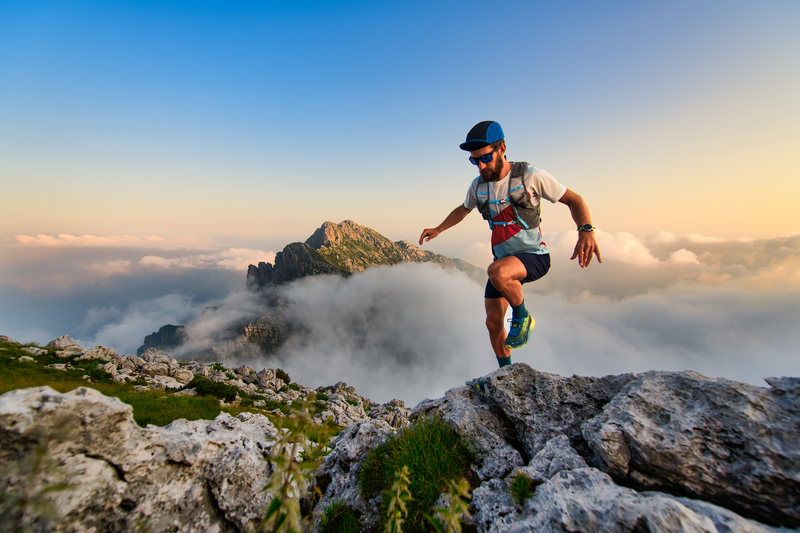
94% of researchers rate our articles as excellent or good
Learn more about the work of our research integrity team to safeguard the quality of each article we publish.
Find out more
ORIGINAL RESEARCH article
Front. Plant Sci. , 05 January 2022
Sec. Plant Pathogen Interactions
Volume 12 - 2021 | https://doi.org/10.3389/fpls.2021.752683
Panax notoginseng (Burk.) F. H. Chen is a Chinese medicinal plant of the Araliaceae family used for the treatment of cardiovascular and cerebrovascular diseases in Asia. P. notoginseng is vulnerable to root rot disease, which reduces the yield of P. notoginseng. In this study, we analyzed the rhizosphere soil and root endophyte microbial communities of P. notoginseng from different geographical locations using high-throughput sequencing. Our results revealed that the P. notoginseng rhizosphere soil microbial community was more diverse than the root endophyte community. Rhodopseudomonas, Actinoplanes, Burkholderia, and Variovorax paradoxus can help P. notoginseng resist the invasion of root rot disease. Ilyonectria mors-panacis, Pseudomonas fluorescens, and Pseudopyrenochaeta lycopersici are pathogenic bacteria of P. notoginseng. The upregulation of amino acid transport and metabolism in the soil would help to resist pathogens and improve the resistance of P. notoginseng. The ABC transporter and gene modulating resistance genes can improve the disease resistance of P. notoginseng, and the increase in the number of GTs (glycosyltransferases) and GHs (glycoside hydrolases) families may be a molecular manifestation of P. notoginseng root rot. In addition, the complete genomes of two Flavobacteriaceae species and one Bacteroides species were obtained. This study demonstrated the microbial and functional diversity in the rhizosphere and root microbial community of P. notoginseng and provided useful information for a better understanding of the microbial community in P. notoginseng root rot. Our results provide insights into the molecular mechanism underlying P. notoginseng root rot and other plant rhizosphere microbial communities.
Panax notoginseng (Burk.) F. H. Chen, belonging to the Araliaceae family, is a Chinese medicinal plant that is mainly grown in Yunnan and Guangxi Provinces of China and is commonly used for the treatment of coronary heart and cardiovascular diseases (Hong et al., 2010; Fan et al., 2016). P. notoginseng is often used in a large variety of traditional Chinese medicine. P notoginseng has been widely used in China and other Asian countries as a therapeutic strategy or health care product, such as hemostatic, anti-thrombotic, and anti-atherosclerotic agent, as well as used to reduce blood pressure and relieve pain, and presents neuroprotection effects (Yang et al., 2019; Guo et al., 2020). Same as the root of the medicinal part of ginseng, the root of P. notoginseng has been used as a raw medicinal material in more than 400 products in 1,300 companies in China (Miao et al., 2006). The World Health Organization has reported that approximately one-third of all deaths worldwide are attributed to cardiovascular diseases (Santhakumar et al., 2018). As the incidence of cardiovascular diseases has increased, and with the modernization of Chinese medicine, the demand for root material of P. notoginseng has been increasing. However, P. notoginseng is vulnerable to various plant diseases, of which root rot is the most serious, limiting P. notoginseng production.
Approximately 70% of medicinal plants have different degrees of pathogen infection related to continuous cropping obstacles (Wu et al., 2013; Zhao Y. P. et al., 2016). The death rates of P. notoginseng seedlings were 2.0–39.4 and 2.6–81.2% in continuous cropping and replanting systems, respectively (Dong et al., 2016). Root rot can reduce P. notoginseng yield by 70% during the entire growing period (Dong et al., 1988). Previous studies showed that Cylindrocarpon destructans var. destructans causes root rot in P. notoginseng (Mao et al., 2014); Fusarium oxysporum and Fusarium solani are associated with root rot disease in P. notoginseng (Ma et al., 2019). Using the internal transcribed spacer (ITS) sequencing technology, the dominant microorganisms in root rot of P. notoginseng was identified as Ilyonectria mors-panacis (Mi et al., 2017), and found several potential pathogenic fungi in the rhizosphere soil of 2-year-old healthy P. notoginseng plants (Miao et al., 2016). Owing to the influence of various plant diseases, continuous cropping obstacles, and the Daodi (the distinctively higher quality of the medicinal materials that grow in a certain area) of P. notoginseng (Liu et al., 2020), the available planting area of P. notoginseng is decreasing. Therefore, to increase the production of P. notoginseng, it is necessary to identify the key pathogens associated with root rot in this species to provide support for research on corresponding prevention methods.
The microbial community plays important roles in nutrient absorption and resistance to environmental pressures, and the rhizosphere microbial community is also essential for plant health and disease suppression in rhizosphere soil. Previous studies have shown that the rhizosphere soil microbial diversity of healthy plants is greater than diseased plants (Brussaard et al., 2007; Bakker et al., 2010). Recently, it has been shown that plant stress responses are linked to the composition of beneficial plant microbial communities (Bakker et al., 2018). The relationship between soybeans and rhizosphere microbial communities shows that resistant soybeans are rich in genes encoding phenazines, phospholipids, and other antifungal-related genes. Furthermore, more rhizosphere bacteria resistant to the Fusarium genotype can be synthesized (Mendes et al., 2018). The microbial communities in the rhizosphere soil and roots of healthy and diseased P. notoginseng have been analyzed through bacterial 16S rRNA and fungal 18S rRNA genes. In the rhizosphere soil of diseased P. notoginseng, microbial communities showed a decrease in alpha diversity, the bacterial community dissimilarity increased, and fungal community dissimilarity decreased. However, the bacterial and fungal community dissimilarity in the roots were not markedly different between healthy and diseased P. notoginseng (Wu et al., 2015). It has also been shown that following root rot infection of P. notoginseng, the content of phenolic acid in the soil is lower in diseased P. notoginseng, and increase the phenolic acid content can effectively prevent the root rot of P. notoginseng (Zhang et al., 2018). Phenolic acid can inhibit the growth of pathogenic bacteria, while also stimulating the production of ferric acid; therefore, adjusting the content of phenolic acid can effectively prevent root rot disease occurrence in P. notoginseng (Zhao et al., 2018).
Metagenomics refers to the total DNA of the microbial community investigated at one point, allowing the identification of all microorganisms in an environment, especially those that are difficult to cultivate in a laboratory (Chen and Pachter, 2005). Metagenomics technology is an effective approach to find unculturable microorganisms and overcome the limitation of amplicons (Wang P. P. et al., 2020). Several studies have applied metagenomics technology to help solve disease-related problems in plants, such as potato (Goss et al., 2014), peas (Gaulin et al., 2007; Taheri et al., 2017), citrus (Duan et al., 2009), and tomatoes (Kwak et al., 2018). Previous studies have investigated root rot in P. notoginseng using the amplicon technologies, such as 16S/18S rRNA sequencing and ITS, and mostly focused on fungi. Even though other species such as Panax ginseng and Panax quinquefolius have been studied (Xing et al., 2010; Vendan et al., 2012; Ji et al., 2018; Deng et al., 2020; Wang Q. et al., 2020), reports on the endophytes and rhizosphere microorganisms of P. notoginseng remain limited and no studies have investigated the microbial community of root rot P. notoginseng using metagenomics.
In this study, the high-throughput sequencing technology was used to sequence the rhizosphere soil and root endophytes of healthy and root rot diseased P. notoginseng. We aimed to reveal the structure and functional difference of microbial community through metagenomic analysis and report the pathogenic and antagonistic bacteria of root rot diseased P. notoginseng. Investigating the correlation between the root rot and microbial community will provide a theoretical basis for the prevention and treatment of root rot in P. notoginseng. Consequently, the findings of this study will ensure the sustainable development of P. notoginseng.
In this study, Lijiang City (LJ) (26°49′59″ N, 100°3′24″ E, altitude: 2,640 m) and Qiubei County (QB) (24°2′49″ N, 103°58′40″ E, altitude: 2,020 m) in Yunnan Province were selected as sampling points of P. notoginseng. We set three biological replicates in each sampling point. In each biological replicate, one diseased (the aboveground parts were wilted and yellowed, and the roots were necrotic) and one healthy plant (no signs of root rot) were selected (Figure 1A). In each plant, the root endophyte and corresponding rhizosphere soil were collected. A total of 24 samples were collected for further analysis.
Figure 1. Characteristics of healthy and root rot diseased Panax notoginseng plants. (A) Diseased and healthy P. notoginseng plant parts. (B) Observed OTUs box plot based on the rhizosphere soil and root endophyte bacteria species abundance. (C) Shannon index box plot based on the rhizosphere soil and root endophyte bacteria species abundance. (D) Boxplots of the top five species in each of the rhizosphere soil and root endophyte groups. Tukey’s HSD test is used to compare the differences between two groups and add the difference group letters.
When collecting the root endophyte of P. notoginseng, the plant was gently lifted from the soil and shaken to remove the dirt and other impurities attached to the roots. Thereafter, the roots were carefully rinsed with sterile water until the root surfaces were free of impurities. A prepared sterile scalpel was used to cut off the cleaned roots (∼100 g) into 50 mL sterile centrifuge tubes and were immediately placed into liquid nitrogen for preservation. Then, the corresponding rhizosphere soil of the plant was collected. A standard soil ring knife was used to collect the rhizosphere soil (∼20 cm under the ground) from each plant and placed into 50 mL sterile centrifuge tubes that were stored in liquid nitrogen for preservation.
DNA isolation performed using the modified CTAB method (Doyle and Doyle, 1987). Briefly, we piped 1,000 μL CTAB lysate into a 2.0 mL EP tube; added lysozyme and 3 g sample to the lysate; placed the EP tube into a 65°C water bath; inverted and mixed several times to fully mix the sample. Then, we centrifuged to collect the supernatant, added phenol: chloroform: isoamyl alcohol (25:24:1), mixed, and centrifuged at 12,000 rpm for 10 min. Subsequently, we removed the supernatant, added chloroform: isoamyl alcohol (24:1), inverted and mixed, and centrifuged again at 12,000 rpm for 10 min. We placed the supernatant into a 1.5 mL centrifuge tube, added isopropanol, mixed, and left to precipitate at −20°C. Then, we centrifuged at 12,000 rpm for 10 min, removed the liquid and washed the precipitate with 1 mL 75% ethanol. After washing twice, the remaining liquid could be collected after centrifugation and removed with a pipette. We placed the final sample in an ultra-clean workbench to dry at 25°C. We added ddH2O to dissolve the DNA sample, 1 μL RNase A was added to digest the RNA, and placed the sample at 37°C for 15 min. The collected DNA sample was used for sequencing.
A Qubit 2.0 fluorometer (Thermo Fisher Scientific Inc., Waltham, MA, United States) was used to accurately quantify the DNA concentration. After the DNA samples were quantified, they were randomly interrupted using the Covaris Focused-ultrasonicator (Covaris, Inc., Woburn, MA, United States), and then the library was prepared following the steps of end repair, A-tailing, sequencing adapters, purification, and PCR amplification followed by Illumina library construction protocol (Illumina Inc., San Diego, CA, United States). Initially, library was constructed, and the inserted fragments of the library were detected using the Agilent 2100 bioanalyzer (Agilent, Santa Clara, CA, United States). After the size of the inserted fragments were standardized (500 bp), the effective concentration of the library was accurately quantified using the Q-PCR method to ensure the quality of the library. Secondly, libraries were pooled to flow cells according to the effective concentration and target data volume requirements. At last, the cBOT was clustered, a NovaSeq 6000 high-throughput sequencing platform (Illumina, Inc., San Diego, CA, United States) was used for sequencing. Each root endophyte sample had approximately 20–30 Gb of sequencing data, and each rhizosphere soil sample had approximately 10–30 Gb of sequencing data.
The FastQC software was used to estimate the quality of the original sequences of 24 samples to generate a quality evaluation report (Andrews, 2010). The Kneaddata tool1 was used to perform quality control and de-hosting the P. notoginseng genome sequence. The Kneaddata process relies on Trimmomatic to remove adaptors (Bolger et al., 2014), linkers, and low-quality sequences (parameters: sequence quality ≥ 20, minimum sequence length ≥ 50 bp). Bowtie2 (Langmead and Salzberg, 2012) was used to mapping the reads to the genome of P. notoginseng (Zhang et al., 2017), and reserved the unmapped reads as finally clean sequences (Table 1). Cleaned reads were used for downstream analyses.
The HUMAnN2 software was utilized to calculate the species and functional composition of the samples (Franzosa et al., 2018). HUMAnN2 does not consider paired-end information in the analysis, therefore the paired-end sequence after quality control is combined as one input file, while the other parameters are the default parameters. MetaPhlAn2 was used to calculate the species composition (Truong et al., 2015). We mapped the clean reads to the HUMAnN2 nucleic acid database using Bowtie2 software (Langmead and Salzberg, 2012), and generated species abundance composition table for each group. We used GraPhlAn to analyze the top 100 representative species in the species composition table at the phylum level (Asnicar et al., 2015). To display the species circle map, R software version 3.4.3 was used to display the heat map of the species at the genus level (R Core Team, 2015, 2020), and to compare the differences in species composition between groups. Finally, LEfSe was used for the species difference analysis (Segata et al., 2011). The LEfSe used the Kruskal–Wallis and Wilcoxon signed-rank tests, signed linear judgment analysis (LDA) logarithmic score, and related P-values to identify species with significant differences in each group (LDA > 4 was considered as significantly different species) (Zuo et al., 2021).
Kraken2 is a high-precision metagenomic sequence classification software based on the k-mer algorithm (Wood et al., 2019). Kraken2 is used for species annotation at the read level, which can rapidly classify sequencing reads for species classification. We compared our data to the Kraken2 standard library, fungi, protozoa, and plasmid databases. The bacteria, archaea, fungi, protozoa, plasmids, and virus libraries were provided by the RefSeq database2. The species composition table was used for species diversity analysis, the species level annotations were extracted and combined to the minimum sequencing amount, and alpha diversity was calculated using the R. In addition, alpha diversity boxplots and the top 30 genera of each group were used to compare the differences.
The EukDetect method (Lind and Pollard, 2021) was used to detect eukaryotic microorganisms in the clean data. The EukDetect pipeline uses a Snakemake workflow engine (Kster and Rahmann, 2018). Microbial eukaryotic genomes were downloaded from NCBI GenBank for all species designated as fungi, protists, other non-vertebrate metazoans, and non-streptophyta archaeplastida species. In addition to the GenBank genomes, 314 genomes and transcriptomes comprising 282 protists, 30 Archaeplastida species, and 2 metazoans curated by the EukProt project were downloaded (Richter et al., 2020).
MEGAHIT (Li et al., 2015) was used for each sample assembly. The contigs of each sample were mixed by group, and the quality of contigs was assessed by QUAST (Alexey et al., 2013). MetaProdigal (Hyatt et al., 2012) was used for gene prediction, and cd-hit-est (Fu et al., 2012) to cluster and de-redundancy the predicted genes to construct a non-redundant gene set (similarity ≥ 95%, coverage ≥ 90%). The non-redundant gene set obtained was used for subsequent analysis. The nucleic acid sequences were quantified using Salmon (Patro et al., 2017). The parameter was set to the metagenomic model (–meta) for all reads that could be binned, and the abundance of genes in each sample was obtained.
We used MetaWRAP (Uritskiy et al., 2018) to excavate the draft genome of a single strain. This process integrates three popular binning software. We selected the MaxBin2 (Wu et al., 2016) and MetaBAT2 (Kang et al., 2019) software for binning. The de-redundant contigs of each group were binned, and the bins were purified. We evaluated and comprehensively analyzed the results to obtain better results. The Checkm database (Parks et al., 2015) requires completeness of more than 70% and a pollution rate of less than 5%, which was used for purification. After purification, the Blobology module was used to visualize the GC content and abundance of contigs by comparing the NCBI nt and taxonomy database was obtained. Samlon (Patro et al., 2017) was used to quantify the bins and calculate the abundance of a single bacterial genome in each sample. Subsequently, each contig in the bin was annotated, and the species of each bin was estimated. Krona was used to visualize the results of bin annotation (Ondov et al., 2011). Finally, Bins were classified using a concatenated set of 122 universal marker proteins by GTDB-Tk to find new species (Chaumeil et al., 2019).
Diamond (Huson and Buchfink, 2015) was used to compare contigs to the UniProt protein library3, which includes the UniRef90 protein database; the MetaCyc metabolic pathway database was used to predict gene pathways4. MinPath5 defines the minimum set of pathways, producing results at the protein, gene, and pathway levels, and produces a functional composition table. The STAMP (Parks et al., 2014) software was used to calculate the functional pathways and to produce a histogram. Significant functional differences between the groups were set at P < 0.05.
The nucleic acid sequence was translated into a protein sequence and compared to the eggNOG (Jaime et al., 2016) database using the Diamond (Huson and Buchfink, 2015). The eggNOG database integrates annotation information, such as Gene Ontology (GO), clusters of orthologous genes (COG), and KEGG orthologs (KO) information. We performed multiple sequence alignment between the query and the eggNOG database to determine the conserved sites and analyze their evolutionary relationships (Jaime et al., 2016). We selected the possible gene names of each sequence predicted by the eggNOG database and eliminated the duplicated results. The eggNOG annotation results were used for subsequent analysis. Diamond (Huson and Buchfink, 2015) was used to align the protein sequence with the carbohydrate-active enzymes database (CAZy) (Vincent et al., 2013). The CAZy database provides an understanding of the nature and extent of the complex carbohydrate metabolism focuses on the differences in carbon source metabolism between species, and was used to compare to the Resfams database using Diamond (Gibson et al., 2015), we predicted the unknown resistance gene and obtained the mechanism and function of the resistance genes in each sample.
The Pseudomonas fluorescens strain (Mingzhou Biotechnology Co., Ltd., Ningbo, China) was expanded using NA (Nutrient Agar) culture medium. Before the experiment, the healthy P. notoginseng was scratched with a sterile knife to facilitate the entry of P. fluorescens into the plant. A control and an experimental group were set up in this study. The diluted P. fluorescens strain was added into the culture bottle with healthy P. notoginseng in the experimental group. The control group was filled with sterile water with the same volume, and each group had three biological replicates, all samples were cultured at 25°C, and the results were observed each day.
A total of 333.90 Gb raw data were produced from the 12 root endophyte samples, with an average of 27.83 Gb raw data per sample. Twelve rhizosphere soil samples produced a total of 173.80 Gb raw data with an average of 14.48 Gb raw data per sample. After assessing the quality of the original data, the Q30 values of all samples were >93%. Quality controls were performed on the raw data to remove host contamination and obtain clean data. After removing the host-related sequences of P. notoginseng, each rhizosphere soil and root endophyte sample had an average of 13.90 and 12.66 Gb of clean data (Table 1 and Supplementary Table 1). Based on the alpha diversity chao1 and observed dilution curves (Supplementary Figure 1) of rhizosphere soil and root endophyte samples, Supplementary Figures 1A,B show that the data were saturated at 500,000 sequences (0.075 Gb) of rhizosphere soil sample. Similarly, Supplementary Figures 1C,D show saturation at 1,000,000 sequences (0.15 Gb) of the root endophyte sample. These results confirm that the sequencing depth of each sample reached saturation, enabling us to perform the next steps of this study.
Kraken2 is a high-precision metagenomic sequence classification software based on the k-mer algorithm, which can classify sequencing data by species (Wood et al., 2019). In this study, the species diversity of each group was analyzed, the alpha diversity and Shannon index boxes were plotted (Figures 1B,C). The other diversity index boxes are shown in Supplementary Figure 2. Boxplots of the top five genera are shown in Figure 1D. The results showed that the overall richness of the rhizosphere soil was higher than the root endophytes. The number of operational taxonomic units (OTUs) in the rhizosphere soil of diseased plants was higher than healthy plants, whereas, in the root endophyte samples, the number of OTUs of healthy plants was higher than diseased plants (Figure 1B). The abundance of Bradyrhizobium in healthy rhizosphere soil was higher than in diseased rhizosphere soil (Figure 1D). In the healthy rhizosphere soil, the top three genera were Bradyrhizobium, Micromonospora, and Streptomyces. The genus-level differences were more evident in the root endophytes between healthy and diseased plants. The three most abundant genus in the healthy root endophytes were Lysobacter, Pseudomonas, and Paraburkholderia. The top three genera in diseased root endophytes were Pseudomonas, Lelliottia, and Variovorax. Lysobacter and Paraburkholderia were more abundant in healthy samples than in root rot diseased samples, whereas Pseudomonas and Lelliottia were more abundant in diseased root endophyte samples (Figure 1D).
We used the species annotation results of MetaPhlAn2, which is more accurate. There are 143 OTUs in rhizosphere soil and 187 OTUs in root endophyte, and we chose the top 100 species, which is more representative and classified them by phylum. As indicated by the colors in the species circle diagrams, there were four main phyla: Actinobacteria, Bacteroides, Firmicutes, and Proteobacteria. Species that did not classify into these four phyla were grouped as others in the circle diagrams. The circle diagrams showed that Proteobacteria occurred in the highest proportion in both the rhizosphere soil and root endophyte samples. The relative occurrence of the phyla in the rhizosphere soil and root endophyte samples are Actinobacteria, 14 and 7%; Bacteroides, 10 and 7%; Firmicutes, 20 and 6%; Proteobacteria, 50 and 77%; others, is 6 and 3%, respectively (Figure 2). The species composition histograms showed the differences at the genus level between different health statuses (Figure 3). A total of 87 genera were detected in the rhizosphere soil and the top seven most abundant genera were Rhodopseudomonas, Actinoplanes, Burkholderia, Caulobacter, Ktedonobacter, Mesorhizobium, and Granulicella (Figure 3A). The rhizosphere soil bacterial community in the diseased and healthy rhizosphere soil samples from LJ and QB showed contrasting results. The abundance of Rhodopseudomonas was higher in the healthy rhizosphere soil at the LJ site, whereas it was more abundant in the root rot diseased rhizosphere soil at the QB site. Ktedonobacter was found only in the rhizosphere soil from QB and was more abundant in the root rot diseased soil sample. In total, 108 genera were detected in the root endophyte samples (Figure 3B). The eight most abundant genera were Pseudomonas, Burkholderia, Variovorax, Afipia, Agrobacterium, Sphingobium, Rhodanobacter, and Janthinobacterium. Pseudomonas showed a high abundance in the diseased root endophyte samples from LJ, and a high abundance in the healthy root endophyte samples from QB, which may be related to the ecological environment of the different locations. The abundance of Burkholderia in the healthy root samples was higher than in diseased samples. Variovorax and Afipia showed high abundance in the healthy root endophyte samples from LJ. Overall, the abundance of Agrobacterium and Sphingobium in the diseased root endophyte samples was higher than in healthy samples. There were eight species with significant differences in the root endophyte and rhizosphere soil samples (LDA > 4 for significant differences; Figure 3C).
Figure 2. Species composition circle diagrams based on the top 100 most abundant species in rhizosphere soil and root endophyte samples. The colors represent the four phyla that contain these species. The purple squares in the first ring indicate the species with an abundance greater than 5 per 1,000. The yellow squares in the second ring indicate the species with an abundance less than 5 per 1,000. The third ring indicates the average value for all samples, where the different shades of green show the different species abundances. (A) Top 100 most abundant species in disease rhizosphere soil. (B) Top 100 most abundant species in healthy rhizosphere soil. (C) Top 100 most abundant species in disease root endophyte. (D) Top 100 most abundant species in healthy root endophyte.
Figure 3. Genus composition heatmaps and corresponding histograms for the microbial communities associated with healthy and root rot Panax notoginseng. (A,B) Relative abundance of the top eight species in each sample. (C) Species differences in the rhizosphere soil and root endophyte microbial communities associated with healthy and root rot P. notoginseng. Display the species with significant differences (LDA > 4) between the groups. Panel (D) shows the top nine species of eukaryotes present in the samples. Lijiang (LJ) and Qiubei (QB) and 1, 2, and 3 represent biological replicate in each location.
We also analyzed the composition of fungi in all samples and a total of 12 eukaryote species in the rhizosphere soil, and 29 in the root endophyte samples were present (Supplementary Table 2). Acaulopage tetraceros, Acrobeloides nanus, Arthrobotrys oligospora ATCC 24927, Dactylellina haptotyla CBS 200.50, and Folsomia candida were unique to root endophytes; A. tetraceros and Chaetomium cochliodes were more abundant in the healthy samples; A. nanus, A. oligospora ATCC 24927, F. candida, and Pseudopyrenochaeta lycopersici were more abundant in the diseased samples; I. mors-panacis was the most abundant species in the diseased samples (Figure 3D).
There were 16 significantly different pathways in the rhizosphere soil. The pathways of the CDP-diacylglycerol biosynthesis I/II, superpathway of L-threonine biosynthesis, and superpathway of L-isoleucine biosynthesis I were significantly up-regulated in the diseased rhizosphere soil samples, whereas the L-lysine biosynthesis III and pentose phosphate pathway were significantly up-regulated in the healthy rhizosphere soil (Figure 4A). There were 12 significantly different functional pathways in the root endophyte samples. Superpathway of L-phenylalanine biosynthesis, 4-amino-2-methyl-5-phosphomethylpyrimidine biosynthesis and pyridoxal 5′-phosphate biosynthesis I was significantly up-regulated in the root endophyte diseased sample, whereas in the healthy root endophyte samples L-valine biosynthesis, L-isoleucine biosynthesis I, and pyruvate fermentation to isobutanol were significantly up-regulated (Figure 4D). The GO term annotation results were mainly divided into three categories: molecular function (MF), cellular component (CC), and biological process (BP). The top 50 GO terms of each group were classified and enriched, and the identified GO terms belonged to the BP category (Figure 4). The enrichment degree of the GO terms in the root endophytes (Figures 4E,F) was higher than that in the rhizosphere soil (Figures 4B,C). The pathways of the top three enrichments in the rhizosphere soil were the BP, metabolic process, and organic substance metabolic process. The carboxylic acid metabolic process and the oxoacid metabolic process were enriched in the root rot diseased soil group and did not appear in the top 20 processes associated with the healthy rhizosphere soil (Figures 4B,C). The macromolecule metabolic process, cellular aromatic compound metabolic process, and organic cyclic compound metabolic process were significantly enriched in healthy root endophytes and did not occur in the top 20 processes of the diseased root endophytes (Figures 4E,F).
Figure 4. Differences in the functional composition of the rhizosphere soil and root endophyte microbial communities associated with healthy and root rot P. notoginseng. Panels (A,D) show the functional pathways with significant differences between healthy and diseased samples, respectively. Panels (B,C,E,F) represent the Gene Ontology (GO) enrichment annotation results for each group.
We de novo assembled the clean data, evaluated the quality of the contig, and then performed de-redundancy, quantification and obtained pure contig for subsequent functional annotation. We obtained 11.22 Gb contigs with an average contig N50 of 1, 287 bp (Supplementary Table 3). We compared the contigs to the eggNOG (Jaime et al., 2016) database, integrated the annotation results, and counted the annotated COG orthologous proteins in each group. The COG classified 24 functional descriptions, and the annotation implied no significant differences among the 24 functional descriptions of the diseased and healthy rhizosphere samples (P > 0.05). The three dominant functions with higher abundance in the rhizosphere soil samples were S: function unknown (healthy rhizosphere soil = 20.61%, diseased rhizosphere soil = 20.85%), E: amino acid transport and metabolism (healthy rhizosphere soil = 9.82%, diseased rhizosphere soil = 9.54%), and C: energy production and conversion (healthy rhizosphere soil = 8.69%, diseased rhizosphere soil = 8.22%; Supplementary Table 3). Among the 24 functional descriptions of the root endophytes, four of them were significantly different between the diseased and healthy samples (P < 0.05), and all of them showed a higher abundance in the diseased samples: E: amino acid transport and metabolism (P = 0.011), N: cell motility (P = 0.028), O: posttranslational modification, protein turnover, chaperones (P = 0.027), and S: function unknown (P = 0.027) (Supplementary Figure 3A). The three dominant functions with a higher abundance in the root endophyte samples were S: function unknown (healthy root endophyte = 20.73%, diseased root endophyte = 19.38%), E: amino acid transport and metabolism (healthy root endophyte = 8.88%, diseased root endophyte = 9.60%), and P: inorganic ion transport and metabolism (healthy root endophyte = 8.29%, diseased root endophyte = 8.63%), the functional pathway of which was relatively abundant in the root rot diseased samples (Supplementary Table 3).
The Resfam database analysis results indicated that there were 15 classifications of mechanisms with 94 functional descriptions. Among them, the diseased rhizosphere soil group had the most resistance genes (106,554), with an average of 17,759 resistance genes per sample. The resistance genes of the other groups were healthy rhizosphere soil (89,250), diseased root endophyte (84,135), and healthy root endophyte (57,122). Overall, there were more resistance genes in the rhizosphere soil than in the root endophytes, and the number of resistance genes in the diseased group was higher than in the healthy group. The ABC transporter, RND antibiotic efflux and gene modulating resistance functions in the root rot disease group were higher than those in the healthy group (Supplementary Table 3 and Supplementary Figure 3B).
The KO function results for each group were counted. Among them, the healthy rhizosphere soil group had a total of 7,898 KOs, the disease rhizosphere soil group presented 8,078 KOs, the healthy root endophyte group presented 9,886 KOs, and the disease root endophyte group presented 10,736 KOs. The number of KOs in the root endophytes was higher than in the rhizosphere soil, and that of KOs in the diseased group was higher than in the healthy group. There was 6,469 core KOs in the four groups, accounting for 54.4% (Supplementary Figure 3C). In addition, each group had unique KOs, which were 106 (0.9%), 124 (1.0%), 435 (3.7%), 921 (7.7%), sorted by healthy rhizosphere soil, disease rhizosphere soil, healthy root endophyte, and disease root endophyte, respectively. The KO results showed that zearalenone synthase (K15418), lysophospholipid acyltransferase (K13519), Pin2-interacting protein (K11135), G protein-coupled receptor 64 (K08451), and MFS transporter (K08181) are unique KO in disease root endophyte.
The CAZy database includes five categories and one related module. Each category was divided into families. Among them, the glycoside hydrolases (GHs), which mainly hydrolyze and rearrange glycosidic bonds, presented a total number of 167 families (Vincent et al., 2013). We found 123 GH families in the healthy rhizosphere soil, 127 in the diseased rhizosphere soil, 126 in the healthy root endophyte, and 131 in the diseased root endophyte group (Supplementary Table 3). The number of GH families in the diseased samples was slightly higher than that in the healthy group. Similarly, there were 110 families of glycosyltransferases (GTs), mainly involved in the formation of glycosidic bonds. In addition, polysaccharide lyases (PLs) presented 40 families, carbohydrate esterases have 17 families, auxiliary activities have 16 families, and carbohydrate-binding modules have 86 families. These families were observed in slightly higher numbers in the diseased group than in the healthy group. The sequence annotation results of CAZy database showed that healthy rhizosphere soil, disease rhizosphere soil, healthy root endophyte, and disease root endophyte show an increasing trend by turns. The healthy rhizosphere soil group had the lowest number of reads matched to the CAZy database, the diseased root endophyte group had the most reads matched to the CAZy database, and the GT family matched ratio was the highest, followed by that of the GH family (Supplementary Table 3).
After assembling the clean data, de-redundant contigs were binned to mine the single bacterial genome. Ultimately, 9 bins were obtained in the healthy rhizosphere soil group, 24 bins in the diseased rhizosphere soil group, and 2 bins in the diseased rhizosphere soil group with >99% completeness. Thaumarchaeota had 99.51% completeness with the genome length of 1.40 Mb; Clostridium had 99.01% completeness with the genome length of 6.31 Mb in the diseased rhizosphere soil (Supplementary Figure 4 and Supplementary Table 4). A total of 41 bins were in the healthy root endophyte group, two of which were 100% complete, namely Flavobacteriaceae (genome length = 5.00 Mb) and Bacteroidetes (genome length = 6.34 Mb). There were 91 bins in the diseased root endophyte group, one of which was 100% complete (Flavobacteriaceae, genome length = 4.3 Mb). We found that these two 100% complete Flavobacteriaceae genomes were two different species under the Flavobacteriaceae genus through similarity comparison. The GC content results indicated that the abundance of orders in the rhizosphere soil was greater than that in the root endophytes (Supplementary Table 4). These results are consistent with the findings of higher microbial diversity in the rhizosphere soil than root endophytes samples (Figure 1B).
The abundance of the purified single bacterial genome in each sample was determined using bin quantification, and the results are displayed in a heat map (Supplementary Figure 4). Bacteria (bin 2) were enriched in the QB-3 sample and Sphingomonadaceae (bin 13) was enriched in the QB-1 sample (Supplementary Figures 4A,C). Alphaproteobacteria (bin 3) were enriched in the LJ diseased root endophyte samples (Supplementary Figure 4D and Supplementary Table 4). Based on taxonomic annotations from the Genome Taxonomy Database (GTDB), the analysis revealed that the bins cover 34 known species (ANI > 95%). There are 132 bins with <95% ANI of the existing genome, which represented potential new species. The GTDB classification of all the 166 bins is shown in Supplementary Table 4.
The shape of the dilution curves for the rhizosphere soil and root endophyte samples significantly differed (Supplementary Figure 1). Among the rhizosphere soil samples (Supplementary Figures 1A,B), only the healthy rhizosphere soil dilution curve shape changed, while the dilution curves of the diseased rhizosphere soil samples remained uniform and the shape of the root endophyte dilution curves was more distinct (Supplementary Figures 1C,D). The evident change in the OTU dilution curve of root endophytes may be attributed to the uneven distribution of the microbial community associated with the roots and other plant parts (Gottel et al., 2011). In addition, when comparing the observed OTUs of rhizosphere soil and root endophyte samples (Figure 1B), we found that the microbial diversity of the rhizosphere soil was higher than that of the root endophytes. Studies have shown that the microbial community of rhizosphere soil is more diverse than root endophytes (Johnson and Jumpponen, 2005; Bulgarelli et al., 2013), with a clear compartmental separation between the rhizosphere soil and root endophyte of plants (Bulgarelli et al., 2012, 2015; Lundberg et al., 2012; Peiffer et al., 2013; Zarraonaindia et al., 2015; Yeoh et al., 2016; Hartman et al., 2017). Our results illustrate this uniqueness of the microbial communities (Figures 3A,B). Previous studies have also shown that the bacteria of root endophytes are recruited from the rhizosphere soil (Chaparro et al., 2014; Edwards et al., 2015), which may explain the higher microbial diversity in the rhizosphere soil than root endophyte.
We compared the bacterial community difference in rhizosphere soil between healthy and diseased samples and found that Rhodopseudomonas was the most abundant in the rhizosphere soil (Figure 3A), especially in the healthy samples from the LJ site. Rhodopseudomonas can promote plant growth, and induce resistance in plants (Hsu et al., 2021). In tobacco, Rhodopseudomonas induces resistance against tobacco mosaic disease (Su et al., 2017). KTSSR54, an active compound produced by Rhodopseudomonas palustris, can help rice inhibit pathogens (Nookongbut et al., 2020). Our results are in line with previous findings showing that Rhodopseudomonas can protect P. notoginseng from root rot. Among the bacteria studied, Actinoplanes have attracted increasing attention since they can inhibit plant pathogens and produce more than 100,000 compounds, such as antifungal compounds, siderophores, hydrocyanic acid, hydrolytic enzymes, and ammonia. Actinoplanes can accelerate the absorption of nutrients and have positively influenced plant growth (Palaniyandi et al., 2013; Sreevidya et al., 2016; Sumaira et al., 2016). Actinoplanes produce many antibiotics, accounting for approximately 45% of the antibiotics on the market (Liu et al., 2012), most of which can treat plant disease (Samac et al., 2003; Cheng et al., 2010; Zucchi et al., 2010; Palaniyandi et al., 2011). In our study, Actinoplanes were more abundant in LJ and QB rhizosphere soil samples (Figure 3A), suggesting that the diseased P. notoginseng plants may have recruited Actinoplanes to resist root rot pathogens, thereby increasing the abundance of Actinoplanes in the soil of diseased plants. Burkholderia was relatively abundant in most samples. Several studies have shown that Burkholderia has antibacterial properties, promotes plant growth and is a potential and effective biological control agent (Jeong et al., 2003; Kunakom and Eustáquio, 2019). Burkholderia is an antagonist of black spot disease in cherries (Ding et al., 2021), and secretes beneficial compounds to protect bananas against Fusarium wilt (Xu et al., 2020). In our study, the abundance of Burkholderia in the healthy root endophyte samples was higher than that in the diseased samples, which is consistent with previous studies (Jeong et al., 2003; Kunakom and Eustáquio, 2019; Xu et al., 2020; Ding et al., 2021), suggesting that Burkholderia is a beneficial bacterium for P. notoginseng. We infer that Burkholderia can protect P. notoginseng from plant diseases.
Pseudomonas, the most abundant dominant bacterial species in the root endophyte samples in our study, was more abundant in the diseased samples from LJ than in other groups (Figure 3B). Pseudomonas has been previously suggested to cause root rot (Miao et al., 2006), and was only identified at the genus level. Herein, we identified Pseudomonas to the species level, with P. fluorescens, known to protect many crop plants against soil-borne diseases caused by phytopathogens (Silby et al., 2009; Liang F. et al., 2020), at the highest abundance. It has also been shown that P. fluorescens, as a biological control agent, can effectively reduce root knot nematode infestation of cucumbers and replace chemical measures for nematode control, thereby increasing cucumber yield and reducing cultivation costs (Panpatte et al., 2020). Screened under in vitro conditions, P. fluorescens has been shown to have antagonistic activity against pea root rot, with high biological control activity (Godebo et al., 2020). A recent report found that Pseudomonas is recruited from roots to resist plant pathogens and was used to verify the disease incidence of plants where it reduces the disease incidence of Arabidopsis (Wen et al., 2020). However, the role of P. fluorescens is still controversial. In this study, we conducted an in vitro experiment to verify whether P. fluorescens has a pathogenic effect on P. notoginseng (Supplementary Figure 5). Combining our data with experimental results, we concluded that P. fluorescens has a pathogenic effect on P. notoginseng. Our results revealed that Variovorax had a higher abundance in most healthy root endophytic samples. We identified Variovorax to the species level and found that the species with the highest abundance was Variovorax paradoxus. Variovorax can facilitate the interaction between plants and microorganisms by manipulating plant hormone levels to balance the growth of plant roots. V. paradoxus can significantly inhibit potato tuber soft rot and is considered to be a new type of potato tuber soft rot biological control agent (Ha et al., 2018). In our study, the abundance of V. paradoxus in healthy root endophyte samples was high. This suggests that V. paradoxus may promote the growth of P. notoginseng and may be an effective biological control agent for root rot disease.
Our analysis showed that I. mors-panacis had the highest ratio in the diseased root endophyte samples (Figure 3D) corroborating with the findings of a previous study that presented I. mors-panacis as the main pathogen of P. notoginseng root rot (Mi et al., 2017). I. mors-panacis is also the main pathogen of P. ginseng root rot (Desrochers et al., 2020; Farh et al., 2020). P. lycopersici is also a plant fungal pathogen that causes severe root rot and root knot disease (Errico et al., 2019). In our study, P. lycopersici had a higher number of reads in the diseased samples, except for disease rhizosphere soil from QB. P. lycopersici has not previously been reported in association with root rot P. notoginseng, hence we infer that P. lycopersici may be a pathogen of P. notoginseng root rot. A. oligospora ATCC 24927, a fungus that feeds on nematodes, is often used as a biological control agent for plant and animal parasitic nematodes (Ahman et al., 2002). The number of reads of this fungus was relatively high in the diseased samples in our study. A. nanus is a type of nematode that feeds on bacteria and was mainly associated with diseased samples in our study. This could account for the high occurrence of A. oligospora ATCC 24927, which feeds on nematodes, in the diseased samples.
Amino acids play an important role in plant growth as well as pathogen infection as they are indispensable nitrogen sources for many nutritional pathogens (Douglas, 1993; Rico and Preston, 2008), hence regulating the content of amino acids is critical for the growth and defense of plants. It has been demonstrated that the increased expression of usually multiple acids moving in and out transporters (UMAMIT) can induce plant resistance to pathogens (Besnard et al., 2021). In addition, the existence of the amino acid-sensing mechanism in plants indicates that changing the level of amino acids by changing the metabolism or transport of amino acids may trigger a defense response; furthermore, resistance-related genes are up-regulated (Yang et al., 2014). Our study found higher amino acid transport and metabolism in healthy rhizosphere soil than in diseased rhizosphere soil. Based on previous findings, we concluded that the upregulation of amino acid transport and metabolism in soil and the enhancement of transport activity would help to resist the invasion of pathogens and improve the resistance of P. notoginseng (Supplementary Table 3). In a study on the resistance signal mechanism of the related tomato root knot nematode disease, the protein with the greatest difference in the differentially expressed protein group belonged to energy production and conversion because the defense response is an energy-consuming process, which may play a key role in the plant’s disease resistance process (Zhao W. et al., 2016). In our study, the energy production and conversion process in diseased roots was three times that of healthy roots and may be closely related to the disease resistance process of P. notoginseng (Supplementary Table 3).
The misuse of antibiotics has contributed to the widespread development of antimicrobial resistance among clinically significant bacterial species, therefore, understanding the mechanism of antibiotics in P. notoginseng is important for facilitating the healthy growth of this plant species. A previous study has shown that Chinese herbal medicines, such as P. notoginseng, can produce compounds that interfere with quorum sensing, which can effectively inhibit pathogens and help delay antibiotic resistance (Koh and Tham, 2011). In our study, ABC transporter (34.16%), gene modulating resistance (20.55%), and RND antibiotic efflux (16.62%) accounted for the highest proportions and the highest number of diseased samples (Supplementary Figure 3B). ABC transporter reactions play major roles in disease resistance of plants (Devanna et al., 2021). The G and B subfamily of the ABC transporter family have been the most researched on related secondary metabolism and is also the key transport family to the defensive process (Hwang et al., 2016; Borghi et al., 2019; Dhara and Raichaudhuri, 2021). The proportion of ABC transporters in the two groups of diseased samples was higher than that in the corresponding two groups of healthy samples, which may be associated with the disease resistance of P. notoginseng. Previous studies have reported that gene modulating resistance helps plants to inhibit pathogenic bacteria and improve plant resistance (Gong et al., 2020; Liang Y. et al., 2020; Wang J. et al., 2020; Corredor-Moreno et al., 2021; Zia et al., 2021), while the RND antibiotic efflux mechanism has an efflux effect on various antibiotics; inhibiting these pumps can mitigate antibiotic resistance (Siriyong et al., 2017; Liang et al., 2019; Ichinose et al., 2020). Our study showed that the RND antibiotic efflux mechanism in diseased samples was greater than that in healthy samples. We speculate that the RND antibiotic efflux mechanism in the diseased samples was active and had an efflux effect on antibiotics. Thus, the reduction of antibiotics in the diseased samples might have weakened the resistance to pathogens. This phenomenon may induce plant roots to produce antibiotic efflux, making it easier for root rot to invade.
According to the CAZy database comparison results, the proportion of GHs in each group was the largest, and the proportion in diseased samples was higher than that of healthy samples. In a study of peanut stem rot caused by fungi, the number of GHs was the highest in the pathogenic secreted protein (Iquebal et al., 2017). In addition, in soybean seed rot, 149 plant cell wall-degrading enzymes were detected, most of which were GHs (Li et al., 2018). Our results are in line with these previous reports. The number of GHs in the diseased samples was higher than healthy samples, suggesting that the root rot-affected parts of P. notoginseng secrete more enzymes related to the GH family. Regarding the GTs, in a study of wheat infection with F. wilt, the GTs in wheat infected with Fusarium graminearum showed an increasing trend (Ha et al., 2016), indicating that infection with P. notoginseng root rot can cause GTs to increase. In short, the increase in the number of GH and GT families can predict the tendency of P. notoginseng to suffer from root rot (Supplementary Table 3).
The original contributions presented in the study are publicly available. This data can be found here: National Center for Biotechnology Information (NCBI) BioProject database under accession number PRJNA753694.
PW and YL wrote and reviewed the manuscript and draw the picture. PW, LY, JS, and YL collected the samples. YY, YQ, CW, DL, and LH provided the analysis ideas and reviewed the manuscript. XC and YL supervised the work and read and approved the final manuscript. All authors contributed to the article and approved the submitted version.
YL acknowledges the financial support from the National Natural Science Foundation of China (Grant No. 31960134) and Key Project at central government level: the ability establishment of sustainable use for valuable Chinese medicine resources (Grant No. 2060302), and Major Science and Technology Special Project of Yunnan Province (Grant No. 202102AA310034).
The authors declare that the research was conducted in the absence of any commercial or financial relationships that could be construed as a potential conflict of interest.
All claims expressed in this article are solely those of the authors and do not necessarily represent those of their affiliated organizations, or those of the publisher, the editors and the reviewers. Any product that may be evaluated in this article, or claim that may be made by its manufacturer, is not guaranteed or endorsed by the publisher.
We thank the editor and reviewers for providing valuable comments on the manuscript.
The Supplementary Material for this article can be found online at: https://www.frontiersin.org/articles/10.3389/fpls.2021.752683/full#supplementary-material
Supplementary Figure 1 | Alpha diversity dilution curves. Each group contained six samples. The abscissa is the total number of sequences, while the ordinate represents the number of OTUs. (A) Healthy and disease rhizosphere soil chao1 alpha diversity dilution curves. (B) Healthy and disease rhizosphere soil observed alpha diversity dilution curves. (C) Healthy and disease root endophyte chao1 alpha diversity dilution curves. (D) Healthy and disease root endophyte observed alpha diversity dilution curves.
Supplementary Figure 2 | Boxplots of diversity in the rhizosphere soil and root endophyte group. (A) ACE index box plot based on the rhizosphere soil and root endophyte bacteria species abundance. (B) Chao1 index box plot based on the rhizosphere soil and root endophyte bacteria species abundance. (C) Invsimpson index box plot based on the rhizosphere soil and root endophyte bacteria species abundance. (D) Simpson index box plot based on the rhizosphere soil and root endophyte bacteria species abundance.
Supplementary Figure 3 | Annotation results of bacteria with significantly different functional descriptions. (A) Amino acid transport and metabolism, cell motility, posttranslational modification, protein turnover, and chaperones, and unknown function. (B) Antibiotic gene percentage of each group. (C) Venn diagram of KEGG orthologs (KO) functional annotation results for each group.
Supplementary Figure 4 | Quantitative heat map of the bins in each group. (A) Quantitative heat map of the bins in healthy rhizosphere soil. (B) Quantitative heat map of the bins in disease rhizosphere soil. (C) Quantitative heat map of the bins in healthy root endophyte. (D) Quantitative heat map of the bins in disease root endophyte.
Supplementary Figure 5 | Vitro infection results of Pseudomonas fluorescens.
Supplementary Table 1 | Sample and data information.
Supplementary Table 2 | Eukaryote information for each sample.
Supplementary Table 3 | Function annotation information.
Supplementary Table 4 | Genomic and classification information of the bins in each group.
Ahman, J., Johansson, T., Olsson, M., Punt, P., van den Hondel, C., and Tunlid, A. (2002). Improving the pathogenicity of a nematode-trapping fungus by genetic engineering of a subtilisin with nematotoxic activity. Appl. Environ. Microbiol. 68, 3408–3415. doi: 10.1128/AEM.68.7.3408-3415.2002
Alexey, G., Vladislav, S., Nikolay, V., and Glenn, T. (2013). QUAST: quality assessment tool for genome assemblies. Bioinformatics 29, 1072–1075. doi: 10.1093/bioinformatics/btt086
Andrews, A. (2010). FastQC: A Quality Control Tool for High Throughput Sequence Data. Available online at: http://www.bioinformatics.babraham.ac.uk/projects/fastqc
Asnicar, F., Weingart, G., Tickle, T. L., Huttenhower, C., and Segata, N. (2015). Compact graphical representation of phylogenetic data and metadata with GraPhlAn. PeerJ 3:e1029. doi: 10.7717/peerj.1029
Bakker, M. G., Glover, J. D., Mai, J. G., and Kinkel, L. L. (2010). Plant community effects on the diversity and pathogen suppressive activity of soil streptomycetes. Appl. Soil Ecol. 46, 35–42.
Bakker, P., Pieterse, C. M. J., Jonge, R. D., and Berendsen, R. L. (2018). The soil-borne legacy. Cell 172, 1178–1180.
Besnard, J., Sonawala, U., Maharjan, B., Collakova, E., and Okumoto, S. (2021). Increased expression of UMAMIT amino acid transporters results in activation of salicylic acid dependent stress response. Front. Plant Sci. 11:606386. doi: 10.3389/fpls.2020.606386
Bolger, A., Lohse, M., and Usadel, B. (2014). Trimmomatic: a flexible trimmer for Illumina sequence data. Bioinformatics 30, 2114–2120. doi: 10.1093/bioinformatics/btu170
Borghi, L., Kang, J., and Francisco, R. D. (2019). Filling the gap: functional clustering of ABC proteins for the investigation of hormonal transport in planta. Front. Plant Sci. 10:422. doi: 10.3389/fpls.2019.00422
Brussaard, L., Ruiter, P., and Brown, G. G. (2007). Soil biodiversity for agricultural sustainability. Agric. Ecosyst. Environ. 121, 33–244.
Bulgarelli, D., Garrido-Oter, R., Münch, P., Weiman, A., Droge, J., Pan, Y., et al. (2015). Structure and function of the bacterial root microbiota in wild and domesticated barley. Cell Host Microbe 17, 392–403. doi: 10.1016/j.chom.2015.01.011
Bulgarelli, D., Rott, M., Schlaeppi, K., Van Themaat, E., Ahmadinejad, N., Assenza, F., et al. (2012). Revealing structure and assembly cues for Arabidopsis root-inhabiting bacterial microbiota. Nature 488, 91–95. doi: 10.1038/nature11336
Bulgarelli, D., Schlaeppi, K., Spaepen, S., Themaat, E., and Schulze, P. (2013). Structure and functions of the bacterial microbiota of plants. Annu. Rev. Plant Biol. 64, 807–838. doi: 10.1146/annurev-arplant-050312-120106
Chaparro, J. M., Badri, D. V., and Vivanco, J. M. (2014). Rhizosphere microbiome assemblage is affected by plant development. ISME J. 8, 790–803. doi: 10.1038/ismej.2013.196
Chaumeil, P. A., Mussig, A. J., and Hugenholtz, P. (2019). GTDB-Tk: a toolkit to classify genomes with the Genome Taxonomy Database. Bioinformatics 36, 1925–1927. doi: 10.1093/bioinformatics/btz848
Chen, K., and Pachter, L. (2005). Bioinformatics for whole-genome shotgun sequencing of microbial communities. PLoS Comput. Biol. 1, 106–112. doi: 10.1371/journal.pcbi.0010024
Cheng, J., Yang, S., Palaniyandi, S., Han, J., Yoon, T., Kim, J., et al. (2010). Azalomycin F complex is an antifungal substance produced by Streptomyces malaysiensis MJM1968 isolated from agricultural soil. J. Korean Soc. Appl. Biol. Chem. 53, 545–552. doi: 10.3839/jksabc.2010.084
Corredor-Moreno, P., Minter, F., Davey, P., Wegel, E., Kular, B., Brett, P., et al. (2021). The branched-chain amino acid aminotransferase TaBCAT1 modulates amino acid metabolism and positively regulates wheat rust susceptibility. Plant Cell 33, 1728–1747. doi: 10.1093/plcell/koab049
Deng, L., Mao, X., Liu, D., Ning, X. Q., and Luo, H. B. (2020). Comparative analysis of physicochemical properties and microbial composition in high-temperature Daqu with different colors. Front. Microbiol. 11:588117. doi: 10.3389/fmicb.2020.588117
Desrochers, N., Walsh, J., Renaud, J., Seifert, K., and Sumarah, M. (2020). Metabolomic profiling of fungal pathogens responsible for root rot in American Ginseng. Metabolites 10:35. doi: 10.3390/metabo10010035
Devanna, B. N., Jaswal, R., Singh, P. K., Kapoor, R., Jain, P., Kumar, G., et al. (2021). Role of transporters in plant disease resistance. Physiol. Plant. 171, 849–867. doi: 10.1111/ppl.13377
Dhara, A., and Raichaudhuri, A. (2021). ABCG transporter proteins with beneficial activity on plants. Phytochemistry 184:112663. doi: 10.1016/j.phytochem.2021.112663
Ding, L., Xu, L., Chu, X., Yang, L., Zhu, H., and Huang, J. (2021). Dissimilarity analysis of microbial communities in the rhizosphere and tissues of diseased and healthy cherry trees (Cerasus pseudocerasus). Can. J. Plant Pathol. 43, 612–621. doi: 10.1080/07060661.2020.1861101
Dong, F. Z., Liu, Z. W., and Le, L. T. (1988). Panax notoginseng in Yunnan. Kunming, CHN: Yunnan Science and Technology Press, 103–114.
Dong, L., Xu, J., Feng, G., Li, X., and Chen, S. (2016). Soil bacterial and fungal community dynamics in relation to Panax notoginseng death rate in a continuous cropping system. Sci. Rep. 6:31802. doi: 10.1038/srep31802
Douglas, A. E. (1993). The nutritional quality of phloem sap utilized by natural aphid populations. Ecol. Entomol. 18, 31–38.
Doyle, J. J., and Doyle, J. L. (1987). A rapid DNA isolation procedure for small quantities of fresh leaf tissue. Phytochem. Bull. 19, 11–15.
Duan, Y., Zhou, L., Hall, D. G., Li, W., and Gottwald, T. (2009). Complete genome sequence of citrus huanglongbing bacterium, ‘Candidatus Liberibacter asiaticus’ obtained through metagenomics. Mol. Plant Microbe Interact. 22, 1011–1020. doi: 10.1094/MPMI-22-8-1011
Edwards, J., Johnson, C., Santos-Medellín, C., Lurie, E., and Sundaresan, V. (2015). Structure, variation, and assembly of the root-associated microbiomes of rice. Proc. Natl. Acad. Sci. U. S. A. 112, E911–E920. doi: 10.1073/pnas.1414592112
Errico, G., Marra, R., Crescenzi, A., Davino, S., Fanigliulo, A., Woo, S., et al. (2019). Integrated management strategies of Meloidogyne incognita and Pseudopyrenochaeta lycopersici on tomato using a Bacillus firmus-based product and two synthetic nematicides in two consecutive crop cycles in greenhouse. Crop Prot. 122, 159–164.
Fan, Z. Y., Miao, C. P., Qiao, X. G., Zheng, Y. K., Chen, H. H., Chen, Y. W., et al. (2016). Diversity, distribution, and antagonistic activities of rhizobacteria of Panax notoginseng. J. Ginseng Res. 40, 97–104. doi: 10.1016/j.jgr.2015.05.003
Farh, E., Kim, Y., Abbai, R., Singh, P., Jung, K., Kim, Y., et al. (2020). Pathogenesis strategies and regulation of ginsenosides by two species of Ilyonectria in Panax ginseng: power of speciation. J. Ginseng Res. 44, 332–340. doi: 10.1016/j.jgr.2019.02.001
Franzosa, E. A., Mciver, L. J., Rahnavard, G., Thompson, L. R., Schirmer, M., Weingart, G., et al. (2018). Species-level functional profiling of metagenomes and metatranscriptomes. Nat. Methods 15, 962–968. doi: 10.1038/s41592-018-0176-y
Fu, L., Niu, B., Zhu, Z., Wu, S., and Li, W. (2012). CD-HIT: accelerated for clustering the next-generation sequencing data. Bioinformatics 28, 3150–3152. doi: 10.1093/bioinformatics/bts565
Gaulin, E., Jacquet, C., Bottin, A., and Dumas, B. (2007). Root rot disease of legumes caused by Aphanomyces euteiches. Mol. Plant Pathol. 8, 539–548. doi: 10.1111/j.1364-3703.2007.00413.x
Gibson, M. K., Forsberg, K. J., and Dantas, G. (2015). Improved annotation of antibiotic resistance determinants reveals microbial resistomes cluster by ecology. ISME J. 9, 207–216. doi: 10.1038/ismej.2014.106
Godebo, A., Germida, J., and Walley, F. (2020). Isolation, identification, and assessment of soil bacteria as biocontrol agents of pea root rot caused by Aphanomyces euteiches. Can. J. Soil Sci. 100, 206–216.
Gong, C., Cheng, M., Li, J., Chen, H., and Zhang, Z. Z. (2020). Alpha-subunit of the chloroplast ATP synthase of tomato reinforces the resistance to grey mold and broad-spectrum resistance in transgenic tobacco. Phytopathology 111, 485–495. doi: 10.1094/PHYTO-06-20-0242-R
Goss, E. M., Tabima, J. F., Cooke, D., Restrepo, S., Fry, W. E., Forbes, G. A., et al. (2014). The Irish potato famine pathogen Phytophthora infestans originated in central Mexico rather than the Andes. Proc. Natl. Acad. Sci. U. S. A. 111, 8791–8796. doi: 10.1073/pnas.1401884111
Gottel, N. R., Castro, H. F., Kerley, M., Yang, Z., Pelletier, D. A., Podar, M., et al. (2011). Distinct microbial communities within the endosphere and rhizosphere of Populus deltoides roots across contrasting soil types. Appl. Environ. Microbiol. 77, 5934–5944. doi: 10.1128/AEM.05255-11
Guo, W. Q., Chen, Y. G., Shi, R. Z., He, K., and Gao, J. L. (2020). 20(S)-Protopanaxdiol suppresses the abnormal granule-monocyte differentiation of hematopoietic stem cells in 4T1 breast cancer-bearing mouse. Evid. Based Complement. Alternat. Med. 2020:8747023. doi: 10.1155/2020/8747023
Ha, N. T., Minh, T. Q., Hoi, P. X., Thuy, N., and Long, H. H. (2018). Biological control of potato tuber soft rot using N-acyl-L-homoserine aactone-degrading endophytic bacteria. Curr. Sci. 115, 1921–1927. doi: 10.18520/cs/v115/i10/1921-1927
Ha, X., Koopmann, B., and Von Tiedemann, A. (2016). Wheat blast and fusarium head blight display contrasting interaction patterns on ears of wheat genotypes differing in resistance. Phytopathology 106, 270–281. doi: 10.1094/PHYTO-09-15-0202-R
Hartman, K., Van, G. A., Roussely-Provent, V., Walser, J. C., and Schlaeppi, K. (2017). Deciphering composition and function of the root microbiome of a legume plant. Microbiome 5:2. doi: 10.1186/s40168-016-0220-z
Hong, B. G., Xiu, M. C., An, N., and Ge, P. C. (2010). Sanchi ginseng (Panax notoginseng (Burkill) F. H. Chen) in China: distribution, cultivation and variations. Genet. Resour. Crop Evol. 57, 453–460. doi: 10.1007/s10722-010-9531-2
Hsu, S., Shen, M., Chen, J., Lur, H., and Liu, C. (2021). The photosynthetic bacterium Rhodopseudomonas palustris strain PS3 exerts plant growth-promoting effects by stimulating nitrogen uptake and elevating auxin levels in expanding leaves. Front. Plant Sci. 12:573634. doi: 10.3389/fpls.2021.573634
Huson, D. H., and Buchfink, B. (2015). Fast and sensitive protein alignment using DIAMOND. Nat. Methods 12, 59–60. doi: 10.1038/nmeth.3176
Hwang, J., Song, W., Hong, D., Ko, D., Yamaoka, Y., Jang, S., et al. (2016). Plant ABC transporters enable many unique aspects of a terrestrial plant’s lifestyle. Mol. Plant 9, 338–355. doi: 10.1016/j.molp.2016.02.003
Hyatt, D., LoCascio, P. F., Hauser, L., and Uberbacher, E. (2012). Gene and translation initiation site prediction in metagenomic sequences. Bioinformatics 28, 2223–2230. doi: 10.1093/bioinformatics/bts429
Ichinose, Y., Nishimura, T., Harada, M., Kashiwagi, R., and Matsui, H. (2020). Role of two sets of RND-type multidrug efflux pump transporter genes, mexAB-oprM and mexEF-oprN, in Virulence of Pseudomonas syringae pv. tabaci 6605. Plant Pathol. J. 36, 148–156. doi: 10.5423/PPJ.OA.11.2019.0273
Iquebal, M. A., Tomar, R. S., Parakhia, M. V., Singla, D., Jaiswal, S., Rathod, V. M., et al. (2017). Draft whole genome sequence of groundnut stem rot fungus Athelia rolfsii revealing genetic architect of its pathogenicity and virulence. Sci. Rep. 7:5299. doi: 10.1038/s41598-017-05478-8
Jaime, H. C., Kristoffer, F., Pedro, C. L., Damian, S., Juhl, J. L., and Christian, V. M. (2016). Fast genome-wide functional annotation through orthology assignment by eggNOG-Mapper. Mol. Biol. Evol. 34, 2115–2122. doi: 10.1093/molbev/msx148
Jeong, Y., Kim, J., Kim, S., Kang, Y., Nagamatsu, T., and Hwang, I. (2003). Toxoflavin produced by Burkholderia glumae causing rice grain rot is responsible for inducing bacterial wilt in many field crops. Plant Dis. 87, 890–895. doi: 10.1094/PDIS.2003.87.8.890
Ji, W., Xue, L., Jin, Z., and Li, H. (2018). Plant growth promoting bacteria increases biomass, effective constituent, and modifies rhizosphere bacterial communities of Panax ginseng. Soil Plant Sci. 69, 1–12. doi: 10.1007/978-3-319-13921-0_1
Johnson, J., and Jumpponen, A. (2005). Can rDNA analyses of diverse fungal communities in soil and roots detect effects of environmental manipulations–a case study from tallgrass prairie. Mycologia 97, 1177–1194. doi: 10.3852/mycologia.97.6.1177
Kang, D., Li, F., Kirton, E., Thomas, A., Egan, R., An, H., et al. (2019). MetaBAT 2: an adaptive binning algorithm for robust and efficient genome reconstruction from metagenome assemblies. PeerJ 7:e7359. doi: 10.7717/peerj.7359
Koh, K. H., and Tham, F. Y. (2011). Screening of traditional Chinese medicinal plants for quorum-sensing inhibitors activity. J. Microbiol. 44, 144–148. doi: 10.1016/j.jmii.2009.10.001
Kster, J., and Rahmann, S. (2018). Snakemake—a scalable bioinformatics workflow engine. Bioinformatics 28, 2520–2522.
Kunakom, S., and Eustáquio, A. E. (2019). Burkholderia as a source of natural products. J. Nat. Prod. 82, 2018–2037. doi: 10.1021/acs.jnatprod.8b01068
Kwak, M. J., Kong, H. G., Choi, K., Kwon, S. K., and Kim, J. F. (2018). Rhizosphere microbiome structure alters to enable wilt resistance in tomato. Nat. Biotechnol. 36, 1100–1109. doi: 10.1038/nbt.4232
Langmead, B., and Salzberg, S. (2012). Fast gapped-read alignment with Bowtie 2. Nat. Methods 9, 357–359. doi: 10.1038/nmeth.1923
Li, D., Liu, C., Luo, R., Sadakane, K., and Lam, T. (2015). MEGAHIT: an ultra-fast single-node solution for large and complex metagenomics assembly via succinct de Bruijn graph. Bioinformatics 31, 1674–1676. doi: 10.1093/bioinformatics/btv033
Li, S., Bryan, M., David, L., and Ji, P. (2018). The interactomic analysis reveals pathogenic protein networks in Phomopsis longicolla underlying seed decay of soybean. Front. Genet. 9:104. doi: 10.3389/fgene.2018.00104
Liang, F., Zhang, B., Yang, Q., Zhang, Y., and Wu, X. (2020). Cyclic-di-GMP regulates the quorum-sensing system and the biocontrol activity of Pseudomonas fluorescens 2P24 through RsmA and RsmE proteins. Genet. Mol. Biol. 86, e02016–20. doi: 10.1128/AEM.02016-20
Liang, Y., Li, Z., Zhang, Y., and Meng, F. (2020). Nbnrp1 mediates Verticillium dahliae effector PevD1-triggered defense responses by regulating sesquiterpenoid phytoalexins biosynthesis pathway in Nicotiana benthamiana. Gene 768:145280. doi: 10.1016/j.gene.2020.145280
Liang, Z., Huang, L., He, F., Zhou, X., and Zhang, L. H. (2019). A substrate-activated efflux pump, DesABC, confers zeamine resistance to Dickeya zeae. mBio 10, e00713–19. doi: 10.1128/mBio.00713-19
Lind, A. L., and Pollard, K. S. (2021). Accurate and sensitive detection of microbial eukaryotes from whole metagenome shotgun sequencing. Microbiome 9:58. doi: 10.1186/s40168-021-01015-y
Liu, X., Bolla, K., Ashforth, E. J., Zhuo, Y., Gao, H., Pei, H., et al. (2012). Systematics-guided bioprospecting for bioactive microbial natural products. Antonie Van Leeuwenhoek 101, 55–66. doi: 10.1007/s10482-011-9671-1
Liu, X., Zhang, Y., Wu, M., Ma, Z., Huang, Z., Tian, F., et al. (2020). The scientific elucidation of daodi medicinal materials. Chin. Med. 15, 1–11. doi: 10.1186/s13020-020-00367-1
Lundberg, D. S., Lebeis, S. L., Paredes, S. H., Yourstone, S., Gehring, J., Malfatti, S., et al. (2012). Defining the core Arabidopsis thaliana root microbiome. Nature 488, 86–90. doi: 10.1038/nature11237
Ma, Y. N., Chen, C. J., Li, Q. Q., Xu, F. R., Cheng, Y. X., and Dong, X. (2019). Monitoring antifungal agents of artemisia annua against Fusarium oxysporum and Fusarium solani, associated with Panax notoginseng root-rot disease. Molecules 24:213. doi: 10.3390/molecules24010213
Mao, Z. S., Long, Y. J., Zhu, Y. Y., Zhu, S. S., and Chen, Z. J. (2014). First Report of Cylindrocarpon destructans var. destructans causing black root rot of Sanqi (Panax notoginseng) in China. Plant Dis. 98:162. doi: 10.1094/PDIS-11-12-1104-PDN
Mendes, L. W., Raaijmakers, J. M., and Hollander, M. D. (2018). Influence of resistance breeding in common bean on rhizosphere microbiome composition and function. ISME J. 12, 212–224. doi: 10.1038/ismej.2017.158
Mi, C., Yang, R., Rao, J., Yang, S., Wei, F., Li, O., et al. (2017). Unveiling of dominant fungal pathogens associated with rusty root rot of Panax notoginseng based on multiple methods. Plant Dis. 101, 2046–2052. doi: 10.1094/PDIS-01-17-0135-RE
Miao, C. P., Mi, Q. L., Qiao, X. G., Zheng, Y. K., and Zhao, L. X. (2016). Rhizospheric fungi of Panax notoginseng: diversity and antagonism to host phytopathogens. J. Ginseng Res. 40, 127–134. doi: 10.1016/j.jgr.2015.06.004
Miao, Z. Q., Li, S. D., Liu, X. Z., Chen, Y. J., and Zhang, K. Q. (2006). The causal microorganisms of Panax notoginseng root rot disease. Sci. Agric. Sin. 39, 1371–1378.
Nookongbut, P., Kantachote, D., Khuong, N., and Tantirungkij, M. (2020). The biocontrol potential of acid-resistant Rhodopseudomonas palustris KTSSR54 and its exopolymeric substances against rice fungal pathogens to enhance rice growth and yield. Biol. Control 150:104354. doi: 10.1016/j.biocontrol.2020.104354
Ondov, B. D., Bergman, N. H., and Phillippy, A. M. (2011). Interactive metagenomic visualization in a Web browser. BMC Bioinformatics 12:385. doi: 10.1186/1471-2105-12-385
Palaniyandi, S. A., Yang, S. H., Cheng, J. H., Meng, L., and Suh, J. W. (2011). Biological control of anthracnose (Colletotrichum gloeosporioides) in yam by Streptomyces sp.MJM5763. J. Appl. Microbiol. 111, 443–455. doi: 10.1111/j.1365-2672.2011.05048.x
Palaniyandi, S. A., Yang, S. H., Zhang, L., and Suh, J. W. (2013). Effects of actinobacteria on plant disease suppression and growth promotion. Appl. Microbiol. Biotechnol. 97, 9621–9636. doi: 10.1007/s00253-013-5206-1
Panpatte, D. G., Shelat, H. N., Jhala, Y. K., and Vyas, R. V. (2020). Fortified bacterial consortium – A novel approach to control root knot nematode in cucumber (cucumis sativum). Biol. Control 155:104528. doi: 10.1016/j.biocontrol.2020.104528
Parks, D. H., Imelfort, M., Skennerton, C. T., Hugenholtz, P., and Tyson, G. W. (2015). CheckM: assessing the quality of microbial genomes recovered from isolates, single cells, and metagenomes. Genome Res. 25, 1043–1055. doi: 10.1101/gr.186072.114
Parks, D. H., Tyson, G. W., Philip, H., and Beiko, R. G. (2014). STAMP: statistical analysis of taxonomic and functional profiles. Bioinformatics 30, 3123–3124. doi: 10.1093/bioinformatics/btu494
Patro, R., Duggal, G., Love, M., Irizarry, R., and Kingsford, C. (2017). Salmon provides fast and bias-aware quantification of transcript expression. Nat. Methods 14, 417–419. doi: 10.1038/nmeth.4197
Peiffer, J. A., Spor, A., Koren, O., Jin, Z., Tringe, S. G., Dangl, J. L., et al. (2013). Diversity and heritability of the maize rhizosphere microbiome under field conditions. Proc. Natl. Acad. Sci. U. S. A. 110, 6548–6553. doi: 10.1073/pnas.1302837110
R Core Team (2015). R: a Language And Environment For Statistical Computing. Vienna: R: Foundation for Statistical Computing.
R Core Team (2020). R: a Language And Environment For Statistical Computing. Vienna, Austria: R Foundation for Statistical Computing.
Richter, D. J., Berney, C., Strassert, J., Burki, F., and Vargas, C. D. (2020). EukProt: a database of genome-scale predicted proteins across the diversity of eukaryotic life. Cold Spring Harb. Lab. 2020:180687.
Rico, A., and Preston, G. (2008). Pseudomonas syringae pv. tomato DC3000 uses constitutive and apoplast-induced nutrient assimilation pathways to catabolize nutrients that are abundant in the tomato apoplast. Mol. Plant Microbe Interact. 21, 269–282. doi: 10.1094/MPMI-21-2-0269
Samac, D., Willert, A., Mcbride, M., and Kinkel, L. (2003). Effects of antibiotic-producing Streptomyces on nodulation and leaf spot in alfalfa. Appl. Soil Ecol. 22, 55–66. doi: 10.1016/s0929-1393(02)00109-9
Santhakumar, A., Battino, M., and Alvarez-Suarez, J. M. (2018). Dietary polyphenols: structures, bioavailability and protective effects against atherosclerosis. Food Chem. Toxicol. 113, 49–65. doi: 10.1016/j.fct.2018.01.022
Segata, N., Izard, J., and Waldron, L. (2011). Metagenomic biomarker discovery and explanation. Genome Biol. 12:R60. doi: 10.1186/gb-2011-12-6-r60
Silby, M. W., Cerde-Tárraga, A., Vernikos, G. S., Giddens, S. R., Jackson, R. W., and Preston, G. M. (2009). Genomic and genetic analyses of diversity and plant interactions of Pseudomonas fluorescens. Genome Biol. 10:R51. doi: 10.1186/gb-2009-10-5-r51
Siriyong, T., Srimanote, P., Chusri, S., and Yingyongnarongkul, B. E. (2017). Conessine as a novel inhibitor of multidrug efflux pump systems in Pseudomonas aeruginosa. BMC Complement. Aaltern. Med. 17:405. doi: 10.1186/s12906-017-1913-y
Sreevidya, M., Gopalakrishnan, S., Kudapa, H., and Varshney, R. K. (2016). Exploring plant growth-promotion actinomycetes from vermicompost and rhizosphere soil for yield enhancement in chickpea. Braz. J. Microbiol. 47, 85–95. doi: 10.1016/j.bjm.2015.11.030
Su, P., Tan, X., Li, C., Zhang, D., Cheng, J., Zhang, S., et al. (2017). Photosynthetic bacterium Rhodopseudomonas palustris GJ-22 induces systemic resistance against viruses. Microb. Biotechnol. 10, 612–624. doi: 10.1111/1751-7915.12704
Sumaira, A., Basharat, A., and Imran, S. (2016). Screening of rhizospheric actinomycetes for various in-vitro and in-vivo plant growth promoting (PGP) traits and for agroactive compounds. Front. Microbiol. 7:1334. doi: 10.3389/fmicb.2016.01334
Taheri, A. E., Chatterton, S., and Gossen, B. (2017). Metagenomic analysis of oomycete communities from the rhizosphere of field pea on the Canadian prairies. Can. J. Microbiol. 63, 758–768. doi: 10.1139/cjm-2017-0099
Truong, D. T., Franzosa, E. A., Tickle, T. L., Scholz, M., Weingart, G., Pasolli, E., et al. (2015). MetaPhlAn2 for enhanced metagenomic taxonomic profiling. Nat. Methods 12, 902–903. doi: 10.1038/nmeth.3589
Uritskiy, G. V., DiRuggiero, J., and Taylor, J. (2018). MetaWRAP—a flexible pipeline for genome-resolved metagenomic data analysis. Microbiome 6:158. doi: 10.1186/s40168-018-0541-1
Vendan, R. T., Lee, S. H., Yu, Y. J., and Rhee, Y. H. (2012). Analysis of bacterial community in the ginseng soil using denaturing gradient gel electrophoresis (DGGE). Indian J. Microbiol. 52, 286–288. doi: 10.1007/s12088-011-0193-3
Vincent, L., Hemalatha, G. R., Elodie, D., Coutinho, P. M., and Bernard, H. (2013). The carbohydrate-active enzymes database (CAZy) in 2013. Nucleic Acids Res. 42, D490–D495. doi: 10.1093/nar/gkt1178
Wang, J., Wang, R., Fang, H., Zhang, C., Zhang, F., Hao, Z., et al. (2020). Two VOZ transcription factors link an E3 ligase and an NLR immune receptor to modulate immunity in rice. Mol. Plant 14, 253–266. doi: 10.1016/j.molp.2020.11.005
Wang, Q., Sun, H., Li, M., Xu, C., and Zhang, Y. (2020). Different age-induced changes in rhizosphere microbial composition and function of Panax ginseng in transplantation mode. Front. Plant Sci. 11:563240. doi: 10.3389/fpls.2020.563240
Wang, P. P., Ye, Y., Liu, D. Q., Cui, X. M., and Liu, Y. (2020). Application of Metagenomics in Plant Diseases Research. Biotechnol. Bull. 36, 146–154.
Wen, T., Zhao, M., Yuan, J., Kowalchuk, G. A., and Shen, Q. (2020). Root exudates mediate plant defense against foliar pathogens by recruiting beneficial microbes. Soil Ecol. Lett. 3, 42–51. doi: 10.1007/s42832-020-0057-z
Wood, D. E., Lu, J., and Langmead, B. (2019). Improved metagenomic analysis with Kraken 2. Genome Biol. 20:257. doi: 10.1186/s13059-019-1891-0
Wu, L., Li, Z., Li, J., Khan, M. A., Huang, W., Zhang, Z., et al. (2013). Assessment of shifts in microbial community structure and catabolic diversity in response to Rehmannia glutinosa monoculture. Appl. Soil Ecol. 67, 1–9. doi: 10.1016/j.apsoil.2013.02.008
Wu, Y. W., Simmons, B. A., and Singer, S. W. (2016). MaxBin 2.0: an automated binning algorithm to recover genomes from multiple metagenomic datasets. Bioinformatics 32, 605–607. doi: 10.1093/bioinformatics/btv638
Wu, Z., Hao, Z., Zeng, Y., Guo, L., Huang, L., and Chen, B. (2015). Molecular characterization of microbial communities in the rhizosphere soils and roots of diseased and healthy Panax notoginseng. Antonie Van Leeuwenhoek 108, 1059–1074. doi: 10.1007/s10482-015-0560-x
Xing, X., Guo, S., and Fu, J. (2010). Biodiversity and distribution of endophytic fungi associated with Panax quinquefolium L. cultivated in a forest reserve. Symbiosis 51, 161–166. doi: 10.1007/s13199-010-0062-6
Xu, Z., Wang, M., Du, J., Huang, T., and Chen, Y. (2020). Isolation of Burkholderia sp. HQB-1, a promising biocontrol bacteria to protect banana against Fusarium wilt through phenazine-1-carboxylic acid secretion. Front. Microbiol. 11:605152. doi: 10.3389/fmicb.2020.605152
Yang, H., Postel, S., Kemmerling, B., and Ludewig, U. (2014). Altered growth and improved resistance of Arabidopsis against Pseudomonas syringae by overexpression of the basic amino acid transporter AtCAT1. Plant Cell Environ. 37, 1404–1414. doi: 10.1111/pce.12244
Yang, M., Yuan, Y., Huang, H., Ye, C., Guo, C., Xu, Y., et al. (2019). Steaming combined with biochar application eliminates negative plant-soil feedback for sanqi cultivation. Soil Tillage Res. 189, 189–198. doi: 10.1016/j.still.2019.02.006
Yeoh, Y., Paungfoo-Lonhienne, C., Dennis, P., Robinson, N., Ragan, M., Schmidt, S., et al. (2016). The core root microbiome of sugarcanes cultivated under varying nitrogen fertilizer application. Environ. Microbiol. 18, 1338–1351. doi: 10.1111/1462-2920.12925
Zarraonaindia, I., Owens, S. M., Weisenhorn, P., West, K., and Gilbert, J. A. (2015). The soil microbiome influences grapevine-associated microbiota. mBio 6, e02527–14. doi: 10.1128/mBio.02527-14
Zhang, D., Li, W., Xia, E., Zhang, Q., Liu, Y., Zhang, Y., et al. (2017). The Medicinal herb Panax notoginseng genome provides insights into ginsenoside biosynthesis and genome evolution. Mol. Plant 10, 903–907. doi: 10.1016/j.molp.2017.02.011
Zhang, Y. M., Cheng, Y. X., Ma, Y. N., Chen, C. J., Xu, F. R., and Dong, X. (2018). Role of phenolic acids from the rhizosphere soils of Panax notoginseng as a double-edge sword in the occurrence of root-rot disease. Molecules 23:819.
Zhao, W., Hao, J., Xing, J., Rui, Y., and Wang, S. J. (2016). Proteomic analysis of phloem proteins leads to the identification of potential candidates for JA-mediated RKN-resistant elements in Solanum lycopersicum. J. Plant Growth Regul. 36, 1–10.
Zhao, Y. P., Lin, S., Chu, L., Gao, J. T., Azeem, S., and Lin, W. (2016). Insight into structure dynamics of soil microbiota mediated by the richness of replanted Pseudostellaria heterophylla. Sci. Rep. 6:26175. doi: 10.1038/srep26175
Zhao, Y. M., Cheng, Y. X., Ma, Y. N., Chen, C. J., and Xu, F. R. (2018). Role of phenolic acids from the rhizosphere soils of Panax notoginseng as a double-edge sword in the occurrence of root-rot disease. Molecules 23:819. doi: 10.3390/molecules23040819
Zia, R., Nawaz, M., Yousaf, S., Amin, I., Hakim, S., Mirza, M., et al. (2021). Seed inoculation of desert-plant growth-promoting rhizobacteria induce biochemical alterations and develop resistance against water stress in wheat. Physiol. Plant. 172, 990–1006. doi: 10.1111/ppl.13362
Zucchi, T. D., Almeida, L. G., and Dossi, F. (2010). Secondary metabolites produced by Propionicimonas sp. (ENT-18) induce histological abnormalities in the sclerotia of Sclerotinia sclerotiorum. BioControl 55, 811–819. doi: 10.1007/s10526-010-9295-9
Keywords: Panax notoginseng, root rot, rhizosphere soil, root endophytes, metagenomic sequencing, microbial community
Citation: Wang P, Yang L, Sun J, Yang Y, Qu Y, Wang C, Liu D, Huang L, Cui X and Liu Y (2022) Structure and Function of Rhizosphere Soil and Root Endophytic Microbial Communities Associated With Root Rot of Panax notoginseng. Front. Plant Sci. 12:752683. doi: 10.3389/fpls.2021.752683
Received: 03 August 2021; Accepted: 13 December 2021;
Published: 05 January 2022.
Edited by:
Bing Yang, Sichuan Academy of Giant Panda, ChinaReviewed by:
Peter Kusstatscher, Graz University of Technology, AustriaCopyright © 2022 Wang, Yang, Sun, Yang, Qu, Wang, Liu, Huang, Cui and Liu. This is an open-access article distributed under the terms of the Creative Commons Attribution License (CC BY). The use, distribution or reproduction in other forums is permitted, provided the original author(s) and the copyright owner(s) are credited and that the original publication in this journal is cited, in accordance with accepted academic practice. No use, distribution or reproduction is permitted which does not comply with these terms.
*Correspondence: Xiuming Cui, c2FucWkzN0B2aXAuc2luYS5jb20=; Yuan Liu, bGl1eXVhbjUxM0BrdXN0LmVkdS5jbg==
Disclaimer: All claims expressed in this article are solely those of the authors and do not necessarily represent those of their affiliated organizations, or those of the publisher, the editors and the reviewers. Any product that may be evaluated in this article or claim that may be made by its manufacturer is not guaranteed or endorsed by the publisher.
Research integrity at Frontiers
Learn more about the work of our research integrity team to safeguard the quality of each article we publish.