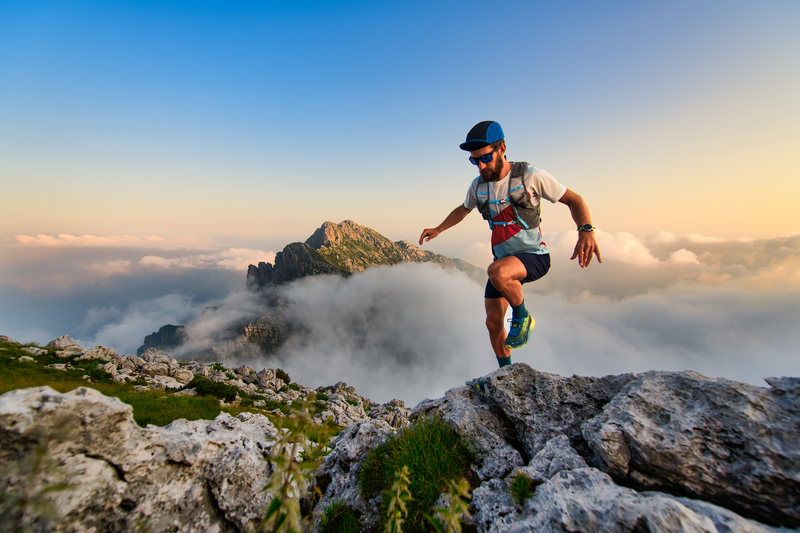
94% of researchers rate our articles as excellent or good
Learn more about the work of our research integrity team to safeguard the quality of each article we publish.
Find out more
REVIEW article
Front. Psychiatry
Sec. Molecular Psychiatry
Volume 16 - 2025 | doi: 10.3389/fpsyt.2025.1452557
This article is part of the Research Topic Advances, Opportunities and Challenges of Using Modern AGI and AIGC Technologies in Depression and Related Disorders View all 4 articles
The final, formatted version of the article will be published soon.
You have multiple emails registered with Frontiers:
Please enter your email address:
If you already have an account, please login
You don't have a Frontiers account ? You can register here
Neurological disorders (e.g., Alzheimer's disease and Parkinson's disease) and mental disorders (e.g., depression and anxiety), pose huge challenges to global public health. The pathogenesis of these diseases can usually be attributed to many factors, such as genetic, environmental and socioeconomic status, which make the diagnosis and treatment of the diseases difficult. As research on the diseases advances, so does the body of medical data. The accumulation of such data provides unique opportunities for the basic and clinical study of these diseases, but the vast and diverse nature of the data also make it difficult for physicians and researchers to precisely extract the information and utilize it in their work. A powerful tool to extract the necessary knowledge from large amounts of data is knowledge graph (KG). KG, as an organized form of information, has great potential for the study neurological and mental disorders when it is paired with big data and deep learning technologies. In this study, we reviewed the application of KGs in common neurological and mental disorders in recent years. We also discussed the current state of medical knowledge graphs, highlighting the obstacles and constraints that still need to be overcome.
Keywords: neurological disorders, Mental Disorders, knowledge graph, Alzheimer's disease, Parkinson's disease, Depression
Received: 21 Jun 2024; Accepted: 28 Feb 2025.
Copyright: © 2025 Wang, Yang, Quan, Fu, Yang and Wang. This is an open-access article distributed under the terms of the Creative Commons Attribution License (CC BY). The use, distribution or reproduction in other forums is permitted, provided the original author(s) or licensor are credited and that the original publication in this journal is cited, in accordance with accepted academic practice. No use, distribution or reproduction is permitted which does not comply with these terms.
* Correspondence:
Ju Wang, Tianjin Medical University, Tianjin, China
Disclaimer: All claims expressed in this article are solely those of the authors and do not necessarily represent those of their affiliated organizations, or those of the publisher, the editors and the reviewers. Any product that may be evaluated in this article or claim that may be made by its manufacturer is not guaranteed or endorsed by the publisher.
Research integrity at Frontiers
Learn more about the work of our research integrity team to safeguard the quality of each article we publish.