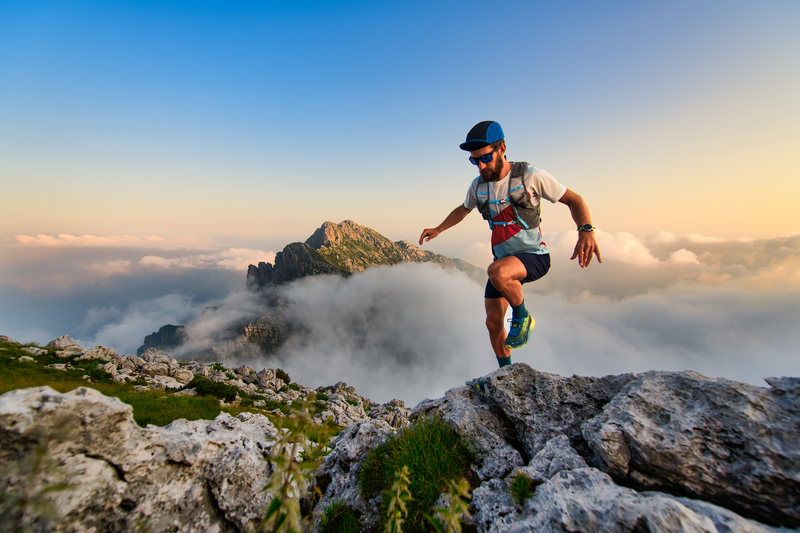
94% of researchers rate our articles as excellent or good
Learn more about the work of our research integrity team to safeguard the quality of each article we publish.
Find out more
ORIGINAL RESEARCH article
Front. Plant Sci.
Sec. Functional Plant Ecology
Volume 16 - 2025 | doi: 10.3389/fpls.2025.1521596
This article is part of the Research Topic Plant Ecophysiology: Responses to Climate Changes and Stress Conditions View all 24 articles
The final, formatted version of the article will be published soon.
You have multiple emails registered with Frontiers:
Please enter your email address:
If you already have an account, please login
You don't have a Frontiers account ? You can register here
Understanding how species traits, climate aridity, and soil resources interact to influence beta diversity is critical for predicting changes in plant community composition. This study aims to investigate how these interactions shape species contributions to spatial turnover and beta diversity, focusing on the unique dryland ecosystems of the Saint Katherine Protectorate (SKP) in Egypt. To address this, we analyzed data from 84 vegetation plots, considering the direct and indirect effects of climatic aridity, soil resources, and species traits (e.g., plant height, leaf production, specific leaf area), as well as the relative abundance of C3 plants and phylogenetic diversity on species contribution to beta diversity (SCBDeff). Using Generalized Linear Models (GLMs) and Structural Equation Modelling (SEMs), the results revealed complex indirect effects of aridity and soil resources on SCBDeff mediated by plant traits. SCBDeff was positively influenced by climatic aridity, particularly in species with greater phylogenetic distance, taller plants, high leaf production, and a higher relative abundance of C3 plants. Conversely, specific leaf area (SLA) had a negative effect. Phylogenetic diversity emerged as a significant driver of beta diversity, with distantly related species contributing more due to functional differentiation and niche partitioning. The findings emphasize the critical role of species traits and environmental conditions in shaping beta diversity. These insights can inform conservation strategies aimed at enhancing ecosystem stability under shifting climatic conditions, particularly in dryland environments where species adaptive traits play a pivotal role.
Keywords: Climatic aridity, species turnover, phylogenetic diversity, species traits, soil resources, species abundance
Received: 02 Nov 2024; Accepted: 18 Feb 2025.
Copyright: © 2025 El-barougy, Dakhil, Gray, El-Keblawy, Galal, Ullah, Elgamal and Bersier. This is an open-access article distributed under the terms of the Creative Commons Attribution License (CC BY). The use, distribution or reproduction in other forums is permitted, provided the original author(s) or licensor are credited and that the original publication in this journal is cited, in accordance with accepted academic practice. No use, distribution or reproduction is permitted which does not comply with these terms.
* Correspondence:
Reham Fekry El-barougy, University of Georgia, Athens, United States
Mohammed A. Dakhil, Helwan University, Helwan, 11792, Cairo, Egypt
Disclaimer: All claims expressed in this article are solely those of the authors and do not necessarily represent those of their affiliated organizations, or those of the publisher, the editors and the reviewers. Any product that may be evaluated in this article or claim that may be made by its manufacturer is not guaranteed or endorsed by the publisher.
Research integrity at Frontiers
Learn more about the work of our research integrity team to safeguard the quality of each article we publish.