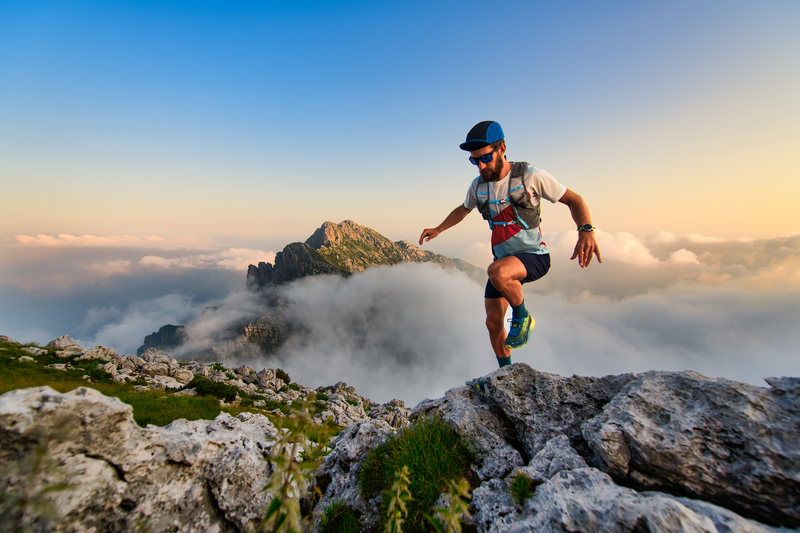
95% of researchers rate our articles as excellent or good
Learn more about the work of our research integrity team to safeguard the quality of each article we publish.
Find out more
ORIGINAL RESEARCH article
Front. Physiol. , 19 September 2024
Sec. Skeletal Physiology
Volume 15 - 2024 | https://doi.org/10.3389/fphys.2024.1403102
Background: The adult human skeleton is composed of cortical and cancellous bone. The proportions of these two types of bone tissue differ in various parts of the skeleton. The aim of this cross-sectional study was to quantify the determinants of bone mineral density (BMD) and bone mineral content in various regions of interest (ROIs) in smokers and never-smokers.
Methods: In this study, 4,332 bone scans of three regions of interest (ROIs) were analyzed: the forearm (distal and proximal), femur, and lumbar spine. Body composition and bone parameters were measured using dual-energy X-ray absorptiometry. Smoking was measured using the Global Adult Tobacco Survey questionnaire. Body mass index (BMI) was calculated, and physical activity (PA) was characterized by the metabolic equivalent of task (MET).
Results: Among women, the interaction between PA (positive β coefficient) and smoking (negative β coefficient) was a significant predictor of BMD in the distal and proximal forearm (adj. R2 = 0.40 and R2 = 0.58; p < 0.001). The interaction of three variables—age, smoking (negative β), and MET (positive β)—was significant for total hip BMD (adj. R2 = 0.54; p < 0.001). The interaction between BMI and MET (positive β) and smoking (negative β) was significant for BMD in the lumbar spine (adj. R2 = 0.62; p < 0.001). In men, the interaction between MET (positive β) and smoking (negative β) was significant for BMD in the forearm and lumbar spine (adj. R2 = 0.44, R2 = 0.46, and R2 = 0.49; p < 0.01). Smoking alone was a significant negative predictor of total hip BMD (adj. R2 = 0.34; p < 0.001).
Conclusion: Among both women and men, never-smokers had significantly better bone parameters than smokers. Smoking was a significant negative predictor for BMD in the various ROIs in both women and men. Physical activity was a significant positive predictor of BMD, with a strong association with bone parameters.
The adult human skeleton is composed of cortical and cancellous bone. The proportions of these two types of bone tissue differ in various parts of the skeleton. Cancellous bone predominates in the vertebrae, whereas long bones contain mostly cortical bone (Compston et al., 2019). Bone remodeling is a process by which old bone is replaced with new bone. During remodeling, voids and osteoid form temporarily because bone resorption and osteoid formation precede mineralized bone formation. The process of mineralization in the bone depends on the rate of bone turnover. A slower rate of bone turnover allows bone to accumulate more minerals before being resorbed in a successive remodeling cycle (Hernandez et al., 2001). Values of bone mineral density (BMD) and bone mineral content (BMC) are used to diagnose bone health. BMD is conditioned by many factors, including genetic predisposition. However, more and more research is focused on identifying modifiable lifestyle factors.
The current research shows that smoking may have detrimental effects on the skeletal system. Recent evidence demonstrates that tobacco smoking causes an imbalance in the mechanisms of bone turnover, leading to lower BMC and BMD, thus significantly increasing the risk of osteoporosis and fractures (Wong et al., 2007; Ward and Klesges, 2001a; Al-Bashaireh et al., 2018). However, reports on the association between cigarette smoking and BMD in men and women are contradictory and often affect only one location of the skeleton, axial or peripheral. From a population health perspective, it is important to know the determinants of BMD in smokers and to identify variables that can help repair the harmful effects of smoking on bone health.
Currently, the high quality of bone tissue measurements allows for detailed determination of mineral density at specific regions of interest (ROIs). The most common sites for measurements are the lumbar spine, proximal femur, forearm, and calcaneus. Each ROI correlates moderately well with the others and provides a reasonable prediction of the risk of generic osteoporosis and fractures. However, this correlation does not necessarily lead to consistent diagnostic classification of an individual when threshold-based criteria are used (Lu et al., 2001). Bone tissue is a living and active tissue. An analysis of the effect of multiple variables on bone density simultaneously in several ROIs can help further investigate their interactions and their mechanisms.
Therefore, in this cross-sectional study on a population-based cohort of adult Caucasian women and men of European origin, we aimed to quantify the determinants of bone mineral density and bone mineral content in various regions of interest among smokers and never-smokers.
This cross-sectional study comprised 4,332 bone scans of men (aged 39.3 ± 13.1 years) and women (aged 44.8 ± 12.5 years) from the Polish population (Caucasian ethnic group, of European origin), divided into three groups according to ROI: forearm (distal and proximal), femur, and lumbar spine. We analyzed 2,646 bone scans from never-smokers and 1,692 from smokers (current or former) at selected ROIs (Table 1).
Table 1. Number of DXA scans (n) in the various regions of interest (ROIs): forearm, femur, and lumbar spine across the groups and by smoking status.
The project was carried out in Warsaw, the capital of Poland. Recruitment for participation in the study was continuous. The criteria for participation included being an adult not diagnosed with or undergoing pharmacological treatment for chronic diseases and providing written consent. In the first stage, a health interview was conducted with each applicant. Subjects with contraindications to densitometric examination were excluded. The exclusion criteria also included hormone replacement therapy in women, kidney disease, cancers, rheumatoid arthritis, bone diseases, nutritional disorders, and long-term steroid treatment. All participants were informed about the aims and schedule of the study and received their test results along with an interpretation.
Body composition and bone parameters were measured using dual-energy X-ray absorptiometry (DXA) with a Norland XR-46 bone densitometer equipped with K edge filtering systems (Swissray-USA, Norland Medical Systems, Madison, WI, United States). Fat mass (FM), fat-free mass (FFM), bone mineral density (BMD), bone mineral content (BMC), and T-scores were measured. This study used the following ROIs for bone parameters: lumbar spine L1–L4, proximal femur (femoral neck, trochanter, and total hip), and distal and proximal parts of the forearm. The long-term precision error for a reference lumbar spine phantom expressed as a percentage coefficient of variance was less than 1% during the study period. All subjects in this study received total and subregional DXA scans. DXA scans were performed by certified radiology technicians. The long-term stability of the densitometer was assessed by daily phantom scans, as recommended by the manufacturer, and calibration standards. A standardized procedure for positioning and data analysis was established and followed for all scans. The effective dose (μSv) for DXA scans of the forearm was less than 1 μSv. The lumbar spine, hip, and whole-body scans each resulted in an effective dose of approximately 1 μSv. The body mass index (BMI) was calculated.
Smoking was measured with the Global Adult Tobacco Survey questionnaire (GATS), section B, on active smoking (AS). A direct interview was conducted by a trained interviewer with extensive experience in collecting data using this method and the GATS questionnaire. The survey was conducted following the guidelines of WHO experts and the GATS methodology used in Poland, including a standard protocol for the interview questionnaires, sample weights, data management, analysis, reporting, and information release. In Poland, the Ministry of Health revised and approved the questionnaires and also appointed two committees—the GATS Poland Scientific Committee and the GATS Poland Steering Committee—that handle the scientific and technical coordination of the nationally representative GATS survey (Kaleta et al., 2009). The following data were collected: smoking status (never-smokers/smokers), smoking duration (number of years of smoking), the number of cigarettes smoked per day, and pack-years of smoking.
The International Physical Activity Questionnaire (IPAQ) was used to assess the present level of physical activity in a direct interview, as recommended in international studies such as the European Health Interview Survey (EUROHIS) and the European Physical Activity Surveillance System (EUPASS). Weekly physical activity was calculated by adding up the values for the metabolic equivalent of task (MET, min/week) obtained during vigorous activity, moderate activity, and walking performed throughout the entire week (Lee et al., 2011).
All statistical analyses were conducted using STATISTICA software (v.12, StatSoft, United States). The normality of the data distribution was assessed using the Shapiro–Wilk test for each variable. In cases where the data were not normally distributed, the Box–Cox transformation was applied. Effect sizes were calculated using Cohen’s d = 2t//(df1/2), (small effect: <0.5; medium effect: 0.5–0.8; large effect: >0.8). To assess the impact of selected variables on BMD and BMC, an analysis of covariance (ANCOVA) was performed. The analysis included covariates such as age, body mass index (BMI), fat mass (FM), fat-free mass (FFM), number of cigarettes smoked per day, and physical activity level measured by the metabolic equivalent of task (MET). Multiple regression analysis was used to identify predictors of BMD and BMC in several ROIs. Statistical significance was set at p < 0.01 and p < 0.001 for all analyses.
Table 2 presents the baseline characteristics of the study group by various ROIs, sex, and smoking status. The analysis of particular variables among the women, taking into account smoking status, showed several significant differences. Significantly higher values (p < 0.001) of all bone parameters (BMD, BMC, and T-score) in all ROIs, as well as MET (min/week), were noted among female never-smokers than among smokers (large effect). Among the group with forearm ROI, the female never-smokers had significantly higher body weight (3.3 kg higher; p < 0.01; small effect) and fat-free mass (2.9 kg; p < 0.001; medium effect) than the smokers. In the group with the forearm ROI, the female never-smokers had significantly higher fat-free mass (1.6 kg higher; p < 0.001; small effect) than the smokers. In the group with the femur ROI, the female never-smokers had significantly higher fat mass (5.3 kg higher; p < 0.001; medium effect) and significantly lower fat-free mass (2.1 kg lower; p < 0.01; medium effect) than the smokers.
Table 2. Baseline characteristics of the study population by various regions of interest (ROIs) and smoking status.
Similar analyses were carried out in the group of men. As in the group of women, significantly higher values (p < 0.01 and p < 0.001) of all bone parameters (BMD, BMC, and T-score) in all ROIs, as well as MET (min/week), were noted in the male never-smokers than in the smokers (in all ROIs, there were large effects except for trochanter BMC, in which there was a small effect). In the group with the forearm ROI, the male never-smokers had significantly higher levels of all somatic parameters (p < 0.001; medium effect) than the smokers. In the group with the femur ROI, the male never-smokers had significantly higher fat-free mass (3.2 kg higher; p < 0.01; medium effect) than the smokers (Table 2).
The results of the covariance analysis (ANCOVA) between BMD in the various ROIs and selected parameters are presented in Table 3. Covariance analysis indicated that, in the women, the main parameters that significantly impact BMD in the distal forearm were MET (min/week) and cigarettes/day (adj.R2 = 0.43). In contrast, physical activity significantly influenced BMD in the proximal forearm and total hip (adj. R2 = 0.60, adj. R2 = 0.56). Fat-free mass and MET significantly influenced the BMD of the lumbar spine (adj. R2 = 0.65). Similarly, the analysis of the men indicated that the main parameters that significantly influenced the BMD of the distal forearm were BMI, FM, FFM, and MET (adj. R2 = 0.48). Physical activity significantly influenced BMD in the proximal forearm and lumbar spine (adj. R2 = 0.46; adj. R2 = 0.51) (Table 3).
Table 3. Relationships between BMD (g/cm2) in the various regions of interest (ROIs) and BMI, body composition, physical activity, and smoking status in men and women (results of ANCOVA, F test, p).
The results of the covariance analysis (ANCOVA) between BMC in the various ROIs and selected parameters are presented in Table 4. The ANCOVA indicated that, in the women, the main parameters that significantly influenced BMC in the distal forearm were FFM, MET, and cigarettes/day (adj. R2 = 0.46). FFM and MET influenced BMC in the proximal forearm and total hip (adj. R2 = 0.50; adj. R2 = 0.48). Four variables, BMI, FM, FFM, and MET, significantly influenced the BMC lumbar spine (adj. R2 = 0.54). Similarly, the analysis of the men indicated that the two parameters, FFM and MET, influenced BMC in the distal forearm (adj. R2 = 0.36). In contrast, only physical activity influenced BMD in the proximal forearm (adj. R2 = 0.52). Two variables, FFM and the number of cigarettes smoked per day, influenced the BMC of the total hip (adj. R2 = 0.44) (Table 4).
Table 4. Relationships between BMC (g) in the various regions of interest (ROIs) and BMI, body composition, physical activity, and smoking status in men and women (results of ANCOVA, F test, p).
The results of the multiple regression analysis are presented in Tables 5, 6. The model explains 34%–62% (adj. R2 = 0.34–0.62) of the variance in BMD determinants in four ROIs (distal forearm, proximal forearm, total hip, and lumbar spine) in both men and women. Among the women, the predictors of interactions of physical activity (positive direction of β coefficient) and smoking (negative direction) were significant for BMD of the distal and proximal forearm (adj. R2 = 0.40 and R2 = 0.58; p < 0.001). It was also found that the predictors of interactions of three variables—age, smoking (negative directions), and MET (positive direction)—were significant for total hip BMD (adj. R2 = 0.54; p < 0.001). Furthermore, the predictors of interactions of BMI and MET (positive direction) and smoking (negative direction) were significant for BMD of the total lumbar spine (adj. R2 = 0.62; p < 0.001). Among the men, the predictors of interactions of physical activity (positive direction) and smoking (negative direction) were significant for BMD in the two forearm ROIs and the lumbar spine (adj. R2 = 0.44, R2 = 0.46, R2 = 0.49; p < 0.01). Smoking itself was a significant predictor (negative value of the standardized β coefficient) for total hip BMD (adj. R2 = 0.34; p < 0.001) (Table 5).
Table 5. Determinant of BMD (g/cm2) in the various regions of interest (ROIs)—results of multiple regression.
Table 6. Determinant of BMC (g) in the various regions of interest (ROIs)—results of multiple regression.
The regression analysis model was also used to evaluate the determinants of BMC (Table 6). The model explains 33%–51% (adj. R2 = 0.33–0.51) of the variance. Among the women, the predictors of two interactions—physical activity (positive direction) and smoking (negative direction of β coefficient)—were significant for BMD of the distal and proximal forearm as well as the lumbar spine (adj. R2 = 0.42, R2 = 0.47, and R2 = 42; p < 0.001). It was also found that the predictors of interactions of five variables—age, BMI, smoking (negative directions), fat mass, and MET (positive direction)—were significant for total hip BMD (adj. R2 = 0.44; p < 0.001).
Among men, the predictors of two interactions—physical activity (positive direction) and smoking (negative direction)—were significant for distal BMC (adj. R2 = 0.33; p < 0.001). It was also found that the predictors of interactions of three variables—smoking (negative directions), fat mass, and MET (positive direction)—were significant for BMC of the proximal forearm (adj. R2 = 0.51; p < 0.01). Furthermore, the predictors of interactions of BMI and smoking (negative direction) and fat mass (positive direction) were significant for BMC of the total hip and lumbar spine (adj. R2 = 0.38, R2 = 0.34; p < 0.01) (Table 6).
Behavioral medicine indicates an ever-growing interest in the impact of lifestyle behaviors on health (Lopuszanska-Dawid, 2023; Kris-Etherton et al., 2022; Adams et al., 2016). As research indicates, bone tissue health also depends on a number of factors, including lifestyle (Proia et al., 2021; Weaver et al., 2016; Sanchez-Trigo et al., 2022; Watson et al., 2018; Kistler-Fischbacher et al., 2021). Peak bone mass is built up until approximately age 25 (Lu et al., 2016), and this process takes time. Similarly, factors that affect BMD do not show an immediate, short-term impact. Both the building of BMD and the decline of BMD are influenced by many variables over a long period of time. This study evaluated the variables of smoking, smoking duration, and physical activity, that is, determinants that interact in the long term, on BMD.
The study found that, regardless of gender, smokers had significantly lower values of all bone parameters (BMD, BMC, and T-score) in all ROIs, as well as lower MET than never-smokers. The study revealed the most important determinants of BMD in the various ROIs among both women and men. Among women, the main parameters that significantly influenced BMD in the distal forearm were MET and the number of cigarettes per day. Physical activity was the only factor that influenced BMD in the proximal forearm and total hip. Fat-free mass and MET influenced BMD in the lumbar spine. The analysis of the men indicated that the main parameters that significantly influenced BMD in the distal forearm were BMI, FM, FFM, and MET. Physical activity was the only factor that influenced BMD in the proximal forearm and lumbar spine.
Smoking is associated with an increased incidence of fractures and a higher risk of osteoporosis (Vestergaard and Mosekilde, 2003; Yoon et al., 2012), but previous studies have indicated that the mechanism by which smoking affects bone health remains unclear. Additionally, the impact of smoking on bone may differ between men and women (Yoon et al., 2012; Kohler et al., 2021). Smoking can affect bone status either directly or indirectly, but the effect depends on factors such as exposure time to the habit and the number of cigarettes smoked per day (Weng et al., 2022). In this study, the number of years of smoking and pack-years did not significantly affect BMD or BMC, but the number of cigarettes smoked per day did significantly affect BMD and BMC in the distal forearm in women and total hip BMC in men.
In postmenopausal Turkish women, the smokers showed significantly lower vertebra and femur neck BMD than nonsmokers. However, that study did not find a significant relationship between the duration of smoking, the number of cigarettes consumed per day, and BMD (Ugurlu et al., 2016). Another study evaluating the relationship between smoking, the number of cigarettes per day, the length of exposure to the drug, and bone parameters in young women found that BMD did not differ between never-smokers, ex-smokers, and current smokers (Callréus et al., 2013). Some studies have found no significant relationship between smoking and bone mineralization or bone mass (Ward and Klesges, 2001a).
The determinants of BMC were also analyzed. Among women, the main parameters that significantly influenced BMC in the distal forearm were FFM, MET, and cigarettes per day. Two variables—FFM and MET—influenced BMC in the proximal forearm and total hip. Four variables—BMI, FM, FFM, and MET—significantly influenced BMC in the lumbar spine. Similarly, the analysis of men indicated that FFM and MET significantly influenced BMC in the distal forearm. Physical activity was the only factor that influenced BMC in the proximal forearm. Two variables—FFM and the number of cigarettes smoked per day—significantly influenced BMC in the total hip. Recent research has shown that tobacco smoking causes an imbalance in bone turnover, leading to decreased BMC, which makes bones more vulnerable to osteoporosis and fractures. Smoking influences bone mass indirectly by altering body weight, the parathyroid hormone–vitamin D axis, adrenal hormones, and sex hormones, as well as increasing oxidative stress on bone tissue. Additionally, tobacco smoke directly affects BMC by impacting bone osteogenesis and angiogenesis (Al-Bashaireh et al., 2018). Cigarette smoking has deleterious effects on bone integrity. In several studies, a correlation has been shown between declining BMC and the number of cigarettes smoked per day and the years of exposure (Abate et al., 2013; Kanis et al., 2005; Tarantino et al., 2021).
In this study, for both men and women, interactions between physical activity (positive influence) and smoking (negative influence) significantly influenced BMD. Physical activity can be an important protective factor in bone health and osteoporosis prevention, as has been shown in many studies (Pinheiro et al., 2020; Ng et al., 2022; Kopiczko, 2020; Kopiczko et al., 2021). Physical activity, especially based on resistance exercises—with weights, multi-directional loading, and weight-bearing—has the most significant physiological effects on bone parameters (Hart et al., 2017; O'Bryan et al., 2022; Kemmler et al., 2020). In several randomized controlled trials, targeted high-impact exercise resulted in increased bone mineral density and bone strength during the prepubertal and peripubertal stages (Ng et al., 2022; Nikander et al., 2010; Hind and Burrows, 2007). Physical activity may be an important factor in protecting bone density in smokers and ex-smokers. Physical activity provides the necessary influence of forces generated from working muscles for proper mineralization. Adequate levels of physical activity affect the normal tissue composition of the body, especially muscle mass and lean body components (Deng et al., 2021; Shin et al., 2011; Lopez et al., 2022; Beaudart et al., 2017; Bao et al., 2020). In this study, FFM also correlated positively with bone parameters in several skeletal regions.
While the detrimental effects of smoking on bone health are well documented, the significance of conducting epidemiological research in this area cannot be overstated (Ward and Klesges, 2001b; Ratajczak et al., 2021). Epidemiological studies provide valuable insights at the population level. By analyzing large cohorts, such as the 4,332 bone scans in our study, we can identify the prevalence and extent of bone density reduction among smokers in a real-world setting. Additionally, such research helps to identify and quantify modifiable risk factors, like smoking and physical activity, that can inform public health interventions. Understanding how these factors interplay allows for developing targeted strategies to mitigate their negative effects. Longitudinal epidemiological studies can help establish causal relationships rather than mere associations (Kundi, 2006). Although our study is cross-sectional, it sets the groundwork for future longitudinal studies that can track changes in bone density over time in relation to smoking behavior. Furthermore, findings from epidemiological studies tend to be more generalizable due to the larger and more diverse populations studied. This enhances the applicability of the results to broader populations, making the conclusions more relevant for public health policies. The data derived from such studies can inform public health policies and smoking cessation programs. By demonstrating the significant negative impact of smoking on bone health, these studies can support legislative measures aimed at reducing smoking prevalence. In conclusion, while the adverse effects of smoking on bone density are well-known, epidemiological research provides a comprehensive understanding of the scale and nuances of this issue. It underscores the importance of preventive measures and supports the development of targeted interventions to improve bone health outcomes in the population.
Our study supports earlier preliminary reports that smoking, particularly prolonged tobacco exposure, results in decreased bone mineralization in various ROIs compared to the never-smoking population. Physical activity can effectively mitigate the development of osteopenia and reduce the heightened risk of osteoporosis in smokers. It is essential to educate young European adults about the adverse effects of smoking, not only on the respiratory system, which is widely recognized, but also on the increased risk of osteoporosis.
The raw data supporting the conclusions of this article will be made available by the authors, without undue reservation.
The studies involving humans were approved by the Bioethics Committee of the National Institute of Public Health, National Institute of Hygiene in Warsaw (protocol number 1/2021). The studies were conducted in accordance with the local legislation and institutional requirements. The participants provided their written informed consent to participate in this study.
AK: conceptualization, data curation, formal analysis, investigation, methodology, project administration, resources, validation, visualization, writing–original draft, and writing–review and editing. MC: formal analysis, validation, writing–original draft, and writing–review and editing. GK: visualization, writing–original draft, and writing–review and editing. BU: funding acquisition, project administration, supervision, writing–original draft, and writing–review and editing.
The author(s) declare that financial support was received for the research, authorship, and/or publication of this article. This research was financed by the Ministry of Science and Higher Education in 2020/2022 as part of the Scientific School of the University of Physical Education in Warsaw: SN No. 5 “Biomedical determinants of physical fitness and sports training in adult population” and the statutory grant of the Wrocław Medical University (SUBZ.E250.24.042).
The authors declare that the research was conducted in the absence of any commercial or financial relationships that could be construed as a potential conflict of interest.
All claims expressed in this article are solely those of the authors and do not necessarily represent those of their affiliated organizations, or those of the publisher, the editors, and the reviewers. Any product that may be evaluated in this article, or claim that may be made by its manufacturer, is not guaranteed or endorsed by the publisher.
AS, active smoking; BMC, bone mineral content; BMD, bone mineral density; BMI, body mass index; d effect, sizes calculated using Cohen’s formula; DXA, dual-energy X-ray absorptiometry; EUPASS, European Physical Activity Surveillance System; EUROHIS, European Health Interview Survey; F, Ronald A. Fisher’s test; FFM, fat-free mass; FM, fat mass; GATS, Global Adult Tobacco Survey questionnaire; IPAQ, International Physical Activity Questionnaire; MET, metabolic equivalent of task; PA, physical activity; p-value, level of statistical significance; R2 adj, the adjusted R-squared values of determination; ROIs, regions of interest; β ± SE, values are regression coefficients ± SE describing the change in BMD.
Abate M., Vanni D., Pantalone A., Salini V. (2013). Cigarette smoking and musculoskeletal disorders. Muscle Ligaments Tendons J. 3, 63–69. doi:10.11138/mltj/2013.3.2.063
Adams M. L., Katz D. L., Shenson D. (2016). A healthy lifestyle composite measure: significance and potential uses. Prev. Med. 84, 41–47. doi:10.1016/j.ypmed.2015.12.005
Al-Bashaireh A. M., Haddad L. G., Weaver M., Chengguo X., Kelly D. L., Yoon S. (2018). The effect of tobacco smoking on bone mass: an overview of pathophysiologic mechanisms. J. Osteoporos. 2, 1206235. doi:10.1155/2018/1206235
Bao W., Sun Y., Zhang T., Zou L., Wu X., Wang D., et al. (2020). Exercise programs for muscle mass, muscle strength and physical performance in older adults with sarcopenia: a systematic review and meta-analysis. Aging Dis. 11 (4), 863–873. doi:10.14336/AD.2019.1012
Beaudart C., Dawson A., Shaw S. C., Harvey N. C., Kanis J. A., Binkley N., et al. IOF-ESCEO Sarcopenia Working Group (2017). Nutrition and physical activity in the prevention and treatment of sarcopenia: systematic review. Osteoporos. Int. 28 (6), 1817–1833. doi:10.1007/s00198-017-3980-9
Callréus M., McGuigan F., Akesson K. (2013). Adverse effects of smoking on peak bone mass may be attenuated by higher body mass index in young female smokers. Calcif. Tissue Int. 93 (6), 517–525. doi:10.1007/s00223-013-9785-8
Compston J. E., McClung M. R., Leslie W. D. (2019). Osteoporosis. Lancet. 393 (10169), 364–376. doi:10.1016/S0140-6736(18)32112-3
Deng K. L., Yang W. Y., Hou J. L., Li H., Feng H., Xiao S. M. (2021). Association between body composition and bone mineral density in children and adolescents: a systematic review and meta-analysis. Int. J. Environ. Res. Public Health 18 (22), 12126. doi:10.3390/ijerph182212126
Hart N. H., Nimphius S., Rantalainen T., Ireland A., Siafarikas A., Newton R. U. (2017). Mechanical basis of bone strength: influence of bone material, bone structure and muscle action. J. Musculoskelet. Neuronal Interact. 17 (3), 114–139.
Hernandez C. J., Beaupré G. S., Marcus R., Carter D. R. (2001). A theoretical analysis of the contributions of remodeling space, mineralization, and bone balance to changes in bone mineral density during alendronate treatment. Bone 29 (6), 511–516. doi:10.1016/s8756-3282(01)00613-5
Hind K., Burrows M. (2007). Weight-bearing exercise and bone mineral accrual in children and adolescents: a review of controlled trials. Bone 40 (1), 14–27. doi:10.1016/j.bone.2006.07.006
Kaleta D., Kozieł A., Miskiewicz P. (2009). Global adult tobacco survey in Poland – the aim and current experiences. Med. P. R. 60 (3), 197–200.
Kanis J. A., Johnell O., Oden A., Johansson H., de Laet C., Eisman J. A., et al. (2005). Smoking and fracture risk: a meta-analysis. Osteoporos. Int. 16, 155–162. doi:10.1007/s00198-004-1640-3
Kemmler W., Shojaa M., Kohl M., von Stengel S. (2020). Effects of different types of exercise on bone mineral density in postmenopausal women: a systematic review and meta-analysis. Calcif. Tissue Int. 107 (5), 409–439. doi:10.1007/s00223-020-00744-w
Kistler-Fischbacher M., Weeks B. K., Beck B. R. (2021). The effect of exercise intensity on bone in postmenopausal women (part 2): a meta-analysis. Bone 143, 115697. doi:10.1016/j.bone.2020.115697
Kohler J. B., Junqueira J. J. M., Silva TCMD, Filho MAGP, Tibério IDFLC, Lopes FDTQS, et al. (2021). Smoking-induced oxidative stress in bone: the effects on bone turnover. J Orthop. & Orthop. Surg 2 (2), 14–23. doi:10.29245/2767-5130/2021/2.1138
Kopiczko A. (2020). Determinants of bone health in adults Polish women: the influence of physical activity, nutrition, sun exposure and biological factors. PLoS One 15 (9), e0238127. doi:10.1371/journal.pone.0238127
Kopiczko A., Adamczyk J. G., Gryko K., Popowczak M. (2021). Bone mineral density in elite masters athletes: the effect of body composition and long-term exercise. Eur. Rev. Aging Phys. Act. 18 (1), 7. [published correction appears in Eur Rev Aging Phys Act. 2021;Jul 3,18(1):13]. doi:10.1186/s11556-021-00262-0
Kris-Etherton P. M., Sapp P. A., Riley T. M., Davis K. M., Hart T., Lawler O. (2022). The dynamic interplay of healthy lifestyle behaviors for cardiovascular health. Curr. Atheroscler. Rep. 24, 969–980. doi:10.1007/s11883-022-01068-w
Kundi M. (2006). Causality and the interpretation of epidemiologic evidence. Environ. Health Perspect. 114 (7), 969–974. doi:10.1289/ehp.8297
Lee P. H., Macfarlane D. J., Lam T. H., Stewart S. M. (2011). Validity of the international physical activity questionnaire short form (IPAQ-SF): a systematic review. Int. J. Behav. Nutr. Phys. Act. 8, 115. doi:10.1186/1479-5868-8-115
Lopez P., Taaffe D. R., Galvão D. A., Newton R. U., Nonemacher E. R., Wendt V. M., et al. (2022). Resistance training effectiveness on body composition and body weight outcomes in individuals with overweight and obesity across the lifespan: a systematic review and meta-analysis. Obes. Rev. 23 (5), e13428. doi:10.1111/obr.13428
Lopuszanska-Dawid M. (2023). Trends in health behavior of polish women in 1986-2021: the importance of socioeconomic status. Int. J. Environ. Res. Public Health 20 (5), 3964. doi:10.3390/ijerph20053964
Lu J., Shin Y., Yen M. S., Sun S. S. (2016). Peak bone mass and patterns of change in total bone mineral density and bone mineral contents from childhood into young adulthood. J. Clin. Densitom. 19 (2), 180–191. doi:10.1016/j.jocd.2014.08.001
Lu Y., Genant H. K., Shepherd J., Zhao S., Mathur A., Fuerst T. P., et al. (2001). Classification of osteoporosis based on bone mineral densities. J. Bone Min. Res. 16 (5), 901–910. doi:10.1359/jbmr.2001.16.5.901
Ng C. A., Scott D., Sim M., Zhu K., Siafarikas A., Hart N. H., et al. (2022). Physical activity estimated by osteogenic potential and energy expenditure has differing associations with bone mass in young adults: the raine study. Arch. Osteoporos. 17 (1), 67. doi:10.1007/s11657-022-01100-1
Nikander R., Sievänen H., Heinonen A., Daly R. M., Uusi-Rasi K., Kannus P. (2010). Targeted exercise against osteoporosis: a systematic review and meta-analysis for optimising bone strength throughout life. BMC Med. 8, 47. doi:10.1186/1741-7015-8-47
O’Bryan S. J., Giuliano C., Woessner M. N., Vogrin S., Smith C., Duque G., et al. (2022). Progressive resistance training for concomitant increases in muscle strength and bone mineral density in older adults: a systematic review and meta-analysis. Sports Med. 52 (8), 1939–1960. doi:10.1007/s40279-022-01675-2
Pinheiro M. B., Oliveira J., Bauman A., Fairhall N., Kwok W., Sherrington C. (2020). Evidence on physical activity and osteoporosis prevention for people aged 65+ years: a systematic review to inform the WHO guidelines on physical activity and sedentary behaviour. Int. J. Behav. Nutr. Phys. Act. 17 (1), 150. doi:10.1186/s12966-020-01040-4
Proia P., Amato A., Drid P., Korovljev D., Vasto S., Baldassano S. (2021). The impact of diet and physical activity on bone health in children and adolescents. Front. Endocrinol. (Lausanne) 13 (12), 704647. doi:10.3389/fendo.2021.704647
Ratajczak A. E., Szymczak-Tomczak A., Rychter A. M., Zawada A., Dobrowolska A., Krela-Kaźmierczak I. (2021). Impact of cigarette smoking on the risk of osteoporosis in inflammatory bowel diseases. J. Clin. Med. 10 (7), 1515. PMID: 33916465; PMCID: PMC8038608. doi:10.3390/jcm10071515
Sanchez-Trigo H., Rittweger J., Sañudo B. (2022). Effects of non-supervised exercise interventions on bone mineral density in adult women: a systematic review and meta-analysis. Osteoporos. Int. 33 (7), 1415–1427. doi:10.1007/s00198-022-06357-3
Shin H., Panton L. B., Dutton G. R., Ilich J. Z. (2011). Relationship of physical performance with body composition and bone mineral density in individuals over 60 Years of age: a systematic review. J. Aging Res. 2011, 191896. doi:10.4061/2011/191896
Tarantino U., Cariati I., Greggi C., Gasbarra E., Belluati A., Ciolli L., et al. (2021). Skeletal system biology and smoke damage: from basic science to medical clinic. Int. J. Mol. Sci. 22, 6629. doi:10.3390/ijms22126629
Ugurlu U., Nayki U., Nayki C., Ulug P., Kulhan M., Yildirim Y. (2016). Assessment of smoking for low bone mineral density in postmenopausal Turkish women. Wien Klin. Wochenschr 128 (3-4), 114–119. doi:10.1007/s00508-015-0867-7
Vestergaard P., Mosekilde L. (2003). Fracture risk associated with smoking: a meta-analysis. J. Intern. Med. 254 (6), 572–583. doi:10.1111/j.1365-2796.2003.01232.x
Ward K. D., Klesges R. C. (2001a). A meta-analysis of the effects of cigarette smoking on bone mineral density. Calcif. Tissue Int. 68 (5), 259–270. doi:10.1007/BF02390832
Ward K. D., Klesges R. C. (2001b). A meta-analysis of the effects of cigarette smoking on bone mineral density. Calcif. Tissue Int. 68 (5), 259–270. doi:10.1007/BF02390832
Watson S. L., Weeks B. K., Weis L. J., Harding A. T., Horan S. A., Beck B. R. (2018). High-intensity resistance and impact training improves bone mineral density and physical function in postmenopausal women with osteopenia and osteoporosis: the LIFTMOR randomized controlled trial. J. Bone Min. Res. 33, 211–220. doi:10.1002/jbmr.3284
Weaver C. M., Gordon C. M., Janz K. F., Kalkwarf H. J., Lappe J. M., Lewis R., et al. (2016). The National Osteoporosis Foundation's position statement on peak bone mass development and lifestyle factors: a systematic review and implementation recommendations. Osteoporos. Int. 27 (4), 1281–1386. doi:10.1007/s00198-015-3440-3
Weng W., Li H., Zhu S. (2022). An overlooked bone metabolic disorder: cigarette smoking-induced osteoporosis. Genes 13, 806. doi:10.3390/genes13050806
Wong P. K. K., Christie J. J., Wark J. D. (2007). The effects of smoking on bone health. Clin. Sci. 113 (5), 233–241. doi:10.1042/CS20060173
Keywords: bone mineral density, various regions of skeleton, Caucasian ethnic group, smoking, physical activity
Citation: Kopiczko A, Czapla M, Kubielas G and Uchmanowicz B (2024) Determinants of bone mineral density in various regions of the skeleton among smokers and non-smokers: the role of physical activity. Front. Physiol. 15:1403102. doi: 10.3389/fphys.2024.1403102
Received: 18 March 2024; Accepted: 29 August 2024;
Published: 19 September 2024.
Edited by:
Maximilian Pangratius de Courten, Victoria University, AustraliaReviewed by:
Zhijun Hu, Shanghai University of Traditional Chinese Medicine, ChinaCopyright © 2024 Kopiczko, Czapla, Kubielas and Uchmanowicz. This is an open-access article distributed under the terms of the Creative Commons Attribution License (CC BY). The use, distribution or reproduction in other forums is permitted, provided the original author(s) and the copyright owner(s) are credited and that the original publication in this journal is cited, in accordance with accepted academic practice. No use, distribution or reproduction is permitted which does not comply with these terms.
*Correspondence: Michał Czapla, bWljaGFsLmN6YXBsYUB1bXcuZWR1LnBs
Disclaimer: All claims expressed in this article are solely those of the authors and do not necessarily represent those of their affiliated organizations, or those of the publisher, the editors and the reviewers. Any product that may be evaluated in this article or claim that may be made by its manufacturer is not guaranteed or endorsed by the publisher.
Research integrity at Frontiers
Learn more about the work of our research integrity team to safeguard the quality of each article we publish.