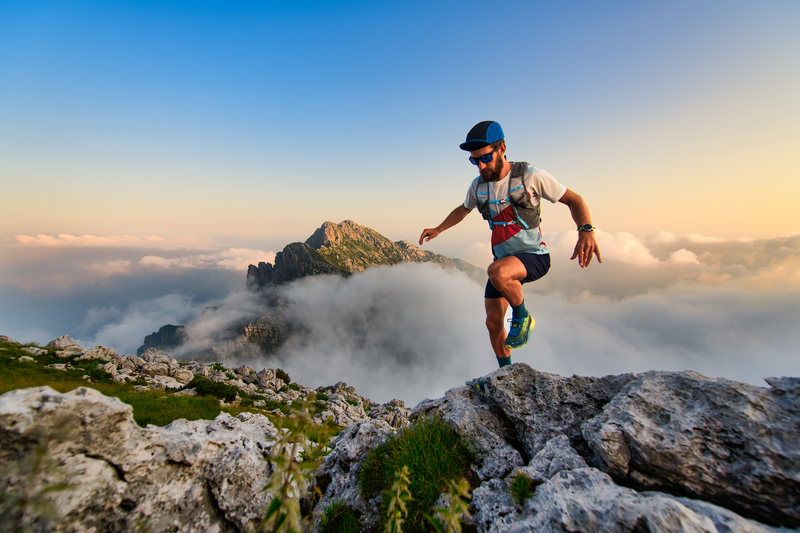
94% of researchers rate our articles as excellent or good
Learn more about the work of our research integrity team to safeguard the quality of each article we publish.
Find out more
ORIGINAL RESEARCH article
Front. Physiol.
Sec. Exercise Physiology
Volume 16 - 2025 | doi: 10.3389/fphys.2025.1547257
The final, formatted version of the article will be published soon.
You have multiple emails registered with Frontiers:
Please enter your email address:
If you already have an account, please login
You don't have a Frontiers account ? You can register here
Background: Muscle fatigue represents a primary manifestation of exercise-induced fatigue.Electromyography (EMG) serves as an effective tool for monitoring muscle activity, with EMG signal analysis playing a crucial role in assessing muscle fatigue. This paper introduces a machine learning approach to classify EMG signals for the automatic detection of muscle fatigue. Methods: Ten adult participants performed isometric contractions of lower limb muscles. The EMG signals were decomposed into multiple intrinsic mode functions (IMFs) using improved complementary ensemble empirical mode decomposition adaptive noise (ICEEMDAN). Timedomain, frequency-domain, time-frequency domain, and nonlinear features associated with muscle fatigue during isometric contraction were analyzed through EMG signals. Dimensionality reduction was achieved using t-distributed stochastic neighbor embedding (t-SNE), followed by machine learning-based classification of fatigue levels. Results: The findings indicated that EMG signal characteristics changed significantly with increasing fatigue. The combination of support vector machines (SVM) and ICEEMDAN achieved an impressive accuracy of 99.8%. Conclusion: The classification performance of this study surpasses that of existing state-of-the-art methods for detecting exercise-induced fatigue. Therefore, the proposed strategy is both valid and effective for supporting the detection of muscle fatigue in training, rehabilitation, and occupational settings.
Keywords: machine learning, isometric contractions, ICEEMDAN, t-SNE, Muscle Fatigue
Received: 23 Dec 2024; Accepted: 24 Feb 2025.
Copyright: © 2025 Sun, Zhang, Liu, cui, sun and Zhang. This is an open-access article distributed under the terms of the Creative Commons Attribution License (CC BY). The use, distribution or reproduction in other forums is permitted, provided the original author(s) or licensor are credited and that the original publication in this journal is cited, in accordance with accepted academic practice. No use, distribution or reproduction is permitted which does not comply with these terms.
* Correspondence:
Chunyan Zhang, Jilin University, Changchun, China
Disclaimer: All claims expressed in this article are solely those of the authors and do not necessarily represent those of their affiliated organizations, or those of the publisher, the editors and the reviewers. Any product that may be evaluated in this article or claim that may be made by its manufacturer is not guaranteed or endorsed by the publisher.
Research integrity at Frontiers
Learn more about the work of our research integrity team to safeguard the quality of each article we publish.