- 1Department of Psychiatry, University of California, Davis, Davis, CA, United States
- 2Department of Psychology, Yale University, New Haven, CT, United States
- 3Department of Psychiatry, Yale University, New Haven, CT, United States
- 4Department of Psychiatry, Semel Institute for Neuroscience and Human Behavior, University of California, Los Angeles, Los Angeles, CA, United States
- 5Biobehavioral Sciences and Psychology, Semel Institute for Neuroscience and Human Behavior, University of California, Los Angeles, Los Angeles, CA, United States
- 6Department of Psychiatry, University of Calgary, Calgary, AB, Canada
- 7Department of Psychiatry, University of North Carolina Chapel Hill, Chapel Hill, NC, United States
- 8Department of Psychiatry Research, Zucker Hillside Hospital, New York, NY, United States
- 9Department of Psychiatry, Harvard University, Cambridge, MA, United States
- 10Department of Psychiatry, University of California, San Francisco, San Francisco, CA, United States
- 11Department of Psychiatry, University of San Diego, San Diego, CA, United States
- 12Department of Psychiatry, Emory University, Atlanta, GA, United States
- 13Department of Computer Science, University of California, Davis, Davis, CA, United States
- 14Department of Psychiatry, University of California, Irvine, Irvine, CA, United States
Background: We previously reported that machine learning could be used to predict conversion to psychosis in individuals at clinical high risk (CHR) for psychosis with up to 90% accuracy using the North American Prodrome Longitudinal Study-3 (NAPLS-3) dataset. A definitive test of our predictive model that was trained on the NAPLS-3 data, however, requires further support through implementation in an independent dataset. In this report we tested for model generalization using the previous iteration of NAPLS-3, the NAPLS-2, using the identical machine learning algorithms employed in our previous study.
Method: Standard machine learning algorithms were trained to predict conversion to psychosis in clinical high risk individuals on the NAPLS-3 dataset and tested on the NAPLS-2 dataset.
Results: NAPLS-2 and -3 individuals significantly differed on most features used in machine learning models. All models performed above chance, with Naive Bayes and random forest methods showing the best overall performance. Importantly, however, overall performance did not match those previously observed when using only NAPLS-3 data.
Conclusion: The results of this study suggest that a machine learning model trained to predict conversion to psychosis on one dataset can be used to train an independent dataset. Performance on the test set was not in the range necessary for clinical application, however. Possible reasons that limited performance are discussed.
Background
In a prior priority data letter in The American Journal of Psychiatry, we reported that machine learning could be used to predict conversion to psychosis in individuals at clinical high risk (CHR) for psychosis with up to 90% accuracy using the North American Prodrome Longitudinal Study-3 (NAPLS-3) dataset (1). As argued by Cannon (2), a definitive test of our predictive model that was trained on the NAPLS-3 data requires further support through implementation in an independent dataset. In this report, in collaboration with the primary investigators of the NAPLS consortium we tested for model generalization using the previous iteration of NAPLS-3, the NAPLS-2, using the identical machine learning algorithms employed in our previous study. We used the NAPLS-2 dataset as a test set because it used mostly the same measures that were used in the NAPLS-3 to predict conversion to psychosis in a similarly-aged sample.
Materials and methods
Participants
NAPLS-2 and 3 are NIMH-funded studies conducted at 8 and 9 sites, respectively. All participants provided written informed consent, including parental consent for minors. The study was approved by all sites’ Institutional Review Boards.
Detailed descriptions of NAPLS-2 and NAPLS-3 participants, including exclusion criteria, are provided in Addington et al. (3) and Addington et al. (4), respectively. Participants were between 12 and 30 years old and were followed up to 2 years. Predictors included those used by the NAPLS-2 calculator (riskcalc.org/napls, see Table 1).
Consistent with prior work (5), conversion to psychosis for a CHR individual was defined as meeting the Presence of Psychotic Symptoms criteria: One of the five Scale of Psychosis-Risk Symptoms (SOPS) positive symptoms must reach a psychotic level of intensity (rated 6) for ≥ 1 hour per day for 4 days per week during the past month, and/or these symptoms seriously impact their functioning. Machine learning models were tested with and without SOPS rescaling prior to analysis [see Table 1 legend for rescaling procedure, based on Cannon et al. (6)].
Analyses
Standard machine learning algorithms were employed using Weka software (University of Waikato, New Zealand) and included logistic regression, naive Bayes, a 3 kernel support vector machine, KStar, J48 decision tree, random forest (max depth 2), decision stump (with 100 iterations of AdaBoost), and multilayer perceptron (see explanation of algorithms below). Classifiers were trained using NAPLS-3 data and tested on NAPLS-2 data. Individuals with missing data were excluded from the analyses. Because of class imbalance, prior to machine learning training data for the minority (converter) class was 800% upsampled using the Synthetic Minority Oversampling Technique (SMOTE) (7) to match the majority (nonconverter) class. By providing more training data of the minority class, this procedure helps to refine the machine learning model decision binary and improve performance (7). SMOTE k (n nearest neighbors) was set to 5.
Naive Bayes
Naive Bayes (8) compares the probability of observing an converter/non-converter for each test data point according to the equation P (Ci | x) = (P (Ci) * p (x | Ci)/p (x) where P(Ci) is the prior probability of class Ci (e.g., converter) occurring, p (x | Ci) is the conditional probability that class Ci is associated with feature observation x, and p (x) is the marginal probability that observation x is observed (effectively constant for any given dataset). The joint model (combining all features) can then be expressed as the product of the probabilities for all features, and the algorithm classifies unseen data as converter or non-converter based on the highest probability.
Support vector machine
SVM classifiers find the maximum-margin hyperplane using only those data instances closest to the separation boundary (i.e. “support vectors”) to determine classification boundaries (9). Both linear and non-linear (using a kernel) classifications can be performed. Polykernel SVM classifiers were evaluated starting with an exponent of 1 and increasing in size until average accuracy (over all 1000 allocations of test/training data) plateaued.
KStar (K-nearest neighbor)
The K* algorithm operates by assigning new data instances to the class that occurs most frequently amongst the k-nearest data points, yj, where j = 1,2…k (10). Distance is then used to retrieve the most similar instances from the data set. The K* function is operationalized as K* (yi,x)= -ln P*(yi,x) where P* is the probability of all transformational paths from instance x to y, i.e., the probability x will arrive at y via a random walk in feature space.
AdaBoost
AdaBoost operates by creating multiple weak classifiers that are weighed by their effectiveness at classifying data (11). Initially, a classifier is created with all instances weighted equally. Next, the weights of the incorrectly predicted instances are increased. The instances that are still misclassified are then selected and their weights increased as well, and so forth. After the complete classifier is constructed, each weak classifier then casts a weighted “vote” as to the class membership of each set of individual test data to make a classification decision.
J48 decision tree
Decision tree classifiers operate hierarchically, with each level representing a feature (e.g., age) (12, 13). Based on the value of that feature the tree either classifies immediately or passes the information to the next level of the tree. The C4.5 algorithm (12) was used for the J48 decision tree, which uses a measure called “information gain” to select each attribute at each stage. In essence, the J48 tree first chooses the feature that most effectively splits the training data into one class or another using a measure called “information gain” (essentially, the effectiveness of feature at classifying data). After this split, the tree then chooses the next most effective feature to split each resulting partition. The process then iteratively repeats until all training data is classified. Performance of the resulting tree is then evaluated on test data.
Random forest
A random forest is a group of decision trees made up of random partitions of training data (14). Each tree casts a “vote” as to the classification of a testing instance and votes are counted to produce the final classification.
Results
Demographic and clinical information for and comparisons between NAPLS-2 and NAPLS-3 participants are provided in Table 1. NAPLS-2 and -3 individuals significantly differed on most features used in machine learning models. Examining conversion rates, 84 out of the 596 CHR individuals in NAPLS-2 (14%) and 62 out of the 598 CHR participants in NAPLS-3 (10%) with no missing data converted over the course of the follow-up period.
Machine learning performance metrics for each machine learning algorithm are provided in Table 2. Briefly, all models performed above chance as evidenced by concordance values [receiver operating characteristic area under the curve (ROC AUC)] values over 50%. Naive Bayes and random forest showed the best overall performance (AUC). Sensitivity and negative predictive values were relatively high and specificity and positive predictive values were relatively low for all models, however. Importantly, overall performance did not match those previously observed when using only NAPLS-3 data (performance metrics from the previous study are also provided in Table 2). For example, when exclusively using NAPLS-3 data (including non-rescaled SIPS scores) for training and testing, the most accurate method (random forest) showed 90% accuracy with 79% sensitivity and 96% specificity (1). When a NAPLS-3-based random forest model was tested on NAPLS-2 data, however, accuracy, sensitivity, and specificity were reduced to 77%, 41%, and 83% (respectively) (Table 2, bottom half).
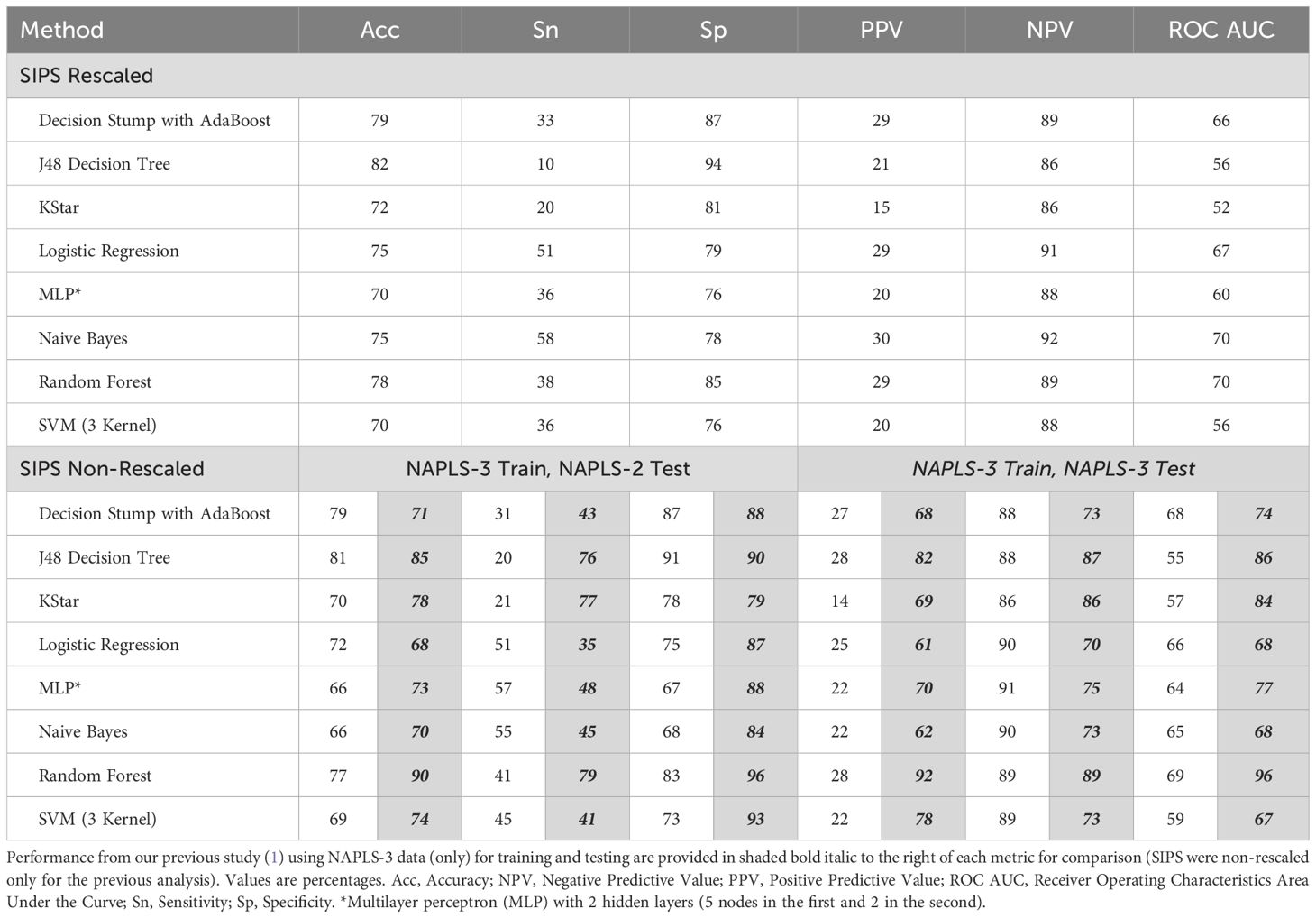
Table 2. Predicting conversion to psychosis using NAPLS-3 clinical/demographic data for training and NAPLS-2 data for testing using various machine learning methods.
Discussion
The results of this study suggest that a machine learning model trained to predict conversion to psychosis on one dataset can be used to train an independent dataset. In this analysis, however, we did not achieve the high level of accuracy as in the training sample and classification success was not in the range generally considered necessary for clinical application (80% positive and negative predictive value). Notably most models in this generalization analysis struggled to identify positive cases (converters), the minority outcome in both samples, although they did show high specificity and AUC scores that were above chance.
One likely reason for the discrepancy in performance is that models that exclusively use NAPLS-3 data may have overfit to that dataset. Chekroud et al. (15) have recently emphasized that machine learning faces a generalizability issue in outcome prediction in studies of psychiatric disorders in that models often do not perform well when tested on unseen data. Consistent with our findings, recent prior work that also sought to predict conversion to psychosis in CHRs observed a significant reduction in performance (from 85% accuracy on the trained sample to 73% on the independent test sample) when a trained model was tested on an independent dataset (16). Although all algorithms here did perform above chance, the fact that they did not perform as well as a model trained within one dataset as in our previous study is in conceptual agreement with the findings of Chekroud et al. (15). An additional potential reason for poor performance is that the assumption of stationarity, core to machine learning methods including random forest, was not met since the levels of key predictor variables differed significantly across the two samples (17) (as shown in Table 1). This presents a significant challenge for researchers seeking to develop generalizable prediction tools using machine learning approaches. One approach to improving generalizability that machine learning researchers are actively investigating is the application of domain adaptation methods, to apply in cases in which source and target domains have different distributions but identical underlying predictive features (18). As recently reviewed by Smucny et al. (19), domain adaptation methods have achieved some success when using magnetic resonance imaging data to predict various outcomes, although only a few studies thus far have been conducted and domain adaptation procedures are still being developed and refined. Finally, it should be noted that the power of the model may have been limited by the relatively low sample size of the minority class in the training dataset (n = 62). Hence, while we are not “there yet” (1), we believe that the field should eschew nihilism regarding the application of predictive analytics to unique and powerful datasets (such as those collected by the NAPLS consortium) in pursuit of a personalized medicine approach to early intervention for young people with psychosis.
Data availability statement
The data analyzed in this study is subject to the following licenses/restrictions: The NAPLS-2 dataset is only available to NAPLS-2 investigators and their collaborators. Requests to access these datasets should be directed to dHlyb25lLmNhbm5vbkB5YWxlLmVkdQ==. NAPLS-3 data is available for download on the National Institutes of Mental Health Data Archive.
Ethics statement
Ethical approval was not required for the study involving humans in accordance with the local legislation and institutional requirements. Written informed consent to participate in this study was not required from the participants or the participants’ legal guardians/next of kin in accordance with the national legislation and the institutional requirements.
Author contributions
JS: Conceptualization, Formal analysis, Investigation, Methodology, Project administration, Resources, Validation, Visualization, Writing – original draft, Writing – review & editing. TC: Data curation, Funding acquisition, Resources, Writing – review & editing. CB: Data curation, Writing – review & editing. JA: Data curation, Writing – review & editing. KC: Data curation, Writing – review & editing. BC: Data curation, Writing – review & editing. MK: Data curation, Writing – review & editing. DM: Data curation, Writing – review & editing. DP: Data curation, Writing – review & editing. WS: Data curation, Writing – review & editing. EW: Data curation, Writing – review & editing. SW: Data curation, Writing – review & editing. ID: Supervision, Writing – review & editing. CC: Supervision, Writing – review & editing.
Funding
The author(s) declare financial support was received for the research, authorship, and/or publication of this article. This work was supported by NIMH grants K01-MH125096 (JS), R01-MH122139 (CC), U01-MH081902 (TC), P50-MH066286 (CB), U01-MH081857 (BC), U01-MH082022 (SW), U01-MH066134 (JA), U01-MH081944 (KC), U01-MH066069 (DP), R01-MH076989 (DM), and U01-MH081988 to (EW).
Acknowledgments
Some of the data and/or research tools used in the preparation of this manuscript were obtained from the National Institute of Mental Health (NIMH) Data Archive (NDA). NDA is a collaborative informatics system created by the National Institutes of Health to provide a national resource to support and accelerate research in mental health. Dataset identifier: Predictors and Mechanisms of Conversion to Psychosis (NAPLS3). This manuscript reflects the views of the authors and may not reflect the opinions or views of the NIH or of the Submitters submitting original data to NDA.
Conflict of interest
TC has served as a consultant for Boehringer Ingelheim and Lundbeck A/S. DM reported personal fees from Neurocrine Biosciences outside the submitted work and has served as a consultant for Aptinyx, Boehringer Ingelheim, Cadent Therapeutics, and Greenwich Biosciences. DP has served as a consultant for Sunovion and Alkermes, has received research support from Boehringer Ingelheim, and has received royalties from American Psychiatric Association Publishing. SW has received personal fees from American Psychiatric Association and Medscape outside the submitted work; had a patent for US patent no. 8492418 B2 issued; has received investigator-initiated research support from Pfizer and sponsor-initiated research support from Auspex and Teva; served as a consultant for Biomedisyn unpaid, Boehringer Ingelheim, and Merck; served as an unpaid consultant to DSM-5; and received royalties from Oxford University Press.
The remaining authors declare that the research was conducted in the absence of any commercial or financial relationships that could be construed as a potential conflict of interest.
The author(s) declared that they were an editorial board member of Frontiers, at the time of submission. This had no impact on the peer review process and the final decision.
Generative AI statement
The author(s) declare that no Generative AI was used in the creation of this manuscript.
Publisher’s note
All claims expressed in this article are solely those of the authors and do not necessarily represent those of their affiliated organizations, or those of the publisher, the editors and the reviewers. Any product that may be evaluated in this article, or claim that may be made by its manufacturer, is not guaranteed or endorsed by the publisher.
References
1. Smucny J, Davidson I, Carter CS. Are we there yet? Predicting conversion to psychosis using machine learning. Am J Psychiatry. (2023) 180:836–40. doi: 10.1176/appi.ajp.20220973
2. Cannon TD. Predicting conversion to psychosis using machine learning: are we there yet? Am J Psychiatry. (2023) 180:789–91. doi: 10.1176/appi.ajp.20220973
3. Addington J, Cadenhead KS, Cornblatt BA, Mathalon DH, Mcglashan TH, Perkins DO, et al. North American Prodrome Longitudinal Study (NAPLS 2): overview and recruitment. Schizophr Res. (2012) 142:77–82. doi: 10.1016/j.schres.2012.09.012
4. Addington J, Liu L, Brummitt K, Bearden CE, Cadenhead KS, Cornblatt BA, et al. North American Prodrome Longitudinal Study (NAPLS 3): Methods and baseline description. Schizophr Res. (2022) 243:262–7. doi: 10.1016/j.schres.2020.04.010
5. Addington J, Liu L, Buchy L, Cadenhead KS, Cannon TD, Cornblatt BA, et al. North american prodrome longitudinal study (NAPLS 2): the prodromal symptoms. J Nerv Ment Dis. (2015) 203:328–35. doi: 10.1097/NMD.0000000000000290
6. Cannon TD, Yu C, Addington J, Bearden CE, Cadenhead KS, Cornblatt BA, et al. An individualized risk calculator for research in prodromal psychosis. Am J Psychiatry. (2016) 173:980–8. doi: 10.1176/appi.ajp.2016.15070890
7. Nitesh V, Bowyer KW, Hall LO, Kegelmeyer WP. Synthetic minority over-sampling technique. J Artif Intell Res. (2002) 16:321–57. doi: 10.1613/jair.953
8. Maron ME. Automatic indexing: an experimental inquiry. J Assoc Computing Machinery. (1961) 8:404–17. doi: 10.1145/321075.321084
9. Burges C. A tutorial on support vector machines for pattern recognition. Data Min Knowledge Discovery. (1998) 2:121–67. doi: 10.1023/A:1009715923555
10. Hart PE. The condensed nearest neighbour rule. IEEE Trans Inf Theory. (1968) 14:515–6. doi: 10.1109/TIT.1968.1054155
11. Viola P, Jones M. Rapid object detection using a boosted cascade of simple features. In: International conference on Computer Vision and Pattern Recognition. New York, New York, USA: Institute of Electrical and Electronics Engineers. (2001). p. 511–8.
12. Quinlan JR. C4.5: Programs for Machine Learning. San Mateo, CA USA: Morgan Kaufmann Publishers (1993).
15. Chekroud AM, Hawrilenko M, Loho H, Bondar J, Gueorguieva R, Hasan A, et al. Illusory generalizability of clinical prediction models. Science. (2024) 383:164–7. doi: 10.1126/science.adg8538
16. Zhu Y, Maikusa N, Radua J, Samann PG, Fusar-Poli P, Agartz I, et al. Using brain structural neuroimaging measures to predict psychosis onset for individuals at clinical high-risk. Mol Psychiatry. (2024) 29:1465–77. doi: 10.1038/s41380-024-02426-7
17. Sugiyama M, Kawanabe M. Machine learning in non-stationary environments: Introduction to covariate shift adaptation. Cambridge, MA, USA: MIT Press (2012).
18. Kouw WM, Loog M. An introduction to domain adaptation and transfer learning (2018). Available online at: https://ui.adsabs.harvard.edu/abs/2018arXiv181211806K (Accessed December 01, 2018).
Keywords: schizophrenia, clinical high risk (CHR), NAPLS, out of sample evaluation, scale of psychosis risk symptoms, generalizability
Citation: Smucny J, Cannon TD, Bearden CE, Addington J, Cadenhead KS, Cornblatt BA, Keshavan M, Mathalon DH, Perkins DO, Stone W, Walker EF, Woods SW, Davidson I and Carter CS (2025) Predicting conversion to psychosis using machine learning: response to Cannon. Front. Psychiatry 15:1520173. doi: 10.3389/fpsyt.2024.1520173
Received: 30 October 2024; Accepted: 18 December 2024;
Published: 15 January 2025.
Edited by:
Jennifer Larimore, Agnes Scott College, United StatesReviewed by:
Nikolaos Smyrnis, National and Kapodistrian University of Athens, GreeceAyse Ulgen, Nottingham Trent University, United Kingdom
Copyright © 2025 Smucny, Cannon, Bearden, Addington, Cadenhead, Cornblatt, Keshavan, Mathalon, Perkins, Stone, Walker, Woods, Davidson and Carter. This is an open-access article distributed under the terms of the Creative Commons Attribution License (CC BY). The use, distribution or reproduction in other forums is permitted, provided the original author(s) and the copyright owner(s) are credited and that the original publication in this journal is cited, in accordance with accepted academic practice. No use, distribution or reproduction is permitted which does not comply with these terms.
*Correspondence: Jason Smucny, anNtdWNueUB1Y2RhdmlzLmVkdQ==