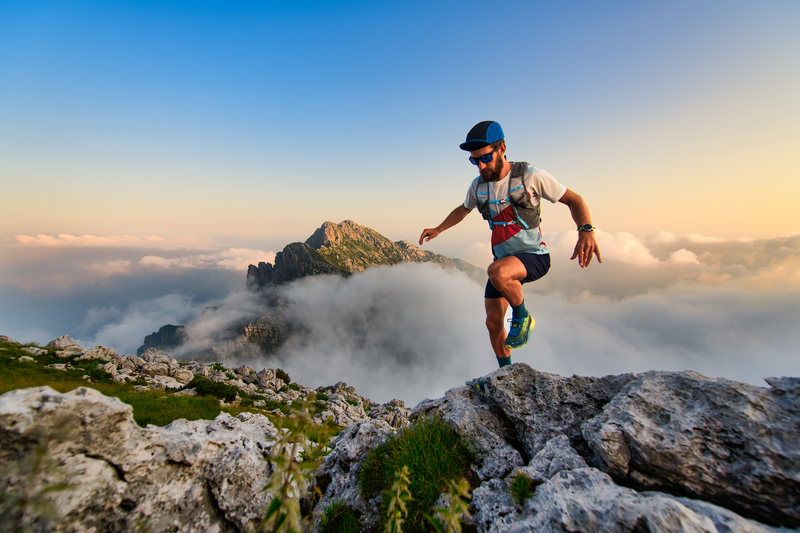
95% of researchers rate our articles as excellent or good
Learn more about the work of our research integrity team to safeguard the quality of each article we publish.
Find out more
ORIGINAL RESEARCH article
Front. Phys. , 25 March 2025
Sec. Statistical and Computational Physics
Volume 13 - 2025 | https://doi.org/10.3389/fphy.2025.1541689
As an important component of transportation facilities, bridges have an increasing demand for inspection and maintenance. However, traditional manual detection methods have many problems in terms of efficiency, accuracy, and safety, making it difficult to meet today’s fast and accurate detection requirements. This article proposes an algorithm for detecting apparent cracks in highway bridges based on MAMBA network and digital image processing technology, this method adopts the detection box form, which can effectively locate and qualitatively detect cracks in concrete bridges accurately. To verify the effectiveness of the model, this paper created a dataset of bridge crack images and used the dataset hyperparameter evolution to obtain default parameters as initial parameters for training. During the training process, we considered adding the CA attention mechanism and the CBAM attention mechanism respectively for the trial process. By comparing the training results, it was found that the model with CA attention mechanism can effectively capture smaller disease features, thus achieving better detection performance. This method has certain advantages in both speed and accuracy, making it more effective in detecting cracks on the bottom of bridges.
Concrete bridges, due to their low cost, wide material sources, strong plasticity, high strength and durability, have been widely used in the construction of high-speed railway bridges [1, 2]. However, the characteristic of easy shrinkage of concrete leads to some cracks in the concrete bridge itself. Some cracks have a short duration and low degree of occurrence and have little impact on bridge safety. There are also some cracks that occur for a long time and have a large length and depth. These cracks affect the appearance and durability of the bridge body, and in severe cases, even threaten the safety of the bridge body, leading to bridge accidents [3, 4]. Therefore, timely detection of cracks in concrete bridges can effectively prevent bridge damage and ensure the safety of high-speed rail transportation. In recent years, the continuous innovation and development of computer, Internet and AI technologies have promoted more and more application scenarios and led the rise of “smart ideas” [5]. Researchers are combining a variety of technologies, including computers, the Internet, IoT and artificial intelligence, to build a comprehensive intelligent solution. In the field of bridge inspection, with the gradual application of mature equipment and technology, traditional bridge inspection methods will be gradually phased out [6–8].
At present, detection techniques based on deep learning have been widely applied in fields such as agriculture, transportation, and aviation. These skills.
Surgery can efficiently replace human eye recognition of image features and has significant advantages such as high efficiency, low cost, and high accuracy [9]. In bridge inspection, these technologies are expected to improve inspection accuracy and efficiency, and reduce inspection costs, thereby better ensuring the safety and reliability of bridge structures. The use of digital imaging technology for apparent disease detection has become a hot topic among scholars at home and abroad, with crack detection being the focus [10]. Merazi Meksen et al. [11] used mathematical morphology methods to extract pixels related to the existence of discontinuities and employed pattern recognition techniques to characterize the discontinuities. Adhikari et al. [12] developed an integrated model based on digital image processing to develop numerical representations of defects and visualize defects through simulated on-site visual inspection. Salman et al. [13] proposed a method based on a linear Gabor filter to analyze the texture in a region to determine whether there is content with a specific frequency in a specific direction, which is very effective for detecting cracks with rich texture. Xu et al. [14] used digital image technology to study the performance evaluation of crack detection methods for reinforced concrete bridges, and analyzed the effects of image grayscale, pixel rate, noise filter, and edge detection considering crack quality. Ahmed et al. [15] used Otsu’s method to detect cracks and multiple filters in images. Wang et al. [16] proposed a local adaptive algorithm for Otsu threshold segmentation and combined it with an improved Sobel operator to remove isolated noise points, thereby extracting crack edge information and improving the accuracy of crack boundary localization. Fujita et al. [17] used a Hessian matrix-based line filter to emphasize the line structures related to cracks. Wang et al. [18] used thresholding to separate cracks from the background. In recent years, with the help of cross-field fusion methods, uncertainty propagation has made some progress in high-dimensional data processing and highly nonlinear scenarios. L. Chen et al. [19] proposed a spatial location feature reduction and arrangement method to classify JFE, which can effectively reduce the feature dimension and consider the integrity and relevance of features. Then, the stacked autoencoder model was constructed and updated by extracting the spatial location features of the sampled JFEs to achieve high-precision classification of the remaining JFEs, and the reliability interval was efficiently calculated according to the classification results. In addition, unlike most traditional uncertainty propagation methods, the method proposed by Q. Chen et al. [20] uses the Gaussian splitting method to divide the input random variables into a Gaussian mixture model. These GMMs have a limited number of components with very little variation. Therefore, the input Gaussian components can be easily propagated to the response and maintain the Gaussian distribution after the nonlinear uncertainty propagation, which can provide an effective method for high-precision nonlinear uncertainty propagation.
The method of detecting apparent defects in concrete bridges based on deep learning can use drones equipped with cameras as carriers to collect bridge images, train deep learning algorithms, and ultimately achieve automated recognition [21]. Compared with traditional methods, this approach can significantly reduce labor costs and is more accurate and safer. The application of this technology can effectively guide bridge maintenance and management work and enhance our ability to ensure bridge safety. In short, the development of this technology will bring more efficient, accurate, and reliable solutions for bridge maintenance in the future.
The occurrence of bridge accidents is often due to a lack of scientific and timely disease detection. According to relevant data, cracks are the most common bridge disease, and most bridge accidents are caused by cracks. Therefore, regular detection of bridge cracks is of utmost importance in bridge inspection. Choosing scientific and effective detection measures for regular inspection of bridges is urgent. At present, there are two traditional detection methods as follows.
Before the emergence of many crack detection instruments, the detection of bridge diseases mainly relied on manual labor. People used the naked eye to search and identify whether bridges had diseases, which had many drawbacks. Firstly, the visual recognition ability is weak, and people rely solely on experience. In many cases, failure to identify diseases in a timely manner can lead to unimaginable consequences. Secondly, the manual detection of diseases has a long cycle, and generally requires scaffolding to be erected under the bridge to observe whether there are cracks at the bottom and sides of the bridge. These preparations require a lot of time. In addition, many roads can only be constructed at night, which poses a safety hazard.
There are currently many methods to replace manual bridge image acquisition and detection, which use inspection vehicles or drones to collect images and detect bridge diseases through image processing, ultrasonic or radiographic scanning. Among them, the detection methods of image processing are the most widely used. The image processing method mainly consists of two steps: capturing images and detecting diseases. Applying this method to detect cracks can achieve the characteristics of fast detection speed and high accuracy, without the need to block road sections during the acquisition process. After the acquisition is completed, appropriate algorithms can be used to detect cracks. However, using image processing algorithms to detect cracks and extract crack features requires manual setting and modification of parameters based on the characteristics of the cracks, which places certain demands on the designer’s experience. Moreover, this method often cannot accurately represent the original data and has low robustness.
The bottom surface of the bridge captured by the camera contains a lot of information, which may include cracks on the bridge deck, traces of pollution on the bridge deck, local raised shadows on the cement on the bridge deck, or other debris. By using digital image processing technology to process images, some interference impurities can be eliminated and converted into image information that is conducive to crack identification. The commonly used digital image processing algorithms include image grayscale correction, image denoising filtering, image binarization, etc [22].
The image of the bridge deck bottom captured by the camera is a color image. Due to the cumbersome processing and slow processing speed of computers, color images are not conducive to actual measurement and analysis. Therefore, we usually convert the collected color images into corresponding grayscale images for processing [23]. The process of converting a color image into a grayscale image is called image grayscale. There are many methods for grayscale image processing, and currently in the field of bridge crack recognition, the most used is the weighted average method. A color image is composed of three RGB channels, each with a value ranging from 0 to 255. The three channels are stacked together to generate a color image. The weighted average method calculates the grayscale image by weighted averaging the three channel components of the color image based on the importance of each channel and other indicators. During the process of forming, collecting, and transmitting digital images, they often suffer from various types of noise pollution due to limitations in the collection equipment, transmission media, and recording devices. These noise signals are completely unrelated to the crack information we need to identify, interfering with the normal recognition of crack information. In severe cases, they can affect the normal analysis of the image and potentially mislead erroneous information. The design scheme of crack detection nodes for highway bridges is shown in Figure 1.
Figure 1 depicts the design of the relevant nodes in the crack detection process of a highway bridge. The solution includes a brief description of the corresponding sensors, data storage, and power supply. In order to improve the recognition and quality of images, reduce excessive noise information, and prevent and modify the links that are prone to noise during the acquisition and transmission process. Filter and denoise the collected image information to identify cracks more clearly and accurately. There are many commonly used filtering methods, including mean filtering, median filtering, etc.
Traditional bridge crack manpower detection methods have many problems in terms of efficiency, accuracy and safety, and it is difficult to meet today’s fast and accurate detection requirements. Based on MAMBA network and digital image processing technology, this paper proposes an algorithm for the detection of apparent cracks in highway bridges. MAMBA simplifies the deep sequence model architecture by merging the previous SSM architecture with the Transformer’s MLP block into a single block, resulting in a simple, homogeneous architecture design that includes a selective state space. Like structured SSM, selective SSM is an independent sequential transformation that can be flexibly incorporated into neural networks. The method adopts the form of detection frame, which can effectively locate and qualitatively detect the cracks of concrete bridges. In this chapter, we will carry out crack image preprocessing and crack identification analysis based on MAMBA network.
The bridge crack images in this article are completely collected from different bridge crack samples in a random environment. The images contain several low-quality images. In order to ensure that the detection method can cope with various complex situations, such as uneven lighting, foggy and cloudy weather, and insufficient light caused by building obstruction on the bridge, a series of special treatments need to be carried out on the crack images. This chapter first introduces basic preprocessing algorithms including Retinex image enhancement algorithm, bilateral filtering, Laplace transform, histogram equalization, etc. Then, in order to extract better quality bridge crack texture information, a bilateral filtering method was proposed that can preserve crack edge details to the maximum extent and remove strong noise to filter out noise. Finally, a crack image enhancement algorithm combining histogram equalization and Laplace transform was proposed to improve image contrast for subsequent processing.
The experimental software is Matlab R2014b, and the effectiveness of the filtering algorithm in this paper is verified by comparing different filtering algorithms. Figure 2 shows the denoising effect of cracks. Figure 2a shows the original crack image, and Figure 2b shows the noise image with added Gaussian white noise of 0.09 intensity and salt and pepper noise of 0.2 noise density. Comparing the original bilateral filtering, wavelet transform, BM3D method with the improved bilateral filtering algorithm proposed in this paper, the experiment will analyze the advantages and disadvantages of the four methods from both subjective and objective perspectives.
Figure 2. Pretreatment Effect of Cracks. (a) Original image of crack. (b) Crack noise image. (c) Bilateral filtering. (d) Wavelet Transform. (e) BM3D method. (f) This article’s method.
As can be seen from Figures 2c-f, the improved bilateral filtering algorithm can remove strong noise while maximizing the retention of crack edge information. This article analyzes the filtering effect from both subjective and objective perspectives and obtains good conclusions. The HEL algorithm combines histogram equalization and Laplacian sharpening to enhance image quality, and its effectiveness has been validated through data analysis. Finally, experimental verification was conducted on the bridge crack images according to the preprocessing process described in this paper. The experimental results showed that although the preprocessing algorithm proposed in this paper consumed a certain amount of computation time, it could not only remove the effects of lighting and noise, cope with various complex situations in the images, but also improve the contrast and quality of the images, thereby improving the accuracy of deep learning algorithms in identifying cracks.
MAMBA combines the previous SSM architecture design with the MLP block of Transformer into one block, simplifying the deep sequence model architecture and forming a simple, homogeneous architecture design that includes a selective state space.
Like structured SSM, selective SSM is also an independent sequence transformation that can be flexibly integrated into neural networks. The H3 architecture is the foundation of the well-known homogeneous architecture design, typically consisting of blocks inspired by linear attention and interleaved MLP (Multi-Layer Perceptron) blocks [24, 25].
MAMBA simplifies this architecture by combining these two parts into one and stacking them evenly. They were inspired by the Gated Attention Unit (GAU), which also performs similar processing on attention.
Among Equation 1, n is the number of neurons in the input layer,
In summary, the selective SSM and MAMBA architecture extensions are fully recursive models, and several key features make them suitable as the backbone of a universal foundational model that runs on sequences.
Among Equation 2,
Among Equation 3, spread is the expansion coefficient.
Selectivity brings powerful performance to intensive models such as language and genomics. During the training process, the amount of computation and memory are linearly related to the length of the sequence, while during the inference process, since there is no need to cache previous elements, the autoregressive unfolding model only requires a constant amount of time for each step. The combination of quality and efficiency has improved the performance of actual data, with a sequence length of up to one million.
The input vector of the Gaussian function is:
Among Equation 4,
The corresponding function image is an S-shaped curve with a value between 0 and 1. The value of the
A network model based on the Pytorch deep learning framework for the task of detecting apparent cracks in bridges. Due to the time-consuming nature of hyperparameter optimization and the need for good computer hardware, we chose to use the default parameters evolved from hyperparameters on the large dataset COCO (Microsoft Common Objects in Context) as initial parameters for training and use the best training parameters obtained from other fields to improve the accuracy of object detection in this study. For small epigenetic diseases, the initial recognition effect is poor, which increases the output scale. Among them, set the number of early stop iterations to two to prevent overfitting. This article has set some training parameters to optimize the training performance of deep learning models. Firstly, set the size of the input image to 640 × 640 × 3 to better process the image data. Secondly, the momentum parameter is used to affect the speed at which the gradient descent algorithm converges to the optimal solution and setting it to 0.9 is beneficial for speeding up the training process.
In addition, to avoid overfitting of the model, the weight decay regularization term (decay) technique is used, set to 0.0005. Learning rate is also an important parameter that controls the speed of weight updates, with 0.002 chosen as the learning rate. For each training session, we set the batch size to 8, which helps improve the accuracy of the network gradient. Finally, a confidence threshold (score = 0.4) was set, and only targets with a confidence level of 0.4 or above were marked to ensure the accuracy of the model output results. The MAMBA model with CA attention mechanism is used as the best model for detecting apparent cracks in bridges. The training process is shown in Figure 3.
The network with added CA attention mechanism performs well in epigenetic disease recognition and has the shortest training time, with broad application prospects. However, some issues were also discovered during the testing and validation process. Mainly due to.
(1) The confidence level is not high. The confidence level of some images is relatively low, reaching around 0.3–0.5. When the confidence level is relatively low, it can lead to a certain degree of misjudgment of the type of target.
(2) There is a deviation in the target box. Some images have situations where the target box cannot contain all the targets or contains too much non target information.
(3) In some images, the neural network failed to correctly recognize the presence of small area disease targets, resulting in these targets being missed. This phenomenon may affect the performance and accuracy of the model.
(4) The previously used neural network model has singularity in the output of disease categories, that is, when a target box contains multiple different types of diseases, it cannot recognize them simultaneously and can only recognize the disease type with the most obvious features. For example, in the case where there are small cracks around the damaged disease, the current model can only identify the damaged disease and cannot simultaneously identify the crack disease.
In the process of training the improved MAMBA network on the RLH processed dataset for bridge cracks, it was found that the accuracy of Accuracy2 and Accuracy3 networks in the network after training to the sixth initiation layer was basically the same as the accuracy over time, and the values of loss2 and loss3 were also similar. Since the accuracy and loss value of crack recognition after the sixth initiation have reached the training effect of the complete network, it is completely reasonable to remove the seven and subsequent initiation layers, which can greatly reduce the training time. After post analysis, the reason for the above situation is that the model is a classification model for multiple labels in the ILSVRC (ImageNet Large Scale Recognition Challenge) competition, and some of the detailed features of individual categories are difficult to extract, so more initiation layers are needed to extract their small features. The crack texture is relatively simple, so by the sixth initiation layer, its features can already be extracted. Therefore, it is reasonable to remove unnecessary initiation layers after the seventh layer to improve the training speed of the network. Figure 4 shows accuracy and training after the initiation layer.
This chapter mainly discusses the practical application of object detection algorithm based on MAMBA network model in the recognition of apparent diseases of concrete bridges. Firstly, traditional object detection methods and deep learning-based object detection methods were introduced, and their respective advantages and disadvantages were analyzed. Finally, the MAMBA network model was chosen as the deep learning training model. Then, the structure and related principles of the YOLOv5 model were introduced in detail, including the input end, Backbone network layer, Neck layer, and Head, while adjusting the corresponding parameters according to the characteristics of the disease. Subsequently, a combination of publicly available disease datasets and images collected by drones was used to collect multiple concrete surface disease images and manually annotate them. The samples were then subjected to batch image enhancement processing and trained using the YOLOv5 model. In addition, CBAM attention mechanism and CA attention mechanism was separately added to the MAMBA network model to improve its performance. Finally, the training results of three different models were compared and evaluated, the training effects were summarized, and the reasons for them were analyzed.
This article mainly uses traditional digital image processing techniques and deep learning principles to identify apparent defects in concrete bridges. The problem of identifying apparent defects in concrete bridges was studied, and a series of steps were implemented from the collection of bridge apparent defect information to detection and result output. This study first preprocesses the collected crack image data, including grayscale, filtering, and binarization operations. During this process, several commonly used methods were compared and the most suitable method for preprocessing crack images was determined. Subsequently, edge detection, image stitching, and geometric dimension analysis were performed on the crack images. Finally, identify key features such as the width, length, and direction of cracks.
This study uses the MAMBA network as the training model for deep learning, and adjusts the corresponding parameters based on the features of apparent disease images. Multiple disease images were created and annotated, and a MAMBA network model was built to train the samples. The CBAM attention mechanism and CA attention mechanism was added to the MAMBA network model for comparison and analysis. It was found that the MAMBA network model with CA attention mechanism had the best accuracy and was more suitable for detecting apparent diseases in concrete bridges. Although deep learning frameworks perform well in disease detection, the actual types of bridge diseases are diverse, and there are interference factors such as incomplete data, insufficient lighting, and shooting angles, which may have a negative impact on the performance of the model. In order to improve its accuracy and robustness, methods such as weakly supervised learning and unsupervised learning can be considered, and training methods and parameters can be optimized for the inherent features of certain diseases, thereby further improving model performance.
The original contributions presented in the study are included in the article/supplementary material, further inquiries can be directed to the corresponding author.
YF: Conceptualization, Data curation, Writing–original draft. ZQ: Methodology, Project administration, Supervision, Writing–review and editing. DJ: Formal Analysis, Funding acquisition, Investigation, Software, Writing–original draft.
The author(s) declare that financial support was received for the research, authorship, and/or publication of this article. National Natural Science Foundation of China (Grant No. 52108315), Research on the Dynamic Response Mechanism of Soil Moisture under the Joint Action of Vegetation and Precipitation on Slope. Natural Science Foundation of Hubei Province for Young Scholars Project (2021CFB286), Time-varying Reliability Analysis of Rainfall Infiltration into Slope Based on Correlated Follower Airport.
The authors declare that the research was conducted in the absence of any commercial or financial relationships that could be construed as a potential conflict of interest.
The author(s) declare that no Generative AI was used in the creation of this manuscript.
All claims expressed in this article are solely those of the authors and do not necessarily represent those of their affiliated organizations, or those of the publisher, the editors and the reviewers. Any product that may be evaluated in this article, or claim that may be made by its manufacturer, is not guaranteed or endorsed by the publisher.
1. Zhang C. Control force characteristics of different control strategies for the wind-excited 76-story benchmark building structure. Adv Struct Eng (2016) 15(4):423–34. doi:10.1260/1369-4332.17.4.543
2. Nguyen ITQ. Higher-order feasible building blocks for lattice structure of oversampled linear-phase perfect reconstruction filter banks. Signal Process. (2009) 13(2):432–45. doi:10.1016/j.sigpro.2009.03.005
3. Braham M, Jaspart JP. Is it safe to design a building structure with simple joints, when they are known to exhibit a semi-rigid behaviour? J Constructional Steel Res (2004) 60(3-5):713–23. doi:10.1016/s0143-974x(03)00138-x
4. Shultz DA. Structure–property relationships in cross-conjugated, high-spin, dinuclear ligands: building blocks for open-shell molecules and materials - ScienceDirect. Polyhedron (2001) 20(11):1627–31. doi:10.1016/S0277-5387(01)00664-7
5. Xu J, Crowley MF, Smith JC. Building a foundation for structure-based cellulosome design for cellulosic ethanol: insight into cohesin-dockerin complexation from computer simulation. Protein Sci (2010) 18(5):949–59. doi:10.1002/pro.105
6. Chad S, Kuech T, et al. Continuously phase-matched terahertz difference frequency generation in an embedded-waveguide structure supporting only fundamental modes. Opt express (2008) 42(1):321–35.
7. Dittmer MP, Kohorst P, Borchers L, Stiesch M. Influence of the supporting structure on stress distribution in all-ceramic FPDs. Int J Prosthodont (2010) 23(1):63–8.
8. A BP, B YCC. Hydration and pore-structure characteristics of high-volume fly ash cement pastes. Construction Building Mater (2020) 35(9):236–43. doi:10.1016/j.conbuildmat.2021.122390
9. Giovanni Z. Opportunities for materials science: from molecules to neural networks. Mrs Bull (2019) 44:124–9. doi:10.1557/mrs.2019.23
10. Tariq Z, Mahmoud M. A new correlation for gas deviation factor for high temperature and high-pressure gas reservoirs using neural networks. Energy and Fuels (2019) 33(MAR.):2426–36. doi:10.1021/acs.energyfuels.9b00171
11. Merazi-Meksen T, Boudraa M, Bachir B. Mathematical morphology for TOFD image analysis and automatic crack detection. Ultrasonics (2014) 54(6):1642–8. doi:10.1016/j.ultras.2014.03.005
12. Adhikari RS, Moselhi O, Bagchi A. Image-based retrieval of concrete crack properties for bridge inspection. Automation in Construction (2014) 39:180–94. doi:10.1016/j.autcon.2013.06.011
14. Xue-Jun X, Xiao-Ning Z. Crack detection of reinforced concrete bridge using video image. J Cent South Univ (2013) 20(9):2605–13. doi:10.1007/s11771-013-1775-5
15. Talab AMA, Huang Z, Fan X, et al. Detection crack in image using Otsumethod and multiple filtering in image processing techniques. Optik (2016) 127(3):1030–3. doi:10.1016/j.ijleo.2015.09.147
16. Wang Y, Zhang JY, Liu JX, Chen ZP, et al. Research on crack detection algorithm of the concrete bridge based on image processing. Proced Computer Sci (2019) 154:610–6. doi:10.1016/j.procs.2019.06.096
18. Wang P, Huang H. Comparison analysis on present image-based crack detection methods in concretestructures. (2010).
19. Chen L, Zhang Z, Yang G, Zhou Q, Xia Y, Jiang C. Evidence-theory-based reliability analysis from the perspective of focal element classification using deep learning approach. ASME J Mech Des (2023) 145(7):071702. doi:10.1115/1.4062271
20. Chen Q, Zhang Z, Fu DJC, Hu D, Jiang C. Enhanced Gaussian-mixture-model-based nonlinear probabilistic uncertainty propagation using Gaussian splitting approach. Struct multidisciplinary optimization (2024) 67(4):49. doi:10.1007/s00158-023-03733-3
21. Sivaram A, Das L, Venkatasubramanian V. Hidden representations in deep neural networks: Part 1. Classification problems. Comput and Chem Eng (2020) 134(4):106669–.17. doi:10.1016/j.compchemeng.2019.106669
22. Ichinose N. Quasiperiodic-chaotic neural networks and short-term analog memory. Int J Bifurcation Chaos (2021) 13(9):453–62. doi:10.1142/S0218127421300032
23. Stokes PW, Cocks DG, Brunger MJ, White RD. Determining cross sections from transport coefficients using deep neural networks. Plasma Sourc Sci Technology (2020) 29(5):055009–65. doi:10.1088/1361-6595/ab85b6
24. Bergen GHHV, Duenk P, Albers CA, et al. Bayesian neural networks with variable selection for prediction of genotypic values. Genet Selection Evol (2020) 52(1):291–302. doi:10.1186/s12711-020-00544-8
Keywords: bridge cracks, classification and localization, MAMBA network, CA attention mechanism, hyperparameter evolution
Citation: Fan Y, Qin Z and Jiang D (2025) Research on the classification and localization method of cracks in highway bridges based on MAMBA network. Front. Phys. 13:1541689. doi: 10.3389/fphy.2025.1541689
Received: 08 December 2024; Accepted: 24 February 2025;
Published: 25 March 2025.
Edited by:
Matteo Cirillo, University of Rome Tor Vergata, ItalyCopyright © 2025 Fan, Qin and Jiang. This is an open-access article distributed under the terms of the Creative Commons Attribution License (CC BY). The use, distribution or reproduction in other forums is permitted, provided the original author(s) and the copyright owner(s) are credited and that the original publication in this journal is cited, in accordance with accepted academic practice. No use, distribution or reproduction is permitted which does not comply with these terms.
*Correspondence: Dongzhu Jiang, ZHpqaWFuZzIwMjBAb3V0bG9vay5jb20=
Disclaimer: All claims expressed in this article are solely those of the authors and do not necessarily represent those of their affiliated organizations, or those of the publisher, the editors and the reviewers. Any product that may be evaluated in this article or claim that may be made by its manufacturer is not guaranteed or endorsed by the publisher.
Research integrity at Frontiers
Learn more about the work of our research integrity team to safeguard the quality of each article we publish.