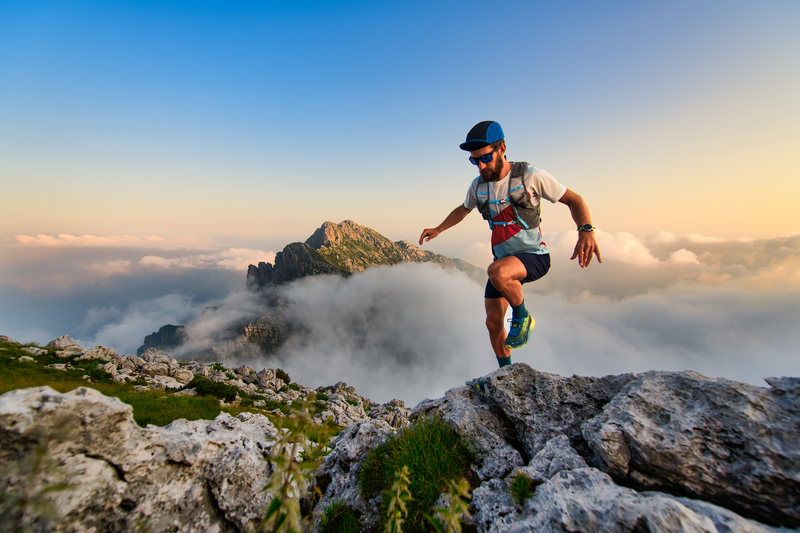
95% of researchers rate our articles as excellent or good
Learn more about the work of our research integrity team to safeguard the quality of each article we publish.
Find out more
ORIGINAL RESEARCH article
Front. Phys. , 29 January 2025
Sec. Social Physics
Volume 12 - 2024 | https://doi.org/10.3389/fphy.2024.1515842
Introduction: With an enormous number of hand images generated over time, leveraging unlabeled images for pose estimation is an emerging yet challenging topic. While some semi-supervised and self-supervised methods have emerged, they are constrained by their reliance on high-quality keypoint detection models or complicated network architectures.
Methods: We propose a novel selfsupervised pretraining strategy for 3D hand mesh regression. Our approach integrates a multi-granularity strategy with pseudo-keypoint alignment in a teacher–student framework, employing self-distillation and masked image modeling for comprehensive representation learning. We pair this with a robust pose estimation baseline, combining a standard vision transformer backbone with a pyramidal mesh alignment feedback head.
Results: Extensive experiments demonstrate HandMIM’s competitive performance across diverse datasets, notably achieving an 8.00 mm Procrustes alignment vertex-point-error on the challenging HO3Dv2 test set, which features severe hand occlusions, surpassing many specially optimized architectures.
Image-based 3D hand reconstruction technology has widespread applications in the smart film industry, such as motion capture, special effects synthesis, virtual production, post-production animation modification, and interactive film production. Meanwhile, 3D hand mesh estimation from monocular RGB images has drawn great attention in computer vision research [1, 2] driven by its potential in various applications, such as action recognition [3, 4], digital human modeling, simultaneous localization and mapping (SLAM) [5–10], and AR/VR. However, training a high-quality hand estimation model is challenging due to complex backgrounds and severe self-occlusion. Furthermore, it is laborious and costly to collect high-quality training pairs, especially in the format of 3D mesh. A limited amount of image-mesh training data are available, making it difficult to train effective and generalizable models. Weakly supervised methods detecting 2D keypoints or measuring noisy depth maps [11] or kinematic priors [12] from off-the-shelf models have been proposed to improve the accuracy of supervised-trained models. However, these methods heavily rely on fine-grained keypoint detectors, such as MediaPipe [13], which struggle with the wide variety of wild images encountered in practice and may produce many noisy labels.
Self-supervised learning is a promising technique for addressing the above problem by exploiting the large quantity of unlabeled image data generated over time. Masked image modeling (MIM) pretraining has emerged as a new paradigm in self-supervised learning based on the vision transformer [14] architecture that divides images into individual patches. In MIM pretraining, we randomly mask a specified ratio of image patches and set the self-supervised learning target to reconstruct the masked patches. Previous works [15, 16] have demonstrated that MIM-based methods can learn better local and global representation than conventional self-supervised methods based on contrastive learning [17]. In contrast to traditional self-supervised methods based on contrastive learning, which focus on high-level feature representation suitable for image classification, MIM-based methods can learn better local and global representations. This is especially critical for low-level, fine-grained regression tasks such as 3D hand estimation, where capturing the equivalence of geometric transformations is essential. The potential ability of MIM to reconstruct masked patches allows the model to understand the spatial relationships within an image at a finer granularity, making it more adept at handling detailed structures like the human hand.
However, most existing self-supervised work focuses on recognition tasks and aims to learn features appropriate for high-level image classification tasks. In low-level regression tasks, mainstream methods cannot capture the equivalence of geometric transformation, a critical characteristic of human/hand pose or mesh regression. Therefore, most state-of-the-art MIM self-supervised pretraining approaches must be adapted for regression tasks such as 3D hand estimation. Figure 1 exhibits the difference between our MIM approach and the previous ones. MIM’s extension to regression tasks like 3D hand mesh estimation offers significant advantages. It leverages the strengths of MIM—such as detailed feature capture and understanding of spatial relationships—while introducing mechanisms specifically tailored for the challenges of regression tasks. We confirmed the abovementioned findings through experiments in Section 4.3.
Figure 1. Comparison with other MIM self-supervised frameworks in vision tasks. Left: MIM techniques such as [16] encourage the model to recover the masked patch of images. Middle: iBOT [15] utilized a vision transformer [14] to extract multi-level features for image details and semantics. Self-distillation mechanics is introduced to learn the semantic [CLS] feature, which is invariant [15] under task-specific transformation. Right: our HandMIM pruned the MIM-training ViT architecture to fit the properties of the regression task. The pose-aware alignment mechanics are designed to enforce the transformation equivalence [18] of [CLS] and the patch features, given the masked pose image, which boosts the regression task. Note that our framework adds the geometric equivalence property to the [CLS] token via pseudo-keypoints and simultaneously learns global, patch, and pixel-level features, which are specially tailored for the fine-grained regression tasks.
In this paper, we conduct the first attempt to apply the effective masked image modeling (MIM) self-supervised technique to 3D hand estimation tasks. We propose HandMIM, a unified and multi-granularity self-supervised pretraining strategy optimized for pose regression tasks. During the pretraining period, we use a teacher-student self-distillation approach, where input hand images are augmented into two views that vary in sizes, rotations, colors, and other factors. The student network is then tasked with reconstructing masked tokens under the guidance of the teacher network. To ensure that the class tokens are semantic with pose-aware knowledge, we introduce the pseudo-keypoint alignment operation in the latent feature space. This operation allows us to undo the geometric transformation in the format of 2D pseudo-keypoints, enabling the network to learn pose equivalence between cross-view tokens. To facilitate high-level and low-level recognition, we adopt token-level recovery between parallel-view masked tokens and pixel-level reconstruction between masked input images and recovered images, respectively. It is important to note that the token recovery is conducted in the same latent space as the pose-aware alignment. We sketch our method in Figure 2 and compare it with related self-supervised works [18, 19] for hand pose/shape estimation. PeCLR [18] is the current state-of-the-art hand pose estimation work using a self-supervised training approach. Our model differs from PeCLR [18] in the following aspects: First, we learn global features using a self-distillation manner rather than the contrastive learning paradigm. Second, we designed the pose-aware keypoint alignment mechanism, making HandMIM exploit the pose knowledge, which as originally coupled with task-irrelated information (such as color, affine transformation, etc.) from the image. Last, token-level self-distillation and pixel-level reconstruction are imposed to learn the local or low-level features, which are vital for regression tasks like 3D mesh estimation. Accordingly, HandMIM overcomes the limitations of contrastive learning and other self-supervised approaches by incorporating multi-granularity feature learning and pose-aware mechanisms in a unified self-distillation-based MIM framework. This combination results in superior performance on 3D hand mesh estimation tasks. In the supervised fine-tuning period, most existing pose estimation methods rely on a combination of grid convolution, transformer structure, and a dedicated and complicated prediction head for better results. We designed a simple yet effective pose estimation pipeline with a standard vision transformer as the backbone, attached by a PyMAF [20] decoder head to promote mesh-image alignment and use the MANO [21] parameters to represent the estimated hand mesh. We loaded the self-supervised, pre-trained weights to transformer blocks and fine-tuned the whole network for hand pose estimation. Extensive experiments demonstrated that our HandMIM can learn better features to improve 3D hand pose estimation precision than alternative self-supervised and fully supervised methods under the same amount of labeled training data. We conducted our main experiments on two mainstream and challenging 3D hand mesh estimation datasets, FreiHAND [22] and HO3Dv2 [23]. We implemented HandMIM on three different sizes of vision transformers, namely ViT-Small, ViT-Base, and ViT-Large, respectively, which show strong scalability. After HandMIM pretraining, we achieved a performance boost of
Figure 2. Comparison with other self-supervised frameworks for hand shape/pose estimation. Left: TempCLR [19] proposes exploiting the temporal relations to generate positive and negative samples for contrastive learning. Middle: PeCLR [18] is noted for implementing a transformation equivalence constraint on extracted global features. It is important to note that both TempCLR [19] and PeCLR [18] are contrastive learning methods that focus solely on global features, neglecting multi-level features that could significantly refine the predicted hand mesh vertices. Right: our contribution stands out with the introduction of pose-aware alignmentmechanics and multi-granularity feature learning, which is the key difference between HandMIM and competitive methods. We first align pseudo-keypoints (represented by the [CLS] token) in the latent space. Concurrently, the [patch] tokens capture detailed features essential for mesh estimation and refinement. For clarity, the token-level self-distillation mechanics are omitted in this comparison. Unlike contrastive learning approaches such as PeCLR [18], which focus on global high-level features, HandMIM’s pixel-level reconstruction enhances the model’s ability to rebuild fine-grained geometric details in hand mesh vertex prediction. This integration helps the model recover from occlusions and challenging hand poses. By predicting the original pixel values from masked input, HandMIM is more effective at handling transformations and occluded regions, which is evident from the improved performance on datasets with hand–object interaction (e.g., HO3Dv2).
Conclusively, the main contributions of our work are in four folds:
1. We adopted a new self-distillation method for 3D hand mesh estimation. This method markedly enhanced the efficiency of learning from potentially unlimited unlabeled hand image data.
2. We designed the pose-aware keypoint alignment mechanism for the MIM paradigm, making HandMIM exploit the pose knowledge, which was originally coupled with task-irrelated information (such as color and affine transformation) from images.
3. The integration of token-level self-distillation and pixel-level reconstruction in our framework allowed the effective learning of both high- and low-level features. These features are crucial for fine-grained regression tasks, including hand mesh estimation.
4. To our knowledge, HandMIM represented the inaugural model pre-trained with masked image modeling mechanics, specifically in the field of hand mesh estimation.
Estimating hand poses aims to predict hand information from a monocular RGB/depth image and can be broadly classified into parametric and non-parametric methods. Parameter methods [25] use statistical priors from parametric hand models like MANO [21] to constrain the regression space and make the prediction more robust in cases of severe occlusions. Except for fully supervised manners, pioneer works [12] predict the MANO parameters with weak supervision, such as hand masks, depth maps, or 2D annotations. Non-parametric methods [26, 27] aim to predict the entire mesh vertices directly using either graph convolutional networks or transformer blocks. Although these methods can generate results that align better with the input image, they are more prone to failure in cases of occlusions and truncations. More recent work has focused on explicitly modeling hand–hand [28], complicated hand–object interactions [29], high inference speed [30], and increased robustness to occlusions [29] that pose new and more complex challenges. Instead of designing dedicated and resource-intensive heads, we proposed a lightweight head that regresses MANO parameters from a pre-trained standard ViT for both single-hand estimation and hand–object interaction predictions.
ViT [14] first introduced vision transformers to the visual field by patching images for transformer blocks. This approach has led to significant progress in image recognition and has also shown promising results in human and hand estimation tasks [1, 2, 27]. For example, Mesh Graphormer [1] designs a transformer-based head fused with graph convolution layers. HaMeR [31] directly utilizes ViT with a transformation head to predict MANO parameters and camera extrinsic with several mixed-label labeled datasets. Keypoint Transformer [2] first collects candidate 2D keypoints and utilizes a transformer encoder-decoder for the mesh predictions. AMVUR [26] further proposes a probabilistic attention-based mesh vertices model to estimate the prior probability distribution of joints and mesh vertices to improve their feature representation.
Most prior works have designed complex structures on top of the transformer or attention blocks. Accordingly, standard transformers cannot easily achieve competitive performance. Our approach attempts to leverage large quantities of unlabeled hand images and surpass existing methods solely based on the standard ViT backbone without any delicate domain-related architecture, demonstrating the effectiveness of our self-supervised regression learning algorithm.
Self-supervised learning is an approach to learning effective feature representation from abundant unlabeled images. Contrastive learning techniques [17] aim to learn by constraining positive pairs to become close in feature space while pushing negative pairs apart and have been employed in the hand pose estimation tasks for improved performance [18, 19, 32]. Masked image modeling (MIM) [15, 16, 33] methods are new paradigms of self-supervised learning that randomly mask a portion of the input image and reconstruct the masked parts via reasoning other unmasked parts. The knowledge of masked images can be learned in alterable manners, including dVAE codebooks in BeiT [33], raw RGB pixels in MAE [16], etc. Previous MIM studies have focused on learning representative features for image classification tasks but have neglected the specificity of pose or mesh regression tasks. To our knowledge, this is the first time that MIM techniques have been extended to such 3D regression tasks. MIM contributes to the proposed HandMIM in two aspects. First, it allows the model to learn local and global features better than traditional contrastive learning methods. It captures spatial relationships within images at a finer granularity, making it more adept at handling detailed structures like human hands. Second, it constitutes an important part of our designed multi-granularity loss functions, which involve both token-level recovery between parallel-view masked tokens and pixel-level reconstruction between masked inputs and recovered images. This dual-level loss facilitates high-level and low-level recognition, ensuring comprehensive representation learning.
In this section, we will discuss the detailed architecture of HandMIM. The pipeline of HandMIM can be found in Figure 3. We start with preliminaries, including basic knowledge of vision transformers, masked image modeling, and self-distillation techniques in Section 3.1. Then, we introduce the detailed design of HandMIM, including pose-aware keypoint alignment in Section 3.2, token-level self-distillation in Section 3.3, and pixel-level reconstruction in Section 3.4. Finally, we illustrate how to apply pre-trained features after self-supervised learning for 3D hand mesh estimation tasks in Section 3.5. The PyTorch-like pseudocode of HandMIM is listed in Algorithms 1–3.
Figure 3. Overall framework of HandMIM. During the self-supervised pretraining phase, we design multi-granularity tasks to acquire pose-aware knowledge, high-level token recovery, and low-level pixel reconstruction. We propose a simple baseline based on the standard vision transformer architecture and the PyMAF [20] decoder in the fine-tuning period. We load the pre-trained weights onto the model, which allows us to estimate 3D hand poses by leveraging the network’s ability to learn pose-sensitive information in hand images.
Given input images
The output of the self-attention module is then passed through an inverted bottleneck multi-layer perceptron (MLP), also known as the feed-forward network. In practice, vision transformers are assembled by stacking a series of transformer blocks. We can obtain models of varying sizes by varying the channel width and layer depth of vision transformers.
Masked image modeling (MIM) is a self-supervised learning technique that has been demonstrated to be a general method for image recognition tasks in many recent works [33]. Given input tokens
MIM encourages the model to learn robust local and global image representations, which is especially important for tasks requiring fine-grained understanding, such as 3D hand mesh estimation.
Self-distillation is a common technique adopted in recent self-supervised learning frameworks [34, 35]. Given an input image
We observe that the 2D pose of hands in input images remains equivalent after some spatial data augmentation, such as random rotation and resizing operations, while the positional information is altered. As justified in our experiments, existing mainstream self-supervised learning methods fail to capture the knowledge of “poses.” In this work, we propose the idea of pose-aware keypoint alignment to extract the pose-relevant knowledge. This is critical for 3D hand mesh estimation tasks, where understanding and preserving the geometric relationships between keypoints (or joints) is essential for accurate reconstruction. Moreover, we choose this method because it efficiently and effectively captures and utilizes pose-relevant knowledge, integrates seamlessly with the self-distillation and multi-granularity learning paradigms, and enhances the overall performance and robustness of 3D hand mesh estimation.
Consider a point
where
Then, we apply the softmax function to
The knowledge of masked image modeling can be acquired through a self-distillation approach proposed by DINO [36]. We treat self-supervised learning as a discriminative task involving two backbones with identical architecture, which play the roles of a teacher network
To fully recognize the images, we use two random image augmentations, denoted as
Note that the softmax function is applied to the channel dimension. We use uppercase letters, that is,
We design specific tasks of self-supervised learning for the class tokens, considering their semantic meanings. For the class tokens, we aim to extract the pose of the original images, which is equivalent after the inverse operation of spatial data augmentations, implemented as pose-aware keypoint alignment in Section 3.2. Because we expect images under different augmentations to have the same pose expression, we adopt a cross-entropy loss between the cross-view images and apply the self-distillation approach in Section 3.1 to measure the discrepancy between teacher and student distribution. Specifically, we obtain the
During the backward period, only the student network requires gradient backpropagation, as we treat the output of the teacher network as ground truth. Subsequently, we update the teacher network through an exponentially moving average (EMA) using the student network.
Given the patch output of the transformer backbone, which represents the spatial knowledge of input images, we can define the patch loss
Hand pose estimation is a low-level task that involves directly analyzing image pixels, in contrast to image classification. Although token-level self-distillation may be effective for higher-level knowledge, it may lack the necessary low-level understanding. To address this, we propose a pixel-level reconstruction module. Because transformer tokens are applied in a patch-based manner, we integrate a pyramid fusion layer following certain intermediate transformer layers and gradually up-sample using transposed convolution (T-Conv). The convolution stride is set to 2. The resulting pyramid fusion output feature maps
In common practice, vision transformers use a patch size of 16; therefore, four iterations of transposed convolution are adopted to recover the original shape of input image
where
The final loss function Equation 12 is the sum of the losses mentioned above:
The above loss function indicates that HandMIM can capture both local detail features and global geometric context via a vision transformer backbone. The transformer architecture naturally handles multi-scale information, but HandMIM goes further by introducing a mechanism that specifically targets different levels of granularity. More specifically, the [Patch] tokens represent local regions of the image and are used to capture fine-grained geometric features essential for mesh estimation and refinement. Pseudo keypoints are aligned in the latent space using the [CLS] token, which acts as a global representation of the entire image. By aligning these keypoints, the model can better understand the pose equivalence between different views of the hand after applying spatial augmentations. Finally, the combination of pixel-level reconstruction and multi-granularity feature learning allows HandMIM to learn how to recover pixels from occlusions and handle complex hand–object interactions more effectively, which is particularly beneficial on datasets like HO3Dv2, which feature severe hand occlusions.
To evaluate the effectiveness and benefits of HandMIM self-supervised pretraining, we fine-tune the pre-trained vision transformer backbone on a supervised 3D hand mesh estimation task. Specifically, we incorporate a keypoint feedback loop after the backbone, similar to the approach used in PyMAF [20], to predict MANO [21] parameters, including joint rotation
The MANO parameter loss
where
In this section, we conducted extensive experiments to evaluate the proposed self-supervised pretraining framework HandMIM. We first introduce our settings on HandMIM pretraining in Section 4.1. Then, we show the results of our pre-trained model on 3D hand mesh estimation tasks in Section 4.2. Finally, we present in-depth analysis and ablation studies in Section 4.3.
We employ vision transformers [14] as our backbone in different sizes, including ViT-Small (ViT-S), ViT-Base (ViT-B), and ViT-Large (ViT-L). Details of the architectures can be found in the supplementary materials. We collect the multi-level features from layers [3,6,9,12] for pixel reconstruction with a decoder consisting of linear layers for feature fusing and transposed convolutions for up-sampling. Input images are augmented through random resizing within the range
As there are currently no standardized datasets for hand pose self-supervised learning, we collect hand images across a variety of datasets for sufficient hand pose and background distributions, including the FreiHAND [22] training set (FreiHAND is a 3D hand pose dataset that records different hand actions performed by 32 people. MANO-based 3D hand pose annotations are provided for each hand image. This training set provides a large number of hand images with green screen or composite backgrounds, offering a wide range of hand poses.), Youtube3DHands [11] (The dataset contains various in-the-wild images, with automatically acquired 3D annotations via key point detection and MANO fitting. It has 47,125 effective frames.), and COCO-WholeBody train and unlabeled images [37] (It is a large-scale dataset with keypoint and bounding box annotations. Approximately 130 K faces and left/right-hand boxes are labeled, resulting in more than 800 K hand keypoints and 4 M face keypoints in total.) For datasets with hand annotations, we directly enlarge the bounding boxes of the hand annotations by a ratio of 2.0 and then crop the hand image. For datasets that do not come with hand annotations, such as some parts of COCO-WholeBody or other unlabeled image collections, we utilized MediaPipe [13], an open-source framework developed by Google. MediaPipe is specifically chosen due to its robust and superfast performance in detecting hands within images. By applying MediaPipe’s hand detection capabilities, the researchers were able to identify and crop out regions of interest (ROIs) containing hands, even in the absence of explicit annotations. This step was crucial because it allowed the inclusion of a vast amount of unlabeled data into the training process, thereby increasing the diversity and quantity of training samples.
We evaluated the performance of HandMIM models against several competitive methods in 3D hand mesh estimation. Our experiments demonstrate that pretraining HandMIM models significantly enhances the accuracy and quality of visualizations in 3D hand mesh estimation tasks and achieves competitive performance in multiple datasets and metrics.
For evaluation, we use two challenging publicly available hand pose estimation datasets, FreiHAND [22] and HO3D v2 [23], in our experiments. The FreiHAND dataset comprises 130,240 training images with a green screen or composite background and 3,960 test images with a real background. HO3Dv2 is a hand–object interaction dataset with complex occlusion that contains 77,558 hand–object 3D pose-annotated RGB images and their corresponding depth maps, 10 different human subjects (three female and seven male individuals), and 10 different objects from the YCB [38] dataset, and its evaluation process is conducted online. Note that the HO3Dv2 dataset is particularly challenging for 3D hand mesh estimation due to its focus on real-world scenarios that introduce a variety of difficulties not commonly found in more controlled datasets. The characteristics of the dataset include severe hand–object occlusions, complex interactions with objects, high-quality annotations, and the online evaluation process.
During training, we set the batch size to 128 and then crop and resize the hand image to
Table 1. Details of the vision transformer architecture, as well as the pretraining and inference time in HandMIM.
We incorporate multiple evaluation metrics for comprehensive analysis and comparison. We use joint-point-error (JPE) and vertex-point-error (VPE) to denote the average L2 distance between the ground truth and predicted keypoints and mesh vertices, respectively. We prefix the metrics with PA and MP to denote Procrustes alignment and scale-and-translation alignment. F-scores are defined as the harmonic means between recall and precision between two meshes given a distance threshold. We also report the area under curve (AUC) following common practice, which denotes the area under the percentage-of-correct-keypoints (PCK) curve for threshold values between 0 mm and 50 mm in 100 equally spaced increments. We report our evaluation results in
We compare our approach with existing methods [1, 18, 24, 27, 30, 39–41] on the mainstream FreiHAND dataset. We conduct self-supervised pretraining with HandMIM using ViT-Small (ViT-S), ViT-Base (ViT-B), and ViT-Large (ViT-L). As shown in Table 2, fine-tuning our approach using HandMIM pre-trained weights consistently improves the performance on both datasets compared to the commonly used ImageNet pre-trained weights (ViT-Small/Large-ImageNet + PyMAF), confirming the effectiveness of HandMIM pretraining. We plot the mesh and pose AUC in Figure 4. Notably, even with the lightweight ViT-Small with 22 M parameters, our approach achieves a competitive Procrustes alignment vertex-point error (PAVPE) of 6.6 mm, which further improves to the best PAVPE of 6.2 mm when we employ ViT-L as the backbone.
Table 2. Results on the FreiHAND [22] dataset. We perform our results before (fine-tuned from ImageNet pre-trained weights) and after HandMIM pretraining and list the lifting ratio compared with the ViT-S baseline.
Figure 4. Pose and mesh AUC comparison with some competitive methods on the FreiHAND and HO3D datasets. * indicates the method is supervised and trained with extra 2D/3D labeled data. It can be observed from the plot that our method achieves the best performance on both datasets for both meshes and poses AUC values with ViT-B as the backbone. (A) FreiHAND Mesh AUC, (B) FreiHAND Pose AUC, (C) HO3D v2 Mesh AUC, and (D) HO3D v2 Pose AUC.
For HO3Dv2, existing methods [29, 43]; [26] design various complex strategies via hand–object interaction information to improve the estimation accuracy. For example, HandOccNet [29] carefully designs a network to tackle severe hand occlusion.
Table 3. Results on the HO3D v2 [23] dataset. Compared with current methods specially designed for hand–object interactions, we achieve better results under a standard backbone with no special operation. All the listed results use the same labeled dataset for supervised learning.
We visualized and compared the hand mesh predictions of our proposed method with some competitive methods on the test sets of FreiHAND [22] and HO3Dv2 [23] in Figures 5, 6 respectively. Compared to existing methods, our method achieved better estimation accuracy for challenging viewpoints, severe occlusion, and difficult gestures. For images in the HO3Dv2 dataset under severe hand–object occlusion, our method can capture local finger clues and infer the overall wrist pose and plausible finger positions, demonstrating its superior robustness to alternative methods, which often adopt complex mechanisms to handle those occlusions. More visualization examples are shown in Figure 7.
Figure 5. Visualizations on the FreiHAND [22] test set. From left to right, we show the input images, the predictions from I2L-MeshNet [24], Tang et al. [41], our HandMIM-Small, and the ground truth. Our method is more robust for hard viewpoints, occlusion, and complicated hand gestures.
Figure 6. Visualization of the HO3D v2 [23] test set. We show the input images, the predictions from HandOccNet [29], Liu et al. [43], and our HandMIM-Small and AMVUR [26]. Our method can capture more precise poses even under the corruption and occlusion of complex objects, achieving results comparable to the strong baseline method AMVUR [26] while using many fewer parameters (195 M versus 22 M).
Figure 7. Qualitative comparison with several methods on the HO3D v2 test set. From left to right, it shows the input images, the overlaid results by HandOccNet [29], Liu et al. [43], and our best HandMIM-Large model.
In this subsection, we presented a series of convincing analysis experiments and ablations to evaluate the effectiveness of HandMIM. We demonstrated the superiority of our method against existing self-supervised methods through comprehensive comparisons. To assess the generalizability of our method, we perform linear prob, cross-dataset, partial fine-tuning analysis, and visualizations of HandMIM.
As we enforce the pose-sensitive knowledge in our latent feature, we can adopt the linear prob strategy to validate their effectiveness. Linear probing is an intuitionistic method for a self-supervised-trained model to show the quality of representation learning by freezing the pre-trained backbone and using a simple MLP layer to predict the output. We use the 2.5D joint representation to regress 2D and 3D keypoints jointly. Concretely, we learned two 3-layer multilayer perceptrons (MLPs) to predict 2D keypoints and 1D relative depth, respectively. The resulting 3D keypoints are calculated according to the camera’s intrinsic parameters. We trained our MLP layer on FreiHAND [22] and split the training and validation set afterward [18]. Note that we only train for 10 epochs with the AdamW optimizer and set the initial lr as 0.01. We report the predicted 2D keypoint error and 3D joint error, as shown in Table 4, which demonstrates: 1) our HandMIM can learn better features than previous self-supervised methods such as PeCLR [18]. 2) The geometric equivalence ingrained in our features plays an important role in predicting accurate 2D/3D keypoints when compared against iBOT [15], which does not encode any pose-aware mechanism in their self-supervised framework and thus obtains a much higher prediction error rate using their features.
Table 4. Results of linear probe regression. We compared our method with the mainstream self-supervised learning method, and our HandMIM outperforms existing methods by a large margin.
As shown in Table 5, we compared the performance of our proposed pose-aware method for 3D hand mesh estimation with two representative self-supervised learning methods, the mainstream masked image modeling method iBOT [15] and the contrastive-learning-based method PeCLR [18]. We conducted these comparisons using the same ViT-Small backbone and the same amount of training data. Our results indicate that HandMIM outperforms iBOT, which is a representative MIM method used for visual recognition tasks. Furthermore, we observed that the contrastive-learning-based method is not suitable for stronger vision transformer architectures, resulting in a significant accuracy drop. These findings demonstrate the superiority of our proposed method over existing self-supervised learning methods for hand estimation tasks.
Table 5. Comparisons with self-supervised methods. We train HandMIM with baselines under the same backbone and pretraining data. Results are evaluated on the FreiHAND [22] test set using the same regression head. PeCLR [18] shows an accuracy drop based on stronger vision transformers. Our HandMIM outperforms existing self-supervised methods by a large margin.
To evaluate the generalizability of our proposed method, we conducted a cross-data validation on 3D hand mesh estimation tasks. Specifically, we fine-tuned our model on the training set of FreiHAND and evaluated its performance on the test set of HO3D v2 and vice versa. Our results, presented in Table 6, demonstrate significant improvements compared to existing self-supervised methods such as PeCLR [18] or TempCLR [19], which indicates the superiority of our approach. Notably, our method even outperforms some recent fully supervised methods [44]; [23] when evaluated on the HO3D v2 test set.
Table 6. Cross-dataset analysis on HO3D and FreiHAND. Methods are trained on FreiHAND and tested on HO3D and vice versa.
We are curious about the effects of hand pose estimation after self-supervised pretraining and visualize the results before and after pre-train in Figure 8. The findings demonstrate that HandMIM pretraining enhances the resilience of 3D hand mesh estimation tasks, indicating the beneficial effects of pretraining. Specifically, the results highlight the positive influence of pretraining on the robustness of hand pose estimation. More visualization examples are shown in the supplementary document.
Figure 8. Visualizations of HandMIM pretraining. Images in the left column are from the FreiHAND [22] test set, while the images in the right column are from HO3D v2 [23]. Using ViT-Base as our backbone, we visualize the predicted mesh before (ViT-B) and after (+HandMIM) self-supervised training. We obtain more precise predictions after HandMIM pretraining.
To further explore the efficacy of the learned features, we employ a partial fine-tuning method based on the protocol proposed in [16]. We sequentially freeze the first several layers while fine-tuning the remaining transformer blocks. The results are presented in Figure 9, which indicates that when we freeze around half of the layers (i.e., 4 of 12), the HandMIM approach shows only a minor decrease in accuracy compared to mainstream masked image modeling methods. Moreover, when we freeze eight or more layers, the performance gap between our method and most fully supervised methods becomes more pronounced. These findings suggest that our approach can effectively learn multi-level hand representations via our multi-level learning approach.
Figure 9. Partial fine-tuning performance comparison between pre-trained weight from mainstream masked image modeling methods and our HandMIM with ViT-Small as the backbone. We use the FreiHAND [22] test set as a metric and adopt iBOT [15] as baselines of MIM methods. We gradually freeze different numbers of blocks to reveal the feature generalizability learned from pretraining.
The pose-aware
Table 7. Ablation studies. We perform ablations on the loss design of HandMIM. Specifically, we remove all three critical losses
To justify the scalability of HandMIM on unlabeled images, we conduct pretrain experiments with a certain proportion of the full dataset. Table 8 shows our evaluation results. We obtain better performance with more unlabeled data (
Figure 10. Scalability of HandMIM pretraining. We pre-trained the backbone ViT-S with two strategies: (i) ViT-S + ImageNet: training ViT-S in a supervised approach with labeled data on ImageNet. (ii) ViT-S + HandMIM: training ViT-S in the self-supervised approach described above with unlabeled data. Both models are connected with PyMAF [20] and fine-tuned for mesh estimation. The PAJPE metric is evaluated for both.
Furthermore, we evaluated the performance of HandMIM across different scales of parameters, specifically using vision transformer small (ViT-S), base (ViT-B), and large (ViT-L) configurations. The results, depicted in Figure 11, demonstrate two key insights: (i) the performance of HandMIM is enhanced with the increase in parameter size, and (ii) HandMIM consistently outperforms other methods when matched for parameter scale.
Figure 11. Performance–parameter trade-off of mainstream 3D hand mesh estimation methods on the FreiHAND [22] test set. We perform vertex-point-error after Procrustes alignment (lower is better). Our proposed HandMIM achieves better trade-offs in various model sizes under the standard ViT backbone.
HandMIM has demonstrated competitive performance across various datasets. Nevertheless, there are still situations where the model might struggle, as shown in Figure 12.
(1) Complex hand–object interactions. When hands are engaged in complex interactions with objects, the model must infer the occluded parts of the hand based on limited visual cues. Although HandMIM shows promise in these scenarios, there is room for improvement, especially when the interaction involves intricate movements or unusual poses that the model has not encountered during training.
(2) Extreme occlusions. Despite advancements in handling occlusions, extremely occluded hands—where large portions of the hand are hidden or covered by other fingers—remain challenging. In these cases, the model may lack sufficient visible information to accurately reconstruct the hand mesh, leading to increased prediction errors.
(3) Dataset variability. The effectiveness of HandMIM depends on the diversity and quality of the pretraining datasets. If the datasets used for pretraining do not adequately cover certain types of hand poses or backgrounds, the model’s ability to generalize to unseen data may be compromised.
Figure 12. The figure demonstrates some common failure cases. (A) Complex hand–object interactions. (B) Extreme occlusion between fingers.
Accordingly, while HandMIM excels in many aspects of 3D hand mesh estimation, it faces challenges related to the quality of pseudo-keypoint generation and potential failures in extreme occlusion scenarios. Addressing these limitations will be essential for further enhancing the robustness and applicability of our model.
In this study, we have introduced HandMIM, a novel self-supervised pretraining strategy specifically designed for 3D hand mesh regression from monocular RGB images. Our approach leverages masked image modeling in conjunction with a multi-granularity strategy and pseudo-keypoint alignment within a teacher–student framework, utilizing self-distillation to learn comprehensive representations. By integrating these components, HandMIM achieves significant improvements over traditional supervised methods, reducing errors by approximately 40%–50%. This underscores the effectiveness of our method in requiring less reliance on labeled training data. The experiments conducted across various datasets highlight HandMIM’s robustness and adaptability, particularly under challenging conditions such as severe occlusions. Notably, it achieved an 8.00 mm PAVPE on the HO3Dv2 test set, outperforming many specialized architectures. Furthermore, scalability tests on unlabeled images demonstrated that increasing the dataset proportion from 25% to 100% progressively decreased the PAVPE from 7.1 mm to 6.57 mm, indicating improved performance with more data. Additionally, evaluating HandMIM using different parameter scales revealed that its performance is enhanced with larger models, and it consistently outperforms other methods when matched for parameter scale. These results suggest that HandMIM not only benefits from deeper networks but also maintains superior performance relative to alternative approaches at similar model sizes. For future work, we propose several directions:
• Exploring the integration of temporal information. Current research focuses on single-image-based estimation. Expanding HandMIM to incorporate sequential video frames could enhance pose estimation accuracy and stability.
• Addressing dual-hand interactions. The current scope is limited to single-hand poses. Future efforts should consider extending the model to handle scenarios involving two interacting hands.
• Generalizing to related tasks. Investigating how the principles behind HandMIM can be applied to other human-centric regression and estimation tasks could broaden its impact.
Overall, HandMIM represents a significant advancement in self-supervised learning for 3D hand pose estimation, setting a new benchmark and opening avenues for further exploration.
The original contributions presented in the study are included in the article/supplementary material; further inquiries can be directed to the corresponding author.
YL: Writing–original draft, Funding acquisition, Formal analysis, Investigation. CW: Writing–original draft, Conceptualization, Data curation, Methodology. HW: Project administration, Supervision, Writing–review and editing.
The author(s) declare that financial support was received for the research, authorship, and/or publication of this article. This work was supported by Science and Technology Research Project of Jiangxi Provincial Department of Education (No. GJJ2203419).
The authors declare that the research was conducted in the absence of any commercial or financial relationships that could be construed as a potential conflict of interest.
The author(s) declare that no Generative AI was used in the creation of this manuscript.
All claims expressed in this article are solely those of the authors and do not necessarily represent those of their affiliated organizations, or those of the publisher, the editors, and the reviewers. Any product that may be evaluated in this article, or claim that may be made by its manufacturer, is not guaranteed or endorsed by the publisher.
1. Lin K, Wang L, Liu Z. Mesh graphormer. IEEE International Conference on Computer Vision ICCV (2021). p. 12939–48.
2. Hampali S, Sarkar SD, Rad M, Lepetit V. Keypoint transformer: solving joint identification in challenging hands and object interactions for accurate 3d pose estimation. In: Proceedings of the IEEE/CVF conference on computer vision and pattern recognition (2022). p. 11090–100.
3. Cai G, Zheng X, Guo J, Gao W. Real-time identification of borehole rescue environment situation in underground disaster areas based on multi-source heterogeneous data fusion. Saf Sci (2025) 181:106690. doi:10.1016/j.ssci.2024.106690
4. Jin W, Tian X, Shi B, Zhao B, Duan H, Wu H. Enhanced uav pursuit-evasion using boids modelling: a synergistic integration of bird swarm intelligence and drl. Comput Mater & Continua (2024) 80:3523–53. doi:10.32604/cmc.2024.055125
5. Hu Z, Qi W, Ding K, Liu G, Zhao Y. An adaptive lighting indoor vslam with limited on-device resources. IEEE Internet Things J (2024) 11:28863–75. doi:10.1109/JIOT.2024.3406816
6. Chen J, Li T, Zhang Y, You T, Lu Y, Tiwari P, et al. Global-and-local attention-based reinforcement learning for cooperative behaviour control of multiple uavs. IEEE Trans Vehicular Technology (2024) 73:4194–206. doi:10.1109/TVT.2023.3327571
7. Chen J, Du C, Zhang Y, Han P, Wei W. A clustering-based coverage path planning method for autonomous heterogeneous uavs. IEEE Trans Intell Transportation Syst (2021) 23:25546–56. doi:10.1109/tits.2021.3066240
8. Zhu P, Pan Z, Liu Y, Tian J, Tang K, Wang Z. A general black-box adversarial attack on graph-based fake news detectors. In: International joint conference on artificial intelligence (IJACI 2024) (2024).
9. Zhu P, Fan Z, Guo S, Tang K, Li X. Improving adversarial transferability through hybrid augmentation. Comput & Security (2024) 139:103674. doi:10.1016/j.cose.2023.103674
10. Guo S, Li X, Zhu P, Mu Z. Ads-detector: an attention-based dual stream adversarial example detection method. Knowledge-Based Syst (2023) 265:110388. doi:10.1016/j.knosys.2023.110388
11. Kulon D, Guler RA, Kokkinos I, Bronstein MM, Zafeiriou S. Weakly-supervised mesh-convolutional hand reconstruction in the wild. In: Proceedings of the IEEE/CVF conference on computer vision and pattern recognition (2020). p. 4990–5000.
12. Spurr A, Iqbal U, Molchanov P, Hilliges O, Kautz J. Weakly supervised 3d hand pose estimation via biomechanical constraints. In: Proceedings of the European conference on computer vision. Springer (2020). p. 211–28.
13. Lugaresi C, Tang J, Nash H, McClanahan C, Uboweja E, Hays M, et al. (2019). Mediapipe: a framework for building perception pipelines. arXiv preprint arXiv:1906.08172
14. Kolesnikov A, Dosovitskiy A, Weissenborn D, Heigold G, Uszkoreit J, Beyer L, et al. (2021). An image is worth 16x16 words: transformers for image recognition at scale
15. Zhou J, Wei C, Wang H, Shen W, Xie C, Yuille A, et al. ibot: image bert pre-training with online tokenizer. In: International conference on learning representations (ICLR) (2022).
16. He K, Chen X, Xie S, Li Y, Dollár P, Girshick R. Masked autoencoders are scalable vision learners. In: Proceedings of the IEEE/CVF conference on computer vision and pattern recognition (2022). p. 16000–9.
17. Oord Avd, Li Y, Vinyals O. Representation learning with contrastive predictive coding. arXiv preprint arXiv:1807.03748 (2018). doi:10.48550/arXiv.1807.03748
18. Spurr A, Dahiya A, Wang X, Zhang X, Hilliges O. Self-supervised 3d hand pose estimation from monocular rgb via contrastive learning. In: IEEE international conference on computer vision (ICCV) (2021). p. 11230–9.
19. Ziani A, Fan Z, Kocabas M, Christen S, Hilliges O. Tempclr: reconstructing hands via time-coherent contrastive learning. In: International conference on 3D vision (3DV) (2022).
20. Zhang H, Tian Y, Zhou X, Ouyang W, Liu Y, Wang L, et al. Pymaf: 3d human pose and shape regression with pyramidal mesh alignment feedback loop. In: 2021 IEEE/CVF international conference on computer vision (ICCV) (2021). p. 11426–36. doi:10.1109/ICCV48922.2021.01125
21. Romero J, Tzionas D, Black MJ. Embodied hands: modeling and capturing hands and bodies together. ACM Trans Graph (2017) 36:1–17. doi:10.1145/3130800.3130883
22. Zimmermann C, Ceylan D, Yang J, Russell B, Argus M, Brox T. Freihand: a dataset for markerless capture of hand pose and shape from single rgb images. IEEE International Conference on Computer Vision ICCV (2019). p. 813–22.
23. Hampali S, Rad M, Oberweger M, Lepetit V. Honnotate: a method for 3d annotation of hand and object poses. In: Proceedings of the IEEE/CVF conference on computer vision and pattern recognition (2020). p. 3196–206.
24. Moon G, Lee KM. I2l-meshnet: image-to-lixel prediction network for accurate 3d human pose and mesh estimation from a single rgb image. In: Proceedings of the European conference on computer vision (2020).
25. Xie P, Xu W, Tang T, Yu Z, Lu C. Ms-mano: enabling hand pose tracking with biomechanical constraints. In: Proceedings of the IEEE/CVF conference on computer vision and pattern recognition (2024). p. 2382–92.
26. Jiang Z, Rahmani H, Black S, Williams BM. A probabilistic attention model with occlusion-aware texture regression for 3d hand reconstruction from a single rgb image. In: Proceedings of the IEEE/CVF conference on computer vision and pattern recognition (2023). p. 758–67.
27. Vasu PKA, Gabriel J, Zhu J, Tuzel O, Ranjan A. Fastvit: a fast hybrid vision transformer using structural reparameterization. In: Proceedings of the IEEE/CVF international conference on computer vision (2023). p. 5785–95.
28. Wang C, Zhu F, Wen S. Memahand: exploiting mesh-mano interaction for single image two-hand reconstruction. In: Proceedings of the IEEE/CVF conference on computer vision and pattern recognition (2023). p. 564–73.
29. Park J, Oh Y, Moon G, Choi H, Lee KM. Handoccnet: occlusion-robust 3d hand mesh estimation network. In: Proceedings of the IEEE/CVF conference on computer vision and pattern recognition (2022). p. 14682–92.
30. Chen X, Liu Y, Dong Y, Zhang X, Ma C, Xiong Y, et al. Mobrecon: mobile-friendly hand mesh reconstruction from monocular image. In: Proceedings of the IEEE/CVF conference on computer vision and pattern recognition (2022). p. 20544–54.
31. Pavlakos G, Shan D, Radosavovic I, Kanazawa A, Fouhey D, Malik J. Reconstructing hands in 3D with transformers. In: Proceedings of the IEEE/CVF conference on computer vision and pattern recognition (2024).
32. Zimmermann C, Argus M, Brox T. Contrastive representation learning for hand shape estimation. In: DAGM German conference on pattern recognition. Springer (2021). p. 250–64.
33. Bao H, Dong L, Piao S, Wei F. BEiT: BERT pre-training of image transformers. In: International conference on learning representations (2022).
34. He K, Fan H, Wu Y, Xie S, Girshick R. Momentum contrast for unsupervised visual representation learning. In: Proceedings of the IEEE/CVF conference on computer vision and pattern recognition (2020). p. 9729–38.
35. Grill J-B, Strub F, Altché F, Tallec C, Richemond P, Buchatskaya E, et al. Bootstrap your own latent-a new approach to self-supervised learning. 34th Conference on Neural Information Processing Systems (NeurIPS 2020) (2020) 33:21271–84. doi:10.5555/3495724.3496201
36. Caron M, Touvron H, Misra I, Jégou H, Mairal J, Bojanowski P, et al. Emerging properties in self-supervised vision transformers. IEEE International Conference on Computer Vision ICCV (2021). p. 9650–60.
37. Jin S, Xu L, Xu J, Wang C, Liu W, Qian C, et al. Whole-body human pose estimation in the wild. In: Proceedings of the European conference on computer vision. Springer (2020). p. 196–214.
38. Xiang Y, Schmidt T, Narayanan V, Fox D (2018). Posecnn: a convolutional neural network for 6d object pose estimation in cluttered scenes
39. Chen P, Chen Y, Yang D, Wu F, Li Q, Xia Q, et al. I2uv-handnet: image-to-uv prediction network for accurate and high-fidelity 3d hand mesh modeling. In: IEEE international conference on computer vision (ICCV) (2021). p. 12929–38.
40. Zhang X, Huang H, Tan J, Xu H, Yang C, Peng G, et al. Hand image understanding via deep multi-task learning. In: IEEE international conference on computer vision (ICCV) (2021). p. 11281–92.
41. Tang X, Wang T, Fu C-W. Towards accurate alignment in real-time 3d hand-mesh reconstruction. In: IEEE international conference on computer vision (ICCV) (2021). p. 11698–707.
42. Lim GM, Jatesiktat P, Ang WT. Mobilehand: real-time 3d hand shape and pose estimation from color image. In: International conference on neural information processing. Springer (2020). p. 450–9.
43. Liu S, Jiang H, Xu J, Liu S, Wang X. Semi-supervised 3d hand-object poses estimation with interactions in time. In: Proceedings of the IEEE/CVF conference on computer vision and pattern recognition (2021).
44. Hasson Y, Varol G, Tzionas D, Kalevatykh I, Black MJ, Laptev I, et al. Learning joint reconstruction of hands and manipulated objects. In: Proceedings of the IEEE/CVF conference on computer vision and pattern recognition (United States: Computer Vision Foundation (CVF) and IEEE) (2019). p. 11807–16.
Keywords: 3D hand mesh estimation, multi-granularity representation, self-supervised learning, masked image modeling, vision transformer
Citation: Li Y, Wang C and Wang H (2025) Toward accurate hand mesh estimation via masked image modeling. Front. Phys. 12:1515842. doi: 10.3389/fphy.2024.1515842
Received: 23 October 2024; Accepted: 18 December 2024;
Published: 29 January 2025.
Edited by:
Peican Zhu, Northwestern Polytechnical University, ChinaReviewed by:
Anas Bilal, Hainan Normal University, ChinaCopyright © 2025 Li, Wang and Wang. This is an open-access article distributed under the terms of the Creative Commons Attribution License (CC BY). The use, distribution or reproduction in other forums is permitted, provided the original author(s) and the copyright owner(s) are credited and that the original publication in this journal is cited, in accordance with accepted academic practice. No use, distribution or reproduction is permitted which does not comply with these terms.
*Correspondence: Huan Wang, d2FuZ2h1YW42QGVtYWlsLnN6dS5lZHUuY24=
Disclaimer: All claims expressed in this article are solely those of the authors and do not necessarily represent those of their affiliated organizations, or those of the publisher, the editors and the reviewers. Any product that may be evaluated in this article or claim that may be made by its manufacturer is not guaranteed or endorsed by the publisher.
Research integrity at Frontiers
Learn more about the work of our research integrity team to safeguard the quality of each article we publish.