- 1School of Management, Beijing Institute of Technology, Beijing, China
- 2School of Economics, Beijing Institute of Technology, Beijing, China
- 3School of Public Policy and Management, University of Chinese Academy of Sciences, Beijing, China
- 4Cyber Finance Department, Postal Savings Bank of China, Beijing, China
The system of scientific innovation can be characterized as a complex, multi-layered network of actors, their products and knowledge elements. Despite the progress that has been made, a more comprehensive understanding of the interactions and dynamics of this multi-layered network remains a significant challenge. This paper constructs a multilayer longitudinal network to abstract institutions, products and ideas of the scientific system, then identifies patterns and elucidates the mechanism through which actor collaboration and their knowledge transmission influence the innovation performance and network dynamics. Aside from fostering a collaborative network of institutions via co-authorship, fine-grained knowledge elements are extracted using KeyBERT from academic papers to build knowledge network layer. Empirical studies demonstrate that actor collaboration and their unique and diverse ideas have a positive impact on the performance of the research products. This paper also presents empirical evidence that the embeddedness of the actors, their ideas and features of their research products influence the network dynamics. This study gains a deeper understanding of the driving factors that impact the interactions and dynamics of the multi-layered scientific networks.
1 Introduction
The system of scientific development and innovation can be described as a complex, self-organizing, and constantly evolving multi-layered network [1]. The rapid accumulation of digital data on the process, as well as the results of scientific innovation, have made it possible to model the overall structure of this dynamic network system [2]. Scientists and institutions draw on knowledge resources from collaboration networks, feeding back into the new creation, recombination, and transmission of knowledge elements, giving rise to new theories, methodologies, and technologies, sparking widespread interest in exploring network dynamics in this system that involves social, managerial and economic values [3–5].
Existing research on the patterns and dynamics of the scientific system modeling started with single-layer complex networks. As the scientific innovation landscape gradually shifts from individual to collaborative activities, research on the complex system began to attract extensive attention from the academic community to a series of topics such as the properties and structure of collaboration networks [6–8], collaboration patterns [9, 10] and the formation and evolution mechanism of collaboration network [11, 12]. These networks mainly built from the co-author articles, co-applicant patents or jointly undertaken research projects reflecting the formal or informal collaborations among individuals, organizations or even countries, are commonly used to reveal patterns of collaboration and research behaviors [13–15].
Further research then illustrated that the scientific innovation system may exist in a multiplex structure state, where its elements are simultaneously embedded in both collaboration networks and knowledge networks [16, 17]. The multi-layered network has been proved to be have an “internetwork effect”, meaning that changes in one network may affect the utility of the other [18]. Knowledge networks in the existing studies are mainly created via co-occurrences in the substance or core elements of innovation products, including keywords of scientific papers [19], IPC codes of patents [20], topics [21], MeSH terms [22], hashtags [23] and so on, reflect the research theme, knowledge flow and combinatorial history in research collaboration.
Although multi-layered network frameworks have been proposed for describing scientific innovation, existing research has not yet developed a comprehensive model to measure the nodes and links within the multiplex structure, including actors, innovative products, and knowledge elements, nor has it fully understood the dynamics mechanisms. Research on complex networks of scientific innovation has been limited to either collaboration networks or knowledge networks, providing only partial views of the systematic structure, and inadequate understanding of the network dynamics. Some prior studies have explored the impact of the properties of knowledge owned by individuals or institutions on the collaboration dynamics [24, 25], which are generally discussed in a macro perspective that also includes economic, geographical, cultural and other factors. Despite the progress that has been made, a more comprehensive understanding of the interaction of the multi-layered networks of scientific innovation, and their network dynamics, remains a significant challenge.
In response to these challenges, we consider the interactions between actors, their innovative products and knowledge element exchanges to reveal how collaboration and knowledge transmission influence the innovation performance and the network dynamics of scientific innovation. This paper constructs multilayer longitudinal networks to abstract institutions, products and ideas of the scientific system, and then elucidate the interaction mechanism among different layers by answering two questions: what features from collaboration and knowledge network affect the innovation product layer and how the embeddedness of the actors, their ideas and research outcome influence the network dynamics. From empirical perspective, H1 Connect academic articles recommendation database is used to perform a case study in the field of protein structure research. We collected scientific papers published from year 2014 to year 2022, which have been recommended by researchers on H1 Connect with associated scores and opinion tags. To further enhance this dataset, we integrated information from the bibliographic database Web of Science. Fine-granular knowledge elements are then extracted using KeyBERT from scientific papers to build knowledge networks. These networks serve as the foundation for identifying patterns of knowledge combination. We finally employ the stochastic actor-oriented models to uncover the underlying mechanisms governing network evolution in the field of protein structure. This comprehensive study gains a deeper understanding of the driving factors that impact the interactions and dynamics of the multi-layered scientific networks.
2 Literature review
2.1 Multi-layered networks for scientific innovation system
The scientific innovation system can be abstracted as an evolving complex system of diverse basic units of science that are dynamically linked and coupled. The key research question is how to model and simulate the system. The large-scale scientific publication datasets have created new opportunities to model and explore this system. With the development of complex network theory and methodology, and also their application in the science of science, the modelling of scientific innovation system has gradually shifted from single layer to multi-layers networks. The collaboration and knowledge networks have always attracted the most attention from scholars, as collaboration reveals innovative behavior, and knowledge reflects results. For example, Guan and Liu [16] have constructed the collaboration networks based on joint assignees of patents and knowledge networks based on the co-application of IPC codes in each patent, and accordingly studied the impact of organizations’ doubly network embeddedness on innovation. Graf and Kalthaus [26] have distinguished the research network into three levels: co-authorship at the researcher level, the collaboration between organizations, and international collaboration between countries. Luo and Zhang [27] have constituted a multi-network includes the collaboration network of R&D organizations, the collaboration network of R&D employees and the knowledge network. Ba, Mao [28] have investigated how city-level collaboration and knowledge networks influence innovation in the energy conservation field.
A rich body of literature concerning the multi-layered networks of the scientific innovation system has revolved around the impact of network embeddedness on innovation. Existing research have long recognized that the collaboration and knowledge recombination could affect innovation performance [29–31]. While network embeddedness, especially multi-network embeddedness offers a unique and valuable lens to gain deeper insights into innovation. Network embeddedness reflects the position of the actor and the connection to other actors in the network [32], which determines the ability to gather, integrate and allocate resources. Gonzalez-Brambila, Veloso [33] have examined how embeddedness in the collaboration network affects the research output and impact of scientists. Zhang and Luo [34] have explored the relationship between innovation and the knowledge network capital (i.e., knowledge combinatorial capacity, knowledge stocks, technological distance and network efficiency).
In summary, most existing literature focus on dual layers network to model and analyze the mechanism of the scientific innovation system. However, prior literature has demonstrated that the scientific innovation system is the evolving set of actors, artifacts, and activities (relations) [35]. Drawing on this, multi-layered networks cover innovation entities (a publication of actors), innovative products (artifacts), knowledge elements (content of artifacts) and the relations among them need to be constructed to better abstract the system. Exploration under this framework warrants further research as well.
2.2 Network dynamics on scientific collaboration
Scientific collaboration forms the fundamental nexus of sharing and connection among actors, which gradually evolves into a collaboration network as the number of entities and connections increase. The majority of existing studies focus on investigating static properties of collaboration networks, such as network structure [36–38] and tie configuration [39–41]. However, the nature of scientific collaboration is far from static, with innovative entities constantly establishing and discontinuing partnerships [42]. An increasing number of scholars start to adopt a dynamic perspective to investigate network generation and evolution. Many statistical methods have been applied to network dynamics analysis, such as stochastic actor-oriented models (SAOMs), exponential random graph models (ERGMs), multiple regression quadratic assignment programs (MRQAPs) and so on. Ma, Yang [11] have used the ERGMs to investigate the formation mechanism of big data technology collaboration networks. Fronzetti Colladon, Grippa [43] have applied SAOMs to investigate the dynamics of knowledge sharing in healthcare and explored factors that are likely to influence the evolution of idea sharing and advice seeking. Aalbers and Ma [44] have examined the influence of organizational relationships complexities to a firms’ technological entry and exit through SAOMs. Empirical analysis on various scales have proved the feasibility and rationality of applying statistical methods like SAOMs to the analysis of the internal mechanism of network generation and evolution, through which the understanding of successful scientific collaborations can be further improved.
In the context of scientific innovation system, the dynamic coupling of units of the actors, innovative products and also knowledge elements affect the ongoing formation and breaking-up of ties in the collaboration network. Brennecke and Rank [45] have proved that different structural features of the firm’s knowledge stock shape the transfer of advice among inventors. Parreira, Machado [24] have found that similar scientific structure could affect the international collaboration. Li, Zhang [46] have found that technological proximity is one of the key factors that promote international green technological collaboration. Meanwhile, the actors’ performance is also closely associated with their scientific collaboration. Publishing high-quality papers could increase the academic reputation of the organization and attract more attention from academia and industry, which will lead to more academic opportunities and attract more partners [25, 47]. However, innovation performance is rarely included in the research framework of collaboration network dynamics, which may focus more on geographical, economic, social, cultural, cognitive and other macro factors.
2.3 Knowledge elements extraction and representations
The knowledge base of an innovative actor is widely regarded as an aggregation of its knowledge elements, while the article keywords or topics and predefined categorizations, such as IPC codes have been valid proxies for knowledge elements [28, 48, 49]. Although these identifiers are intuitive and clear, it is often difficult to grasp the rich context and semantic information of the text when deeply analyzing and understanding the micro-knowledge structure at the institutional or individual level [17]. Based on this, how to effectively extract knowledge elements from scientific texts becomes the key to further build knowledge networks.
Methods for extracting terms from unstructured text can be divided into four categories: statistical methods, clustering-based methods, graph-based methods, and deep learning methods. Statistical learning methods use determined mathematical functions to identify words with abnormal frequencies and generally do not require any information other than word frequency statistics from corpora. The aim of the clustering methods is to cluster the candidate terms, and then select the most representative terms from each cluster. The graph based methods represent the document into a graph, and use the graph ranking method to identify key terms [50]. Knowledge embedding methods based on deep learning have become a research focus in recent years for knowledge extraction from unstructured scientific texts [51]. This kind of method can make full use of the semantic and contextual information of words and phrases, and realize the accurate localization of knowledge elements in text. The unsupervised method KeyBERT uses BERT embedding to extract keywords that best represent the underlying text. Due to its focus on relevancy in sentences, contextual information in scientific texts can be taken into account when extracting knowledge elements, and the extraction results have been evidenced to be superior to traditional methods in terms of the similarity of keywords specified by the author [50].
3 Methodology
In this study, we abstract and model the complex scientific innovation system with innovative entities (actors), innovative products (artifacts) and knowledge elements, and the relations among these nodes. As illustrated in Figure 1, the multi-layered network consists of three layers of collaboration network, knowledge network and innovative products. The collaboration network is established through co-publication relationships among institutions. Here we select institutions, a population of individual authors, as the agent of actors, as this scale better captures the actors’ ownership of knowledge. Scientific papers, as the main form of innovative products, constitute the innovative product layer. The knowledge network is constructed based on the co-occurrence of knowledge elements extracted from the papers. As shown in Figure 1, each institution may produce a certain number of papers, and these research outcomes consist of knowledge elements that constitute institutions’ knowledge base. The knowledge network and collaboration network are interconnected through the product layer.
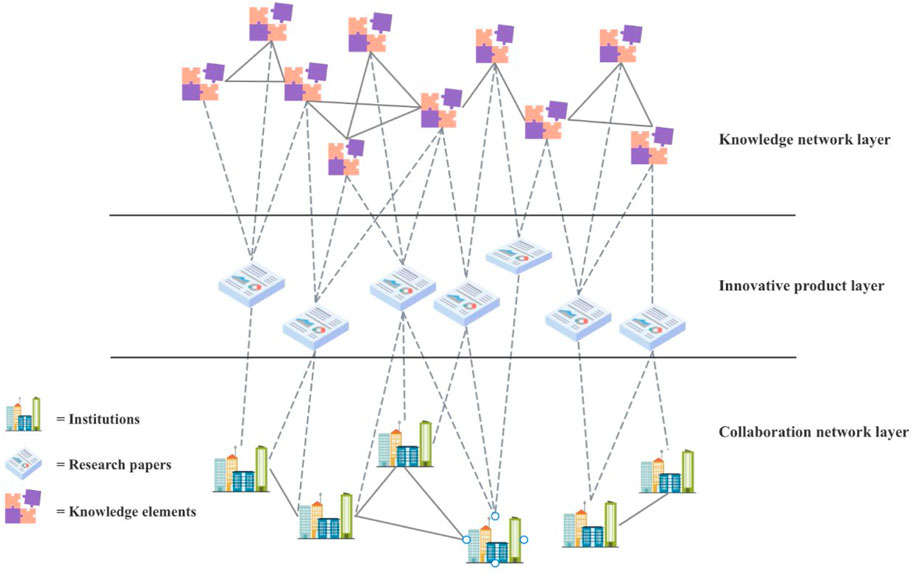
Figure 1. Framework of the multi-layered networks of modeling interactions of institutions, research papers and knowledge elements.
Based on the abstract scientific innovation system, this study introduces a model of multi-layered network to explore the interaction and dynamics of scientific innovation. The methodology is comprised of four parts: (1) the multi-layered network construction, including the process of knowledge element extraction and construction of the knowledge network and collaboration network in which the institutions embedded; (2) the measurement of the actors’ and their knowledge elements’ embeddedness characteristics in the multi-layered network; (3) the investigation about how network embeddedness characteristics of institutions and their knowledge affect performance of innovation products; (4) the network dynamics analysis of the actors in the multi-layered network using SAOMs.
3.1 Multi-layered network construction
The knowledge elements of an institution, is the core competitive resources of innovation activities [52]. It has been proven that knowledge plays an important role in the dynamic changes of the cooperative relationship [25, 53]. In this study, we extract fine-granular knowledge elements using KeyBERT to build knowledge networks. These networks serve as the foundation for capturing patterns of knowledge combination, through which the deep structural and relational features can be intuitively represented and explored.
Knowledge elements refer to the facts, theories or methods of a certain topic in scientific or technical research, which are commonly used to represent the dimensions of knowledge areas [19, 54]. To capture rich information from scientific papers, we use KeyBERT, an unsupervised keyword extraction algorithm, to extract the set of terms that are most semantically representative to the content of the paper. KeyBERT algorithm relies on BERT pre-trained model to generate vectors of documents and candidate terms, and extracts terms by comparing the cosine similarity between them [55], which enables to select high-quality terms in scientific texts [50]. Since KeyBERT supports many embedding models, we choose SciBERT [56], which trained on scientific text, to obtain vector representation with state-of-the-art performance. Figure 2 shows the process of knowledge element extraction using KeyBERT, and the specific steps are as follows:
Step I: Creating the list of candidate terms: Extract N-grams (
Step II: Word embedding: Apply SciBERT model to generate embedded representations of the document and candidate words in the same vector space.
Step III: Selecting the most representative terms of a document: Calculate the cosine similarity between the word embedding vectors and document embedding vector to extract the top N terms with the highest similarity to best describe the document content. Considering that documents of different lengths may contain different amounts of knowledge, the value of N is determined according to the length of the document. N is set as 5% of the document length.
This study constructs the knowledge network based on their co-occurrence relationships in scientific papers. Knowledge elements are linked through the combination process of scientific innovation [57], which forms the knowledge networks over time [19, 58]. Then following the prior studies [17, 27, 59], institutions are extracted from the datasets as the actors of generating innovation products, research papers; and ties are created based on their co-publication relations to form the collaboration network. Institutions and the knowledge elements they possess are linked through the jointly published papers, which form the innovation product layer.
3.2 Characteristics of the multi-layered network
The aim of our study is to investigate how the embeddedness of the actors and their knowledge transmission in the scientific system influence the innovation performance and network dynamics. This requires capturing the characteristics of institutions and their knowledge embedded in different layers of the multi-layered network. Indicators at the knowledge network, collaboration network and innovative product layer are described in Table 1 respectively.
This study measures the knowledge transmission and recombination features using four indicators, including diversity, uniqueness, combinatorial capability and knowledge proximity. The knowledge diversity and uniqueness stands for the variety and scarcity of the knowledge devoted to innovation activities [45]. The combinatorial capability relates to the position of an institution’s knowledge elements relative to other elements [60], indicating the capability of accessing new information in the transmission [61]. In the calculation, the degree centrality of knowledge represents the feasibility and desirability of combination with other knowledge elements [27], while structural holes in the network implies non-redundant combination opportunities and further inventive capacity [16]. Knowledge proximity refers to the similarity of knowledge base between different institutions. Higher knowledge proximity reduces adverse selection risk caused by information asymmetry problems in the partner selection [53, 62]. In this study, we apply the doc2vec algorithm to generate vectors and then calculate cosine similarity between knowledge elements to measure knowledge proximity. In the collaboration network, being in a central position in the collaboration network allows institutions to access information and resources more effectively [54]. Due to the preferential attachment mechanism [3, 63], we apply the degree centrality to evaluate the direct partners of an institution.
The innovation performance of an institution is measured by the level of its innovative products. High-quality papers improve the academic reputation and visibility of the institutions, thus attracting more collaborators. In this study, we estimate the innovation performance of institutions using the H1 Connect innovation scores of their published papers. These scores are provided by senior researchers within the H1 Connect database who contribute their expertise by reading, reviewing, and recommending research papers on the platform.
3.3 Regression model
The recombination and transmission of existing knowledge are essential for institutions to achieve outstanding innovative output [64, 65]. From a network perspective, features from collaboration and knowledge network may affect the innovation product layer. Accordingly, we perform regression analysis of innovation performance and knowledge and collaboration characteristics.
As the innovation system is evolving, we model the evolution of collaboration networks and knowledge networks into different stages according to the publication year of the research papers. In regression analysis, these stages are seen as multiple time windows. The multi-layered network is constructed in each time window. All variables described in Section 3.2 have been calculated at the actor level and normalized respectively1. We then apply ordinary least squares (OLS) model with natural logarithm transformation of the explained variables for our estimation, the regression function is shown in Equation 1 2.
Where
To isolate the effects of knowledge and collaboration features on innovation performance, additional control variables are considered in the analysis. One of such variables is the number of disciplines, computed by the number of unique WoS categories of papers published by the institution, which may affect the innovation performance. Besides, we measure the innovation input with the number of authors involved in papers published by the institution, which can serve as a proxy for the amount of human resources involved in the innovation activities [66]. For institution
Finally, we perform an additional analysis by using another indicator of innovation performance, the average number of citations, to provide a more comprehensive result. Citation are widely accepted as a measure of scientific impact and thus as a partial aspect of innovation performance. We calculate the additional indicator by dividing the total citations of papers published by institution
3.4 Modeling network dynamics
We then model the network dynamics from an actor-oriented perspective, using stochastic actor-oriented model (SAOM). Actor layer of the multi-layered network, i.e. the collaboration network, is composed of a set of cooperation relations that are not independent of each other, and their relational changes (e.g., presence/absence of ties) may be the result of the network structure characteristics among actors or dyads, which is the endogenous effect difficult to measure in traditional regression models [67, 68]. SAOM is a statistical approach for modeling the process of network change with longitudinal network data using econometric discrete choice models and dynamic Markov models, which is able to capture endogenous effects related to the network and effectively deal with multicollinearity problems through built-in model [67, 69], thus enables us to understand which factors and dynamics could influence actors’ collaboration from the network perspective.
SAOM is an actor-based simulation model where the change of network ties over time is driven by the actor’s choices in accordance with a set of goals/preferences [68]. These preferences are modeled as an “evaluation function” (a linear combination of parameters and local graph statistics) that the actor seeks to maximize, which is shown in Equation 2. The model incorporate parameters embedded in network structure that endogenously influence the probabilities of tie changes and parameters related to characteristics of actors that exogenously influence the tie formation or termination [70]. Actors in the model are viewed as making choices one-at-a-time in mini-steps to maximize the evaluation function and possible changes can occur across different time points in sequence. The actor-oriented formulation also offers an explicit lens to gain a direct interpretation of parameters in SAOM [69].
where
The objective of using SAOM is to investigate which attributes characterize and affect the dynamic evolution of collaboration networks. These attributes include the knowledge dimension characteristics of institutions, their innovation performance and position in collaboration network. Table 2 summarizes and explains the indicators of different types required by the SAOM in this study. We estimate SAOM using the RSiena library, available in R statistical software [71].
4 Empirical study
4.1 Data
Our empirical analysis is performed on scientific paper data in the area of protein structure. With the implementation of the Human Proteome Project as well as the application of artificial intelligence in protein structure prediction [72], the field of protein structure research is characterized by rapid knowledge growth, diverse science linkages and widely existing collaborations. Our analysis mainly uses data from two primary sources, namely, (1) scientific papers and their peer-review information from H1 Connect research articles recommendation database4and (2) bibliographic data of the scientific papers from Web of Science (WoS) database5. Our final sample consists of papers published between 2014 and 2022 in the field of protein structure recommended in the H1 Connect.
Since innovation activities require sufficient time investment, and the scientific collaboration could last for three to 5 years [73], we choose a three-year time window to compare the network evolution in different periods. We divide the dataset into three periods: 2014–2016, 2017–2019, and 2020–2022, and select institutions that have co-published papers with other institutions within at least two periods. We then clean and consolidate the institutions in the dataset to ensure the standardization and consistency of their names6 and then distinguish them into two categories—industrial organization and academic institution—the former is identified by the words “Ltd” (Limited),“Co” (Company) and so on contained in the name while the latter is identified by the words “University”, “Institution”, “School”, “College”, “Faculty” and so on [17]. Besides, since the keyword extraction in this study is based on abstract, papers missing abstract are deleted.
The innovation performance of institutions in this study is measured by the peer review scores in H1 Connect. The reviewers are experts who are global opinion leaders in the life sciences and medicine science. Peer experts give higher scores to papers that show outstanding innovation and importance, and existing research has proved that better recommendation scores are associated with higher performing papers [74].
4.2 Descriptive statistics
Figure 3 visualizes the collaboration networks in three periods, 2014–2016,2017–2019 and 2020–2022, respectively. Institutions are represented as nodes, whereas co-publishing relations are represented as ties. The descriptive network statistics are presented in Table 37. The number of institutions participating in the protein structure research and their ties increase over three periods. The average number of collaborators for each institution also increased from 4.056 to 6.678, while the average path length of the network decreases from 3.220 to 2.929. This phenomenon indicates the connections between institutions and the efficiency of information transmission have been enhanced in the collaboration network layer. In addition, these networks do not show an obvious change in their density from the first to the third period, which means that the number of realized linkages grows at a similar rate as the number of actors.
We then select and create co-occurrence networks of knowledge elements, as illustrated in Figure 4, where the node size represents the frequency of the terms. Our visualization reveals several fundamental research topics in the field of protein structure, such as “protein structure”, “structure prediction”, “protein interaction” and so on. Moreover, we also recognize the emergence of some hot topics, for example, in the third period, 2020–2022, “coronavirus sars” and “syndrome coronavirus” have received more attention and effort. Besides, these snapshots also show the tendency of increasing and strengthening knowledge linkages in the field of protein structure.
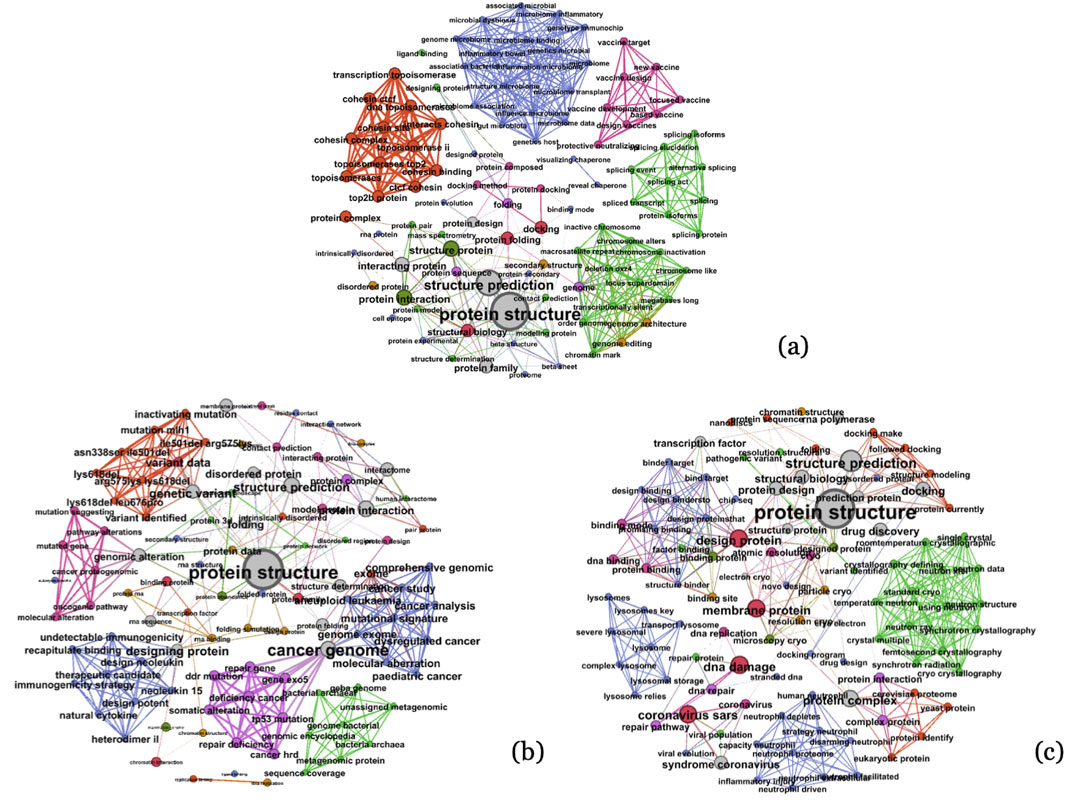
Figure 4. Co-occurrence network of the top 100 most frequently used keywords for the periods of: (A) 2014–2016; (B) 2017–2019; (C) 2020–2022.
We compute the number of unique knowledge elements for each period and find an increasing trend, as shown in Table 4. It describes the descriptive statistical results of variables in knowledge layer and innovation performance in the product layer. The mean and median values of knowledge uniqueness and knowledge diversity have increased distinctly over three periods, which can be interpreted as a hint of vibrant innovation and knowledge production activities in the field of protein structure. Moreover, institutions’ innovation performance, i.e. the average of innovation scores of papers published by institutions, also present an increasing trend in the mean and median values over the three periods, showing the vitality of high-quality research in the field of protein structure. However, the increase of standard deviation of innovation performance reveals that the difference of innovation capability between organizations expands over time.
4.3 Regression results
We present the results of estimating the regression models in Equation 1 in Table 5, testing what features from collaboration and knowledge network affect the innovation product performance. Model 1 reports the baseline OLS regression results, while Model 2 estimates the OLS regression with cluster-robust standard errors for robustness checks. In Model 1, the estimated coefficient for knowledge diversity is positive and significant (
For an additional analysis, we use average citation counts with natural logarithm transformation as an alternative measure of innovation performance, and report the regression results in Table 6. Model 3 presents the baseline OLS regression results, while Model 4 estimates the OLS regression with cluster-robust standard errors for robustness checks. Consist with regression results using peer-reviewed scores as measure of innovation performance, knowledge diversity, knowledge uniqueness and degree centrality in collaboration network have a significant and positive effect on citation performance in model 3 and model 4. Moreover, knowledge combinatorial capability shows a significant and positive influence on average citation counts, and knowledge proximity positively affects the citation counts. This suggests that the innovation output of institutions whose research is more closely aligned with others are more likely to gain citations and attentions. For the control variables, in Models 3 and 4, the number of disciplines has a negative impact on innovation performance in line with Model 1 and 2, but the coefficient for innovation input is positive and significant, indicating the number of scholars participating in innovative activities has expanded the visibility and impact of the research.
To summarize, it can be concluded that the knowledge diversity and uniqueness, the degree centrality in the collaboration network positively affect the institution’s innovative performance measured by both the peer-reviewed scores and citations. Besides, the knowledge combinatorial capability and proximity have a positive impact on citations.
4.4 SAOM results
The SAOM estimation results are presented in Table 7. Convergence is good for the model, since the overall maximum convergence ratio is less than 0.25 and all convergence t-ratios are below 0.1. The estimation of the rate parameters shows that the tendency of institutions to change collaboration relations amplifies over time, from approximately 8.5 opportunities per organization in Period 1 to around 17.5 opportunities per institution in Period 2. The coefficient of degree (density) is negative and significant (
We conduct robustness tests by altering the random seeds of the SAOM (Supplementary Table A1, A2). The random seed plays a crucial role in the iterations and parameter updates of the SAOM. If the model has converged to a stable state, different random seeds will produce similar results, indicating that the model outcomes are robust [71]. Changing random seeds in our model yields the same results as the initial findings in Table 7, reinforcing the robustness of our conclusions.
5 Discussion and conclusion
The scientific innovation is a system can be described as a multilayer network with complex structure, while more substantial efforts would be required to model the system and explore its dynamic mechanisms [2]. This paper constructs a multi-layered network to model scientific innovation system, in which the collaboration, innovation products and knowledge elements interact through the actors’ innovation activities. Building on this, we analyze how social and knowledge network embeddedness of actors, and their ideas affects the innovation performance and the network dynamics.
Our empirical analysis is based on a dataset of research articles with review scores in the research area of protein structure. The key findings of our study highlight the positive effects of knowledge uniqueness, knowledge diversity and the number of partners of institutions influence the innovation performance of the research outputs. We also find that, in addition to these factors, knowledge combinatorial capability and proximity have a positive impact on citations. Second, this paper also presents empirical evidence that from a dynamic perspective, the institution’s innovation performance positively affects the network dynamics, indicate that institutions with outstanding innovation products pursue establishing new collaboration and keep active in innovation activities. Besides, the knowledge diversity has a positive impact in the dynamics of the network, while the knowledge proximity plays a negative role, suggesting that actors tend to seek various diverse and distinct knowledge when choosing partners. These findings do not fully align with existing research [59, 75]. This may be related to datasets from different fields that were selected for empirical study. Emerging areas such as protein structure may have actors and links in the network are incentives to change, as reflected in our data. In this situation, institutions with more diverse and distinct knowledge are more inclined to collaborate with others to increase learning opportunities and thus achieve possible innovations. These reflect the heterogeneity of the underlying driving mechanisms of complex scientific systems and require further exploration and investigation.
Our results also indicate that some of the knowledge characteristics that promote innovation performance also serve as catalysts for network dynamics. Collaborations serves as the core driver of innovation [76, 77] and the organizations’ performance is relevant to the positions they occupy in the collaboration network [78]. The knowledge fusion fostered in the multi-layer innovation network positively affects the actors’ innovation output through collaboration, and the performance continue to influence the network dynamics, resulting in the creation of new ideas and findings. Our empirical results demonstrate that the position of an institution in the collaboration network fosters innovation, while their innovation performance reciprocally influences the evolution of scientific collaboration. This interplay elucidates the co-evolutionary process occurring between various layers within the scientific innovation system [4]. These results have direct implication for both innovation organizations and policymakers to encourage collaboration and incentivize innovation.
This study makes contributions as follows. It contributes to the existing literature on scientific innovation system by abstracting and modeling this system via a multi-layered complex network covering innovation entities (a population of actors), innovative products (artifacts), knowledge elements (content of artifacts) and the relations among them. Besides, this study enriches the methods of measuring characteristics of the multi-layered network. Based on this, we investigate the underlying factors that impact innovation performance and investigate the mechanism through which the actor collaboration and their knowledge transmission in the scientific system influence network dynamics. We provide a framework for future research to study the patterns and evolutionary mechanism of scientific innovation systems.
This study also has several limitations. First, we explore the network dynamics of the scientific innovation system from an actor-oriented perspective. However, economic and social factors may also play an important role in it, which is hard to capture in our data. Second, the underlying mechanisms governing the scientific innovation system are rather complex and heterogeneous. This study takes the publication data in the field of protein structure as an example to shed light on it. This is an inspiring and meaningful attempt, but the complex scientific innovation system should be further explored in different contexts in future work.
Data availability statement
The data analyzed in this study is subject to the following licenses/restrictions: The publication data and the corresponding review data are extracted from two databases, H1 connect and Web of Science. Readers can seek out and examine related publications and review data on their own following the instruction in the manuscript. Requests to access these datasets should be directed to YJ, MTgzMzIyNzM4MjhAMTYzLmNvbQ==.
Author contributions
YJ: Formal Analysis, Visualization, Writing–original draft. HC: Conceptualization, Funding acquisition, Validation, Writing–review and editing. JL: Data curation, Formal Analysis, Software, Writing–review and editing. XuW: Funding acquisition, Writing–review and editing. RG: Funding acquisition, Writing–review and editing. XiW: Writing–review and editing.
Funding
The author(s) declare that financial support was received for the research, authorship, and/or publication of this article. This work was supported by the National Natural Science Foundation of China under Grant Nos. 72004009, J2324014, 72074020, and 72204245.
Conflict of interest
Author XW was employed by company Postal Savings Bank of China.
The remaining authors declare that the research was conducted in the absence of any commercial or financial relationships that could be construed as a potential conflict of interest.
The author(s) declared that they were an editorial board member of Frontiers, at the time of submission. This had no impact on the peer review process and the final decision.
Publisher’s note
All claims expressed in this article are solely those of the authors and do not necessarily represent those of their affiliated organizations, or those of the publisher, the editors and the reviewers. Any product that may be evaluated in this article, or claim that may be made by its manufacturer, is not guaranteed or endorsed by the publisher.
Supplementary material
The Supplementary Material for this article can be found online at: https://www.frontiersin.org/articles/10.3389/fphy.2024.1492731/full#supplementary-material.
Footnotes
1The knowledge proximity for regression model is calculated by
2Although many indicators are related to network density, the main dependent and independent variables in the regression are calculated from the institution’ perspective, hence we have not included the network density, as it is a fixed value.
31 for academic institutions or 2 for industrial organizations.
4Last accessed on 15 January 2024.
5The citation dataset downloaded on 1 October 2024.
6Due to the limitations of the data itself, “University of California System” is listed as a single organization, and majority of records from both Web of Science and H1 Connect do not differentiate between the universities within the system.
7The descriptive network statistics are calculated by Gephi.
References
1. Fortunato S, Bergstrom CT, Boerner K, Evans JA, Helbing D, Milojevic S, et al. Science of science. Science (2018) 359(6379). doi:10.1126/science.aao0185
2. Zeng A, Shen Z, Zhou J, Wu J, Fan Y, Wang Y, et al. The science of science: from the perspective of complex systems. Phys Rep (2017) 714-715:1–73. doi:10.1016/j.physrep.2017.10.001
3. Barabâsi A-L, Jeong H, Néda Z, Ravasz E, Schubert A, Vicsek T. Evolution of the social network of scientific collaborations. Physica A: Stat Mech its Appl (2002) 311(3-4):590–614. doi:10.1016/s0378-4371(02)00736-7
4. Zhang Y, Chen K. Network growth dynamics: the simultaneous interaction between network positions and research performance of collaborative organisations. Technovation (2022) 115:102538. doi:10.1016/j.technovation.2022.102538
5. Chen H, Jin Q, Wang X. How embeddedness affects the evolution of collaboration: the role of knowledge stock and social interactions. arXiv (2023). doi:10.48550/arXiv.2311.05909
6. Abbasi A, Hossain L, Leydesdorff L. Betweenness centrality as a driver of preferential attachment in the evolution of research collaboration networks. J Informetrics (2012) 6(3):403–12. doi:10.1016/j.joi.2012.01.002
7. Dworkin JD, Shinohara RT, Bassett DS. The emergent integrated network structure of scientific research. PLOS ONE (2019) 14(4):e0216146. doi:10.1371/journal.pone.0216146
8. Pinto PE, Vallone A, Honores G. The structure of collaboration networks: findings from three decades of co-invention patents in Chile. J Informetrics (2019) 13(4):100984. doi:10.1016/j.joi.2019.100984
9. Fan L, Guo L, Wang X, Xu L, Liu F. Does the author’s collaboration mode lead to papers’ different citation impacts? An empirical analysis based on propensity score matching. J Informetrics (2022) 16(4):101350. doi:10.1016/j.joi.2022.101350
10. Liu J, Guo X, Xu S, Song Y, Ding K. A new interpretation of scientific collaboration patterns from the perspective of symbiosis: an investigation for long-term collaboration in publications. J Informetrics (2023) 17(1):101372. doi:10.1016/j.joi.2022.101372
11. Ma Y, Yang X, Qu S, Kong L. Research on the formation mechanism of big data technology cooperation networks: empirical evidence from China. Scientometrics (2022) 127(3):1273–94. doi:10.1007/s11192-022-04270-4
12. Gao J, Liu S, Li Z. Cooperative evolution of China's excellent innovative research groups from the perspective of innovation ecosystem: taking an “environmental biogeochemistry” research innovation group as a case study. Int J Environ Res Public Health (2021) 18(23):12584. doi:10.3390/ijerph182312584
13. Kong X, Shi Y, Wang W, Ma K, Wan L, Xia F. The evolution of turing award collaboration network: bibliometric-level and network-level metrics. IEEE Trans Comput Soc Syst (2019) 6:1318–28. doi:10.1109/tcss.2019.2950445
14. Noben I, Brouwer J, Deinum JF, Hofman WHA. The development of university teachers’ collaboration networks during a departmental professional development project. Teach Teach Educ (2022) 110:103579. doi:10.1016/j.tate.2021.103579
15. Grenno FE, dos Santos CZ, Schiavetti A, Profice CC. NGO scientific collaboration networks for marine conservation in the southern cone: a case study. Environ Sci and Pol (2023) 148:103554. doi:10.1016/j.envsci.2023.103554
16. Guan J, Liu N. Exploitative and exploratory innovations in knowledge network and collaboration network: a patent analysis in the technological field of nano-energy. Res Pol (2016) 45(1):97–112. doi:10.1016/j.respol.2015.08.002
17. Chen H, Jin Q, Wang X, Xiong F. Profiling academic-industrial collaborations in bibliometric-enhanced topic networks: a case study on digitalization research. Technol Forecast Soc Change (2022) 175:121402. doi:10.1016/j.techfore.2021.121402
18. Jiang H, Xia J, Cannella AA, Xiao T. Do ongoing networks block out new friends? Reconciling the embeddedness constraint dilemma on new alliance partner addition. Strateg Manage J (2018) 39(1):217–41. doi:10.1002/smj.2695
19. Guan J, Yan Y, Zhang JJ. The impact of collaboration and knowledge networks on citations. J Informetrics (2017) 11(2):407–22. doi:10.1016/j.joi.2017.02.007
20. Choi J, Yoon J. Measuring knowledge exploration distance at the patent level: application of network embedding and citation analysis. J Informetrics (2022) 16(2):101286. doi:10.1016/j.joi.2022.101286
21. Song B, Suh Y. Identifying convergence fields and technologies for industrial safety: LDA-based network analysis. Technol Forecast Soc Change (2019) 138:115–26. doi:10.1016/j.techfore.2018.08.013
22. Wang S, Ma Y, Mao J, Bai Y, Liang Z, Li G. Quantifying scientific breakthroughs by a novel disruption indicator based on knowledge entities. J Assoc Inf Sci Technol (2022) 74:150–67. doi:10.1002/asi.24719
23. Hellsten I, Leydesdorff L. Automated analysis of actor–topic networks on twitter: new approaches to the analysis of socio-semantic networks. J Assoc Inf Sci Technol (2020) 71(1):3–15. doi:10.1002/asi.24207
24. Parreira MR, Machado KB, Logares R, Diniz-Filho JAF, Nabout JC. The roles of geographic distance and socioeconomic factors on international collaboration among ecologists. Scientometrics (2017) 113(3):1539–50. doi:10.1007/s11192-017-2502-z
25. Wu L, Yi F, Bu Y, Lu W, Huang Y. Toward scientific collaboration: a cost-benefit perspective. Res Pol (2024) 53(2):104943. doi:10.1016/j.respol.2023.104943
26. Graf H, Kalthaus M. International research networks: determinants of country embeddedness. Res Pol (2018) 47(7):1198–214. doi:10.1016/j.respol.2018.04.001
27. Luo T, Zhang Z. Multi-network embeddedness and innovation performance of R&D employees. Scientometrics (2021) 126(9):8091–107. doi:10.1007/s11192-021-04106-7
28. Ba Z, Mao J, Ma Y, Liang Z. Exploring the effect of city-level collaboration and knowledge networks on innovation: evidence from energy conservation field. J Informetrics (2021) 15(3):101198. doi:10.1016/j.joi.2021.101198
29. Schumpeter J, Backhaus U. The theory of economic development. In: J Backhaus, editor. Joseph alois schumpeter: entrepreneurship, style and vision. Boston, MA: Springer US (2003) p. 61–116.
30. Uzzi B, Mukherjee S, Stringer M, Jones B. Atypical combinations and scientific impact. Science (2013) 342(6157):468–72. doi:10.1126/science.1240474
31. Adams J. Collaborations: the fourth age of research. Nature (2013) 497(7451):557–60. doi:10.1038/497557a
32. Granovetter M. Economic action and social structure: the problem of embeddedness. Am J Sociol (1985) 91(3):481–510. doi:10.1086/228311
33. Gonzalez-Brambila CN, Veloso FM, Krackhardt D. The impact of network embeddedness on research output. Res Pol (2013) 42(9):1555–67. doi:10.1016/j.respol.2013.07.008
34. Zhang Z, Luo T. Network capital, exploitative and exploratory innovations—from the perspective of network dynamics. Technol Forecast Soc Change (2020) 152:119910. doi:10.1016/j.techfore.2020.119910
35. Granstrand O, Holgersson M. Innovation ecosystems: a conceptual review and a new definition. Technovation (2020) 90-91:102098. doi:10.1016/j.technovation.2019.102098
36. Newman MEJ. Coauthorship networks and patterns of scientific collaboration. Proc Natl Acad Sci (2004) 101(Suppl. l_1):5200–5. doi:10.1073/pnas.0307545100
37. Badar K, Hite J, Ashraf N. Knowledge network centrality, formal rank and research performance: evidence for curvilinear and interaction effects. Scientometrics (2015) 105:1553–76. doi:10.1007/s11192-015-1652-0
38. Wang L, Li G, Ma Y, Yang L. Structure properties of collaboration network with tunable clustering. Inf Sci (2020) 506:37–50. doi:10.1016/j.ins.2019.08.002
39. Menger LM, Stallones L, Cross JE, Henry KL, Chen PY. Strengthening suicide prevention networks: interorganizational collaboration and tie strength. Psychosocial Intervention (2015) 24(3):155–65. doi:10.1016/j.psi.2015.07.005
40. Wang J. Knowledge creation in collaboration networks: effects of tie configuration. Res Pol (2016) 45(1):68–80. doi:10.1016/j.respol.2015.09.003
41. Moon H, Di Benedetto A, Kim SK. The effect of network tie position on a firm’s innovation performance. J Business Res (2022) 144:821–9. doi:10.1016/j.jbusres.2022.02.035
42. Petersen AM. Quantifying the impact of weak, strong, and super ties in scientific careers. Proc Natl Acad Sci (2015) 112(34):E4671–E80. doi:10.1073/pnas.1501444112
43. Fronzetti Colladon A, Grippa F, Broccatelli C, Mauren C, McKinsey S, Kattan J, et al. Boosting advice and knowledge sharing among healthcare professionals. J Knowledge Manage (2023) 27(8):2017–33. doi:10.1108/jkm-06-2022-0499
44. Aalbers R, Ma R. The roles of supply networks and board interlocks in firms’ technological entry and exit: evidence from the Chinese automotive industry. Manage Organ Rev (2023) 19(2):279–315. doi:10.1017/mor.2023.5
45. Brennecke J, Rank O. The firm’s knowledge network and the transfer of advice among corporate inventors—a multilevel network study. Res Pol (2017) 46(4):768–83. doi:10.1016/j.respol.2017.02.002
46. Li Y, Zhang Y, Lee C-C, Li J. Structural characteristics and determinants of an international green technological collaboration network. J Clean Prod (2021) 324:129258. doi:10.1016/j.jclepro.2021.129258
47. Petersen AM, Fortunato S, Pan RK, Kaski K, Penner O, Rungi A, et al. Reputation and impact in academic careers. Proc Natl Acad Sci (2014) 111(43):15316–21. doi:10.1073/pnas.1323111111
48. Dibiaggio L, Nasiriyar M, Nesta L. Substitutability and complementarity of technological knowledge and the inventive performance of semiconductor companies. Res Pol (2014) 43(9):1582–93. doi:10.1016/j.respol.2014.04.001
49. Guan J, Liu N. Invention profiles and uneven growth in the field of emerging nano-energy. Energy Policy (2015) 76:146–57. doi:10.1016/j.enpol.2014.11.024
50. Khan MQ, Shahid A, Uddin MI, Roman M, Alharbi A, Alosaimi W, et al. Impact analysis of keyword extraction using contextual word embedding. PeerJ Comput Sci (2022) 8:e967. doi:10.7717/peerj-cs.967
51. Zhai Y, Ding Y, Zhang H. Innovation adoption: broadcasting versus virality. J Assoc Inf Sci Technol (2021) 72(4):403–16. doi:10.1002/asi.24420
52. Grant RM. Toward a knowledge-based theory of the firm. Strateg Manage J (1996) 17(S2):109–22. doi:10.1002/smj.4250171110
53. Yayavaram S, Srivastava MK, Sarkar M. Role of search for domain knowledge and architectural knowledge in alliance partner selection. Strateg Manage J (2018) 39(8):2277–302. doi:10.1002/smj.2791
54. Wang C, Rodan S, Fruin M, Xu X. Knowledge networks, collaboration networks, and exploratory innovation. Acad Manage J (2014) 57(2):484–514. doi:10.5465/amj.2011.0917
55. P Sharma, and Y Li, editors. Self-supervised contextual keyword and keyphrase retrieval with self-labelling (2019).
56. Beltagy I, Cohan A, Lo K. SciBERT: pretrained contextualized embeddings for scientific text. ArXiv. 2019;abs/1903.10676.
57. Fleming L. Recombinant uncertainty in technological search. Manage Sci (2001) 47(1):117–32. doi:10.1287/mnsc.47.1.117.10671
58. Garud R, Kumaraswamy A. Vicious and virtuous circles in the management of knowledge: the case of infosys technologies. MIS Q (2005) 29(1):9–33. doi:10.2307/25148666
59. Jin Q, Chen H, Wang X, Xiong F. How do network embeddedness and knowledge stock influence collaboration dynamics? Evidence from patents. J Informetrics (2024) 18(4):101553. doi:10.1016/j.joi.2024.101553
60. Lian X, Guo Y, Su J. Technology stocks: a study on the characteristics that help transfer public research to industry. Res Pol (2021) 50(10):104361. doi:10.1016/j.respol.2021.104361
62. Reuer JJ, Tong TW, Tyler BB, Ariño A. Executive preferences for governance modes and exchange partners: an information economics perspective. Strateg Manage J (2013) 34(9):1104–22. doi:10.1002/smj.2064
63. Reinholt M, Pedersen T, Foss NJ. Why a central network position isn't enough: the role of motivation and ability for knowledge sharing in employee networks. Acad Manage J (2011) 54(6):1277–97. doi:10.5465/amj.2009.0007
64. Carnabuci G, Operti E. Where do firms' recombinant capabilities come from? Intraorganizational networks, knowledge, and firms' ability to innovate through technological recombination. Strateg Manage J (2013) 34(13):1591–613. doi:10.1002/smj.2084
65. Schillebeeckx SJD, Lin Y, George G, Alnuaimi T. Knowledge recombination and inventor networks: the asymmetric effects of embeddedness on knowledge reuse and impact. J Manage (2021) 47(4):838–66. doi:10.1177/0149206320906865
66. Fronzetti Colladon A, Guardabascio B, Venturini F. A new mapping of technological interdependence. Res Pol (2025) 54(1):105126. doi:10.1016/j.respol.2024.105126
67. Snijders TAB, van de Bunt GG, Steglich CEG. Introduction to stochastic actor-based models for network dynamics. Social Networks (2010) 32(1):44–60. doi:10.1016/j.socnet.2009.02.004
68. Chen H, Mehra A, Tasselli S, Borgatti S. Network dynamics and organizations: a review and research agenda. J Manage (2022) 48:1602–60. doi:10.1177/01492063211063218
69. Block P, Stadtfeld C, Snijders TAB. Forms of dependence: comparing SAOMs and ERGMs from basic principles. Sociological Methods and Res (2019) 48(1):202–39. doi:10.1177/0049124116672680
70. Snijders TAB. Stochastic actor-oriented models for network dynamics. Annu Rev Stat Its Appl (2017) 4:343–63. doi:10.1146/annurev-statistics-060116-054035
72. Omenn GS, Lane L, Overall CM, Pineau C, Packer NH, Cristea IM, et al. The 2022 report on the human Proteome from the HUPO human Proteome project. J Proteome Res (2023) 22(4):1024–42. doi:10.1021/acs.jproteome.2c00498
73. Tong TW, Reuer JJ, Peng MW. International joint ventures and the value of growth options. Acad Manage J (2008) 51:1014–29. doi:10.5465/amj.2008.34789680
74. Bornmann L. Interrater reliability and convergent validity of F1000Prime peer review. J Assoc Inf Sci Technol (2015) 66(12):2415–26. doi:10.1002/asi.23334
75. Chen H, Song X, Jin Q, Wang X. Network dynamics in university-industry collaboration: a collaboration-knowledge dual-layer network perspective. Scientometrics (2022) 127(11):6637–60. doi:10.1007/s11192-022-04330-9
76. Taylor A, Greve HR. Superman or the fantastic four? Knowledge combination and experience in innovative teams. Acad Manage J (2006) 49:723–40. doi:10.5465/amj.2006.22083029
77. Melin G. Pragmatism and self-organization: research collaboration on the individual level. Res Pol (2000) 29(1):31–40. doi:10.1016/s0048-7333(99)00031-1
Keywords: scientific innovation, complex network, network dynamics, stochastic actor-oriented model, collaboration network, knowledge network
Citation: Jia Y, Chen H, Liu J, Wang X, Guo R and Wang X (2025) Exploring network dynamics in scientific innovation: collaboration, knowledge combination, and innovative performance. Front. Phys. 12:1492731. doi: 10.3389/fphy.2024.1492731
Received: 07 September 2024; Accepted: 21 November 2024;
Published: 03 January 2025.
Edited by:
Xuzhen Zhu, Beijing University of Posts and Telecommunications (BUPT), ChinaReviewed by:
Aurelio Patelli, Enrico Fermi Center for Study and Research, ItalySiming Deng, Dalian University of Technology, China
Zhongjin Li, Hangzhou Dianzi University, China
Copyright © 2025 Jia, Chen, Liu, Wang, Guo and Wang. This is an open-access article distributed under the terms of the Creative Commons Attribution License (CC BY). The use, distribution or reproduction in other forums is permitted, provided the original author(s) and the copyright owner(s) are credited and that the original publication in this journal is cited, in accordance with accepted academic practice. No use, distribution or reproduction is permitted which does not comply with these terms.
*Correspondence: Hongshu Chen, aG9uZ3NodS5jaGVuQGJpdC5lZHUuY24=