: Methodology, Resources, Validation, Writing – original draft. JW: Resources, Validation, Writing – original draft. TJ: Investigation, Methodology, Validation, Writing – original draft. FD: Investigation, Resources, Validation, Writing – original draft. CL: Investigation, Validation, Writing – original draft. XL: Supervision, Validation, Writing – review & editing. CT: Resources, Supervision, Validation, Writing – review & editing. LL: Funding acquisition, Supervision, Writing – review & editing.
The author(s) declare that financial support was received for the research and/or publication of this article. This study was supported by the National Natural Science Foundation of China (82174527); the Project of First Class Universities and High-level Dual Discipline for Guangzhou University of Chinese Medicine (A1-2601-24-414-109Z71, A1-2601-24-415-109Z50, and A1-2601-24-415-109Z76); and Zhongshan Coma and Wake-up Promotion Workshop of Traditional Chinese Medicine (A2-3105-242-109-001).
We sincerely thank every staff member and participant who participated in the included studies. It was their joint efforts that made the subsequent research a reality.
The authors declare that the research was conducted in the absence of any commercial or financial relationships that could be construed as a potential conflict of interest.
The author(s) declare that no Generative AI was used in the creation of this manuscript.
All claims expressed in this article are solely those of the authors and do not necessarily represent those of their affiliated organizations, or those of the publisher, the editors and the reviewers. Any product that may be evaluated in this article, or claim that may be made by its manufacturer, is not guaranteed or endorsed by the publisher.
The Supplementary Material for this article can be found online at: https://www.frontiersin.org/articles/10.3389/fpsyt.2025.1540260/full#supplementary-material
1. Velligan DI, Rao S. Schizophrenia: salient symptoms and pathophysiology. J Clin Psychiatry. (2023) 84:1–2. doi: 10.4088/JCP.MS21078COM7
2. Ochoa S, Usall J, Cobo J, Labad X, Kulkarni J. Gender differences in schizophrenia and first-episode psychosis: a comprehensive literature review. Schizophr Res Treat. (2012) 2012:916198. doi: 10.1155/2012/916198
3. Association AP. Diagnostic and statistical manual of mental disorders:DSM-5. 5th ed. Washington, D.C: American Psychiatric Association (2013).
4. Laursen TM. Causes of premature mortality in schizophrenia: a review of literature published in 2018. Curr Opin Psychiatry. (2019) 32:388–93. doi: 10.1097/YCO.0000000000000530
5. Davison J, O'Gorman A, Brennan L, Cotter DR. A systematic review of metabolite biomarkers of schizophrenia. Schizophr Res. (2018) 195:32–50. doi: 10.1016/j.schres.2017.09.021
6. Holmes E, Tsang TM, Huang JT, Leweke FM, Koethe D, Gerth CW, et al. Metabolic profiling of CSF: evidence that early intervention may impact on disease progression and outcome in schizophrenia. PloS Med. (2006) 3:e327. doi: 10.1371/journal.pmed.0030327
7. Wang Y, Xu D, Liu X, Cheng M, Huang J, Liu D, et al. Discovery of potential female-specific biomarkers for major depressive disorder by LC-MS-based metabolomics. J Pharm BioMed Anal. (2024) 254:116572. doi: 10.1016/j.jpba.2024.116572
8. Pan S, Yin L, Liu J, Tong J, Wang Z, Zhao J, et al. Metabolomics-driven approaches for identifying therapeutic targets in drug discovery. MedComm (2020). (2024) 5:e792. doi: 10.1002/mco2.792
9. Wang J, Dong P, Zheng S, Mai Y, Ding J, Pan P, et al. Advances in gut microbiome in metabonomics perspective: based on bibliometrics methods and visualization analysis. Front Cell Infect Microbiol. (2023) 13:1196967. doi: 10.3389/fcimb.2023.1196967
10. Lumbreras B, Porta M, Marquez S, Pollan M, Parker LA, Hernandez-Aguado I. QUADOMICS: an adaptation of the quality assessment of diagnostic accuracy assessment (QUADAS) for the evaluation of the methodological quality of studies on the diagnostic accuracy of '-omics'-based technologies. Clin Biochem. (2008) 41:1316–25. doi: 10.1016/j.clinbiochem.2008.06.018
11. Hayden JA, van der Windt DA, Cartwright JL, Cote P, Bombardier C. Assessing bias in studies of prognostic factors. Ann Intern Med. (2013) 158:280–6. doi: 10.7326/0003-4819-158-4-201302190-00009
12. Balonov I, Mattis M, Jarmusch S, Koletzko B, Heinrich K, Neumann J, et al. Metabolomic profiling of upper GI Malignancies in blood and tissue: a systematic review and meta-analysis. J Cancer Res Clin Oncol. (2024) 150:331. doi: 10.1007/s00432-024-05857-5
13. Xuan J, Pan G, Qiu Y, Yang L, Su M, Liu Y, et al. Metabolomic profiling to identify potential serum biomarkers for schizophrenia and risperidone action. J Proteome Res. (2011) 10:5433–43. doi: 10.1021/pr2006796
14. Song M, Liu Y, Zhou J, Shi H, Su X, Shao M, et al. Potential plasma biomarker panels identification for the diagnosis of first-episode schizophrenia and monitoring antipsychotic monotherapy with the use of metabolomics analyses. Psychiatry Res. (2023) 321:115070. doi: 10.1016/j.psychres.2023.115070
15. Okamoto N, Ikenouchi A, Watanabe K, Igata R, Fujii R, Yoshimura R. A metabolomics study of serum in hospitalized patients with chronic schizophrenia. Front Psychiatry. (2021) 12:763547. doi: 10.3389/fpsyt.2021.763547
16. Ye J, Chen H, Wang Y, Chen H, Huang J, Yang Y, et al. A preliminary metabolomics study of the database for biological samples of schizophrenia among chinese ethnic minorities. BMC Psychiatry. (2024) 24:262. doi: 10.1186/s12888-024-05660-z
17. Koike S, Bundo M, Iwamoto K, Suga M, Kuwabara H, Ohashi Y, et al. A snapshot of plasma metabolites in first-episode schizophrenia: a capillary electrophoresis time-of-flight mass spectrometry study. Transl Psychiatry. (2014) 4:e379. doi: 10.1038/tp.2014.19
18. Cui G, Qing Y, Li M, Sun L, Zhang J, Feng L, et al. Salivary metabolomics reveals that metabolic alterations precede the onset of schizophrenia. J Proteome Res. (2021) 20:5010–23. doi: 10.1021/acs.jproteome.1c00504
19. Liu Y, Song X, Liu X, Pu J, Gui S, Xu S, et al. Alteration of lipids and amino acids in plasma distinguish schizophrenia patients from controls: a targeted metabolomics study. Psychiatry Clin Neurosci. (2021) 75:138–44. doi: 10.1111/pcn.13194
20. Wang X, Xie J, Ma H, Li G, Li M, Li S, et al. The relationship between alterations in plasma metabolites and treatment responses in antipsychotic-naïve female patients with schizophrenia. World J Biol Psychiatry. (2024) 25:106–15. doi: 10.1080/15622975.2023.2271965
21. Wang DF, Sun XY, Yan JJ, Ren BA, Cao B, Lu QB, et al. Alterations of eicosanoids and related mediators in patients with schizophrenia. J Psychiatr Res. (2018) 102:168–78. doi: 10.1016/j.jpsychires.2018.04.002
22. Liu P, Jing Y, Collie ND, Dean B, Bilkey DK, Zhang H. Altered brain arginine metabolism in schizophrenia. Transl Psychiatry. (2016) 6:e871. doi: 10.1038/tp.2016.144
23. Yao JK, Dougherty GG, Reddy RD, Keshavan MS, Montrose DM, Matson WR, et al. Altered interactions of tryptophan metabolites in first-episode neuroleptic-naive patients with schizophrenia. Mol Psychiatry. (2010) 15:938–53. doi: 10.1038/mp.2009.33
24. Mednova IA, Chernonosov AA, Kasakin MF, Kornetova EG, Semke AV, Bokhan NA, et al. Amino acid and acylcarnitine levels in chronic patients with schizophrenia: a preliminary study. Metabolites. (2021) 11:1–11. doi: 10.3390/metabo11010034
25. Wang T, Li P, Meng X, Zhang J, Liu Q, Jia C, et al. An integrated pathological research for precise diagnosis of schizophrenia combining LC-MS/(1)h NMR metabolomics and transcriptomics. Clin Chim Acta. (2022) 524:84–95. doi: 10.1016/j.cca.2021.11.028
26. Costa AC, Riça LB, van de Bilt M, Zandonadi FS, Gattaz WF, Talib LL, et al. Application of lipidomics in psychiatry: plasma-based potential biomarkers in schizophrenia and bipolar disorder. Metabolites. (2023) 13:600–14. doi: 10.3390/metabo13050600
27. Krzyściak W, Bystrowska B, Karcz P, Chrzan R, Bryll A, Turek A, et al. Association of blood metabolomics biomarkers with brain metabolites and patient-reported outcomes as a new approach in individualized diagnosis of schizophrenia. Int J Mol Sci. (2024) 25:2294–323. doi: 10.3390/ijms25042294
28. Ward KM, Yeoman L, Mchugh C, Kraal AZ, Flowers SA, Rothberg AE, et al. Atypical antipsychotic exposure may not differentiate metabolic phenotypes of patients with schizophrenia. Pharmacotherapy. (2018) 38:638–50. doi: 10.1002/phar.2119
29. Wang DF, Sun XY, Maziade M, Mao W, Zhang CB, Wang JY, et al. Characterising phospholipids and free fatty acids in patients with schizophrenia: a case-control study. World J Biol Psychiatry. (2021) 22:161–74. doi: 10.1080/15622975.2020.1769188
30. Cao B, Wang DF, Pan ZH, Brietzke E, Mcintyre RS, Musial N, et al. Characterizing acyl-carnitine biosignatures for schizophrenia: a longitudinal pre- and post-treatment study. Transl Psychiatry. (2019) 9:19–31. doi: 10.1038/s41398-018-0353-x
31. Cao B, Wang DF, Brietzke E, Mcintyre RS, Pan ZH, Cha D, et al. Characterizing amino-acid biosignatures amongst individuals with schizophrenia: a case-control study. Amino Acids. (2018) 50:1013–23. doi: 10.1007/s00726-018-2579-6
32. Ji E, Weickert CS, Purves-Tyson T, White C, Handelsman DJ, Desai R, et al. Cortisol-dehydroepiandrosterone ratios are inversely associated with hippocampal and prefrontal brain volume in schizophrenia. Psychoneuroendocrinology. (2021) 123:104916–23. doi: 10.1016/j.psyneuen.2020.104916
33. Bicikova M, Hill M, Ripova D, Mohr P, Hampl R. Determination of steroid metabolome as a possible tool for laboratory diagnosis of schizophrenia. J Steroid Biochem Mol Biol. (2013) 133:77–83. doi: 10.1016/j.jsbmb.2012.08.009
34. Liu ML, Zheng P, Liu Z, Xu Y, Mu J, Guo J, et al. GC-MS based metabolomics identification of possible novel biomarkers for schizophrenia in peripheral blood mononuclear cells. Mol Biosyst. (2014) 10:2398–406. doi: 10.1039/c4mb00157e
35. Su Q, Bi F, Yang S, Yan H, Sun X, Wang J, et al. Identification of plasma biomarkers in drug-naïve schizophrenia using targeted metabolomics. Psychiatry Investig. (2023) 20:818–25. doi: 10.30773/pi.2023.0121
36. Yin B, Cai Y, Teng T, Wang X, Liu X, Li X, et al. Identifying plasma metabolic characteristics of major depressive disorder, bipolar disorder, and schizophrenia in adolescents. Transl Psychiatry. (2024) 14:163. doi: 10.1038/s41398-024-02886-z
37. Fujii T, Hattori K, Miyakawa T, Ohashi Y, Sato H, Kunugi H. Metabolic profile alterations in the postmortem brains of patients with schizophrenia using capillary electrophoresis-mass spectrometry. Schizophr Res. (2017) 183:70–4. doi: 10.1016/j.schres.2016.11.011
38. Cao B, Wang D, Pan Z, Mcintyre RS, Brietzke E, Subramanieapillai M, et al. Metabolic profiling for water-soluble metabolites in patients with schizophrenia and healthy controls in a chinese population: a case-control study. World J Biol Psychiatry. (2020) 21:357–67. doi: 10.1080/15622975.2019.1615639
39. Wang D, Cheng SL, Fei Q, Gu H, Raftery D, Cao B, et al. Metabolic profiling identifies phospholipids as potential serum biomarkers for schizophrenia. Psychiatry Res. (2019) 272:18–29. doi: 10.1016/j.psychres.2018.12.008
40. Orešič M, Tang J, Seppänen-Laakso T, Mattila I, Saarni SE, Saarni SI, et al. Metabolome in schizophrenia and other psychotic disorders: a general population-based study. Genome Med. (2011) 3:19–32. doi: 10.1186/gm233
41. Du Y, Chen L, Li XS, Li XL, Xu XD, Tai SB, et al. Metabolomic identification of exosome-derived biomarkers for schizophrenia: a large multicenter study. Schizophr Bull. (2021) 47:615–23. doi: 10.1093/schbul/sbaa166
42. Tasic L, Pontes J, Carvalho MS, Cruz G, Dal Mas C, Sethi S, et al. Metabolomics and lipidomics analyses by (1)h nuclear magnetic resonance of schizophrenia patient serum reveal potential peripheral biomarkers for diagnosis. Schizophr Res. (2017) 185:182–9. doi: 10.1016/j.schres.2016.12.024
43. Liu L, Zhao J, Chen Y, Feng R. Metabolomics strategy assisted by transcriptomics analysis to identify biomarkers associated with schizophrenia. Anal Chim Acta. (2020) 1140:18–29. doi: 10.1016/j.aca.2020.09.054
44. Wang SP, Jiang XJ, Ding R, Chen BB, Lyu HY, Liu JY, et al. MS-IDF: a software tool for nontargeted identification of endogenous metabolites after chemical isotope labeling based on a narrow mass defect filter. Anal Chem. (2022) 94:3194–202. doi: 10.1021/acs.analchem.1c04719
45. Wang Z, Yuan X, Zhu Z, Pang L, Ding S, Li X, et al. Multiomics analyses reveal microbiome-gut-brain crosstalk centered on aberrant gamma-aminobutyric acid and tryptophan metabolism in drug-naïve patients with first-episode schizophrenia. Schizophr Bull. (2024) 50:187–98. doi: 10.1093/schbul/sbad026
46. Fan Y, Gao Y, Ma Q, Yang Z, Zhao B, He X, et al. Multi-omics analysis reveals aberrant gut-metabolome-immune network in schizophrenia. Front Immunol. (2022) 13:812293. doi: 10.3389/fimmu.2022.812293
47. Al AK, Haußleiter IS, Dudley E, Donev R, Brüne M, Juckel G, et al. Multiplatform metabolome and proteome profiling identifies serum metabolite and protein signatures as prospective biomarkers for schizophrenia. J Neural Transm (Vienna). (2015) 122 Suppl 1:S111–22. doi: 10.1007/s00702-014-1224-0
48. Tasic L, Larcerda A, Pontes J, Da CT, Nani JV, Martins LG, et al. Peripheral biomarkers allow differential diagnosis between schizophrenia and bipolar disorder. J Psychiatr Res. (2019) 119:67–75. doi: 10.1016/j.jpsychires.2019.09.009
49. Qiao Y, Zhang L, He S, Wen H, Yu YM, Cao CH, et al. Plasma metabonomics study of first-episode schizophrenia treated with olanzapine in female patients. Neurosci Lett. (2016) 617:270–6. doi: 10.1016/j.neulet.2016.02.031
50. Fukushima T, Iizuka H, Yokota A, Suzuki T, Ohno C, Kono Y, et al. Quantitative analyses of schizophrenia-associated metabolites in serum: serum d-lactate levels are negatively correlated with gamma-glutamylcysteine in medicated schizophrenia patients. PloS One. (2014) 9:e101652. doi: 10.1371/journal.pone.0101652
51. He Y, Yu Z, Giegling I, Xie L, Hartmann AM, Prehn C, et al. Schizophrenia shows a unique metabolomics signature in plasma. Transl Psychiatry. (2012) 2:e149. doi: 10.1038/tp.2012.76
52. Kageyama Y, Kasahara T, Morishita H, Mataga N, Deguchi Y, Tani M, et al. Search for plasma biomarkers in drug-free patients with bipolar disorder and schizophrenia using metabolome analysis. Psychiatry Clin Neurosci. (2017) 71:115–23. doi: 10.1111/pcn.12461
53. Yang X, Sun L, Zhao A, Hu X, Qing Y, Jiang J, et al. Serum fatty acid patterns in patients with schizophrenia: a targeted metabonomics study. Transl Psychiatry. (2017) 7:e1176. doi: 10.1038/tp.2017.152
54. Cui G, Qing Y, Hu X, Wang P, Sun L, Yang X, et al. Serum metabolomic profiling based on fourier transform-ion cyclotron resonance-mass spectrometry: do the dysfunctions of metabolic pathways reveal a universal risk of oxidative stress in schizophrenia? Antioxid Redox Signal. (2020) 33:679–88. doi: 10.1089/ars.2020.8141
55. Liu ML, Zhang XT, Du XY, Fang Z, Liu Z, Xu Y, et al. Severe disturbance of glucose metabolism in peripheral blood mononuclear cells of schizophrenia patients: a targeted metabolomic study. J Transl Med. (2015) 13:226. doi: 10.1186/s12967-015-0540-y
56. Qing Y, Wang P, Cui G, Zhang J, Liang K, Xia Z, et al. Targeted metabolomics reveals aberrant profiles of serum bile acids in patients with schizophrenia. Schizophrenia. (2022) 8. doi: 10.1038/s41537-022-00273-5
57. Yan H, Li G, Zhang X, Zhang C, Li M, Qiu Y, et al. Targeted metabolomics-based understanding of the sleep disturbances in drug-naive patients with schizophrenia. BMC Psychiatry. (2024) 24:355. doi: 10.1186/s12888-024-05805-0
58. Shi M, Du X, Jia Y, Zhang Y, Jia Q, Zhang X, et al. The identification of novel schizophrenia-related metabolites using untargeted lipidomics. Cereb Cortex. (2024) 34. doi: 10.1093/cercor/bhae160
59. Yan L, Zhou J, Wang D, Si D, Liu Y, Zhong L, et al. Unbiased lipidomic profiling reveals metabolomic changes during the onset and antipsychotics treatment of schizophrenia disease. Metabolomics. (2018) 14:80. doi: 10.1007/s11306-018-1375-3
60. Liu H, Huang Z, Zhang X, He Y, Gu S, Mo D, et al. Association between lipid metabolism and cognitive function in patients with schizophrenia. Front Psychiatry. (2022) 13:1013698. doi: 10.3389/fpsyt.2022.1013698
61. Jiang Y, Sun X, Hu M, Zhang L, Zhao N, Shen Y, et al. Plasma metabolomics of schizophrenia with cognitive impairment: a pilot study. Front Psychiatry. (2022) 13:950602. doi: 10.3389/fpsyt.2022.950602
62. Chen X, Xu J, Tang J, Dai X, Huang H, Cao R, et al. Dysregulation of amino acids and lipids metabolism in schizophrenia with violence. BMC Psychiatry. (2020) 20:97. doi: 10.1186/s12888-020-02499-y
63. Zhang K, Wu S, Wang Y, Zhou Y, Guo J, Xiao A, et al. Histidine metabolic pathway contributes to clozapine-induced sialorrhea based on nontargeted metabolomics. Neuropsychobiology. (2023) 82:271–86. doi: 10.1159/000528807
64. Krishnamurthy D, Harris LW, Levin Y, Koutroukides TA, Rahmoune H, Pietsch S, et al. Metabolic, hormonal and stress-related molecular changes in post-mortem pituitary glands from schizophrenia subjects. World J Biol Psychiatry. (2013) 14:478–89. doi: 10.3109/15622975.2011.601759
65. Osman E, Mohammad ZA, Sharip S, Md IZ, Tan JK. Metabolomic profiling reveals common metabolic alterations in plasma of patients with toxoplasma infection and schizophrenia. Genes (Basel). (2022) 13. doi: 10.3390/genes13081482
66. Li X, Yang C, Liang X, Li D, Zhou Z, Xiao H, et al. Metabolomics and cytokine analysis for identification of schizophrenia with auditory hallucination. Clin Invest Med. (2022) 45:E39–48. doi: 10.25011/cim.v45i2.38096
67. Messinis A, Panteli E, Paraskevopoulou A, Zymarikopoulou A, Filiou MD. Altered lipidomics biosignatures in schizophrenia: a systematic review. Schizophr Res. (2024) 271:380–90. doi: 10.1016/j.schres.2024.06.043
68. Zinellu A, Tommasi S, Carru C, Sotgia S, Mangoni AA. A systematic review and meta-analysis of nitric oxide-associated arginine metabolites in schizophrenia. Transl Psychiatry. (2024) 14:439. doi: 10.1038/s41398-024-03157-7
69. Hsu M, Ouyang W. A systematic review of effectiveness of omega-3 fatty acid supplementation on symptoms, social functions, and neurobiological variables in schizophrenia. Biol Res Nurs. (2021) 23:723–37. doi: 10.1177/10998004211020121
70. Cao B, Chen Y, Ren Z, Pan Z, Mcintyre RS, Wang D. Dysregulation of kynurenine pathway and potential dynamic changes of kynurenine in schizophrenia: a systematic review and meta-analysis. Neurosci Biobehav Rev. (2021) 123:203–14. doi: 10.1016/j.neubiorev.2021.01.018
71. Miyajima M. Amino acids: key sources for immunometabolites and immunotransmitters. Int Immunol. (2020) 32:435–46. doi: 10.1093/intimm/dxaa019
72. Calabrese V, Mancuso C, Calvani M, Rizzarelli E, Butterfield DA, Stella AMG. Nitric oxide in the central nervous system: neuroprotection versus neurotoxicity. Nat Rev Neurosci. (2007) 8:766–75. doi: 10.1038/nrn2214
73. Majumdar R, Barchi B, Turlapati SA, Gagne M, Minocha R, Long S, et al. Glutamate, ornithine, arginine, proline, and polyamine metabolic interactions: the pathway is regulated at the post-transcriptional level. Front Plant Sci. (2016) 7:78. doi: 10.3389/fpls.2016.00078
74. Qian Y, Zhou F, Chen Q, Dong F, Xu H, Sun Y, et al. Arginine alleviates LPS-induced leukocytes inflammation and apoptosis via adjusted NODs signaling. Fish Shellfish Immunol. (2024) 154:109985. doi: 10.1016/j.fsi.2024.109985
75. Yan W, Liang Y, Zhang Q, Wang D, Lei M, Qu J, et al. Arginine methylation of SIRT7 couples glucose sensing with mitochondria biogenesis. EMBO Rep. (2018) 19. doi: 10.15252/embr.201846377
76. Mondanelli G, Iacono A, Allegrucci M, Puccetti P, Grohmann U. Immunoregulatory interplay between arginine and tryptophan metabolism in health and disease. Front Immunol. (2019) 10:1565. doi: 10.3389/fimmu.2019.01565
77. Ovalle Rodriguez P, Ramirez Ortega D, Blanco Ayala T, Roldan Roldan G, Perez de la Cruz G, Gonzalez Esquivel DF, et al. Modulation of kynurenic acid production by n-acetylcysteine prevents cognitive impairment in adulthood induced by lead exposure during lactation in mice. Antioxid (Bassel). (2023) 12. doi: 10.3390/antiox12122035
78. Markovic M, Petronijevic N, Stasevic M, Stasevic Karlicic I, Velimirovic M, Stojkovic T, et al. Decreased plasma levels of kynurenine and kynurenic acid in previously treated and first-episode antipsychotic-naive schizophrenia patients. Cells. (2023) 12. doi: 10.3390/cells12242814
79. Hare SM, Adhikari BM, Mo C, Chen S, Wijtenburg SA, Seneviratne C, et al. Tryptophan challenge in individuals with schizophrenia and healthy controls: acute effects on circulating kynurenine and kynurenic acid, cognition and cerebral blood flow. Neuropsychopharmacology. (2023) 48:1594–601. doi: 10.1038/s41386-023-01587-3
80. Sathyasaikumar KV, Notarangelo FM, Kelly DL, Rowland LM, Hare SM, Chen S, et al. Tryptophan challenge in healthy controls and people with schizophrenia: acute effects on plasma levels of kynurenine, kynurenic acid and 5-hydroxyindoleacetic acid. Pharm (Basel). (2022) 15. doi: 10.3390/ph15081003
81. Huang J, Tong J, Zhang P, Zhou Y, Li Y, Tan S, et al. Elevated salivary kynurenic acid levels related to enlarged choroid plexus and severity of clinical phenotypes in treatment-resistant schizophrenia. Brain Behav Immun. (2022) 106:32–9. doi: 10.1016/j.bbi.2022.08.001
82. Wagh VV, Vyas P, Agrawal S, Pachpor TA, Paralikar V, Khare SP. Peripheral blood-based gene expression studies in schizophrenia: a systematic review. Front Genet. (2021) 12:736483. doi: 10.3389/fgene.2021.736483
83. Burke L, Guterman I, Palacios Gallego R, Britton RG, Burschowsky D, Tufarelli C, et al. The janus-like role of proline metabolism in cancer. Cell Death Discovery. (2020) 6:104. doi: 10.1038/s41420-020-00341-8
84. Qin X, Chen J, Zhou T. 22q11.2 deletion syndrome and schizophrenia. Acta Biochim Biophys Sin (Shanghai). (2020) 52:1181–90. doi: 10.1093/abbs/gmaa113
85. Yao Y, Jin C, Liao Y, Huang X, Wei Z, Zhang Y, et al. Schizophrenia-like behaviors arising from dysregulated proline metabolism are associated with altered neuronal morphology and function in mice with hippocampal PRODH deficiency. Aging Dis. (2024) 15:1952–68. doi: 10.14336/AD.2023.0902
86. Crabtree GW, Gogos JA. Role of endogenous metabolite alterations in neuropsychiatric disease. ACS Chem Neurosci. (2018) 9:2101–13. doi: 10.1021/acschemneuro.8b00145
87. Small SA, Schobel SA, Buxton RB, Witter MP, Barnes CA. A pathophysiological framework of hippocampal dysfunction in ageing and disease. Nat Rev Neurosci. (2011) 12:585–601. doi: 10.1038/nrn3085
88. Provenzano FA, Guo J, Wall MM, Feng X, Sigmon HC, Brucato G, et al. Hippocampal pathology in clinical high-risk patients and the onset of schizophrenia. Biol Psychiatry. (2020) 87:234–42. doi: 10.1016/j.biopsych.2019.09.022
89. Marenco S, Meyer C, Kuo S, van der Veen JW, Shen J, Dejong K, et al. Prefrontal GABA levels measured with magnetic resonance spectroscopy in patients with psychosis and unaffected siblings. Am J Psychiatry. (2016) 173:527–34. doi: 10.1176/appi.ajp.2015.15020190
90. Adams RA, Pinotsis D, Tsirlis K, Unruh L, Mahajan A, Horas AM, et al. Computational modeling of electroencephalography and functional magnetic resonance imaging paradigms indicates a consistent loss of pyramidal cell synaptic gain in schizophrenia. Biol Psychiatry. (2022) 91:202–15. doi: 10.1016/j.biopsych.2021.07.024
91. Fan L, Zhang Z, Ma X, Liang L, Wang Y, Yuan L, et al. Glutamate levels and symptom burden in high-risk and first-episode schizophrenia: a dual-voxel study of the anterior cingulate cortex. J Psychiatry Neurosci. (2024) 49:E367–76. doi: 10.1503/jpn.240094
92. Yan H, Li G, Zhang X, Zhang C, Li M, Qiu Y, et al. Targeted metabolomics-based understanding of the sleep disturbances in drug-naïve patients with schizophrenia. BMC Psychiatry. (2024) 24:355. doi: 10.1186/s12888-024-05805-0
93. Zheng J, Lin Z, Ko C, Xu J, Lin Y, Wang J. Analysis of gut microbiota in patients with exacerbated symptoms of schizophrenia following therapy with amisulpride: a pilot study. Behav Neurol. (2022) 2022:4262094. doi: 10.1155/2022/4262094
94. Zhao Y, Lai Y, Darweesh SKL, Bloem BR, Forsgren L, Hansen J, et al. Gut microbial metabolites and future risk of parkinson's disease: a metabolome-wide association study. Mov Disord. (2024). doi: 10.1002/mds.30054
Keywords: schizophrenia, metabolomics, metabolite, pathway enrichment, systematic review
Citation: Yao G, Zeng J, Huang Y, Lu H, Ping J, Wan J, Jiang T, Deng F, Li C, Liu X, Tang C and Lu L (2025) Discovery of biological markers for schizophrenia based on metabolomics: a systematic review. Front. Psychiatry 16:1540260. doi: 10.3389/fpsyt.2025.1540260
Received: 05 December 2024; Accepted: 27 February 2025;
Published: 28 March 2025.
Edited by:
Gianluca Baldanzi, Università degli Studi del Piemonte Orientale, ItalyReviewed by:
Martina Di Bartolomeo, Università degli studi di Teramo, ItalyCopyright © 2025 Yao, Zeng, Huang, Lu, Ping, Wan, Jiang, Deng, Li, Liu, Tang and Lu. This is an open-access article distributed under the terms of the Creative Commons Attribution License (CC BY). The use, distribution or reproduction in other forums is permitted, provided the original author(s) and the copyright owner(s) are credited and that the original publication in this journal is cited, in accordance with accepted academic practice. No use, distribution or reproduction is permitted which does not comply with these terms.
*Correspondence: Xinxia Liu, c3lsaXV4aW54aWFAMTYzLmNvbQ==; Chunzhi Tang, am9yZGFuNjY0QDE2My5jb20=; Liming Lu, bHVsaW1pbmdsZW9uQDEyNi5jb20=
†These authors have contributed equally to this work
Disclaimer: All claims expressed in this article are solely those of the authors and do not necessarily represent those of their affiliated organizations, or those of the publisher, the editors and the reviewers. Any product that may be evaluated in this article or claim that may be made by its manufacturer is not guaranteed or endorsed by the publisher.
Research integrity at Frontiers
Learn more about the work of our research integrity team to safeguard the quality of each article we publish.