- 1Graduate School of Sport and Exercise Sciences, Osaka University of Health and Sport Sciences, Kumatori, Osaka, Japan
- 2Department of Health and Sport Sciences, Graduate School of Medicine, Osaka University, Toyonaka, Osaka, Japan
- 3Department of Sports Medical Biomechanics, Graduate School of Medicine, Osaka University, Suita, Osaka, Japan
Introduction: With the widespread use of wearable sensors, various methods to evaluate external physical loads using acceleration signals measured by inertial sensors in sporting activities have been proposed. Acceleration-derived external physical loads have been evaluated as a simple indicator, such as the mean or cumulative values of the target interval. However, such a conventional simplified indicator may not adequately represent the features of the external physical load in sporting activities involving various movement intensities. Therefore, we propose a method to evaluate the external physical load of tennis player based on the histogram of acceleration-derived signal obtained from wearable inertial sensors.
Methods: Twenty-eight matches of 14 male collegiate players and 55 matches of 55 male middle-aged players wore sportswear-type wearable sensors during official tennis matches. The norm of the three-dimensional acceleration signal measured using the wearable sensor was smoothed, and the rest period (less than 0.3 G of at least 5 s) was excluded. Because the histogram of the processed acceleration signal showed a bimodal distribution, for example, high- and low-intensity peaks, a Gaussian mixture model was fitted to the histogram, and the model parameters were obtained to characterize the bimodal distribution of the acceleration signal for each player.
Results: Among the obtained Gaussian mixture model parameters, the linear discrimination analysis revealed that the mean and standard deviation of the high-intensity side acceleration value accurately classified collegiate and middle-aged players with 93% accuracy; however, the conventional method (only the overall mean) showed less accurate classification results (63%).
Conclusion: The mean and standard deviation of the high-intensity side extracted by the Gaussian mixture modeling is found to be the effective parameter representing the external physical load of tennis players. The histogram-based feature extraction of the acceleration-derived signal that exhibit multimodal distribution may provide a novel insight into monitoring external physical load in other sporting activities.
Introduction
Wearable sensor devices allowing objective and real-time monitoring are becoming widespread in competitive sports (Patel et al., 2015; Iqbal et al., 2016; Camomilla et al., 2018; Aroganam et al., 2019). Sports scientists, trainers, coaches, and athletes use wearable devices to evaluate physical loads to improve performance and reduce the risk of injury (Cross et al., 2016; Malone et al., 2017; Esmaeili et al., 2018; Duggan et al., 2021). Physical load monitoring indicators have been classified into internal and external physical loads (Halson, 2014). The external physical load is defined as the work completed, as assessed by mechanical indices, whereas internal physical loads are physiological and psychological stresses imposed by external loads, as assessed by heart-rate-based indices, other bio-signals, and questionnaires (Halson, 2014). Excessive external loads resulting from training activities and competitive matches are the major causes of injury in skeletal muscle systems (Gabbett, 2004; Rogalski et al., 2013). Particularly, the frequency of high-intensity acceleration/deceleration movement is associated with subsequent muscular damage and causes a decline in neuromuscular function (Young et al., 2012; Hulin et al., 2016; Russell et al., 2016; Taylor et al., 2018; Gastin et al., 2019). Therefore, external physical loads have been used as an indicator to prevent overload-related injuries during daily training and competition (Gabbett, 2004; Rogalski et al., 2013).
Position-derived evaluation using the global positioning system (GPS) and local positioning system (LPS) have been used to assess external physical loads in various competitive sports (Coutts and Duffield, 2010; Waldron et al., 2011; Cummins et al., 2013; Johnston et al., 2014; Wellman et al., 2016; Mujika, 2017; Linke et al., 2018). The travel distance, speed, and acceleration of the players were evaluated using time-series changes in the player’s position (Johnston et al., 2018; Clemente et al., 2019). However, GPS-based devices have some limitations: they cannot be used indoors, their validity and reliability may decrease when including short-cutting and jumping movements, and the travel distance and speed have been reported to be underestimated (Duffield et al., 2010; Rawstorn et al., 2014; Vickery et al., 2014; Scott et al., 2016). Although LPS acquires position data with higher accuracy than GPS in both indoor and outdoor environments (Hoppe et al., 2018; Alt et al., 2020), the time-consuming set up and calibration of antennas prior to measurement are practical concerns for the daily use of LPS.
The acceleration-derived evaluation of physical loads mitigates the disadvantage of position-derived evaluation of external physical loads (Cambers et al., 2015; Spangler et al., 2018). Wearable and small inertial sensors incorporating acceleration sensors have been used to evaluate the external physical load in competitive matches and daily practice (Sato et al., 2009; Alexander et al., 2016). The inertial sensor can record three-dimensional acceleration at a high sampling frequency over long period (Sato et al., 2009; Wundersitz et al., 2015b; Cambers et al., 2015; Alexander et al., 2016; Spangler et al., 2018). The norm of acceleration or norm of change in acceleration is a major index of acceleration-derived physical loads and has been used as a valid and reliable index (Boyd et al., 2011; Bredt et al., 2020; Byrkjedal et al., 2022). These acceleration-derived physical loads have been evaluated as simple representatives, such as mean or cumulative values across the target interval (Rowlands et al., 2015; Bowen et al., 2017; Staunton et al., 2017; Gentles et al., 2018; Staunton et al., 2018; Reche-Soto et al., 2019).
If the acceleration-derived physical load can be assumed to be normally distributed, the mean value is appropriate as a representative value. In contrast, the distribution of the acceleration-derived physical load in sports that include exercises of various intensities, ranging from walking to sprinting, remains unclear. The mean value may not adequately represent the features of the acceleration-derived external physical load assuming a simple unimodal normal distribution. Therefore, we aimed to propose a method to evaluate acceleration-derived physical loads based on the histogram of the acceleration signal obtained from wearable inertial sensors.
Materials and methods
Participants
The participants of this study were 14 male collegiate (age: 19.5 ± 1.4 years; height: 168.6 ± 1.4 cm; body mass: 63.2 ± 3.5 kg) and 55 male middle-aged players (age: 54.8 ± 8.7 years; height: 171.1 ± 6.0 cm; body mass: 69.3 ± 9.9 kg) who participated in regional qualifier rounds for national tennis championships in their categories (intercollege championship and master’s championship) (Table1). All participants provided written informed consent, and the study was approved by the institutional review board (19537-2).

TABLE 1. Descriptive statistics of participant’s age, body height, and body mass. Data was presented as mean and standard deviation.
Data collection
Each participant wore a spandex sportswear-type sensing wear (sportswear-type wearable) particularly designed for physical activity measurement (MATOUSVS, Teijin Frontier Sensing Ltd., Osaka, Japan). The sensing unit, comprising an inertia sensor and a data logger (SS-ECGHRAG, Sports Sensing Ltd, Fukuoka, Japan), was securely fixed at the upper back of the sensing wear. Before data measurement, because we confirmed that the size of the sensing wear was properly adjusted to the upper body of each participant, the acceleration data measured with the fixed inertia sensor accurately reflected the participant’s movement. In addition, the feasibility of this sensing wear to record exercise intensity has been validated (Marutani et al., 2022). In total, 28 and 55 singles matches were recorded from collegiate and middle-aged plyers, respectively. The three-dimensional acceleration signals for 22 games of collegiate players were recorded at 1,000 Hz, and the data for 6 games of collegiate players, as well as 55 games of middle-aged players were recorded at 200 Hz. The processed data (Supplementary Material) was not influenced by the difference in sampling frequency.
Proposed method
The recorded three-dimensional acceleration signal was filtered using a second-order Butterworth band-pass filter (0.5–20 Hz) to remove the high-frequency noise and baseline shift owing to the long-time recording, which also attenuated the gravity component from the acceleration signals. The cut-off frequency was determined with reference to previous studies using trunk mounted inertia sensor for evaluating locomotive motion (walking, jogging, and running), team sports, and contact sports (Wundersitz et al., 2015a; Wundersitz et al., 2015b; Wundersitz D. W. T. et al., 2015). After calculating the norm of the filtered acceleration signal (
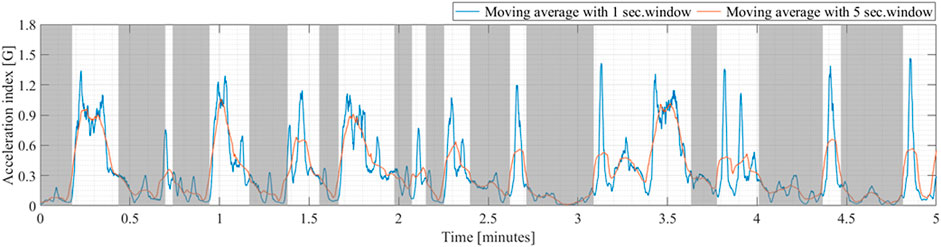
FIGURE 1. Time-series change in acceleration index (blue) and classification of in-play (white) and rest interval (gray) based on the processed acceleration (orange). Data of 5 min was extracted from approximately 1-hour data of a competitive tennis match to visualize in-play and rest intervals.
The histogram of the acceleration index during the in-play showed a meaningful bimodal distribution, which comprised a low-intensity peak that appeared at approximately 0.25 G and a high-intensity peak at approximately 0.9 G in the representative data (Figure 2). To characterize this meaningful distribution of the in-play acceleration index value, a Gaussian mixture model was fitted to the histogram of the acceleration index during the in-play (Figure 2).
where p(x) is the resultant mixture of two probability density functions of the normal distributions
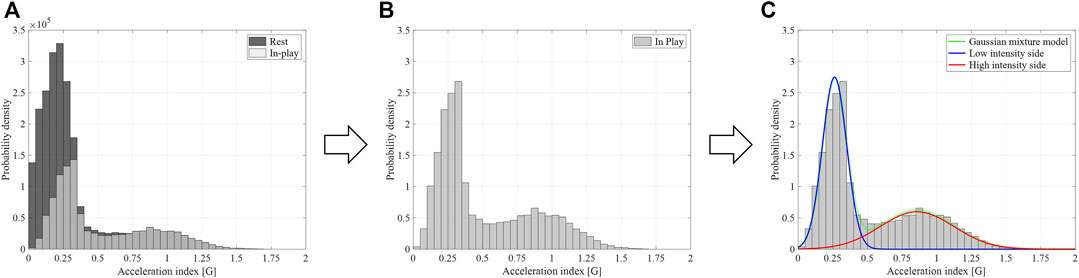
FIGURE 2. Process of Gaussian mixture modeling: (A) Histogram of the acceleration index during the classified in-play and rest intervals, (B) Histogram of the acceleration index during the extracted in-play interval, (C) Fitted bimodal Gaussian mixture model.
Comparison of proposed and conventional methods
The proposed and conventional methods were compared to validate the effectiveness of the proposed method. In previous studies, the norm of the raw acceleration signal was calculated, and the mean value across the target period (
Results
Eighty-three games played by collegiate and middle-aged players revealed that the acceleration index during in-play showed a bimodal distribution, which was approximated using a Gaussian mixture model comprising two normal distribution models (Figure 3). The intra-subject, inter-subject, and inter-group differences in the Gaussian mixture model on the histogram of the acceleration index are shown in Figure 3.
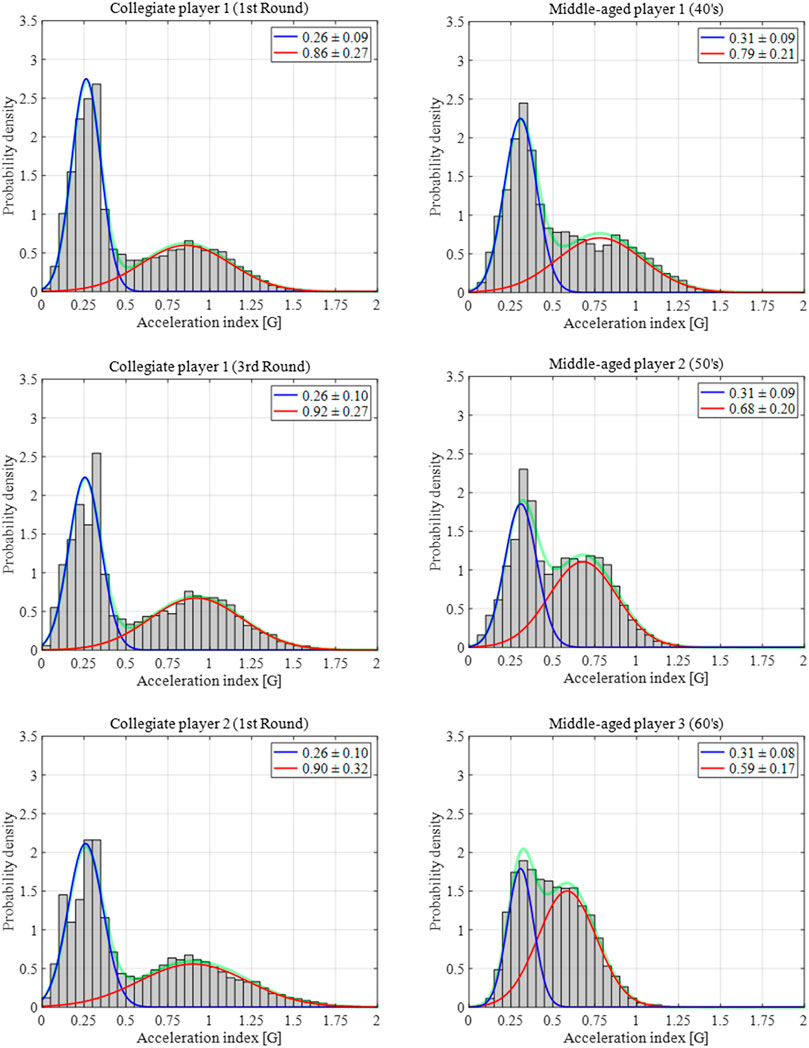
FIGURE 3. Intra- and inter-subject differences, as well as inter-group differences of the Gaussian mixture model on the histogram of the acceleration index. The green line denotes the fitted Gaussian mixture model comprising the high- (red) and low-intensity (blue) sides.
The mean (
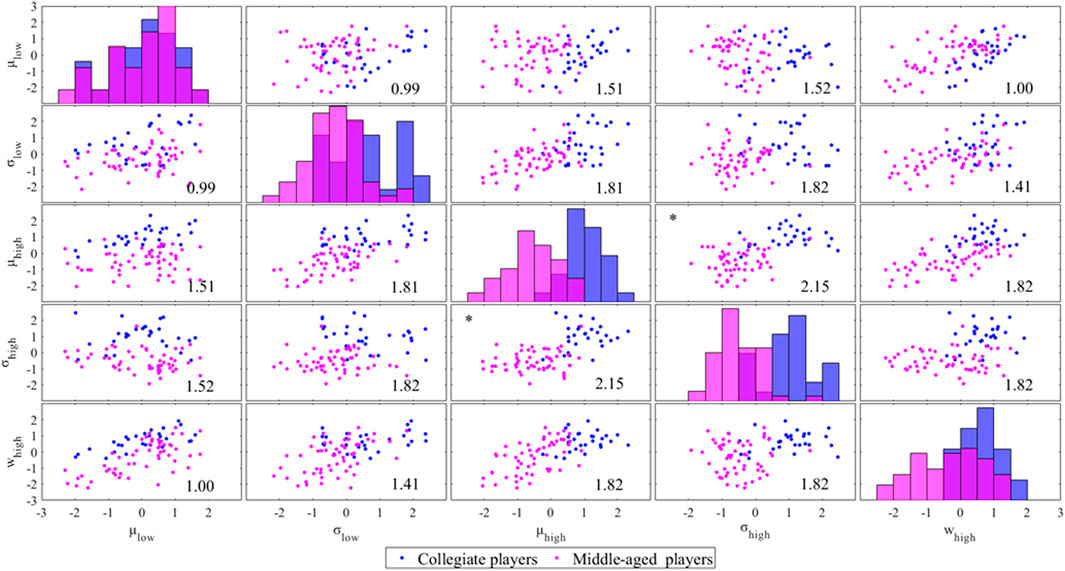
FIGURE 4. Scatter matrix of parameters of the bimodal Gaussian mixture model labeled by two groups. The parameters were standardized by the mean and standard deviation. The number in each panel shows the Euclidean distance between the mean values of two groups. The asterisks (*) shows the combination of parameters with the greatest Euclidean distance.
The Euclidean distance in the plane composed of the mean (
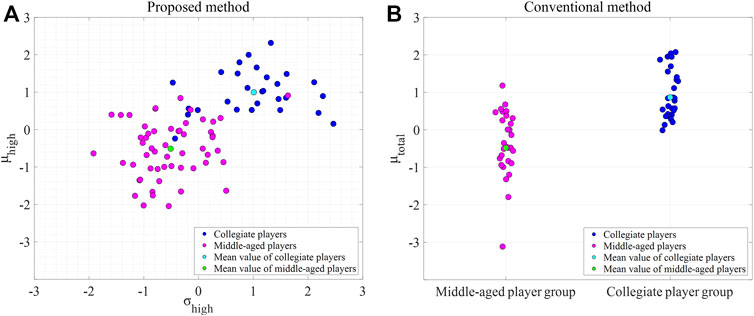
FIGURE 5. Comparison between the proposed (A) and conventional method (B). The proposed method used the mean and standard deviation of the high-intensity side. Each parameter was standardized using the mean and standard deviation.
Discussion
We proposed a method to evaluate the external physical load based on the histogram of the acceleration index recorded by wearable sensors. The histogram of the acceleration index during the competitive tennis match showed a bimodal distribution that could be modeled using a Gaussian mixture model. The mean (
The histogram of the acceleration index during the competitive tennis match showed a bimodal distribution and was adequately approximated using the bimodal Gaussian mixture model (Figure 3). Our previous study conducted an incremental loading test on a treadmill to examine the change in the acceleration index from quiet standing to sprinting (Marutani et al., 2022). Interpreting the results of our previous study in the context of the present result, we found that the peak of distribution of low- and high-intensity sides were approximately equivalent to the walking and jogging, respectively, and the high-intensity side is widely distributed from the jogging to running and sprinting (Marutani et al., 2022). We speculate that the low-intensity activity may represent the waiting for the opponent to hit during a rally, whereas the high-intensity side may represent the ball chasing and swing movements. A bimodal distribution was observed in the histogram of collegiate players’ acceleration index compared with that of middle-aged players. The histogram of
The mean (
This study has two major limitations. First, the proposed method was only tested for competitive tennis matches. However, we believe that the proposed method to evaluate the external physical load based on the distribution of the acceleration index applies to other sporting activities during competition and training. Second, the tested dataset was only recorded from male tennis players because of the structural limitation of sensing wear. However, if a sportswear-type wearable device designed for women can record the acceleration signal using a trunk-mounted inertia sensor, the shape feature of bimodal distribution of female players would show a similar trend to male players. However, its intensity of female players is expected to be different from that of male players.
Conclusion
We proposed a method to evaluate the external physical load based on the histogram of the acceleration index recorded by wearable sensors. The mean and standard deviation of the high-intensity side extracted by the Gaussian mixture modeling are found to be the effective parameter representing the external physical load of tennis players. The histogram-based feature extraction of the acceleration-derived signal that exhibit the multimodal distribution may provide a novel insight into monitoring external physical load in various sporting activities.
Data availability statement
The raw data supporting the conclusion of this article will be made available by the authors, without undue reservation.
Ethics statement
The studies involving human participants were reviewed and approved by the Ethical Review Board Osaka University Hospital. The patients/participants provided their written informed consent to participate in this study.
Author contributions
YM, SK, EM, and KN designed the study. YM, SK, KY, TY, and KN performed data collection. YM, SK, IO conducted analyses. YM, SK, IO, KY, TY, EM, and KN conducted interpretation. YM, SK, IO, EM, and KN wrote the original draft. All authors read and approved the final manuscript.
Funding
This work was partially supported by Sports Research Innovation Project (SRIP) grant sponsored by the Japan Sports Agency and “Innovation Platform for Society 5.0” Program sponsored by Ministry of Education, Culture, Sports, Science and Technology (MEXT).
Conflict of interest
The authors declare that the research was conducted in the absence of any commercial or financial relationships that could be construed as a potential conflict of interest.
Publisher’s note
All claims expressed in this article are solely those of the authors and do not necessarily represent those of their affiliated organizations, or those of the publisher, the editors and the reviewers. Any product that may be evaluated in this article, or claim that may be made by its manufacturer, is not guaranteed or endorsed by the publisher.
Supplementary material
The Supplementary Material for this article can be found online at: https://www.frontiersin.org/articles/10.3389/fphys.2023.1161182/full#supplementary-material
References
Alexander, J. P., Hopkinson, T. L., Wundersitz, D. W. T., Serpell, B. G., Mara, J. K., and Ball, N. B. (2016). Validity of a wearable accelerometer device to measure average acceleration values during high-speed running. J.Strength Cond.Res. 30 (11), 3007–3013. doi:10.1519/JSC.0000000000001396
Alt, P. S., Baumgart, C., Ueberschar, O., Freiwald, J., and Hoppe, M. W. (2020). Validity of a local positioning system during outdoor and indoor conditions for team sports. Sensors 20, 5733. doi:10.3390/s20205733
Aroganam, G., Manivannan, N., and Harrison, D. (2019). Review on wearable technology sensors used in consumer sport applications. Sensors 19, 1983. doi:10.3390/s19091983
Beato, M., De Keijzer, K. L., Carty, B., and Connor, M. (2019). Monitoring fatigue during intermittent exercise with accelerometer-derived metrics. Front. Physiol. 10, 780. doi:10.3389/fphys.2019.00780
Bowen, L., Gross, A. S., Gimpel, M., and Li, F. X. (2017). Accumulated workloads and the acute:chronic workload ratio relate to injury risk in elite youth football players. Br. J. Sports Med. 51, 452–459. doi:10.1136/bjsports-2015-095820
Boyd, L. J., Ball, K., and Aughey, R. J. (2011). The reliability of MinimaxX accelerometers for measuring physical activity in Australian football. Int. J. Sports Physiol. Perform. 6, 311–321. doi:10.1123/ijspp.6.3.311
Bredt, S., Chagas, M. H., Peixoto, G. H., Menzel, H. J., and De Andrade, A. G. P. (2020). Understanding player load: Meanings and limitations. J. Hum. Kinet. 71, 5–9. doi:10.2478/hukin-2019-0072
Byrkjedal, P. T., Luteberget, L. S., Bjornsen, T., Ivarsson, A., and Spencer, M. (2022). Simulated game-based ice hockey match design (scrimmage) elicits greater intensity in external load parameters compared with official matches. Front. Sports Act. Living 4, 822127. doi:10.3389/fspor.2022.822127
Cambers, R., Gabbett, T. J., Cole, M. H., and Beard, A. (2015). The use of wearable microsensors to quantify sport-specific movements. Sports Med. 45, 1065–1081. doi:10.1007/s40279-015-0332-9
Camomilla, V., Bergamini, E., Fantozzi, S., and Vannozzi, G. (2018). Trends supporting the in-field use of wearable inertial sensors for sport performance evaluation: A systematic review. Sensors 18, 873. doi:10.3390/s18030873
Clemente, F. M., Rabbani, A., Conte, D., Castillo, D., Afonso, J., Truman Clark, C. C., et al. (2019). Training/match external load ratios in professional soccer players: A full-season study. Int. J. Environ. Res. Public Health 16 (17), 3057. doi:10.3390/ijerph16173057
Coutts, A. J., and Duffield, R. (2010). Validity and reliability of GPS devices for measuring movement demands of team sports. J. Sci. Med. Sport 13, 133–135. doi:10.1016/j.jsams.2008.09.015
Cross, M. J., Williams, S., Trewartha, G., Kemp, S. P., and Stokes, K. A. (2016). The influence of in-season training loads on injury risk in professional rugby union. Int. J. Sports Physiol. Perform. 11, 350–355. doi:10.1123/ijspp.2015-0187
Cummins, C., Orr, R., O'connor, H., and West, C. (2013). Global positioning systems (GPS) and microtechnology sensors in team sports: A systematic review. Sports Med. 43, 1025–1042. doi:10.1007/s40279-013-0069-2
Duffield, R., Reid, M., Baker, J., and Spratford, W. (2010). Accuracy and reliability of GPS devices for measurement of movement patterns in confined spaces for court-based sports. J. Sci. Med. Sport 13, 523–525. doi:10.1016/j.jsams.2009.07.003
Duggan, J. D., Moody, J. A., Byrne, P. J., Cooper, S. M., and Ryan, L. (2021). Training load monitoring considerations for female gaelic team sports: From theory to practice. Sports 9, 84. doi:10.3390/sports9060084
Esmaeili, A., Stewart, A. M., Hopkins, W. G., Elias, G. P., Lazarus, B. H., Rowell, A. E., et al. (2018). Normal variability of weekly musculoskeletal screening scores and the influence of training load across an Australian football league season. Front. Physiol. 9, 144. doi:10.3389/fphys.2018.00144
Gabbett, T. J. (2004). Reductions in pre-season training loads reduce training injury rates in rugby league players. Br. J. Sports Med. 38 (6), 743–749. doi:10.1136/bjsm.2003.008391
Gastin, P. B., Hunkin, S. L., Fahrner, B., and Robertson, S. (2019). Deceleration, acceleration, and impacts are strong contributors to muscle damage in professional Australian football. J. Strength Cond. Res. 33 (12), 3374–3383. doi:10.1519/JSC.0000000000003023
Gentles, J. A., Coniglio, C. L., Besemer, M. M., Morgan, J. M., and Mahnken, M. T. (2018). The demands of a women's college soccer season. Sports 6 (1), 16. doi:10.3390/sports6010016
Halson, S. L. (2014). Monitoring training load to understand fatigue in athletes. Sports Med. 44 (2), S139–S147. doi:10.1007/s40279-014-0253-z
Hoppe, M. W., Baumgart, C., Polglaze, T., and Freiwald, J. (2018). Validity and reliability of GPS and LPS for measuring distances covered and sprint mechanical properties in team sports. PLoS One 13, e0192708. doi:10.1371/journal.pone.0192708
Hulin, B. T., Gabbett, T. J., Lawson, D. W., Caputi, P., and Sampson, J. A. (2016). The acute:chronic workload ratio predicts injury: High chronic workload may decrease injury risk in elite rugby league players. Br. J. Sports Med. 50, 231–236. doi:10.1136/bjsports-2015-094817
Iqbal, M. H., Aydin, A., Brunckhorst, O., Dasgupta, P., and Ahmed, K. (2016). A review of wearable technology in medicine. J. R. Soc. Med. 109, 372–380. doi:10.1177/0141076816663560
Johnston, J. Richard., Watsford, L. Mark., Kelly, J. Stephen., Pine, J. Matthew., and Spurrs, W. Robert. (2014). Validity and interunit reliability of 10 Hz and 15 Hz GPS units for assessing athlete movement demands. J.Strength Cond.Res. 28 (6), 1649–1655. doi:10.1519/JSC.0000000000000323
Johnston, R. D., Murray, N. B., and Austin, D. J. (2018). The influence of pre-season training loads on in-season match activities in professional Australian football players. Sci. Med. Footb. 3, 143–149. doi:10.1080/24733938.2018.1501160
Linke, D., Link, D., and Lames, M. (2018). Validation of electronic performance and tracking systems EPTS under field conditions. PLoS One 13, e0199519. doi:10.1371/journal.pone.0199519
Malone, J. J., Lovell, R., Varley, M. C., and Coutts, A. J. (2017). Unpacking the black box: Applications and considerations for using GPS devices in sport. Int. J. Sports Physiol. Perform. 12, S218–S226. doi:10.1123/ijspp.2016-0236
Marutani, Y., Konda, S., Ogasawara, I., Yamasaki, K., Yokoyama, T., Maeshima, E., et al. (2022). An experimental feasibility study evaluating the adequacy of a sportswear-type wearable for recording exercise intensity. Sensors 22, 2577. doi:10.3390/s22072577
Mujika, I. (2017). Quantification of training and competition loads in endurance sports: Methods and applications. Int. J. Sports Physiol. Perform. 12, S29–S217. doi:10.1123/ijspp.2016-0403
Patel, M. S., Asch, D. A., and Volpp, K. G. (2015). Wearable devices as facilitators, not drivers, of health behavior change. JAMA 313, 459–460. doi:10.1001/jama.2014.14781
Rawstorn, J. C., Maddison, R., Ali, A., Foskett, A., and Gant, N. (2014). Rapid directional change degrades GPS distance measurement validity during intermittent intensity running. PLoS One 9 (4), e93693. doi:10.1371/journal.pone.0093693
Reche-Soto, P., Cardona-Nieto, D., Diaz-Suarez, A., Bastida-Castillo, A., Gomez-Carmona, C., Garcia-Rubio, J., et al. (2019). Player load and metabolic power dynamics as load quantifiers in soccer. J. Hum. Kinet. 69, 259–269. doi:10.2478/hukin-2018-0072
Reid, M., and Duffield, R. (2014). The development of fatigue during match-play tennis. Br. J. Sports Med. 48 (1), i7–i11. doi:10.1136/bjsports-2013-093196
Rogalski, B., Dawson, B., Heasman, J., and Gabbett, T. J. (2013). Training and game loads and injury risk in elite Australian footballers. J. Sci. Med. Sport 16 (6), 499–503. doi:10.1016/j.jsams.2012.12.004
Rowlands, A. V., Fraysse, F., Catt, M., Stiles, V. H., Stanley, R. M., Eston, R. G., et al. (2015). Comparability of measured acceleration from accelerometry-based activity monitors. Med. Sci. Sports Exerc 47, 201–210. doi:10.1249/MSS.0000000000000394
Russell, M., Sparkes, W., Northeast, J., Cook, C. J., Bracken, R. M., and Kilduff, L. P. (2016). Relationships between match activities and peak power output and Creatine Kinase responses to professional reserve team soccer match-play. Hum. Mov. Sci. 45, 96–101. doi:10.1016/j.humov.2015.11.011
Sato, K., Smith, S. L., and Sands, W. A. (2009). Validation of an accelerometer for measuring sport performance. J.Strength Cond.Res. 23 (1), 341–347. doi:10.1519/JSC.0b013e3181876a01
Scott, T. U. macfarlane., Scott, J. Tannath., and Kelly, G. Vincent. (2016). The validity and reliability of global positioning systems in team sport: A brief review. J.Strength Cond.Res. 30 (5), 1470–1490. doi:10.1519/JSC.0000000000001221
Spangler, R., Rantalainen, T., Gastin, P. B., and Wundersitz, D. (2018). Inertial sensors are a valid tool to detect and consistently quantify jumping. Int. J. Sports Med. 39, 802–808. doi:10.1055/s-0044-100793
Staunton, C., Wundersitz, D., Gordon, B., and Kingsley, M. (2018). Accelerometry-derived relative exercise intensities in elite women's basketball. Int. J. Sports Med. 39, 822–827. doi:10.1055/a-0637-9484
Staunton, C., Wundersitz, D., Gordon, B., and Kingsley, M. (2017). Construct validity of accelerometry-derived force to quantify basketball movement patterns. Int. J. Sports Med. 38, 1090–1096. doi:10.1055/s-0043-119224
Taylor, R. J., Sanders, D., Myers, T., Abt, G., Taylor, C. A., and Akubat, I. (2018). The dose-response relationship between training load and aerobic fitness in academy rugby union players. Int. J. Sports Physiol. Perform. 13, 163–169. doi:10.1123/ijspp.2017-0121
Truppa, L., Guaitolini, M., Garofalo, P., Castagna, C., and Mannini, A. (2020). Assessment of biomechanical response to fatigue through wearable sensors in semi-professional football referees. Sensors 21, 66. doi:10.3390/s21010066
Vickery, W. M., Dascombe, B. J., Baker, J. D., Higham, D. G., Spratford, W. A., and Duffield, R. (2014). Accuracy and reliability of GPS devices for measurement of sports-specific movement patterns related to cricket, tennis, and field-based team sports. J.Strength Cond.Res. 28 (6), 1697–1705. doi:10.1519/JSC.0000000000000285
Waldron, M., Worsfold, P., Twist, C., and Lamb, K. (2011). Concurrent validity and test-retest reliability of a global positioning system (GPS) and timing gates to assess sprint performance variables. J. Sports Sci. 29, 1613–1619. doi:10.1080/02640414.2011.608703
Ward, P. A., Ramsden, S., Coutts, A. J., Hulton, A. T., and Drust, B. (2016). Positional differences in running and nonrunning activities during elite American football training. J.Strength Cond.Res. 32 (7), 2072–2084. doi:10.1519/JSC.0000000000002294
Wellman, A. D., Coad, S. C., Goulet, G. C., and Mclellan, C. P. (2016). Quantification of competitive game demands of NCAA division I college football players using global positioning systems. J. Strength Cond. Res. 30, 11–19. doi:10.1519/JSC.0000000000001206
Wundersitz, D. W., Gastin, P. B., Robertson, S., Davey, P. C., and Netto, K. J. (2015a). Validation of a trunk-mounted accelerometer to measure peak impacts during team sport movements. Int. J. Sports Med. 36, 742–746. doi:10.1055/s-0035-1547265
Wundersitz, D. W., Gastin, P. B., Robertson, S. J., and Netto, K. J. (2015b). Validity of a trunk-mounted accelerometer to measure physical collisions in contact sports. Int. J. Sports Physiol. Perform. 10, 681–686. doi:10.1123/ijspp.2014-0381
Wundersitz, D. W. T., Gastin, P. B., Richter, C., Robertson, S. J., and Netto, K. J. (2015c). Validity of a trunk-mounted accelerometer to assess peak accelerations during walking, jogging and running. Eur. J. Sport Sci. 15, 382–390. doi:10.1080/17461391.2014.955131
Keywords: wearable sensor device, exercise intensity, accelerometry, histogram, multimodal distribution
Citation: Marutani Y, Konda S, Ogasawara I, Yamasaki K, Yokoyama T, Maeshima E and Nakata K (2023) Gaussian mixture modeling of acceleration-derived signal for monitoring external physical load of tennis player. Front. Physiol. 14:1161182. doi: 10.3389/fphys.2023.1161182
Received: 08 February 2023; Accepted: 13 March 2023;
Published: 24 March 2023.
Edited by:
Dale Wilson Chapman, Curtin University, AustraliaReviewed by:
Kevin Netto, Curtin University, AustraliaGrant Malcolm Duthie, Australian Catholic University, Australia
Copyright © 2023 Marutani, Konda, Ogasawara, Yamasaki, Yokoyama, Maeshima and Nakata. This is an open-access article distributed under the terms of the Creative Commons Attribution License (CC BY). The use, distribution or reproduction in other forums is permitted, provided the original author(s) and the copyright owner(s) are credited and that the original publication in this journal is cited, in accordance with accepted academic practice. No use, distribution or reproduction is permitted which does not comply with these terms.
*Correspondence: Shoji Konda, skonda.med@osaka-u.ac.jp