- 1First Clinical Medical College, Soochow University, Suzhou, China
- 2Department of Radiology, School of Medicine, Tongren Hospital, Shanghai Jiao Tong University, Shanghai, China
- 3Department of Radiology, The First Affiliated Hospital of Soochow University, Suzhou, China
Objective: No new U-net (nnU-Net) is a newly-developed deep learning neural network, whose advantages in medical image segmentation have been noticed recently. This study aimed to investigate the value of the nnU-Net-based model for computed tomography angiography (CTA) imaging in assisting the evaluation of carotid artery stenosis (CAS) and atherosclerotic plaque.
Methods: This study retrospectively enrolled 93 CAS-suspected patients who underwent head and neck CTA examination, then randomly divided them into the training set (N = 70) and the validation set (N = 23) in a 3:1 ratio. The radiologist-marked images in the training set were used for the development of the nnU-Net model, which was subsequently tested in the validation set.
Results: In the training set, the nnU-Net had already displayed a good performance for CAS diagnosis and atherosclerotic plaque segmentation. Then, its utility was further confirmed in the validation set: the Dice similarity coefficient value of the nnU-Net model in segmenting background, blood vessels, calcification plaques, and dark spots reached 0.975, 0.974 0.795, and 0.498, accordingly. Besides, the nnU-Net model displayed a good consistency with physicians in assessing CAS (Kappa = 0.893), stenosis degree (Kappa = 0.930), the number of calcification plaque (Kappa = 0.922), non-calcification (Kappa = 0.768) and mixed plaque (Kappa = 0.793), as well as the max thickness of calcification plaque (intraclass correlation coefficient = 0.972). Additionally, the evaluation time of the nnU-Net model was shortened compared with the physicians (27.3 ± 4.4 s vs. 296.8 ± 81.1 s, p < 0.001).
Conclusion: The automatic segmentation model based on nnU-Net shows good accuracy, reliability, and efficiency in assisting CTA to evaluate CAS and carotid atherosclerotic plaques.
Introduction
Carotid artery stenosis (CAS), a common vascular disease, is one of the main pathological causes of ischemic stroke, which could be identified in nearly 20% of stroke patients; meanwhile, the prevalence of asymptomatic CAS ranges from 0.3% to 5.7% (Cheng et al., 2019; Carreira et al., 2020; Arasu et al., 2021; Heck and Jost, 2021). Concerning the initiation of CAS, the atherosclerotic plaque is nonignorable, which leads to narrowness or occlusion in the main neck arteries and further impacts the blood supply (Bos et al., 2021; Larson et al., 2021; Qaja et al., 2022). Due to the unique structure of the carotid artery (the Willis circle), a large number of patients are evaluated as asymptomatic CAS, who are easy to miss the best treatment timing and suffer from poor prognosis; hence, the accurate and timely diagnosis is essential (Carreira et al., 2020; US Preventive Services Task Force et al., 2021). At present, digital subtraction angiography (DSA) is considered to be the golden standard of CAS diagnosis; as it is an invasive surgery with a relatively high-dose contrast agent, many patients tend to choose computed tomography angiography (CTA), whose trauma and radiation quantity are milder (Dos Santos et al., 2016; Dohring et al., 2021). However, the CTA images still need clinicians to provide artificial judgment, which would take a relatively long time and the accuracy highly depends on the clinical experiences of physicians (Ghekiere et al., 2017). Therefore, some assistant diagnostic means [such as artificial intelligence (AI)] are generated to improve the efficiency and accuracy of CAS diagnosis, so as to promote the application of CTA in clinical practice.
U-net, a semantic segmentation algorithm of fully convolutional networks, is widely used in various imaging automatic segmentation and auxiliary diagnoses of many vascular diseases (Meshram et al., 2020; Zhou et al., 2021; Yin et al., 2022). For instance, a previous study develops a carotid segmentation model based on U-net to segment carotid bifurcation in CTA images (Zhou et al., 2021). No new U-net (nnU-Net), based on U-net technology, is a newly-developed deep learning neural network with a self-adapting function, which could handle various image properties and target structures (Isensee et al., 2021; Zhang et al., 2021b). Recently, the advantages of nnU-Net in medical image segmentation have been noticed in some studies (Heidenreich et al., 2021; Savjani, 2021). One study establishes a self-configuring nnU-Net model for automatic infarct segmentation in myocardial infarction patients; meanwhile, the infarct zone volumes obtained from this model and manual show good consistency (Heidenreich et al., 2021). Nevertheless, the application of nnU-Net in assisting CTA to evaluate CAS and to segment atherosclerotic plaque is rare.
Thus, this study established a nnU-Net-based automatic-segmentation model for CTA imaging in the training set and further verified its accuracy in the validation set, aiming to investigate its performance in assisting the evaluation of CAS and atherosclerotic plaque.
Materials and methods
Participants
This study retrospectively enrolled 93 patients with suspected CAS who underwent head and neck CTA examination between February 2021 and November 2021. The inclusion criteria were: 1) patients with suspected CAS, and had typical symptoms including transient monocular amaurosis or visual field defect, aphasia, limb numbness, clumsiness of motion; 2) had two or more risk factors for stroke, including hypertension, atrial fibrillation, diabetes, dyslipidemia, smoking, overweight, and history of transient ischemic attack; 3) underwent head and neck CTA examination; 4) had CAS and plaque confirmed by CTA. The exclusion criteria were: 1) history of interventional or surgical treatment, such as carotid stenting or carotid endarterectomy; 2) had cervical hemangioma or carotid artery vascular malformation; 3) CTA images were unclear or incomplete and insufficient for subsequent image processing; 4) missing clinical data. All 93 patients with suspected CAS were randomly divided into a training set (N = 70) and a validation set (N = 23) in a 3:1 ratio. The Institutional Review Board of The First Affiliated Hospital of Soochow University approved this study. The written informed consents were obtained from all participants.
Clinical data collection
Clinical data of all patients were obtained through Electronic Health Record System, including age, gender, BMI (body mass index), smoke, hypertension, hyperlipidemia, diabetes, CKD (chronic kidney disease), CVD (cardiovascular disease), education level, marriage status, and place of residence.
Computed tomography angiography image acquisition
The CTA examination was performed using a Toshiba 320-row-detector spiral computed tomography (CT) scanner (Aquilion ONE, Toshiba Medical Systems, Tokyo, Japan). The patients were placed in a supine position during the examination and were scanned at the end of the expiration when holding their breath. The parameters of scanning were as follows: reference 186 mAs, tube voltage 100 kV, pitch 0.8, rotation time 0.5 s, scanning time 3–4 s, delay time 2 s, collimator width 160 mm × 0.5 mm. Scan range was from the aortic arch to the level of both eyes, and the localization line was at the ascending aortic arch and the skull base, respectively. After completion of the conventional neck scan, the iodinated contrast media, iopamidol injection (Isovue-M®, United States) was used for the enhanced scan. A 30–45 ml bolus of non-ionic contrast material was injected by a dual barrel high-pressure injector at a flow rate of 4.5–5.0 ml/s with the threshold set at 180 HU in the descending aorta. Then, the scan raw data was transferred to the Vitrea (Vital Images, Minnetonka, MN, United States) software for post-processing. After the bone images were subtracted, the carotid vessels were reconstructed by image post-processing such as raw map, volumetric imaging, multi-planar reconstruction, and maximum density projection.
Physicians’ annotations
The luminal stenosis location, plaque location, and plaque type (calcified plaque, non-calcified plaque, and mixed plaque) on CTA images of all patients were marked by two senior professional radiologists. Besides, the number of calcified plaques, non-calcified plaques, mixed plaques, the maximum calcified plaque thickness, and overall evaluation time was recorded. The plaques were segmented and labeled by the ITK-Snap tool (available at http://www.itksnap.org/pmwiki/pmwiki.php?n=Downloads.SNAP3). In case of disagreement, the two doctors discussed and decided on the best results.
Carotid artery stenosis and its severity evaluation
The percentage of stenosis was calculated via the diameter measurements and the North American Symptomatic Carotid Endarterectomy Trial (NASCET) formula (North American Symptomatic Carotid Endarterectomy Trial Collaborators et al., 1991). Then the stenosis degree (if multiple stenoses were present, the most severe one was applied) was classified according to the Society of Cardiovascular Computed Tomography (SCCT) classification (American College of Cardiology Foundation et al., 2012). In detail, stenosis degree was classified as follows: 0%, no stenosis; 1%–49%: mild stenosis; 50%–69%: moderate stenosis; 70%–99%: severe stenosis; and 100%: blocking. Meanwhile, the occurrence of CAS was defined as “≥1% stenosis.”
No new U-net model development
After enhancing and normalizing the images, the annotated images were thresholded for binarization. Combined with threshold segmentation and region growing algorithm, the common carotid artery to the extracranial segment of the internal carotid artery was segmented. The marked images in the training set were used for the development of a nnU-Net model, introducing residual convolution and null convolution modules. After forming the algorithm, the model was tested in the validation set. The principle of the nnU-Net deep neural network framework referred to the previous studies (Isensee et al., 2021). In the nnU-Net network, the size of the input and output 4D image block was 2 × 48 × 160 × 160. The encoding and decoding stages both contained five layers. In the coding phase, the first layer contained two 3 × 3 × 3 convolutions and two Leaky Linear Units (ReLU), and other layers contained a 3 × 3 × 3 convolution and a Leaky ReLU in turn. Except for the last convolution layer, each layer was followed by a 3 × 3 × 3 down sampling convolution with strides of 2 in each dimension. The channel number of the first convolution layer was set to 30, and the following convolution layer doubled the number of channels in turn. In the decoding phase, the five deconvolution layers with a size of 3 × 3 × 3 and stride size of 2 were deployed corresponding to the five down-sampling convolution layers. Every up-convolution layer was followed by two 3 × 3 × 3 convolutions. Each convolution layer except for the last layer halved the number of featuremaps channels in turn. The last layer reduced the number of output channels to the number of labels using a 1 × 1 × 1 convolution kernel. Five shortcut connections from layers of equal resolution in the analysis path transmitted the essential high-resolution features to the synthesis path. In the training step, the batch size was set to 4. Adam optimizer with an initial learning rate of 3e-4 was used to optimize network weight. The network was trained 21,900 times in 300 epochs. The detailed framework of the nnU-Net model was presented (Supplementary Figure S1). The coronal and axial imaging presentations of plaque segmentation assessed by the nnU-Net model and physicians in the training set (Supplementary Figures S2A–F) and the validation set (Supplementary Figures S3A–F) were displayed. The green area represented calcification plaques; the red area represented vascular lumens; the blue area represented dark spots.
The performance of the nnU-Net model in segmenting carotid plaque calcification was evaluated via area accuracy. The manually marked carotid plaque calcification areas in the dataset were used as the standard and compared with the results of the nnU-Net model automatic segmentation. Then the Dice similarity coefficient (DSC) was assessed as an evaluation index. DSC was a common measurement for assessing the similarity between manual and automatic segmentation; DSC ≥ 0.8 was considered a high similarity (Dionisio et al., 2021). The specific formula was as follows:
where SEG = segmented picture; GT = ground truth. In this study, SEG represented the calcified area obtained by nnU-Net model automatic segmentation and GT represented the calcified area obtained by manual segmentation.
Statistical analysis
The statistical analyses were performed via SPSS v27.1 (IBM Corp., United States). The graphs were mapped by R V.4.0.5 (ggplot2 package, available at www.r-project.org) and GraphPad Prism v8.01 (GraphPad Software Inc., United States). The comparison of variables between the training set and validation set was evaluated through Student’s t-test, Chi-square test, Fisher exact test or Mann-Whitney U test. The consistency between the nnU-Net model and physicians in evaluating CAS was assessed through the Kappa coefficient test (Kappa ≥ 0.7 was considered highly consistent, 0.4 ≤ Kappa < 0.7 was considered moderately consistent, Kappa < 0.4 was considered weakly consistent). The consistency between the nnU-Net model and physicians in measured max thickness of calcification plaque was evaluated through the intraclass correlation coefficient (ICC) test. A p value < 0.05 indicated statistical significance.
Results
Clinical characteristics
The whole process of this study was presented (Figure 1). A total of 93 patients with a mean age of 62.1 ± 8.3 years were randomly divided into the training set (N = 70) and validation set (N = 23) in a 3:1 ratio, whose mean age was 61.6 ± 8.5 years and 63.7 ± 7.8 years, respectively (Table 1). Concerning gender, the total patients consisted of 37 (39.8%) females and 56 (60.2%) males; besides, there were 29 (41.4%) females and 41 (58.6%) males in the training set, 8 (34.8%) females and 15 (65.2%) males in the validation set. Notably, all clinical characteristics were of no difference between the training set and validation set, including age, gender, BMI, smoke, hypertension, hyperlipidemia, diabetes, CKD, CVD, education, marriage status, and location (all p > 0.050). The detailed clinical characteristics were listed in Table 1.
The segmentation performance of the no new U-net model
In the training set, the nnU-Net model achieved the DSC value of 0.977, 0.962, 0.791, and 0.489 in segmenting background, blood vessels, calcification plaques, and dark spots. Meanwhile, the respective DSC value of the nnU-Net reached 0.975, 0.974, 0.795, and 0.498 in the validation set (Table 2).
Consistency of carotid artery stenosis diagnosis and stenosis degree evaluation between the no new U-net model and physicians
The nnU-Net model and physicians displayed good consistency in assessing CAS both in the training set (Kappa = 0.860, p < 0.001) and validation set (Kappa = 0.893, p < 0.001) (Table 3). Also, the nnU-Net model exhibited a pleasing consistency with the physicians in evaluating stenosis degree both in the training set (Kappa = 0.874, p < 0.001) and the validation set (Kappa = 0.930, p < 0.001) (Table 4).
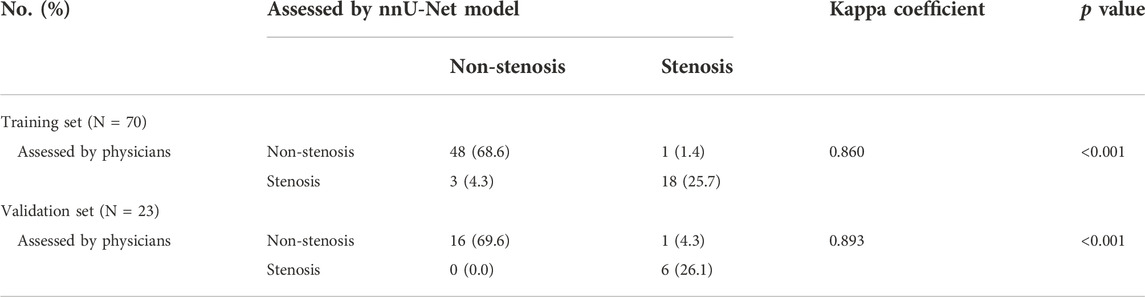
TABLE 3. Consistency between nnU-Net model and physicians in evaluating carotid artery stenosis in training and validation sets.
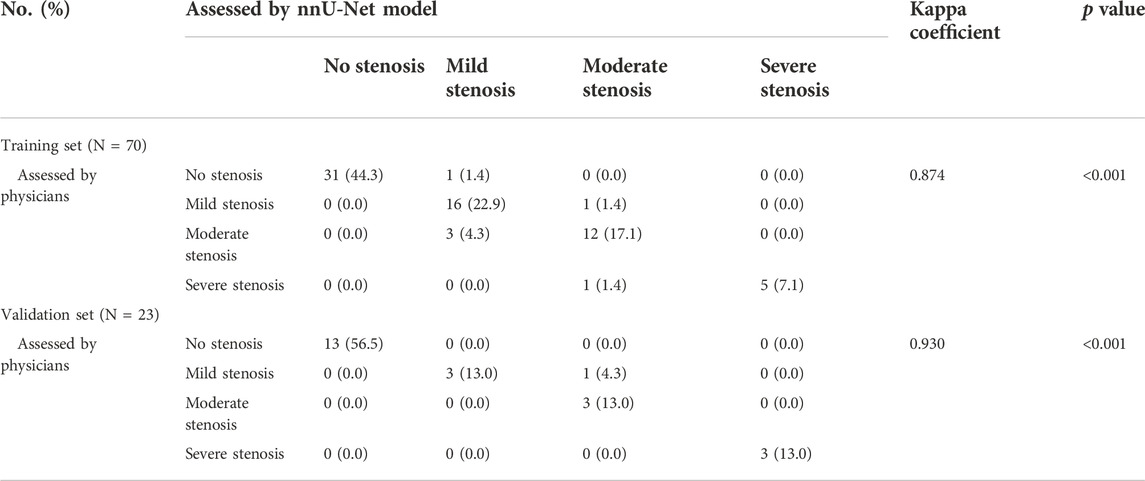
TABLE 4. Consistency between nnU-Net model and physicians in evaluating carotid artery stenosis degree in training and validation sets.
Consistency of atherosclerotic plaque number and max thickness evaluation between the no new U-net model and physicians
In the training set, the nnU-Net model and physicians showed good consistency in evaluating the number of calcification plaque (Kappa = 0.892, p < 0.001) and the number of mixed plaque (Kappa = 0.705, p < 0.001), while they only displayed a moderate consistency in evaluating the number of non-calcification plaque (Kappa = 0.617, p < 0.001) (Figures 2A–C). Besides, in the validation set, the bubble plots showed good consistency between the nnU-Net model and physicians in evaluating the number of calcification plaque (Kappa = 0.922, p < 0.001), the number of non-calcification plaque (Kappa = 0.768, p < 0.001), and the number of mixed plaque (Kappa = 0.793, p < 0.001) (Figures 2D–F). The above data suggested that the nnU-Net model achieved the highest consistency with physicians in evaluating calcification plaque number, followed by mixed plaque number, and the lowest in non-calcification plaque number.
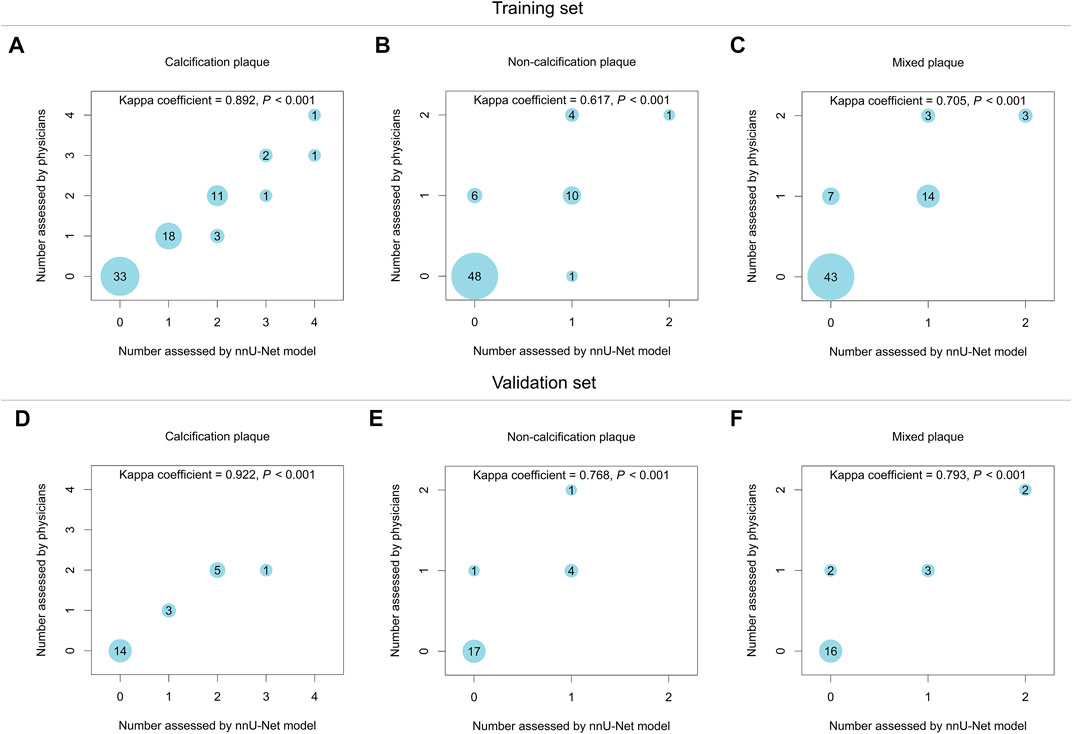
FIGURE 2. The nnU-Net model showed good consistency of atherosclerotic plaque number evaluation with physicians in the training set and validation set. The consistency between the nnU-Net model and physicians in evaluating the number of calcification plaque (A), non-calcification plaque (B), and mixed plaque (C) in the training set. The consistency between the nnU-Net model and physicians in evaluating the number of calcification plaque (D), non-calcification plaque (E), and mixed plaque (F) in the validation set.
Concerning the evaluation of calcification plaque max thickness, the nnU-Net model also achieved good consistency with physicians both in the training set (ICC = 0.959, p < 0.001, Figure 3A) and the validation set (ICC = 0.972, p < 0.001, Figure 3B).
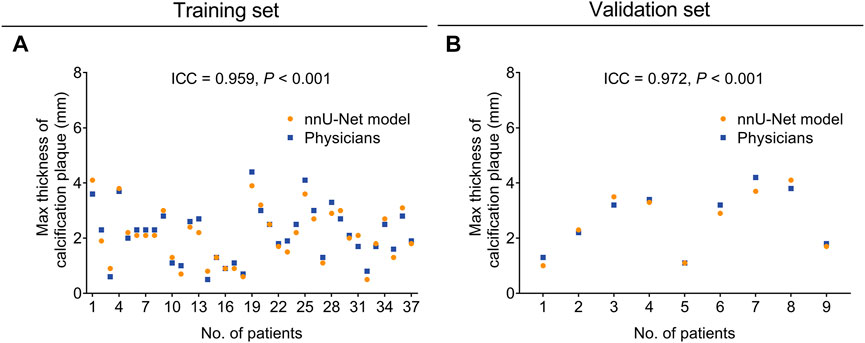
FIGURE 3. The nnU-Net model showed good consistency of calcification plaque max thickness evaluation with physicians in the training set and validation set. The consistency between the nnU-Net model and physicians in evaluating the max thickness of calcification plaque in the training set (A) and the validation set (B).
Comparison of evaluation time between the no new U-net model and physicians
The evaluation time of the nnU-Net model was shortened compared with the physicians both in the training set (30.2 ± 8.4 s vs. 295.0 ± 111.9 s, p < 0.001, Figure 4A) and the validation set (27.3 ± 4.4 s vs. 296.8 ± 81.1 s, p < 0.001, Figure 4B).
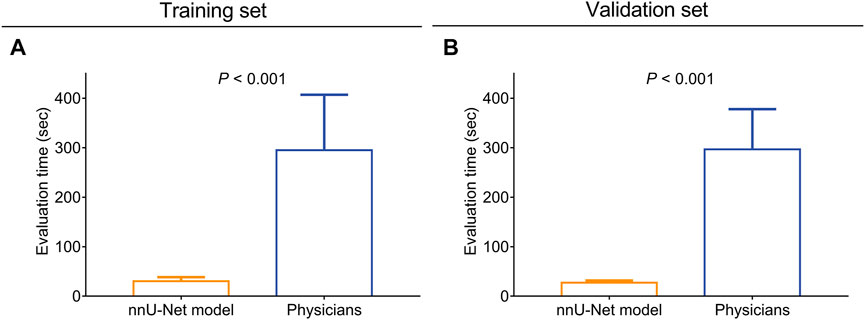
FIGURE 4. The nnU-Net model took less time for CAS and atherosclerotic plaque evaluation compared with physicians. Comparison of the evaluation time between the nnU-Net and physicians in the training set (A) and the validation set (B).
Discussion
U-Net has been widely applied in medical imaging auxiliary diagnoses and vessel segmentation for several cardiovascular and cerebrovascular diseases since its development (Shelhamer et al., 2017; Dong et al., 2021; Zhao et al., 2021; Yin et al., 2022). For instance, a previous study develops a U-Net-based deep learning model to automatically segment coronary arteries in invasive coronary angiography, whose average DSC reaches 0.889, proving its potency in auxiliary coronary artery disease diagnosis (Zhao et al., 2021). However, as a newly-established algorithm based on three U-net architectures, the clinical application of the nnU-Net model in assisting CTA to diagnose CAS has not been studied yet. In this study, the nnU-Net model showed good performance in blood vessel segmentation with a DSC value of 0.977 in the training set and 0.975 in the validation set, which was numerically superior compared to the aforementioned study (Zhao et al., 2021). A probable reason might be that: U-Net had some inevitable shortcomings, including the inability to extract good features, insufficient high-resolution contour information, and the asymmetry between edge-cutting form and feature image (Li et al., 2022). While nnU-Net realized optimization of preprocessing, training, and data post-processing, which avoided ambiguity in contour segmentation (Zhang et al., 2021a). Therefore, the blood-vessel segmenting performance of the nnU-Net model was better than the U-Net model. Additionally, the consistency of CAS diagnosis and stenosis degree evaluation between the nnU-Net model and physicians was also satisfying, which might also contribute to its accurate blood-vessel segmenting performance. Besides, the data set was divided into the training set and validation set in a 3:1 ratio, which could be explained by that: the data set of this study was relatively small, and the majority of data should be applied for model establishment to reduce the risk of overfitting.
Apart from the blood vessels, the exact segmentation of atherosclerotic plaque is also essential for CAS diagnosis (Kondakov and Lelyuk, 2021; Kowara and Cudnoch-Jedrzejewska, 2021). However, the calcified area frequently exists shadow artifacts and “blooming artifacts,” which would interfere with the diagnosing accuracy of AI-assisted diagnosis under CTA and cause detection errors (Jinnouchi et al., 2020). While, in the present study, the DSC value of the nnU-Net model in segmenting calcification plaques reached 0.791 (in the training set) and 0.795 (in the validation set); meanwhile, the consistency between the nnU-Net model with physicians was also pleasing in evaluating atherosclerotic plaque numbers and the max thickness of calcification plaque. The results reflected the good atherosclerotic plaque-segmentation performance of the nnU-Net model, which could be explained as follows: the nnU-Net algorithm sharpened the edge of calcification components during the imaging processing, meanwhile, its deep learning methods on the edge features of calcification plaque and other components improved the segmentation accuracy (Li et al., 2021). Unfortunately, the DSC value of the nnU-Net model in segmenting dark spots was only 0.489 and 0.498 in the training set and the validation set, correspondingly, suggesting that the network structure needed further improvement.
Considering that the traditional manual image-marking is time-consuming and inefficient, which greatly occupies the diagnosis time of clinicians, the time-saving advantage of nnU-Net is highlighted in previous studies (Mihelic et al., 2021). For instance, a previous study reports that the nnU-Net model takes approximately 20 s for the whole breast segmentation and nearly 15 s for the fibroglandular tissue segmentation under the dynamic contrast-enhanced magnetic resonance images (Huo et al., 2021). Similarly, the current study also noticed that the evaluation time of the nnU-Net model was shortened compared with the physicians in both the training set (30.2 ± 8.4 s vs. 295.0 ± 111.9 s) and the validation set (27.3 ± 4.4 s vs. 296.8 ± 81.1 s). A possible explanation might be as follows: nnU-Net was an automated configuration, covering the entire segmentation without any manual decision, meanwhile, it contained simple execution rules which realized the simplified compute resources (Isensee et al., 2021; Tan et al., 2021). Consequently, owing to its flexible and reliable strategy, nnU-Net exhibited its convenience in CAS assessment and atherosclerotic plaque segmentation. Additionally, the evaluation time represented the mean value of 300 epochs, whose difference between the validation set and training set lacked statistical significance; hence, it could not be concluded that the feasibility and efficiency were improved after the training set.
Some limitations were noticed in this study. Firstly, the small sample size (N = 93) might lead to a relatively weak statistical power. Meanwhile, the small number of patients in the validation set would hinder the presentation of the efficacy of the nnU-Net-based automatic segmentation model. Thus, further studies with a larger data set were necessary. Secondly, this was a retrospective study whose selective bias was hard to avoid. Thirdly, in order to validate the universality of this model, external validation was necessary to conduct in further studies. Fourthly, this study enrolled CAS-suspected patients (but not CAS-confirmed or stroke patients) to generally investigate its potential in the cardio/cerebrovascular field; hence, further studies conducted in some specific diseases were warranted.
Collectively, the automatic segmentation model based on nnU-Net shows good accuracy, reliability, and efficiency in assisting CTA to evaluate CAS and carotid atherosclerotic plaques, implying the prospect of deep-learning AI technology in auxiliary imaging diagnosis.
Data availability statement
The original contributions presented in the study are included in the article/Supplementary Material, further inquiries can be directed to the corresponding authors.
Ethics statement
The studies involving human participants were reviewed and approved by The Institutional Review Board of The First Affiliated Hospital of Soochow University. The patients/participants provided their written informed consent to participate in this study.
Author contributions
XW and YG were responsible for the conceptualization, project administration, resources, validation, and visualization. YZ and LC were responsible for the acquisition and analysis of data and methodology. WL was involved in the interpretation of data and reviewing and editing of the manuscript. All authors read and approved the final manuscript.
Conflict of interest
The authors declare that the research was conducted in the absence of any commercial or financial relationships that could be construed as a potential conflict of interest.
Publisher’s note
All claims expressed in this article are solely those of the authors and do not necessarily represent those of their affiliated organizations, or those of the publisher, the editors and the reviewers. Any product that may be evaluated in this article, or claim that may be made by its manufacturer, is not guaranteed or endorsed by the publisher.
Supplementary material
The Supplementary Material for this article can be found online at: https://www.frontiersin.org/articles/10.3389/fphys.2022.1057800/full#supplementary-material
References
American College of Cardiology FoundationAmerican College of RadiologyAmerican Institute of Ultrasound in MedicineAmerican Society of EchocardiographyAmerican Society of NephrologyIntersocietal Commission for the Accreditation of Vascular Laboratorieset al. (2012). ACCF/ACR/AIUM/ASE/ASN/ICAVL/SCAI/SCCT/SIR/SVM/SVS 2012 appropriate use criteria for peripheral vascular ultrasound and physiological testing part I: arterial ultrasound and physiological testing: a report of the American College of Cardiology foundation appropriate use criteria task Force, American College of radiology, American Institute of ultrasound in medicine, American society of echocardiography, American society of nephrology, intersocietal commission for the accreditation of vascular laboratories, society for cardiovascular angiography and interventions, society of cardiovascular computed tomography, society for interventional radiology, society for vascular medicine, and society for vascular surgery. J. Vasc. Surg. 56 (1), e17–e51. doi:10.1016/j.jvs.2012.05.054
Arasu R., Arasu A., Muller J. (2021). Carotid artery stenosis: An approach to its diagnosis and management. Aust. J. Gen. Pract. 50 (11), 821–825. doi:10.31128/AJGP-10-20-5664
Bos D., Arshi B., van den Bouwhuijsen Q. J. A., Ikram M. K., Selwaness M., Vernooij M. W., et al. (2021). Atherosclerotic carotid plaque composition and incident stroke and coronary events. J. Am. Coll. Cardiol. 77 (11), 1426–1435. doi:10.1016/j.jacc.2021.01.038
Carreira M., Duarte-Gamas L., Rocha-Neves J., Andrade J. P., Fernando-Teixeira J. (2020). Management of the carotid artery stenosis in asymptomatic patients. Rev. Port. Cir. Cardiotorac. Vasc. 27 (3), 159–166.
Cheng S. F., Brown M. M., Simister R. J., Richards T. (2019). Contemporary prevalence of carotid stenosis in patients presenting with ischaemic stroke. Br. J. Surg. 106 (7), 872–878. doi:10.1002/bjs.11136
Dionisio F. C. F., Oliveira L. S., Hernandes M. A., Engel E. E., de Azevedo-Marques P. M., Nogueira-Barbosa M. H. (2021). Manual versus semiautomatic segmentation of soft-tissue sarcomas on magnetic resonance imaging: evaluation of similarity and comparison of segmentation times. Radiol. Bras. 54 (3), 155–164. doi:10.1590/0100-3984.2020.0028
Dohring C. L., Geiger J. T., Motyl C. M., Wang M., Stoner M. C., Doyle A. J. (2021). A reappraisal of CT angiography derived duplex ultrasound velocity criteria with a comparison to digital subtraction angiography in patients with carotid artery stenosis. Ann. Vasc. Surg. 76, 185–192. doi:10.1016/j.avsg.2021.05.004
Dong L., Jiang W., Lu W., Jiang J., Zhao Y., Song X., et al. (2021). Automatic segmentation of coronary lumen and external elastic membrane in intravascular ultrasound images using 8-layer U-Net. Biomed. Eng. Online 20 (1), 16. doi:10.1186/s12938-021-00852-0
Dos Santos F. L., Joutsen A., Paci M., Salenius J., Eskola H. (2016). Automatic detection of carotid arteries in computed tomography angiography: a proof of concept protocol. Int. J. Cardiovasc. Imaging 32 (8), 1299–1310. doi:10.1007/s10554-016-0880-6
Ghekiere O., Salgado R., Buls N., Leiner T., Mancini I., Vanhoenacker P., et al. (2017). Image quality in coronary CT angiography: challenges and technical solutions. Br. J. Radiol. 90 (1072), 20160567. doi:10.1259/bjr.20160567
Heck D., Jost A. (2021). Carotid stenosis, stroke, and carotid artery revascularization. Prog. Cardiovasc. Dis. 65, 49–54. doi:10.1016/j.pcad.2021.03.005
Heidenreich J. F., Gassenmaier T., Ankenbrand M. J., Bley T. A., Wech T. (2021). Self-configuring nnU-net pipeline enables fully automatic infarct segmentation in late enhancement MRI after myocardial infarction. Eur. J. Radiol. 141, 109817. doi:10.1016/j.ejrad.2021.109817
Huo L., Hu X., Xiao Q., Gu Y., Chu X., Jiang L. (2021). Segmentation of whole breast and fibroglandular tissue using nnU-Net in dynamic contrast enhanced MR images. Magn. Reson. Imaging 82, 31–41. doi:10.1016/j.mri.2021.06.017
Isensee F., Jaeger P. F., Kohl S. A. A., Petersen J., Maier-Hein K. H. (2021). nnU-Net: a self-configuring method for deep learning-based biomedical image segmentation. Nat. Methods 18 (2), 203–211. doi:10.1038/s41592-020-01008-z
Jinnouchi H., Sato Y., Sakamoto A., Cornelissen A., Mori M., Kawakami R., et al. (2020). Calcium deposition within coronary atherosclerotic lesion: Implications for plaque stability. Atherosclerosis 306, 85–95. doi:10.1016/j.atherosclerosis.2020.05.017
Kondakov A., Lelyuk V. (2021). Clinical molecular imaging for atherosclerotic plaque. J. Imaging 7 (10), 211. doi:10.3390/jimaging7100211
Kowara M., Cudnoch-Jedrzejewska A. (2021). Pathophysiology of atherosclerotic plaque development-contemporary experience and new directions in research. Int. J. Mol. Sci. 22 (7), 3513. doi:10.3390/ijms22073513
Larson A. S., Brinjikji W., Savastano L., Rabinstein A. A., Saba L., Huston J., et al. (2021). Carotid intraplaque hemorrhage and stenosis: At what stage of plaque progression does intraplaque hemorrhage occur, and when is it most likely to Be associated with symptoms? AJNR. Am. J. Neuroradiol. 42 (7), 1285–1290. doi:10.3174/ajnr.A7133
Li Y. C., Shen T. Y., Chen C. C., Chang W. T., Lee P. Y., Huang C. J. (2021). Automatic detection of atherosclerotic plaque and calcification from intravascular ultrasound images by using deep convolutional neural networks. IEEE Trans. Ultrason. Ferroelectr. Freq. Control 68 (5), 1762–1772. doi:10.1109/TUFFC.2021.3052486
Li D., Chu X., Cui Y., Zhao J., Zhang K., Yang X. (2022). Improved U-Net based on contour prediction for efficient segmentation of rectal cancer. Comput. Methods Programs Biomed. 213, 106493. doi:10.1016/j.cmpb.2021.106493
Meshram N. H., Mitchell C. C., Wilbrand S., Dempsey R. J., Varghese T. (2020). Deep learning for carotid plaque segmentation using a dilated U-net architecture. Ultrason. Imaging 42 (4-5), 221–230. doi:10.1177/0161734620951216
Mihelic S. A., Sikora W. A., Hassan A. M., Williamson M. R., Jones T. A., Dunn A. K. (2021). Segmentation-less, automated, vascular vectorization. PLoS Comput. Biol. 17 (10), e1009451. doi:10.1371/journal.pcbi.1009451
North American Symptomatic Carotid Endarterectomy Trial Collaborators Barnett H. J. M., Taylor D. W., Haynes R. B., Sackett D. L., Peerless S. J., et al. (1991). Beneficial effect of carotid endarterectomy in symptomatic patients with high-grade carotid stenosis. N. Engl. J. Med. 325 (7), 445–453. doi:10.1056/NEJM199108153250701
Qaja E., Tadi P., Theetha Kariyanna P. (2022). “Carotid artery stenosis,” in StatPearls (Treasure Island (FL)).
Savjani R. (2021). nnU-Net: Further automating biomedical image autosegmentation. Radiol. Imaging Cancer 3 (1), e209039. doi:10.1148/rycan.2021209039
Shelhamer E., Long J., Darrell T. (2017). Fully convolutional networks for semantic segmentation. IEEE Trans. Pattern Anal. Mach. Intell. 39 (4), 640–651. doi:10.1109/TPAMI.2016.2572683
Tan W., Huang P., Li X., Ren G., Chen Y., Yang J. (2021). Analysis of segmentation of lung parenchyma based on deep learning methods. J. Xray. Sci. Technol. 29 (6), 945–959. doi:10.3233/XST-210956
US Preventive Services Task Force Krist A. H., Davidson K. W., Mangione C. M., Barry M. J., Cabana M., et al. (2021). Screening for asymptomatic carotid artery stenosis: US preventive services task Force recommendation statement. JAMA 325 (5), 476–481. doi:10.1001/jama.2020.26988
Yin X. X., Sun L., Fu Y., Lu R., Zhang Y. (2022). U-Net-Based medical image segmentation. J. Healthc. Eng. 2022, 4189781. doi:10.1155/2022/4189781
Zhang G., Yang Z., Huo B., Chai S., Jiang S. (2021a). Automatic segmentation of organs at risk and tumors in CT images of lung cancer from partially labelled datasets with a semi-supervised conditional nnU-Net. Comput. Methods Programs Biomed. 211, 106419. doi:10.1016/j.cmpb.2021.106419
Zhang G., Yang Z., Huo B., Chai S., Jiang S. (2021b). Multiorgan segmentation from partially labeled datasets with conditional nnU-Net. Comput. Biol. Med. 136, 104658. doi:10.1016/j.compbiomed.2021.104658
Zhao C., Vij A., Malhotra S., Tang J., Tang H., Pienta D., et al. (2021). Automatic extraction and stenosis evaluation of coronary arteries in invasive coronary angiograms. Comput. Biol. Med. 136, 104667. doi:10.1016/j.compbiomed.2021.104667
Keywords: nnU-Net, automatic segmentation, computed tomography angiography, carotid artery stenosis, atherosclerotic plaque
Citation: Zhu Y, Chen L, Lu W, Gong Y and Wang X (2022) The application of the nnU-Net-based automatic segmentation model in assisting carotid artery stenosis and carotid atherosclerotic plaque evaluation. Front. Physiol. 13:1057800. doi: 10.3389/fphys.2022.1057800
Received: 08 October 2022; Accepted: 11 November 2022;
Published: 06 December 2022.
Edited by:
Dalin Tang, Worcester Polytechnic Institute, United StatesReviewed by:
Xiaoya Guo, Nanjing University of Posts and Telecommunications, ChinaSeung Jaeseok, Seoul National University Hospital, South Korea
Huchuan Zhou, Army Medical University, China
Copyright © 2022 Zhu, Chen, Lu, Gong and Wang. This is an open-access article distributed under the terms of the Creative Commons Attribution License (CC BY). The use, distribution or reproduction in other forums is permitted, provided the original author(s) and the copyright owner(s) are credited and that the original publication in this journal is cited, in accordance with accepted academic practice. No use, distribution or reproduction is permitted which does not comply with these terms.
*Correspondence: Yongjun Gong, R1lKMjg1NEBzaHRyaG9zcGl0YWwuY29t; Ximing Wang, d2FuZ3hpbWluZzE5OThAMTYzLmNvbQ==
†These authors have contributed equally to this work