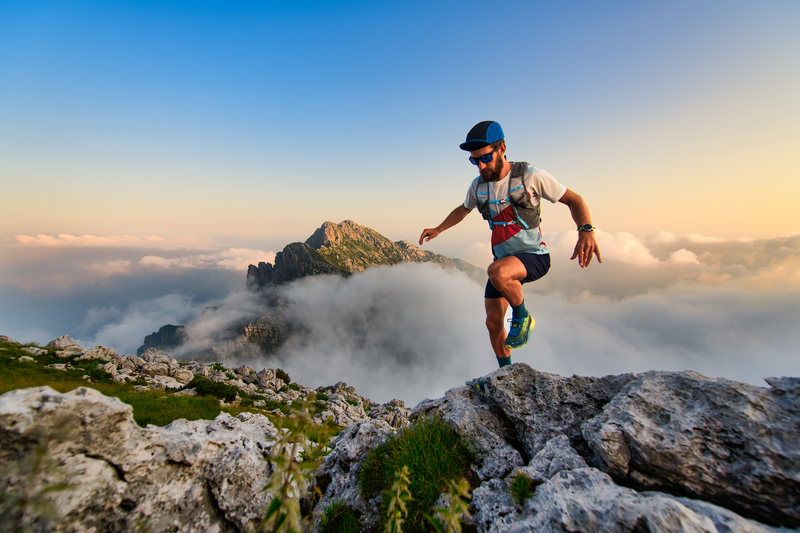
94% of researchers rate our articles as excellent or good
Learn more about the work of our research integrity team to safeguard the quality of each article we publish.
Find out more
SYSTEMATIC REVIEW article
Front. Physiol.
Sec. Exercise Physiology
Volume 16 - 2025 | doi: 10.3389/fphys.2025.1551645
The final, formatted version of the article will be published soon.
You have multiple emails registered with Frontiers:
Please enter your email address:
If you already have an account, please login
You don't have a Frontiers account ? You can register here
Introduction: The "no pain, no gain" philosophy has long influenced athletic training approaches, particularly in high-intensity workouts like metabolic resistance training (MRT). However, the necessity of discomfort-inducing training for optimal athletic performance remains debatable. This systematic review and meta-analysis examined whether MRT provided comparable or better results than traditional training methods in trained athletes.Methods: A systematic search of PubMed/MEDLINE, Web of Science, Scopus, and SPORTDiscus (Jan 2004 - Dec 2024) identified RCTs comparing MRT with traditional training in athletes. Two reviewers screened studies and assessed bias risk using Cochrane RoB 2. Random - effects meta - analyses were conducted for outcomes like VO2max, peak power, sprint performance, blood lactate, time to exhaustion, and jump height. GRADE was used to evaluate evidence certainty.Results: Eleven studies (n=276 participants) met inclusion criteria. MRT demonstrated a statistically significant improvement in sprint performance (SMD = 1.18, 95% CI: 0.00 to 2.36, p < 0.0001) and countermovement jump height (SMD = 0.80, 95% CI: -0.04 to 1.64, p = 0.0007), indicating notable gains in explosive power. VO2max improvements were observed (SMD = 0.30, 95% CI: -0.19 to 0.79, p = 0.10) but did not reach statistical significance. Peak power output showed a moderate but non-significant positive effect (SMD = 0.54, 95% CI: -2.05 to 3.13, p = 0.55), while blood lactate changes varied widely (SMD = -1.68, 95% CI: -8.58 to 5.22, p = 0.29), reflecting high heterogeneity across studies. Time to exhaustion presented a small positive effect (SMD = 0.23, 95% CI: 0.00 to 0.46, p = 0.18), but without statistical significance. Subgroup analyses revealed that younger adults (19-25 years) and experienced athletes benefited the most from MRT, with low-frequency training (≤2 sessions/week) yielding the most favorable adaptations. Moderator analysis confirmed that sprint performance had the strongest response to MRT, while aerobic measures exhibited more variability.Conclusion: The evidence demonstrates the capacity of MRT to enhance athletic performance comparable to or exceeding traditional training methods while requiring reduced time commitment. These findings suggest that optimal performance adaptations can be achieved through well-designed MRT protocols without necessitating excessive training volumes.
Keywords: metabolic resistance training (MRT), high-intensity interval training (HIIT), Athletic Performance, Athlete development, Meta-analysis
Received: 26 Dec 2024; Accepted: 25 Feb 2025.
Copyright: © 2025 Yu and Ding. This is an open-access article distributed under the terms of the Creative Commons Attribution License (CC BY). The use, distribution or reproduction in other forums is permitted, provided the original author(s) or licensor are credited and that the original publication in this journal is cited, in accordance with accepted academic practice. No use, distribution or reproduction is permitted which does not comply with these terms.
* Correspondence:
Chuanwei Ding, Capital Institute of Physical Education and Sports, Beijing, 100088, Beijing, China
Disclaimer: All claims expressed in this article are solely those of the authors and do not necessarily represent those of their affiliated organizations, or those of the publisher, the editors and the reviewers. Any product that may be evaluated in this article or claim that may be made by its manufacturer is not guaranteed or endorsed by the publisher.
Research integrity at Frontiers
Learn more about the work of our research integrity team to safeguard the quality of each article we publish.