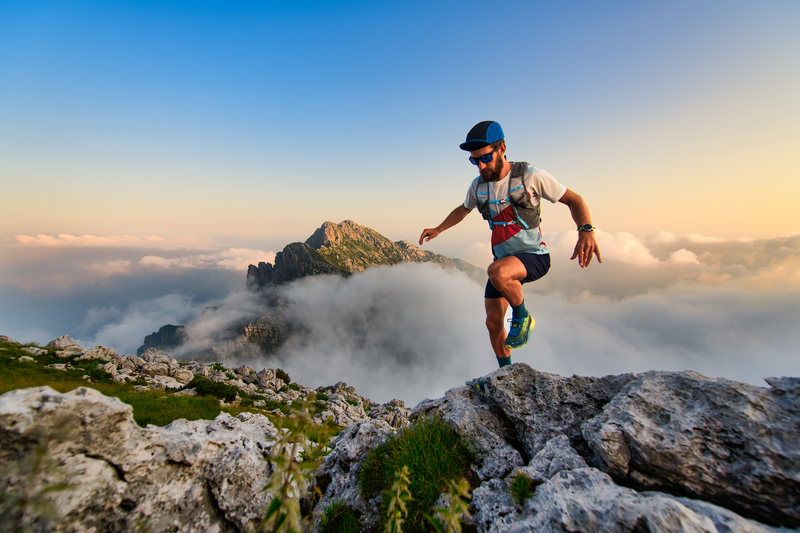
94% of researchers rate our articles as excellent or good
Learn more about the work of our research integrity team to safeguard the quality of each article we publish.
Find out more
ORIGINAL RESEARCH article
Front. Plant Sci.
Sec. Sustainable and Intelligent Phytoprotection
Volume 16 - 2025 | doi: 10.3389/fpls.2025.1492110
This article is part of the Research Topic Precision Information Identification and Integrated Control: Pest Identification, Crop Health Monitoring, and Field Management View all 12 articles
The final, formatted version of the article will be published soon.
You have multiple emails registered with Frontiers:
Please enter your email address:
If you already have an account, please login
You don't have a Frontiers account ? You can register here
In the natural harvesting conditions of cherry tomatoes, the robotic vision for harvesting faces challenges such as lighting, overlapping, and occlusion among various environmental factors. To ensure accuracy and efficiency in detecting cherry tomatoes in complex environments, the study proposes a precise, real-time, and robust target detection algorithm: the CTDA model, to support robotic harvesting operations in unstructured environments. The model, based on YOLOv8, introduces a lightweight downsampling method to restructure the backbone network, incorporating adaptive weights and receptive field spatial characteristics to ensure that low-dimensional small target features are not completely lost. By using softpool to replace maxpool in SPPF, a new SPPFS is constructed, achieving efficient feature utilization and richer multi-scale feature fusion. Additionally, by incorporating a dynamic head driven by the attention mechanism, the recognition precision of cherry tomatoes in complex scenarios is enhanced through more effective feature capture across different scales. According to experimental findings, CTDA has strong robustness and adaptability in complicated environments. The detection accuracy reaches 94.3%, with a recall rate and mAP@0.5 of 91.5% and 95.3% respectively, while mAP@0.5:0.95 is 76.5% and FPS is 154.1 frames per second. Compared to YOLOv8, its mAP@0.5 has increased by 2.9% while maintaining detection speeds, with a model size of 6.7M, deployable on edge devices for rapid detection, and providing technical support for automated harvesting robots for greenhouse cherry tomatoes.
Keywords: Picking robot, Cherry tomato detection, deep learning, YOLO, Multi-scale feature fusion
Received: 14 Oct 2024; Accepted: 21 Feb 2025.
Copyright: © 2025 Liang, Zhang, Lin, Wang, Li and Zou. This is an open-access article distributed under the terms of the Creative Commons Attribution License (CC BY). The use, distribution or reproduction in other forums is permitted, provided the original author(s) or licensor are credited and that the original publication in this journal is cited, in accordance with accepted academic practice. No use, distribution or reproduction is permitted which does not comply with these terms.
* Correspondence:
Xiaojuan Li, Xinjiang University, Urumqi, China
Xiangjun Zou, Xinjiang University, Urumqi, China
Disclaimer: All claims expressed in this article are solely those of the authors and do not necessarily represent those of their affiliated organizations, or those of the publisher, the editors and the reviewers. Any product that may be evaluated in this article or claim that may be made by its manufacturer is not guaranteed or endorsed by the publisher.
Research integrity at Frontiers
Learn more about the work of our research integrity team to safeguard the quality of each article we publish.