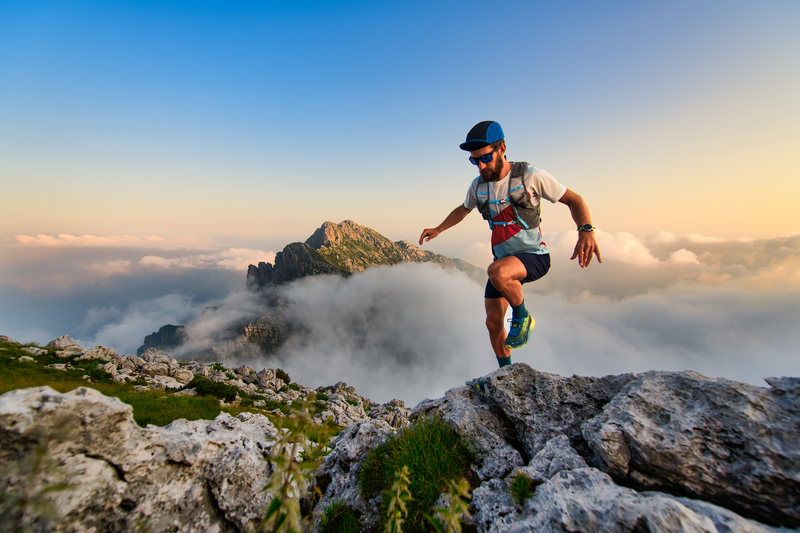
94% of researchers rate our articles as excellent or good
Learn more about the work of our research integrity team to safeguard the quality of each article we publish.
Find out more
ORIGINAL RESEARCH article
Front. Plant Sci.
Sec. Technical Advances in Plant Science
Volume 16 - 2025 | doi: 10.3389/fpls.2025.1536177
This article is part of the Research Topic Optimizing Fertilizer and Irrigation for Specialty Crops Using Precision Agriculture Technologies View all 8 articles
The final, formatted version of the article will be published soon.
You have multiple emails registered with Frontiers:
Please enter your email address:
If you already have an account, please login
You don't have a Frontiers account ? You can register here
Nondestructive quantification of leaf chlorophyll content (LCC) of banana and its spatial distribution across growth stages from remotely sensed data provide an effective avenue to diagnose nutritional deficiency and guide management practices. Unmanned aerial vehicle (UAV) hyperspectral imagery can document abundant texture features (TFs) and spectral information in field experiment due to the high spatial and spectral resolutions. However, the benefits of using the fine spatial resolution accessible from UAV data for estimating LCC for banana have not been adequately quantified. In this study, two types of image features including vegetation indices (VIs) and TFs extracted from the first three principal component analyzed images (TFs-PC1, TFs-PC2, TFs-PC3) were employed. We proposed two methods of image feature combination for banana LCC inversion, which are a two-pair feature combination and a multivariable feature combination based on four machine learning algorithms (MLRAs). The results indicated that compared to conventionally used VIs alone, the banana LCC estimated with both proposed VI and TF combination methods were all significantly improved. Comprehensive analyses of the linear relationships between all constructed two-pair feature combinations and LCC indicated that the ratio of Mean to Modified red-edge sample ratio index (MEA/MSRre) stood out (R2=0.745, RMSE=2.17). For multivariable feature combinations, four MLRAs using original or two selected VIs and TFs-PC1 combination groups resulted in better LCC estimation than other input variables. We concluded that the nonlinear Gaussian process regression model with the VIs and TFs-PC1 combination selected by maximal information coefficient as input achieved the highest accuracy in LCC prediction for banana, with the highest R2 of 0.776 and lowest RMSE of 2.04. This study highlights the potential of the proposed image feature combination method for deriving high resolution maps of banana LCC fundamental for precise nutritional diagnosing and operational agriculture management.
Keywords: leaf chlorophyll content, Banana, image feature combinations, machine learning, UAV unmanned aerial vehicle hyperspectral imagery
Received: 28 Nov 2024; Accepted: 20 Jan 2025.
Copyright: © 2025 Kong, Ma, Ye, Wang, Nie, Zhou, Huang and Fan. This is an open-access article distributed under the terms of the Creative Commons Attribution License (CC BY). The use, distribution or reproduction in other forums is permitted, provided the original author(s) or licensor are credited and that the original publication in this journal is cited, in accordance with accepted academic practice. No use, distribution or reproduction is permitted which does not comply with these terms.
* Correspondence:
Weiping Kong, Chinese Academy of Sciences (CAS), Beijing, China
Disclaimer: All claims expressed in this article are solely those of the authors and do not necessarily represent those of their affiliated organizations, or those of the publisher, the editors and the reviewers. Any product that may be evaluated in this article or claim that may be made by its manufacturer is not guaranteed or endorsed by the publisher.
Research integrity at Frontiers
Learn more about the work of our research integrity team to safeguard the quality of each article we publish.